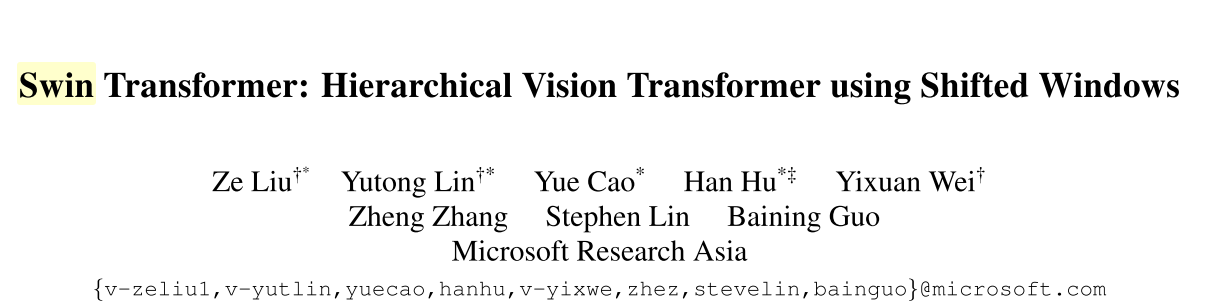
1. Motivation
将transformer从NLP应用于CV领域存在以下2个方面的挑战,图像尺度的多样性,以及图像像素相对于words的高分辨率,这会造成内存大的花销。
Challenges in adapting Transformer from language to vision arise from differences between the two domains, such as large variations in the scale of visual entities and the high resolution of pixels in images compared to words in text.
本文中作者希望扩大transformer的适用范围,使得它可以作为一个通用的backbone,应用于CV领域。
In this paper, we seek to expand the applicability of Transformer such that it can serve as a general-purpose backbone for computer vision, as it does for NLP and as CNNs do in vision.
图1表示了VIT与本文提出的Swin Transformer的区别。
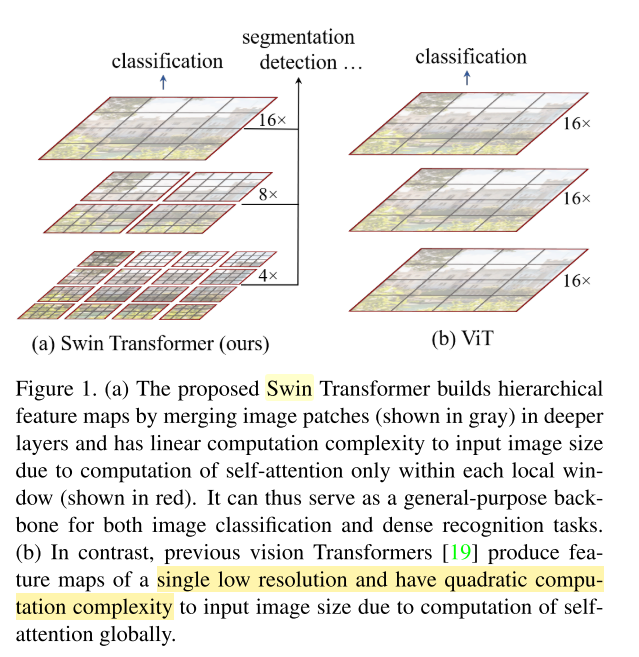
2. Contribution
为了解决以上2种问题,作者提出了Swin Transformer,用于构建分层hierarchical的,移动窗口的视觉transformer backbone。并且在目标检测,实例分割,图像分类,语义分割上都取得SOTA的结果。
3. Method
3.1 Overall Architecture
如图3,Swin Transformer的整体结构,其中包含了VIT中的patch partition linear embedding以及patch merging,所不同的是提出了一个Swin Transformer Block,其中包含了W-MSA,以及基于shifted的SW-MSA,外加一些LN和MLP,残差的常规操作。
