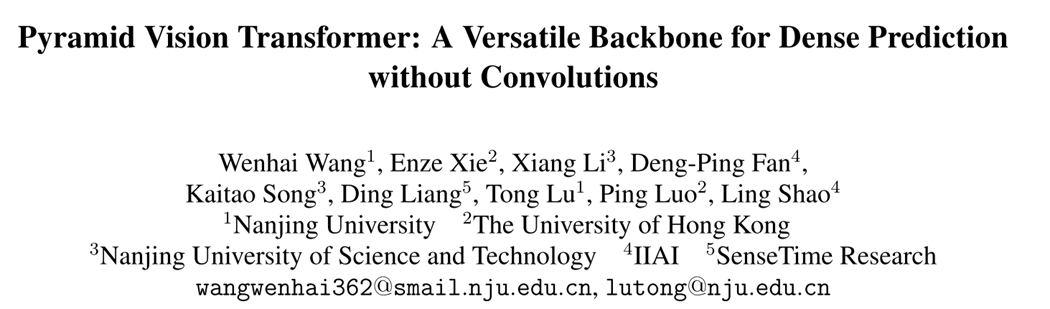
paper: https://arxiv.org/abs/2102.12122
code: https://github.com/whai362/PVT/
1. Motivation
- 作者想实现一个纯净的,无卷积操作的transformer backbone,用于稠密任务。
As far as we know, exploring a clean and convolution-free Transformer backbone to address dense prediction tasks in computer vision is rarely studied.
- VIT的局限性,输入的特征图的分辨率较低,并且stride只能是16或者32,计算和内存开销都很大。
As far as we know, exploring a clean and convolution-free Transformer backbone to address dense prediction tasks in computer vision is rarely studied.
- (1) its output feature map has only a single scale with low resolution
- (2) its computations and memory cost are relatively high even for common input image size
图1为CNN网络,VIT网络以及作者提出的PVT,3种backbone的对比。
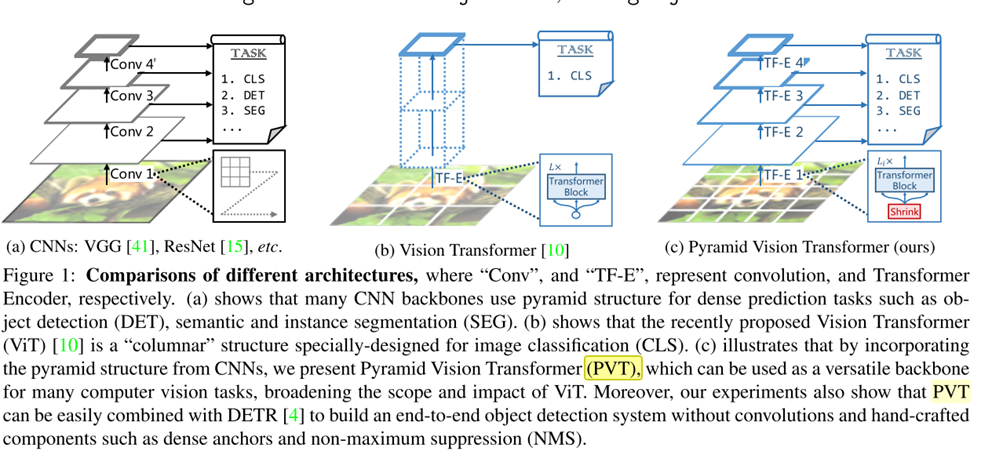
2. Contribution
- 本文提出了PVY金字塔视觉transformer,作为多种像素级别的任务的backbone,并且是不需要卷积的。
We propose Pyramid Vision Transformer (PVT), which is the first backbone designed for various pixel-level dense prediction tasks without convolutions. Combining PVT and DETR, we can build an end-to-end object detection system without convolutions and hand-crafted components such as dense anchors and non-maximum(NMS).
- PVT中实现了渐进收缩金字塔progressive shrinking pyramid以及 空间维度降维attention(SRA),提出的新结构可以用来减少资源的消耗,使得PVT对学习多尺度以及高分辨率的featmap更加的灵活,从而来实现dense predictions(典型工作就是检测和分割)。
We overcome many difficulties when porting Transformer to dense pixel-level predictions, by designing progressive shrinking pyramid and spatial-reduction attention (SRA), which are able to reduce the resource consumption of using Transformer, making PVT flexible to learn multi-scale and high-resolution feature maps.
- PVT可以应用于图像分类,目标检测,语义分割,实例分割等等。
We verify PVT by applying it to many different tasks, e.g., image classification, object detection, and semantic segmentation.
3. Method
3.1 Overall Architecture
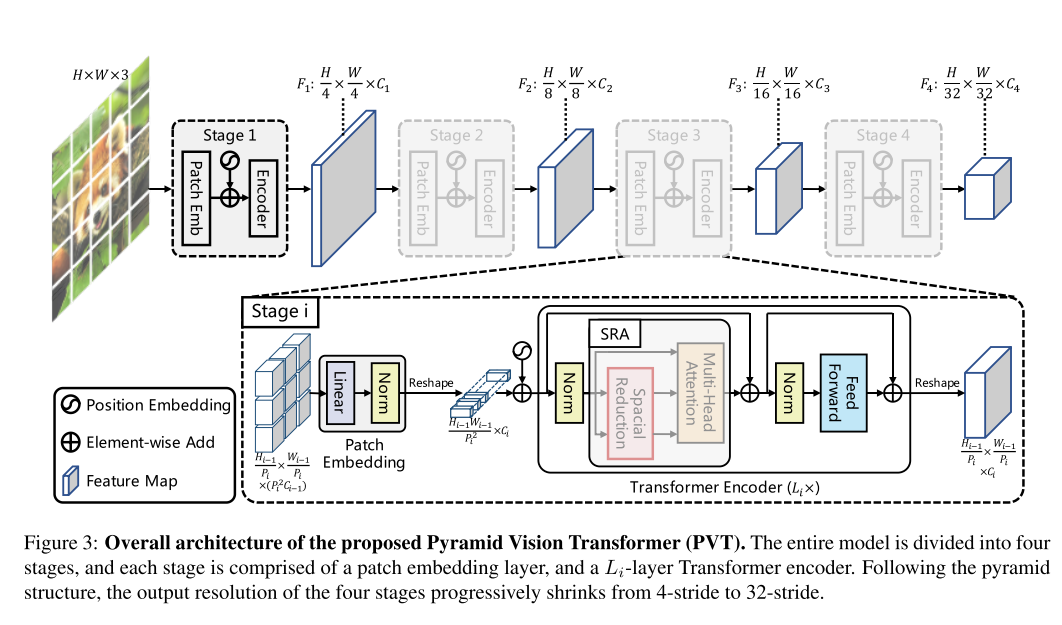
3.2 Feature Pyramid for Transformer
首先对于 H × W × 3 H \times W \times 3 H×W×3,首先根据patch size=4,分为16个patch,为了不改变图像带下,就将图像变为 H i − 1 P i × H i − 1 P i × ( P i 2 C i − 1 ) = H i − 1 4 × H i − 1 4 × ( 4 × 4 × 3 ) \frac{H_{i-1}}{P_i}\times \frac{H_{i-1}}{P_i} \times (P_i^2C_{i-1})=\frac{H_{i-1}}{4}\times \frac{H_{i-1}}{4} \times (4\times 4 \times 3) PiHi−1×PiHi−1×(Pi2Ci−1)=4Hi−1×4