3.pandas
pandas的常用数据类型
- Series 一维,带标签数组
- DataFrame 二维,Series容器
pandas 显示所有行列
pd.set_option('display.max_columns', None)
#显示所有行
pd.set_option('display.max_rows', None)
pandas之读取外部数据
我们的这组数据存在csv中,我们直接使用pd. read_csv即可
和我们想象的有些差别,我们以为他会是一个Series类型,但是他是一个DataFrame,那么接下来我们就来了解这种数据类型
但是,还有一个问题:
对于数据库比如mysql或者mongodb中数据我们如何使用呢?
pd.read_sql(sql_sentence,connection)
1.Series
1.创建
In [59]: import string
In [60]: t = pd.Series(np.arange(10), index=list(string.ascii_uppercase[:10]))
In [61]: t
Out[61]:
A 0
B 1
C 2
D 3
E 4
F 5
G 6
H 7
I 8
J 9
dtype: int64
In [62]: type(t)
Out[62]: pandas.core.series.Series
In [63]: a = {string.ascii_uppercase[i]:i for i in range(10)}
In [64]: a
Out[64]:
{'A': 0,
'B': 1,
'C': 2,
'D': 3,
'E': 4,
'F': 5,
'G': 6,
'H': 7,
'I': 8,
'J': 9}
In [65]: pd.Series(a)
Out[65]:
A 0
B 1
C 2
D 3
E 4
F 5
G 6
H 7
I 8
J 9
dtype: int64
# nan 在pandas 中是浮点型
In [67]: t = pd.Series(a, index=list(string.ascii_uppercase[5:20]))
In [68]: t
Out[68]:
F 5.0
G 6.0
H 7.0
I 8.0
J 9.0
K NaN
L NaN
M NaN
N NaN
O NaN
P NaN
Q NaN
R NaN
S NaN
T NaN
dtype: float64
2.切片和索引
In [71]: t
Out[71]:
A 0
B 1
C 2
D 3
E 4
F 5
G 6
H 7
I 8
J 9
dtype: int64
In [72]: t[2:10:2]
Out[72]:
C 2
E 4
G 6
I 8
dtype: int64
In [73]: t[2]
Out[73]: 2
In [76]: t[[2,3,6]]
Out[76]:
C 2
D 3
G 6
dtype: int64
In [77]: t[t>4]
Out[77]:
F 5
G 6
H 7
I 8
J 9
dtype: int64
In [78]: t["F"]
Out[78]: 5
In [79]: t[["A","F"]]
Out[79]:
A 0
F 5
dtype: int64
切片:直接传入start end 或者步长
索引:一个的时候直接传入序号或者index,多个的时候传入序号或者index的列表
3.索引和值
In [80]: t.index
Out[80]: Index(['A', 'B', 'C', 'D', 'E', 'F', 'G', 'H', 'I', 'J'], dtype='object')
In [81]: t.values
Out[81]: array([0, 1, 2, 3, 4, 5, 6, 7, 8, 9])
In [82]: type(t.index)
Out[82]: pandas.core.indexes.base.Index
In [83]: type(t.valuse)
Out[85]: numpy.ndarray
Series 本质上由两个数组组成,一个数组构成对象的键(index,索引),一个数组构成对象的值(values),键->值
ndarray的很多方法都可以运用于Series类型,比如argmax, clip
Series有where方法,但是和ndarray不同
2.DataFrame
In [86]: t=pd.DataFrame(np.arange(12).reshape((3, 4)))
In [87]: t
Out[87]:
0 1 2 3
0 0 1 2 3
1 4 5 6 7
2 8 9 10 11
DataFrame对象既有行索引,又有列索引
行索引,表明不同行,横向索引,叫index,0轴,axis=0
列索引,表名不同列,纵向索引,叫columns,1轴,axis=1
DataFrame基础属性
In [89]: df.shape 行数 列数
