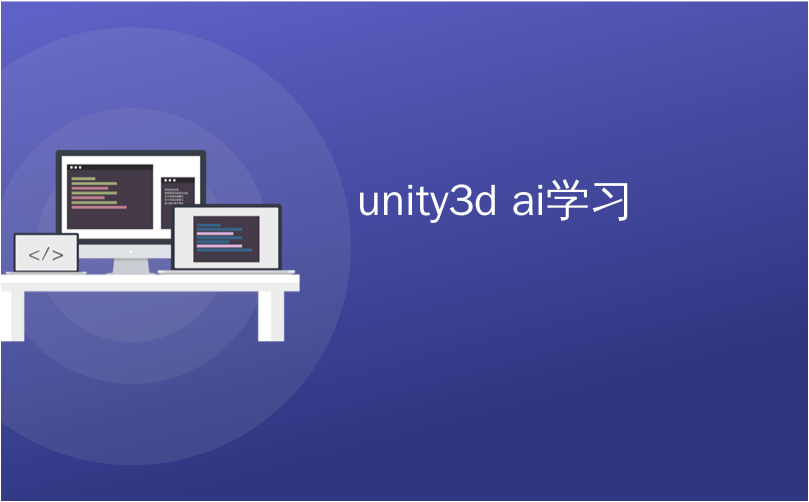
unity3d ai学习
Welcome to the second entry in the Unity AI Blog series! For this post, I want to pick up where we left off last time, and talk about how to take a Contextual Bandit problem, and extend it into a full Reinforcement Learning problem. In the process, we will demonstrate how to use an agent which acts via a learned Q-function that estimates the long-term value of taking certain actions in certain circumstances. For this example we will only use a simple gridworld, and a tabular Q-representation. Fortunately, this, basic idea applies to almost all games. If you like to try out the Q-learning demo, follow the link here. For a deeper walkthrough of how Q-learning works, continue to the full text below.
欢迎来到Unity AI博客系列中的第二篇文章! 对于这篇文章,我想谈谈上次中断的地方,并讨论如何解决上下文强盗问题 ,并将其扩展到完整的强化学习问题。 在此过程中,我们将演示如何使用通过学习的Q函数起作用的代理,该Q函数估计在某些情况下采取某些行动的长期价值。 在此示例中,我们将仅使用简单的gridworld和表格Q表示。 幸运的是,这个基本思想几乎适用于所有游戏。 如果您想尝试Q学习演示,请点击此处的链接。 要深入了解Q学习的工作原理,请继续下面的全文。
Q学习算法 (The Q-Learning Algorithm)
Contextual Bandit Recap
上下文强盗回顾
The goal when doing Reinforcement Learning is to train an agent which can learn to act in ways that maximizes future expected rewards within a given environment. In the last post in this series, that environment was relatively static. The state of the environment was simply which of the three possible rooms the agent was in, and the actions were choosing which chest within that room to open. Our algorithm learned the Q-function for each of these state-action pairs: Q(s, a). This Q-function corresponded to the expected future reward that would be acquired by taking that action within that state over time. We called this problem the “Contextual Bandit.”
进行强化学习的目的是训练能够在给定环境中以最大化未来预期收益的方式行事的代理商。 在本系列的最后一篇文章中,该环境是相对静态的。 环境状态仅是代理程序所在的三个可能的房间中的哪个,而动作则在选择该房间中要打开哪个箱子。 我们的算法为以下每个状态动作对学习了Q函数:Q(s,a)。 该Q函数对应于预期的未来奖励,该奖励将通过在该状态内随着时间的推移采取该操作而获得。 我们称这个问题为“背景强盗”。
The Reinforcement Learning Problem
强化学习问题
The lack of two things kept that Contextual Bandit example from being a proper Reinforcement Learning problem: sparse rewards, and state transitions. By sparse rewards, we refer to the fact that the agent does not receive a reward for every action it takes. Sometimes these rewards are “delayed,” in that certain actions which may in fact be optimal, may not provide a payout until a series of optimal actions have been taken. To use a more concrete example, an agent may be following the correct path, but it will only receive a reward at the end of the path, not for every step along the way. Each of those actions may have been essential to getting the final reward, even if they didn’t provide a reward at the time. We need a way to perform credit assignment, that is, allowing the agent to learn that earlier actions were valuable, even if only indirectly.