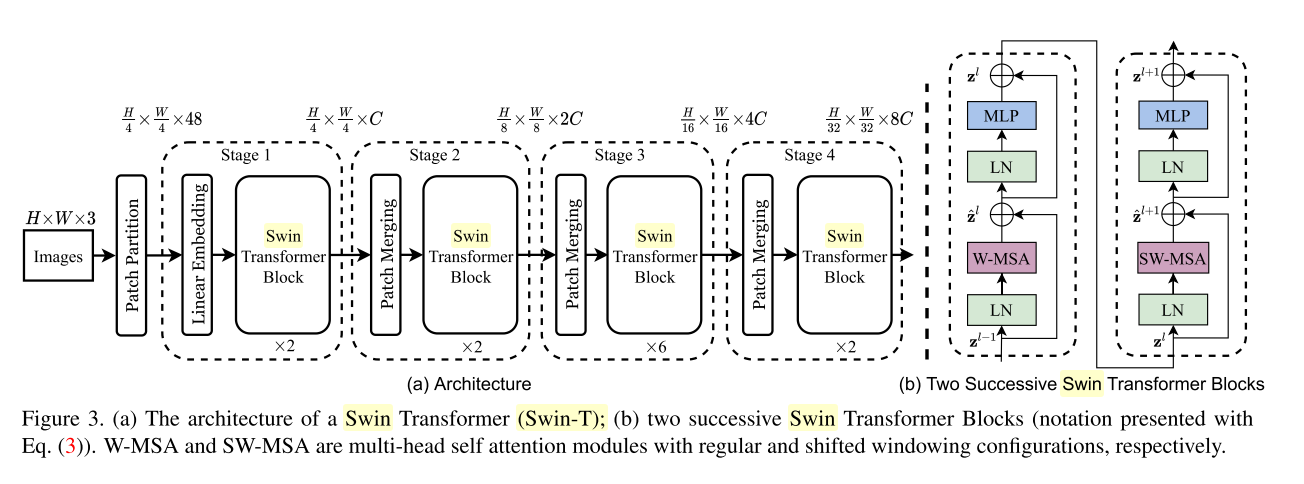
3.2 Shifted Window based Self-Attention
3.2.1 Self-attention in non-overlapped windows
MSA与W-MSA之间的时间复杂度比较。

3.2.2 Shifted window partitioning in successive blocks
基于窗口的self-atte缺乏窗口之间的联系,因此为了得到cross-window的联系,同时保留高效的非重叠的窗口计算代价,作者引入了shifted window partition 方法。
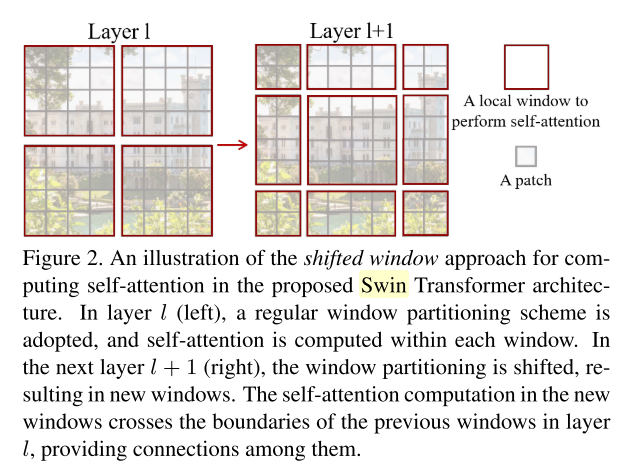
successive transformer block中的W-MSA以及SW-MSA的公式如下:
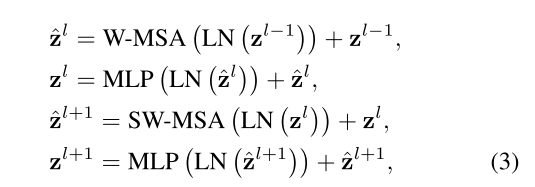
3.3. Efficient batch computation for shifted configuratio
由于移动窗口之后,windows的数量会从h/M x w/M变为(h/M+1) x (w/M+1),并且有些windows的大小是小于MxM的,作者为了减少计算开销,提出了一个更高效的方法,如图4所示,cyclic-shifting towards the topleft direction。这样子做每个batched window(理解为整个大window)由feature map中不邻近(adjacent)的sub-windows组成,然后使用masking mechanism来限制每一个sub-window的self-attn,这样子就不用将小的windows pad填充为MxM的windows了。
the number of batched windows remains the same as that of regular window partitioning,