Out[89]: (3, 4)
In [90]: df.dtypes # 列数据类型
Out[90]:
0 int64
1 int64
2 int64
3 int64
dtype: object
In [91]: df.ndim # 数组维度
Out[91]: 2
In [92]: df.columns # 列索引
Out[92]: RangeIndex(start=0, stop=4, step=1)
In [93]: df.values # 対象值 二维ndarry数组
DataFrame整体情况查询
In [94]: df.head(2) # 头部几行
Out[94]:
0 1 2 3
0 0 1 2 3
1 4 5 6 7
In [95]: df.tail(2) # 末尾几行
Out[95]:
0 1 2 3
1 4 5 6 7
2 8 9 10 11
In [96]: df.info() # 相关信息概览:行数,列数。列索引,列非空值个数,列类型,内存占用
<class 'pandas.core.frame.DataFrame'>
RangeIndex: 3 entries, 0 to 2
Data columns (total 4 columns):
# Column Non-Null Count Dtype
--- ------ -------------- -----
0 0 3 non-null int64
1 1 3 non-null int64
2 2 3 non-null int64
3 3 3 non-null int64
dtypes: int64(4)
memory usage: 224.0 bytes
In [97]: df.describe() # 快速综合统计结果:基数,均值,标准差,最大值,四分位数,最小值
Out[97]:
0 1 2 3
count 3.0 3.0 3.0 3.0
mean 4.0 5.0 6.0 7.0
std 4.0 4.0 4.0 4.0
min 0.0 1.0 2.0 3.0
25% 2.0 3.0 4.0 5.0
50% 4.0 5.0 6.0 7.0
75% 6.0 7.0 8.0 9.0
max 8.0 9.0 10.0 11.0
1.取行或者列
df_sorted = df.sort_values(by="Count_AnimalName") # 按照字段df_sorted[:100]
2.loc
还有更多的经过pandas优化过的选择方式:
- df.loc 通过标签索引行数据
- df.iloc 通过位置获取行数据
In [99]: t = pd.DataFrame(np.arange(12).reshape((3, 4)), index=list(string.ascii_uppercase[:3]), columns=list(string.a
...: scii_uppercase[-4:]))
In [100]: t
Out[100]:
W X Y Z
A 0 1 2 3
B 4 5 6 7
C 8 9 10 11
In [101]: t.loc["A", "W"] # 选择值
Out[101]: 0
In [102]: t.loc["A", ["W", "Y"]]
Out[102]:
W 0
Y 2
Name: A, dtype: int64
In [103]: type(t.loc["A", ["W", "Y"]])
Out[103]: pandas.core.series.Series
In [104]: t.loc[["A", "C"], ["W", "Z"]] # 选择间隔的多行
Out[104]:
W Z
A 0 3
C 8 11
In [107]: t.loc["A":, ["W", "Z"]] # 选择切片
Out[107]:
W Z
A 0 3
B 4 7
C 8 11
3.iloc
In [108]: t
Out[108]:
W X Y Z
A 0 1 2 3
B 4 5 6 7
C 8 9 10 11
In [109]: t.iloc[1:3, [2,3]]
Out[109]:
Y Z
B 6 7
C 10 11
In [110]: t.iloc[1:3, 1:3]
Out[110]:
X Y
B 5 6
C 9 10
赋值修改:
In [115]: t.iloc[1:2, 0:2]=100
In [116]: t
Out[116]:
W X Y Z
A 0 1 100 3
B 100 100 6 7
C 8 9 10 11
In [111]: t.loc["A", "Y"] =100
4.布尔索引
- & 且
- | 或
注:不同条件间要用括号括起来
回到之前狗的名字的问题上,假如我们想找到所有的使用次数超过800的狗的名字,应该怎么选择?
df[df["count"]>800]
回到之前狗的名字的问题上,假如我们想找到所有的使用次数超过700并且名字的字符串的长度大于4的狗的名字,应该怎么选择?
