#!/usr/bin/env python
'''
Affine invariant feature-based image matching sample.
This sample is similar to find_obj.py, but uses the affine transformation
space sampling technique, called ASIFT [1]. While the original implementation
is based on SIFT, you can try to use SURF or ORB detectors instead. Homography RANSAC
is used to reject outliers. Threading is used for faster affine sampling.
[1] http://www.ipol.im/pub/algo/my_affine_sift/
USAGE
asift.py [--feature=<sift|surf|orb|brisk>[-flann]] [ <image1> <image2> ]
--feature - Feature to use. Can be sift, surf, orb or brisk. Append '-flann'
to feature name to use Flann-based matcher instead bruteforce.
Press left mouse button on a feature point to see its matching point.
'''
# Python 2/3 compatibility
from __future__ import print_function
import numpy as np
import cv2
# built-in modules
import itertools as it
from multiprocessing.pool import ThreadPool
# local modules
from common import Timer
from find_obj import init_feature, filter_matches, explore_match
def affine_skew(tilt, phi, img, mask=None):
'''
affine_skew(tilt, phi, img, mask=None) -> skew_img, skew_mask, Ai
Ai - is an affine transform matrix from skew_img to img
'''
h, w = img.shape[:2]
if mask is None:
mask = np.zeros((h, w), np.uint8)
mask[:] =
使用SIFT实现图像配准
最新推荐文章于 2022-12-19 14:49:33 发布
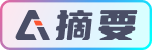