缺少两件事使上下文强盗示例成为适当的强化学习问题: 稀疏的奖励 和 状态转换 。 稀疏奖励是指代理人不会因其采取的每项行动而获得奖励的事实。 有时,这些奖励是“延迟的”,因为实际上可能是最佳的某些操作在采取一系列最佳操作之前可能无法提供回报。 用一个更具体的例子来说,一个代理可能遵循正确的路径,但是它只会在路径的尽头获得报酬,而不是沿途的每一步。 这些动作中的每一个对于获得最终的奖励可能都是必不可少的,即使当时他们没有提供奖励。 我们需要一种执行 信用分配的方法 ,也就是说,允许代理人了解到,即使只是间接地,早期的行动也很有价值。
The second missing element is that in full reinforcement learning problems there are transitions between states. This way, our actions not only produce rewards according to a reward function: R(s, a) ⇨ r, but also produce new states, according to a state transition function: P(s, a) ⇨ s’. A concrete example here is that every step taken while walking along a path brings the agent to a new place in that path, hence a new state. Therefore we want our agent not only to learn to act to optimize the current possible reward, but act to move toward states we know provide even larger rewards.
第二个缺少的要素是,在全面强化学习问题中,状态之间存在转换。 这样一来,我们的行动按照奖励功能不仅生产奖励:R(S,A)⇨R, 还会产生新的状态,根据状态转换函数:P(S,A)⇨S'。 这里的一个具体示例是,沿着路径行走时采取的每一个步骤都会将特工带到该路径中的新位置,从而建立新的状态。 因此,我们希望我们的代理人不仅要学会采取行动以优化当前可能的回报,而且还要采取行动朝着我们所知的州提供更大的回报。
Bellman Updates
贝尔曼更新
While these two added elements of complexity may at first seem unrelated, they are in fact directly connected. Both imply a relationship between future states our agent might end up in, and future rewards our agent might receive. We can take advantage of this relationship to learn to take optimal actions under these circumstances with a simple insight. Namely, that under a “true” optimal Q-function (a theoretical one which we may or may not ever reach ourselves) the value of a current state and action can be decomposed into to the immediate reward r plus the discounted maximum future expected reward from the next state the agent will end up in for taking that action:
尽管这两个增加的复杂性元素乍一看似乎并不相关,但实际上它们是直接相连的。 两者都暗示我们的代理商可能会进入的未来状态之间的关系,以及我们的代理商可能会获得的未来奖励。 我们可以利用这种关系,以简单的见识来学习在这种情况下采取最佳行动。 即,在“真实的”最佳Q函数(一种我们可能会或可能永远不会达到的理论值)下,当前状态和动作的值可分解为即时奖励 r 加折现的最大未来预期奖励从下一个状态开始,代理将最终采取该操作:

This is called the Bellman equation, and can be written as follows:
这称为Bellman方程,可以编写如下:

Here ? (gamma) is a discount term, which relates to how much we want our agent to care about future possible rewards. If we set ? to 1.0, our agent would value all possible future rewards equally, and in training episodes which never end, the value estimate might increase to infinity. For this reason, we set ? to something greater than 0 and less than 1. Typical values are between 0.7 and 0.99.
