1
Introduction
spectral graph theory
graph signal processing (GSP)
Fourier basis
eigenvalue decomposition (EVD)
2
Proposed Technique
Generalizing CNNs to graphs requires three fundamental steps:
(i) the design of localized convolutional fifilters on graphs
(ii) a graph coarsening procedure that groups together similar vertices
(iii) a graph pooling operation that trades spatial resolution for higher fifilter resolution.
2.1
Learning Fast Localized Spectral Filters
There are two strategies to defifine convolutional fifilters; either from a spatial approach or from a spectral approach.
Graph Fourier Transform




A signal
x : V → R defined on the nodes of the graph may be regarded as a vector

x
i
is the value of
x
at the
i
th node
,
,
(这个D也就是每个点有几条边,加权)
L标准化定义:


As L is a real symmetric positive semidefifinite matrix(实对称半正定矩阵
),it has a complete set of orthonormal eigenvectors
(一组完全正交的特征向量),known as the graph Fourier modes, and their associated ordered real nonnegative eigenvalues
(相关的有序实非负特征值),
identified as the frequencies of the graph.
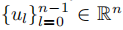

L分解:
,
,



所以:把一个信号x的图傅里叶变换定义为:

反过来:

Spectral fifiltering of graph signals




Polynomial parametrization for localized fifilters
non-parametric fifilters两个局限:
(i) they are not localized in space
(ii) their learning complexity is in
O
(
n
), the dimensionality of the data.
所以,用下面这个
polynomial fifilter
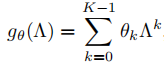

(可能内容不全)