df[(df["row_name"].str.len()>4)&(df["count"]>800)]
5.字符串方法
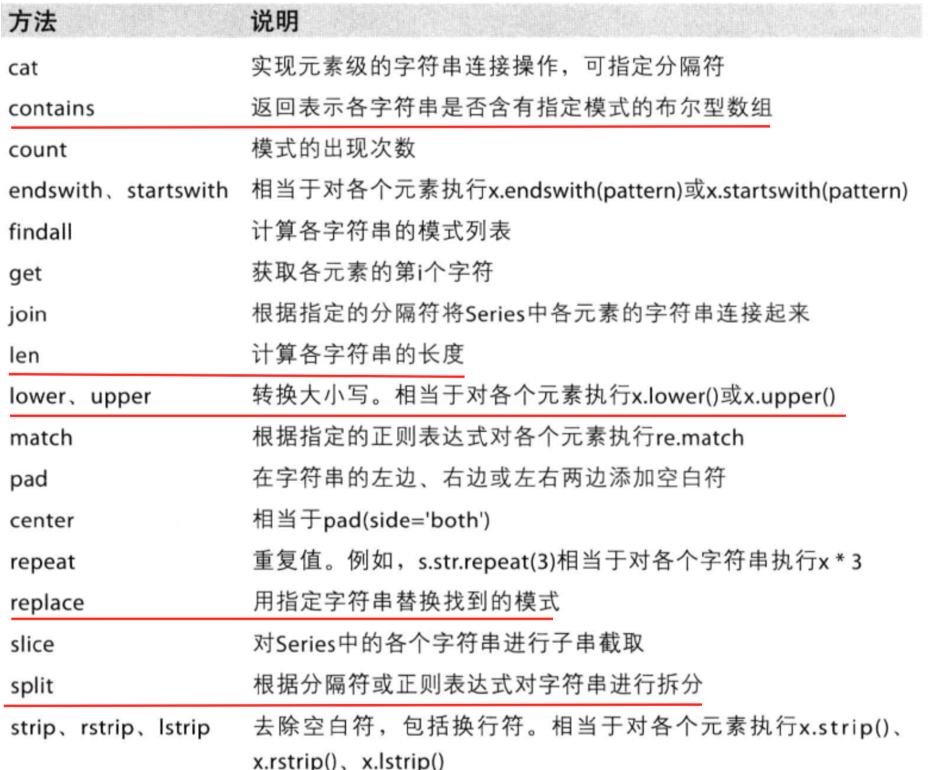
6.缺失数据的处理
对于NaN的数据,在numpy中我们是如何处理的?
在pandas中我们处理起来非常容易
判断数据是否为NaN:pd.isnull(df),pd.notnull(df)
处理方式1:删除NaN所在的行列dropna (axis=0, how=‘any’, inplace=False)
处理方式2:填充数据,t.fillna(t.mean()),t.fiallna(t.median()),t.fillna(0)
处理为0的数据:t[t==0]=np.nan
当然并不是每次为0的数据都需要处理
计算平均值等情况,nan是不参与计算的,但是0会
假设现在我们有一组从2006年到2016年1000部最流行的电影数据,我们想知道这些电影数据中评分的平均分,导演的人数等信息,我们应该怎么获取?
数据来源:https://www.kaggle.com/damianpanek/sunday-eda/data
7.pandas常用统计方法
rating_mean = df["Rating"].mean()
temp_list = df["Actors"].str.split(",").tolist()
nums = set([i for j in temp_list for i in j])
max_runtime = df["Runtime (Minutes)"].max()
max_runtime_index = df["Runtime (Minutes)"].armax()
min_runtime = df["Runtime (Minutes)"].min()
min_runtime_index = df["Runtime (Minutes)"].armin()
runtime_median = df["Runtime (Minutes)"].median()
df["mean"] = df.apply(lambda x:x.mean(), axis=1)
c = df.apply(lambda x:x.mean(), axis=0)
df1 = df.append(c, ignore_index=True)
df1.reindex()
print(df1)
a b c d mean
0 0.0 1.0 2.0 3.0 1.5
1 4.0 5.0 6.0 7.0 5.5
2 8.0 9.0 10.0 11.0 9.5
3 4.0 5.0 6.0 7.0 5.5
8.数据合并之join
join:默认情况下他是把行索引相同的数据合并到一起
In [35]: t
Out[35]:
q w e r t
a 0 1 2 3 4
b 5 6 7 8 9
c 10 11 12 13 14
d 15 16 17 18 19
In [36]: t1 = pd.DataFrame(np.arange(20).reshape((5, 4)), index=["a", "b", "c", "d", "e"], columns=["1", "2",
...: "3", "4"])
In [37]: t1
Out[37]:
1 2 3 4
a 0 1 2 3
b 4 5 6 7
c 8 9 10 11
d 12 13 14 15
e 16 17 18 19
In [38]: t
Out[38]:
q w e r t
a 0 1 2 3 4
b 5 6 7 8 9
c 10 11 12 13 14
d 15 16 17 18 19
In [39]: t1.join(t)
Out[39]:
1 2 3 4 q w e r t
a 0 1 2 3 0.0 1.0 2.0 3.0 4.0
b 4 5 6 7 5.0 6.0 7.0 8.0 9.0
c 8 9 10 11 10.0 11.0 12.0 13.0 14.0
d 12 13 14 15 15.0 16.0 17.0 18.0 19.0
e 16 17 18 19 NaN NaN NaN NaN NaN
In [40]: t.join(t1)
Out[40]:
q w e r t 1 2 3 4
a 0 1 2 3 4 0 1 2 3
b 5 6 7 8 9 4 5 6 7