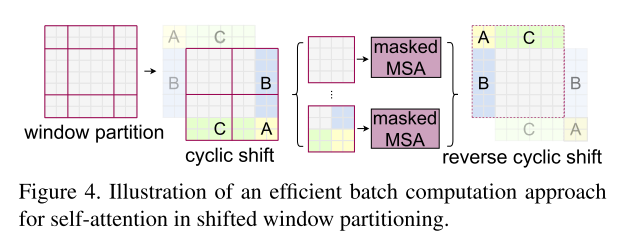
3.3.4 Relative position bias
在计算self-atte时,作者加入了 relative position bias, Q , K , V ∈ R M 2 × d , Q,K,V \in R^{M^2 \times d}, Q,K,V∈RM2×d, B ∈ R M 2 × M 2 B \in R^{M^2 \times M^2} B∈RM2×M2,来计算每一个head之间的相似性,如公式4所示:

因此,相对坐标的范围为:[-M+1, M-1],(跟relative position embedding那篇文章是一样的思路),注意这里是类似算一个(x,y),例如,对于(0,0)这个pixel,(3,3)到原点的距离为(3,3),也就是没有将二维坐标flat操作,因此构建出realtive position bias matrix B ^ ∈ R ( 2 M − 1 ) × ( 2 M − 1 ) \hat B \in R^{(2M-1)\times (2M-1)} B^∈R(2M−1)×(2M−1)。代码示例如下:
def get_relative_distances(window_size):
indices = torch.tensor(np.array([[x, y] for x in range(window_size) for y in range(window_size)])) # [window_size ** 2, 2]
distances = indices[None, :, :] - indices[:, None, :] # [window_size ** 2 , window_size ** 2, 2]
return distances
class WindowAttention(nn.Module):
def __init__(self, dim, heads, head_dim, shifted, window_size, relative_pos_embedding):
super().__init__()
....
....
if self.relative_pos_embedding:
self.relative_indices = get_relative_distances(window_size) + window_size - 1 # 归一化为正数 [value+ M-1] max value add from (M-1) to (M-1 + M-1), i.e. 2M-1 in one axis, [ 2M-1, 2M-1] in [x,y] axis.
self.pos_embedding = nn.Parameter(torch.randn(2 * window_size - 1, 2 * window_size - 1)) # [2M-1, 2M-1]
else:
self.pos_embedding = nn.Parameter(torch.randn(window_size ** 2, window_size ** 2))
self.to_out = nn.Linear(inner_dim, dim)
4. Experiments
4.1. Image Classification on ImageNet-1K
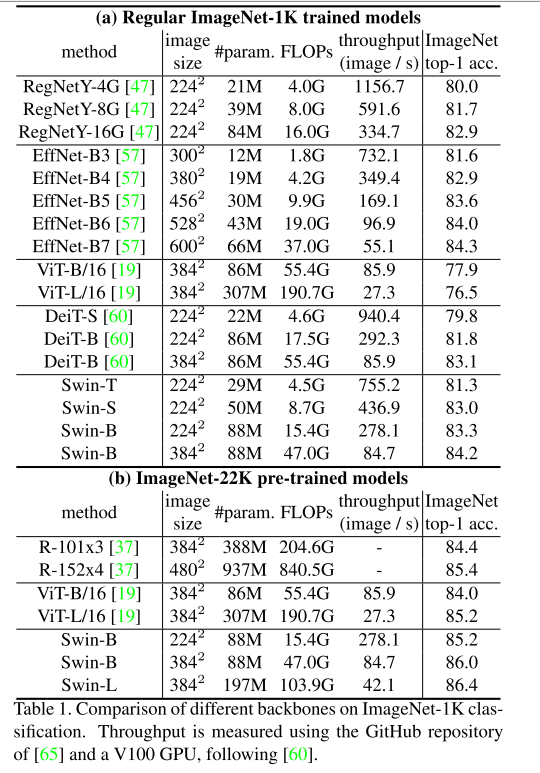
4.2. Object Detection on COCO
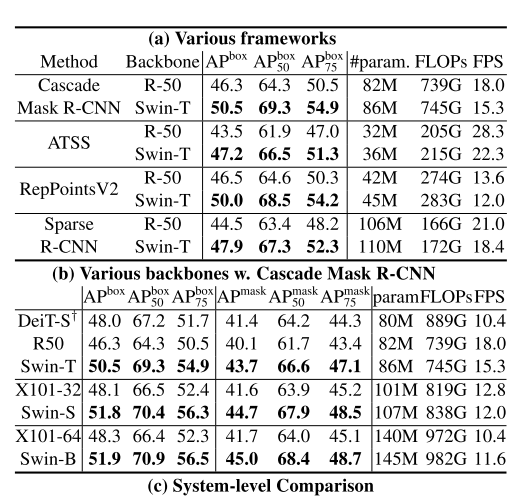
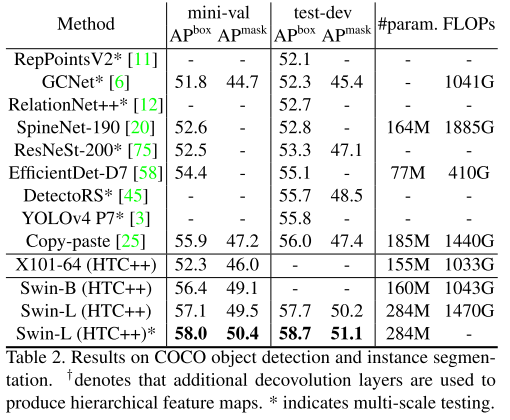
4.3. Semantic Segmentation on ADE20K
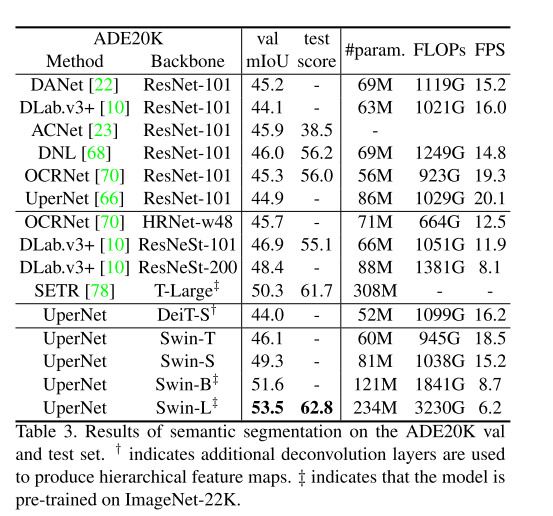
4.4. Ablation Study
4.4.1 Shifted Windows Relative position bias
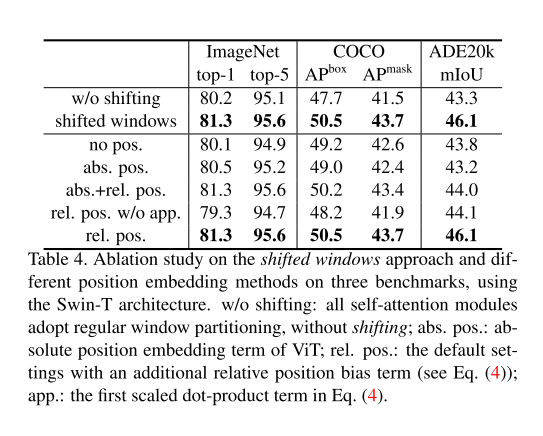
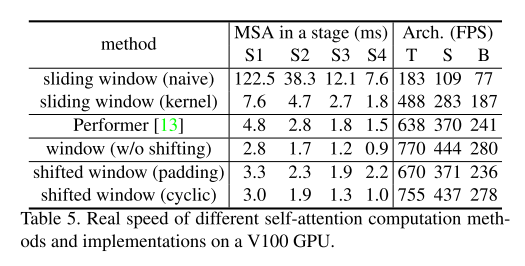
### 4.4.2 Different self-attention methods
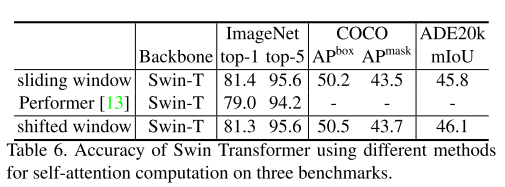