1. 相关说明
2. CMakeLists.txt
# 指定最低的CMAKE版本
CMAKE_MINIMUM_REQUIRED(VERSION 3.22 FATAL_ERROR)
# 创建项目
PROJECT(PCLSamples LANGUAGES CXX)
# 指定CPLUSPLUS标准
SET(CMAKE_CXX_STANDARD_REQUIRED ON)
SET(CMAKE_CXX_STANDARD 14)
SET(CMAKE_C_STANDARD 14)
SET(CMAKE_C_STANDARD_REQUIRED ON)
# 查找第三方依赖库
FIND_PACKAGE(PCL 1.11 REQUIRED)
# 添加可执行文件
ADD_EXECUTABLE(PCLSample src/main.cpp)
# 添加头文件目录
TARGET_INCLUDE_DIRECTORIES(PCLSample PRIVATE ${PCL_INCLUDE_DIRS})
# 添加库目录
TARGET_LINK_DIRECTORIES(PCLSample PRIVATE ${PCL_LIBRARY_DIRS})
# 添加编译器定义
TARGET_COMPILE_DEFINITIONS(PCLSample PRIVATE ${PCL_DEFINITIONS})
# 取消已有的编译器定义
#SET_TARGET_PROPERTIES(PCLSample PROPERTIES COMPILE_FLAGS "/UBOOST_ALL_NO_LIB-DBOOST_ALL_NO_LIB")
# 添加依赖库文件
target_link_libraries(PCLSample PRIVATE ${PCL_LIBRARIES})
3. main.cpp
#include <limits>
#include <fstream>
#include <vector>
#include <Eigen/Core>
#include <pcl/memory.h>
#include <pcl/pcl_macros.h>
#include <pcl/point_types.h>
#include <pcl/point_cloud.h>
#include <pcl/io/pcd_io.h>
#include <pcl/kdtree/kdtree_flann.h>
#include <pcl/filters/passthrough.h>
#include <pcl/filters/voxel_grid.h>
#include <pcl/features/normal_3d.h>
#include <pcl/features/fpfh.h>
#include <pcl/registration/ia_ransac.h>
void calculateTemplate(_In_ const pcl::PointCloud<pcl::PointXYZ>::Ptr cloudPtr,
_In_ const float normalRadius,
_In_ const float featureRadius,
_Out_ pcl::PointCloud<pcl::FPFHSignature33>::Ptr& featurePtr)
{
pcl::PointCloud<pcl::Normal>::Ptr normalPtr(new pcl::PointCloud<pcl::Normal>);
featurePtr = pcl::PointCloud<pcl::FPFHSignature33>::Ptr(new pcl::PointCloud<pcl::FPFHSignature33>);
pcl::search::KdTree<pcl::PointXYZ>::Ptr searchMothodPtr(new pcl::search::KdTree<pcl::PointXYZ>);
pcl::NormalEstimation<pcl::PointXYZ, pcl::Normal> normalEstimation;
normalEstimation.setInputCloud(cloudPtr);
normalEstimation.setSearchMethod(searchMothodPtr);
normalEstimation.setRadiusSearch(normalRadius);