c 10 11 12 13 14 8 9 10 11
d 15 16 17 18 19 12 13 14 15
9.数据合并之merge
In [41]: t
Out[41]:
q w e r t
a 0 1 2 3 4
b 5 6 7 8 9
c 10 11 12 13 14
d 15 16 17 18 19
In [42]: t1
Out[42]:
1 2 3 4
a 0 1 2 3
b 4 5 6 7
c 8 9 10 11
d 12 13 14 15
e 16 17 18 19
In [43]: t.merge(t1, left_on='r', right_on='4') # 默认的合并方式inner,并集
Out[43]:
q w e r t 1 2 3 4
0 0 1 2 3 4 0 1 2 3
In [44]: t.merge(t1, left_on='r', right_on='4', how='inner')
Out[44]:
q w e r t 1 2 3 4
0 0 1 2 3 4 0 1 2 3
In [45]: t.merge(t1, left_on='r', right_on='4', how='outer') # merge outer,交集,NaN补全
Out[45]:
q w e r t 1 2 3 4
0 0.0 1.0 2.0 3.0 4.0 0.0 1.0 2.0 3.0
1 5.0 6.0 7.0 8.0 9.0 NaN NaN NaN NaN
2 10.0 11.0 12.0 13.0 14.0 NaN NaN NaN NaN
3 15.0 16.0 17.0 18.0 19.0 NaN NaN NaN NaN
4 NaN NaN NaN NaN NaN 4.0 5.0 6.0 7.0
5 NaN NaN NaN NaN NaN 8.0 9.0 10.0 11.0
6 NaN NaN NaN NaN NaN 12.0 13.0 14.0 15.0
7 NaN NaN NaN NaN NaN 16.0 17.0 18.0 19.0
In [46]: t.merge(t1, left_on='r', right_on='4', how='left') # merge left,左边为准,NaN补全
Out[46]:
q w e r t 1 2 3 4
0 0 1 2 3 4 0.0 1.0 2.0 3.0
1 5 6 7 8 9 NaN NaN NaN NaN
2 10 11 12 13 14 NaN NaN NaN NaN
3 15 16 17 18 19 NaN NaN NaN NaN
In [47]: t.merge(t1, left_on='r', right_on='4', how='right') # merge right,右边为准,NaN补全
Out[47]:
q w e r t 1 2 3 4
0 0.0 1.0 2.0 3.0 4.0 0 1 2 3
1 NaN NaN NaN NaN NaN 4 5 6 7
2 NaN NaN NaN NaN NaN 8 9 10 11
3 NaN NaN NaN NaN NaN 12 13 14 15
4 NaN NaN NaN NaN NaN 16 17 18 19
现在我们有一组关于全球星巴克店铺的统计数据,如果我想知道美国的星巴克数量和中国的哪个多,或者我想知道中国每个省份星巴克的数量的情况,那么应该怎么办?
思路:遍历一遍,每次加1 ???
数据来源:https://www.kaggle.com/starbucks/store-locations/data
10.分组和聚合
grouped = df.groupby(by=“columns_name”)
grouped是一个DataFrameGroupBy对象,是可迭代的
grouped中的每一个元素是一个元组
元组里面是(索引(分组的值),分组之后的DataFrame)
DataFrameGroupBy对象有很多经过优化的方法
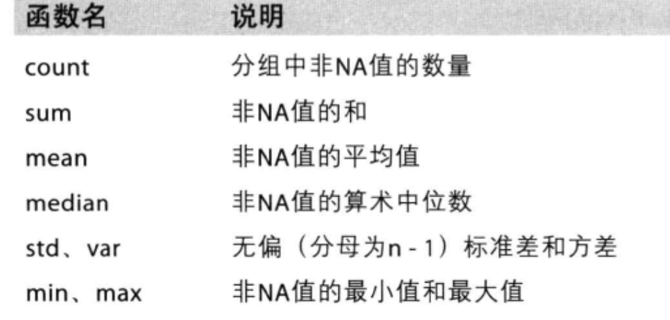
如果我们需要对国家和省份进行分组统计,应该怎么操作呢?
grouped = df.groupby(by=[df[“Country”],df[“State/Province”]])
很多时候我们只希望对获取分组之后的某一部分数据,或者说我们只希望对某几列数据进行分组,这个时候我们应该怎么办呢?