这里 ? (gamma)是一个折扣术语,与我们希望我们的代理商关心未来可能的奖励有多少有关。 如果我们设置? 到1.0,我们的代理人将平均评估未来所有可能的回报,并且在永远不会结束的训练中,价值估计可能会增加到无穷大。 因此,我们设置? 大于0且小于1的值。典型值在0.7到0.99之间。
The Bellman equation is useful because it provides a way for us to think about updating our Q-function by bootstrapping from the Q-function itself. Q*(s, a) refers to an optimal Q-function, but even our current, sub-optimal Q value estimates of the next state can help push our estimates of the current state in a more accurate direction. Since we are relying primarily on the true rewards at each step, we can trust that the Q-value estimates themselves will slowly improve. We can use the Bellman equation to inform the following new Q-learning update:
Bellman方程很有用,因为它为我们提供了一种通过从Q函数本身进行引导来考虑更新Q函数的方法。 Q *(s,a)是指最佳Q函数,但是即使我们对下一个状态的当前,次优Q值估计也可以帮助将我们对当前状态的估计推向更准确的方向。 由于我们主要依赖于每个步骤的真实回报,因此我们可以相信Q值估计值自身会逐渐提高。 我们可以使用Bellman方程通知以下新的Q学习更新:

This looks similar to our previous contextual bandit update algorithm, except that our Q-target now includes the discounted future expected reward at the next step.
这看起来与我们先前的上下文强盗更新算法相似,不同之处在于,我们的Q目标现在包括了下一步的预期未来折价折扣。
Exploration
勘探
In order to ensure that our agent properly explores the state space, we will utilize a form of exploration called epsilon-greedy. To use epsilon-greedy, we simply set an epsilon value ϵ to 1.0, and decrease it by a small amount every time the agent takes an action. When the agent chooses an action, it either picks argmax(Q(s, a)), the greedy action, or takes a random action with probability ϵ. The intuition is that at the beginning of training our agent’s Q-value estimates are likely to be very poor, but as we learn about the world, and ϵ decreases, our Q-function will slowly correspond more to the true Q-function of the environment, and the actions we take using it will be increasingly accurate.
为了确保我们的代理正确探索状态空间,我们将使用一种称为 epsilon-greedy 的探索形式 。 要使用epsilon-greedy,我们只需将epsilon值设置为1.0,并在代理每次执行操作时将其减小一点。 当主体选择一个动作时,它要么选择 argmax(Q(s,a)) ,即贪婪的动作,要么采取概率为a的随机动作。 直觉是,在训练开始时,我们的特工的Q值估算值可能很差,但是随着我们对世界的了解和ϵ的减少,我们的Q函数将慢慢地更对应于Q值的真实Q函数。环境,我们采取的行动将越来越准确。
Unity Gridworld (The Unity Gridworld)
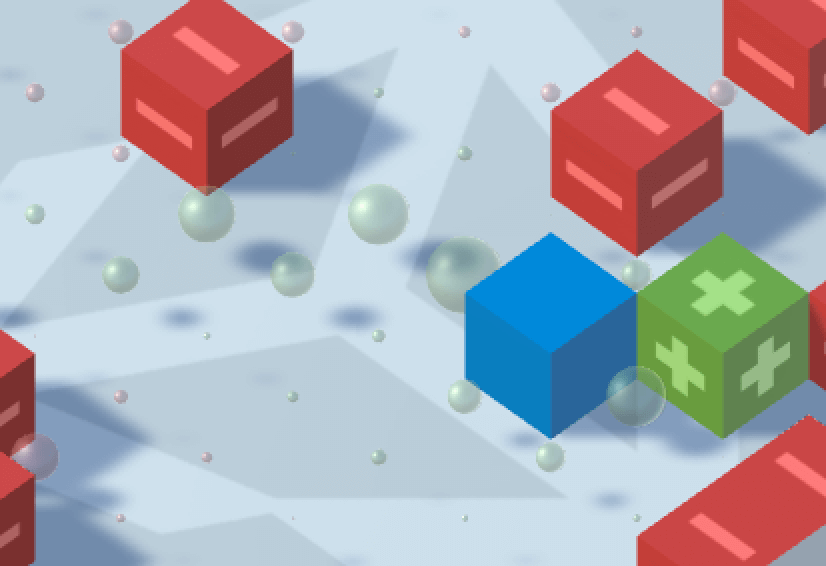
The blue block corresponds to the agent, the red blocks to the obstacles, and the green block to the goal position. The green and red spheres correspond to the value estimates for each of the states within the GridWorld.