normalEstimation.compute(*normalPtr);
pcl::FPFHEstimation<pcl::PointXYZ, pcl::Normal, pcl::FPFHSignature33> featureEstimation;
featureEstimation.setInputCloud(cloudPtr);
featureEstimation.setInputNormals(normalPtr);
featureEstimation.setSearchMethod(searchMothodPtr);
featureEstimation.setRadiusSearch(featureRadius);
featureEstimation.compute(*featurePtr);
}
int main(int argc, char** argv)
{
std::vector<pcl::PointCloud<pcl::PointXYZ>::Ptr> clouds;
std::vector<pcl::PointCloud<pcl::FPFHSignature33>::Ptr> features;
std::ifstream input_stream("./data/object_templates.txt");
while (input_stream.good())
{
std::string pcd_filename;
std::getline(input_stream, pcd_filename);
if (pcd_filename.empty() || pcd_filename.at(0) == '#')
continue;
pcl::PointCloud<pcl::PointXYZ>::Ptr cloudPtr(new pcl::PointCloud<pcl::PointXYZ>);
pcl::io::loadPCDFile(pcd_filename, *cloudPtr);
pcl::PointCloud<pcl::FPFHSignature33>::Ptr featurePtr;
calculateTemplate(cloudPtr, 0.02f, 0.02f, featurePtr);
std::cout << "计算路径为 " << pcd_filename << " 的点云特征完成" << std::endl;
clouds.push_back(cloudPtr);
features.push_back(featurePtr);
}
input_stream.close();
pcl::PointCloud<pcl::PointXYZ>::Ptr cloudTtarget(new pcl::PointCloud<pcl::PointXYZ>);
pcl::io::loadPCDFile("./data/person.pcd", *cloudTtarget);
std::cout << "目标点云读取完成" << std::endl;
{
pcl::PassThrough<pcl::PointXYZ> pass;
pass.setInputCloud(cloudTtarget);
pass.setFilterFieldName("z");
pass.setFilterLimits(0, 1.0);
pass.filter(*cloudTtarget);
std::cout << "目标点云直通滤波完成" << std::endl;
}
{
pcl::VoxelGrid<pcl::PointXYZ> vox_grid;
vox_grid.setInputCloud(cloudTtarget);
vox_grid.setLeafSize(0.005f, 0.005f, 0.005f);
pcl::PointCloud<pcl::PointXYZ>::Ptr tempCloud(new pcl::PointCloud<pcl::PointXYZ>);
vox_grid.filter(*tempCloud);
cloudTtarget = tempCloud;
std::cout << "目标点云降采样完成" << std::endl;
}
pcl::PointCloud<pcl::FPFHSignature33>::Ptr targetFeaturePtr;