获取分组之后的某一部分数据:
df.groupby(by=[“Country”,“State/Province”])[“Country”].count()
对某几列数据进行分组:
df[“Country”].groupby(by=[df[“Country”],df[“State/Province”]]).count()
观察结果,由于只选择了一列数据,所以结果是一个Series类型
如果我想返回一个DataFrame类型呢?
t1 = df[[“Country”]].groupby(by=[df[“Country”],df[“State/Province”]]).count()
t2 = df.groupby(by=[“Country”,“State/Province”])[[“Country”]].count()
以上的两条命令结果一样
和之前的结果的区别在于当前返回的是一个DataFrame类型
那么问题来了:
和之前使用一个分组条件相比,当前的返回结果的前两列是什么?
t = df.groupby(["a", "b"]).agg({"c":"sum", "d":"mean"}).reset_index(drop=False)
11.索引和复合索引
简单的索引操作:
- 获取index:df.index
- 指定index :df.index = [‘x’,‘y’]
- 重新设置index : df.reindex(list(“abcedf”))
- 指定某一列作为index :df.set_index(“Country”,drop=False)
- 返回index的唯一值:df.set_index(“Country”).index.unique()
假设a为一个DataFrame,那么当a.set_index([“c”,“d”])即设置两个索引的时候是什么样子的结果呢?
a = pd.DataFrame({‘a’: range(7),‘b’: range(7, 0, -1),‘c’: [‘one’,‘one’,‘one’,‘two’,‘two’,‘two’, ‘two’],‘d’: list(“hjklmno”)})
12.Series复合索引
In [156]: t
Out[156]:
W X Y Z Q
A e 1 2 3 s
B e 5 6 7 s
C e 9 10 11 s
In [157]: c = t.set_index(["W", "Q"])["X"]
In [158]: c
Out[158]:
W Q
e s 1
s 5
s 9
Name: X, dtype: int64
In [162]: c.swaplevel()["s"]
Out[162]:
W
e 1
e 5
e 9
Name: X, dtype: int64
In [163]:
In [163]:
In [163]: c.swaplevel()
Out[163]:
Q W
s e 1
e 5
e 9
Name: X, dtype: int64
13.DataFrame复合索引
In [164]: t
Out[164]:
W X Y Z Q
A e 1 2 3 s
B e 5 6 7 s
C e 9 10 11 s
In [165]: x = t.set_index(["W", "X"])["Y"]
In [167]: x
Out[167]:
W X
e 1 2
5 6
9 10
Name: Y, dtype: int64
In [168]: x.loc["e"]
Out[168]:
X
1 2
5 6
9 10
Name: Y, dtype: int64
In [170]: x.loc["e"][1]
Out[170]: 2
In [171]: x
Out[171]:
W X
e 1 2
5 6
9 10
Name: Y, dtype: int64
In [172]: x.swaplevel().loc[5]
Out[172]:
W
e 6
Name: Y, dtype: int64
现在我们有全球排名靠前的10000本书的数据,那么请统计一下下面几个问题:
1.不同年份书的数量
2.不同年份书的平均评分情况
收据来源:https://www.kaggle.com/zygmunt/goodbooks-10k
现在我们有2015到2017年25万条911的紧急电话的数据,请统计出出这些数据中不同类型的紧急情况的次数,如果我们还想统计出不同月份不同类型紧急电话的次数的变化情况,应该怎么做呢?
