import torch
import torch.nn as nn
import torch.nn.functional as F
class VGG16(nn.Module):
def __init__(self):
super(VGG16, self).__init__()
self.conv1_1 = nn.Conv2d(3, 64, 3)
self.conv1_2 = nn.Conv2d(64, 64, 3, padding=(1, 1))
self.maxpool1 = nn.MaxPool2d((2, 2), padding=(1, 1))
self.conv2_1 = nn.Conv2d(64, 128, 3)
self.conv2_2 = nn.Conv2d(128, 128, 3, padding=(1, 1))
self.maxpool2 = nn.MaxPool2d((2, 2), padding=(1, 1))
self.conv3_1 = nn.Conv2d(128, 256, 3)
self.conv3_2 = nn.Conv2d(256, 256, 3, padding=(1, 1))
self.conv3_3 = nn.Conv2d(256, 256, 3, padding=(1, 1))
self.maxpool3 = nn.MaxPool2d((2, 2), padding=(1, 1))
self.conv4_1 = nn.Conv2d(256, 512, 3)
self.conv4_2 = nn.Conv2d(512, 512, 3, padding=(1, 1))
self.conv4_3 = nn.Conv2d(512, 512, 3, padding=(1, 1))
self.maxpool4 = nn.MaxPool2d((2, 2), padding=(1, 1))
self.conv5_1 = nn.Conv2d(512, 512, 3)
self.conv5_2 = nn.Conv2d(512, 512, 3, padding=(1, 1))
self.conv5_3 = nn.Conv2d(512, 512, 3, padding=(1, 1))
self.maxpool5 = nn.MaxPool2d((2, 2), padding=(1, 1))
self.fc1 = nn.Linear(512 * 7 * 7, 4096)
self.fc2 = nn.Linear(4096, 4096)
self.fc3 = nn.Linear(4096, 1000)
def forward(self, x):
in_size = x.size(0)
out = self.conv1_1(x)
out = F.relu(out)
out = self.conv1_2(out)
out = F.relu(out)
out = self.maxpool1(out)
out = self.conv2_1(out)
out = F.relu(out)
out = self.conv2_2(out)
out = F.relu(out)
out = self.maxpool2(out)
out = self.conv3_1(out)
out = F.relu(out)
out = self.conv3_2(out)
out = F.relu(out)
out = self.conv3_3(out)
out = F.relu(out)
out = self.maxpool3(out)
out = self.conv4_1(out)
out = F.relu(out)
out = self.conv4_2(out)
out = F.relu(out)
out = self.conv4_3(out)
out = F.relu(out)
out = self.maxpool4(out)
out = self.conv5_1(out)
out = F.relu(out)
out = self.conv5_2(out)
out = F.relu(out)
out = self.conv5_3(out)
out = F.relu(out)
out = self.maxpool5(out)
out = out.view(in_size, -1)
out = self.fc1(out)
out = F.relu(out)
out = self.fc2(out)
out = F.relu(out)
out = self.fc3(out)
out = F.log_softmax(out, dim=1)
return out
- 输入图像尺寸为224x224x3,经64个通道为3的3x3的卷积核,步长为1,padding=same填充,卷积两次,再经ReLU激活,输出的尺寸大小为224x224x64
- 经max pooling(最大化池化),滤波器为2x2,步长为2,图像尺寸减半,池化后的尺寸变为112x112x64
- 经128个3x3的卷积核,两次卷积,ReLU激活,尺寸变为112x112x128
- max pooling池化,尺寸变为56x56x128
- 经256个3x3的卷积核,三次卷积,ReLU激活,尺寸变为56x56x256
- max pooling池化,尺寸变为28x28x256
- 经512个3x3的卷积核,三次卷积,ReLU激活,尺寸变为28x28x512
- max pooling池化,尺寸变为14x14x512
- 经512个3x3的卷积核,三次卷积,ReLU,尺寸变为14x14x512
- max pooling池化,尺寸变为7x7x512
- 然后view(),将数据拉平成向量,变成一维51277=25088。
- 再经过两层1x1x4096,一层1x1x1000的全连接层(共三层),经ReLU激活
- 最后通过softmax输出1000个预测结果
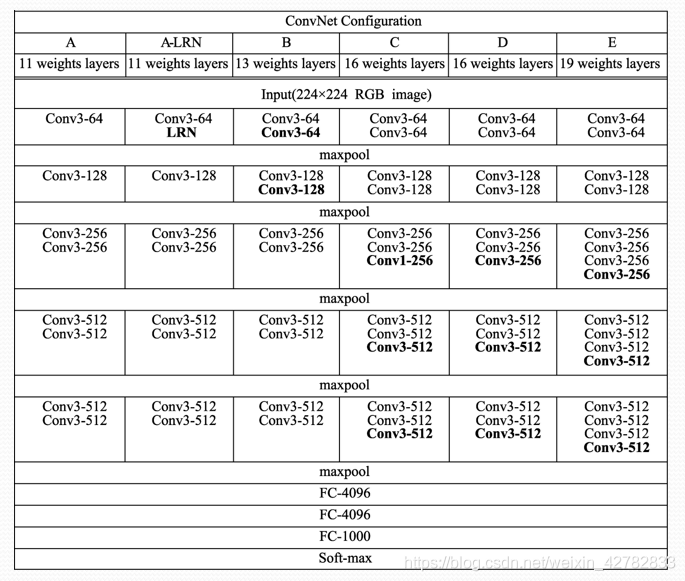