calculateTemplate(cloudTtarget, 0.02f, 0.02f, targetFeaturePtr);
pcl::SampleConsensusInitialAlignment<pcl::PointXYZ, pcl::PointXYZ, pcl::FPFHSignature33> sacIA;
sacIA.setMinSampleDistance(0.05f);
sacIA.setMaxCorrespondenceDistance(0.01f * 0.01f);
sacIA.setMaximumIterations(500);
sacIA.setInputTarget(cloudTtarget);
sacIA.setTargetFeatures(targetFeaturePtr);
std::vector<float> scores;
std::vector<Eigen::Matrix4f> transformations;
for (size_t i = 0; i < clouds.size(); i++)
{
sacIA.setInputSource(clouds[i]);
sacIA.setSourceFeatures(features[i]);
pcl::PointCloud<pcl::PointXYZ> registration_output;
sacIA.align(registration_output);
std::cout << "第 " << i << " 个模板匹配成功" << std::endl;
scores.push_back((float)sacIA.getFitnessScore(0.01f * 0.01f));
transformations.push_back(sacIA.getFinalTransformation());
}
float lowest_score = std::numeric_limits<float>::infinity();
int indexBest = 0;
for (std::size_t i = 0; i < scores.size(); ++i)
{
if (scores[i] < lowest_score)
{
lowest_score = scores[i];
indexBest = (int)i;
}
}
auto transformBest = transformations[indexBest];
printf("Best fitness score: %f\n", lowest_score);
Eigen::Matrix3f rotation = transformBest.block<3, 3>(0, 0);
Eigen::Vector3f translation = transformBest.block<3, 1>(0, 3);
printf("\n");
printf(" | %6.3f %6.3f %6.3f | \n", rotation(0, 0), rotation(0, 1), rotation(0, 2));
printf("R = | %6.3f %6.3f %6.3f | \n", rotation(1, 0), rotation(1, 1), rotation(1, 2));
printf(" | %6.3f %6.3f %6.3f | \n", rotation(2, 0), rotation(2, 1), rotation(2, 2));
printf("\n");
printf("t = < %0.3f, %0.3f, %0.3f >\n", translation(0), translation(1), translation(2));
pcl::PointCloud<pcl::PointXYZ> transformed_cloud;
pcl::transformPointCloud(*clouds[indexBest], transformed_cloud, transformBest);
pcl::io::savePCDFileBinary("output.pcd", transformed_cloud);
return EXIT_SUCCESS;
}
4. 效果图
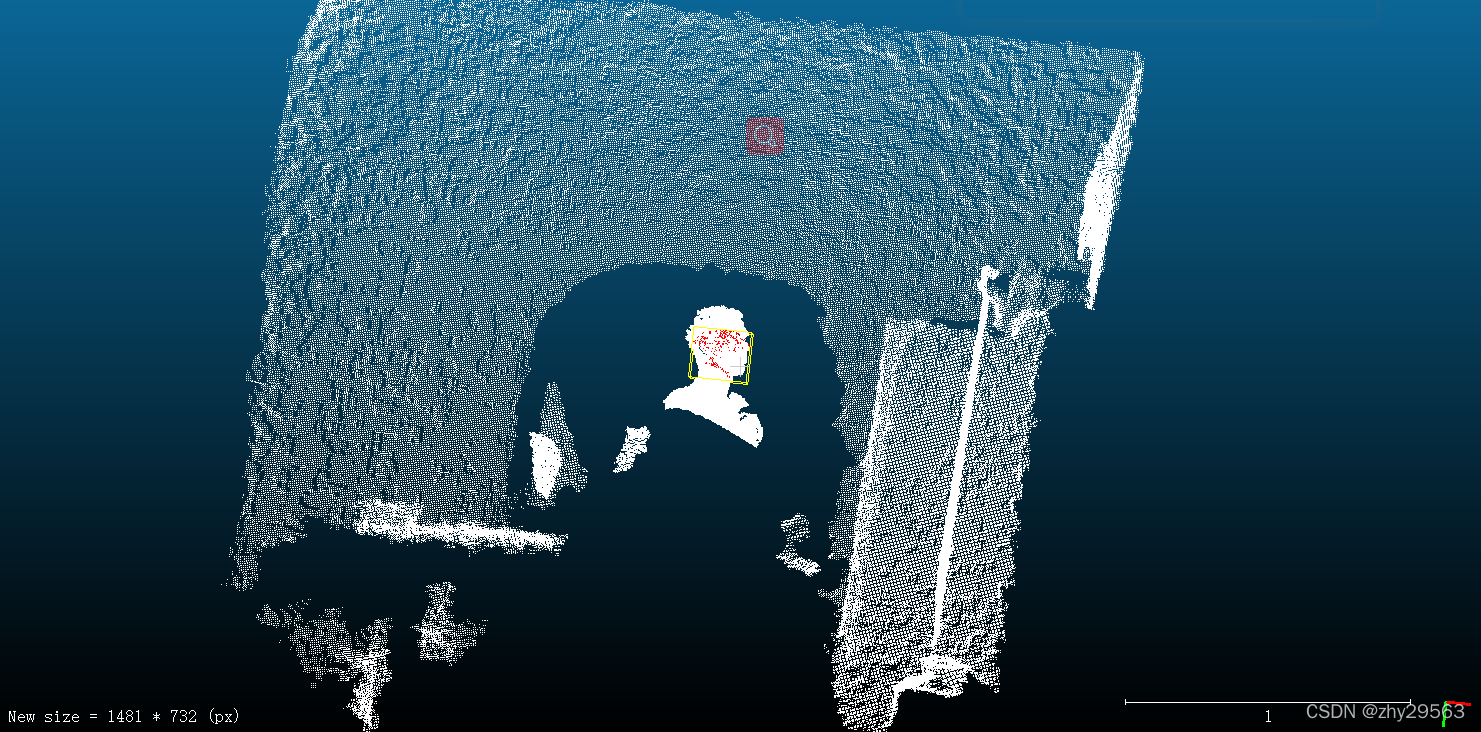