数据来源:https://www.kaggle.com/mchirico/montcoalert/data
14.pandas中的时间序列
In [177]: pd.date_range(start='20200101', end='20200120', periods=None, freq=
...: "D")
Out[177]:
DatetimeIndex(['2020-01-01', '2020-01-02', '2020-01-03', '2020-01-04',
'2020-01-05', '2020-01-06', '2020-01-07', '2020-01-08',
'2020-01-09', '2020-01-10', '2020-01-11', '2020-01-12',
'2020-01-13', '2020-01-14', '2020-01-15', '2020-01-16',
'2020-01-17', '2020-01-18', '2020-01-19', '2020-01-20'],
dtype='datetime64[ns]', freq='D')
In [179]: pd.date_range(start='20200101', end='20200920', periods=None, freq=
...: "BM")
Out[179]:
DatetimeIndex(['2020-01-31', '2020-02-28', '2020-03-31', '2020-04-30',
'2020-05-29', '2020-06-30', '2020-07-31', '2020-08-31'],
dtype='datetime64[ns]', freq='BM')
In [180]: pd.date_range(start='20200101', end='20200920', periods=None, freq=
...: "WOM-3FRI")
Out[180]:
DatetimeIndex(['2020-01-17', '2020-02-21', '2020-03-20', '2020-04-17',
'2020-05-15', '2020-06-19', '2020-07-17', '2020-08-21',
'2020-09-18'],
dtype='datetime64[ns]', freq='WOM-3FRI')
In [181]:
15.关于频率的更多缩写
[外链图片转存失败,源站可能有防盗链机制,建议将图片保存下来直接上传(img-gxim4yf6-1607510406958)(https://cdn.nlark.com/yuque/0/2020/png/2355152/1606124356624-ec4514be-bc16-4deb-a3b6-edc5113a7fd6.png#align=left&display=inline&height=858&margin=%5Bobject%20Object%5D&name=image.png&originHeight=858&originWidth=1333&size=306472&status=done&style=none&width=1333)]
16.在DataFrame中使用时间序列
index=pd.date_range(“20170101”,periods=10)
df = pd.DataFrame(np.random.rand(10),index=index)
回到最开始的911数据的案例中,我们可以使用pandas提供的方法把时间字符串转化为时间序列
df[“timeStamp”] = pd.to_datetime(df[“timeStamp”],format="")
format参数大部分情况下可以不用写,但是对于pandas无法格式化的时间字符串,我们可以使用该参数,比如包含中文
'2020-06-19', '2020-07-17', '2020-08-21',
'2020-09-18', '2020-10-16'],
dtype='datetime64[ns]', freq='WOM-3FRI')
In [184]: index=pd.date_range("20170101",periods=10)
...:
In [185]: index
Out[185]:
DatetimeIndex(['2017-01-01', '2017-01-02', '2017-01-03', '2017-01-04',
'2017-01-05', '2017-01-06', '2017-01-07', '2017-01-08',
'2017-01-09', '2017-01-10'],
dtype='datetime64[ns]', freq='D')
In [186]: df = pd.DataFrame(np.random.rand(10),index=index)
...:
In [187]: df
Out[187]:
0
2017-01-01 0.371337
2017-01-02 0.187369
2017-01-03 0.976766
2017-01-04 0.836943
2017-01-05 0.622517
2017-01-06 0.337794
2017-01-07 0.385050
2017-01-08 0.216031
2017-01-09 0.223580
2017-01-10 0.102507
17.pandas重采样
重采样:指的是将时间序列从一个频率转化为另一个频率进行处理的过程,将高频率数据转化为低频率数据为降采样,低频率转化为高频率为升采样
pandas提供了一个resample的方法来帮助我们实现频率转化
In [188]: t = pd.DataFrame(np.random.uniform(10, 50, (100, 1)), index=pd.date
...: _range(start='20170101', periods=100))
In [189]: t
Out[189]:
0
2017-01-01 35.280383
2017-01-02 47.925842
2017-01-03 15.328393
2017-01-04 16.277246
2017-01-05 21.440968
... ...
2017-04-06 38.093319
2017-04-07 32.995266
2017-04-08 34.061548
2017-04-09 26.361549
2017-04-10 36.474314
[100 rows x 1 columns]
In [190]: t.resample("M").mean()
Out[190]:
0
2017-01-31 28.750880
2017-02-28 29.117664
2017-03-31 31.109807
2017-04-30 32.058395
In [191]: t.resample("QS-JAN").count()
Out[191]:
0
2017-01-01 90
2017-04-01 10
In [192]: t.resample("10D").count()
Out[192]:
0
2017-01-01 10
2017-01-11 10
2017-01-21 10
2017-01-31 10
2017-02-10 10
2017-02-20 10
2017-03-02 10
2017-03-12 10
2017-03-22 10
2017-04-01 10
现在我们有北上广、深圳、和沈阳5个城市空气质量数据,请绘制出5个城市的PM2.5随时间的变化情况
观察这组数据中的时间结构,并不是字符串,这个时候我们应该怎么办?
数据来源: https://www.kaggle.com/uciml/pm25-data-for-five-chinese-cities
18.PeriodIndex
之前所学习的DatetimeIndex可以理解为时间戳
那么现在我们要学习的PeriodIndex可以理解为时间段
periods = pd.PeriodIndex(year=data[“year”],month=data[“month”],day=data[“day”],hour=data[“hour”],freq=“H”)
那么如果给这个时间段降采样呢?
data = df.set_index(periods).resample(“10D”).mean()