蓝色方块对应于特工,红色方块对应于障碍物,绿色方块对应于目标位置。 绿色和红色球形对应于GridWorld中每个状态的值估计。
To demonstrate a Q-learning agent, we have built a simple GridWorld environment using Unity. The environment consists of the following: 1- an agent placed randomly within the world, 2- a randomly placed goal location that we want our agent to learn to move toward, 3- and randomly placed obstacles that we want our agent to learn to avoid. The state (s) of the environment will be an integer which corresponds to the position on the grid. The four actions (a) will consist of (Up, Down, Left, and Right), and the rewards (r) will be: +1 for moving to the state with the goal, -1 for moving to the state with an obstacle, and -0.05 for each step, to encourage quick movement to the goal on the part of the agent. Each episode will end after 100 steps, or when the agent moves to a state with either a goal or obstacle. Like in the previous tutorial, the agent’s Q values will be stored using a table, where the rows correspond to the state, and the columns to the possible actions. You can play with this environment and agent within your Web browser here, and download the Unity project to modify for use in your own games here. As the agent explores the environment, colored orbs will appear in each of the GridWorld states. These correspond to the agent’s average Q-value estimate for that state. Once the agent learns an optimal policy, it will be visible as a direct value gradient from the start position to the goal.
为了演示Q学习代理,我们使用Unity构建了一个简单的GridWorld环境。 该环境包括以下内容:1-一个随机放置在世界上的特工,2-一个我们希望我们的学习者朝其移动的随机放置的目标位置,3-我们希望我们的学习者避免避开的随机放置的障碍物。 环境的 状态 (多个) 将是一个整数,其对应于在网格上的位置。 四个动作 (a) 将由(上,下,左和右)组成,而奖励 (r) 将为:+1移至有目标的状态,-1移至有障碍的状态,并为每个步骤设置-0.05,以鼓励特工将此目标快速移至目标。 每个情节将在100步后结束,或者当特工移动到有目标或障碍的状态时结束。 与上一教程中一样,代理的Q值将使用表格存储,表格中的行对应于状态,而列则对应于可能的操作。 你可以用这个环境和代理网络浏览器中播放 这里 ,并下载团结项目修改用在自己的游戏 在这里 。 随着代理探索环境,彩色球将出现在每个GridWorld状态中。 这些对应于该状态的业务代表的平均Q值估计。 代理了解到最佳策略后,它将显示为从起始位置到目标的直接值梯度。
向前走 (Going Forward)
The agent and environment presented here represent a classic tabular formulation of the Q-learning problem. If you are thinking that perhaps there is not much in common with this basic environment and the ones you find in contemporary games, do not worry. In the years since the algorithm’s introduction in the 90s, there have been a number of important developments to allow Q-learning to be used in more varied and dynamic situations. One prime example is DeepMind’s Deep Q-Network which was used to learn to play dozens of different ATARI games directly from pixels, a feat impossible using only a lookup table like the one here. In order to accomplish this, they utilized an agent which was controlled by a Deep Neural Network (DNN). By using a neural network it is possible to learn a generalized Q-function which can be applied to completely unseen states, such as novel combinations of pixels on a screen.
这里介绍的主体和环境代表了Q学习问题的经典表格形式。 如果您认为此基本环境与现代游戏中没有太多共同点,请不要担心。 自从90年代算法问世以来,已有许多重要的进展,使Q学习可用于更多变化和动态的情况。 一个很好的例子是DeepMind的Deep Q-Network,它被用来学习直接从像素上玩几十种不同的ATARI游戏,仅使用像此处的查找表就不可能实现这一壮举。 为了完成此任务,他们使用了由深度神经网络(DNN)控制的代理。 通过使用神经网络,可以学习可应用于完全看不见的状态(例如屏幕上像素的新颖组合)的广义Q函数。
In the next few weeks we will release an interface with a set of algorithms and example projects to allow for the training of similar Deep Reinforcement Learning agent in Unity games and simulations. For a sneak peek of what these tools are capable of, you can check out the video link here. While this initial release will be limited, and aimed primarily at those working in research, industry, and game QA testing, we at Unity are excited about the possibilities opened up by using modern Deep Learning methods to learn game behavior. We hope that as this work matures, it will spark interest in using ML within game to control complex NPC behavior, game dynamics, and more. We are at the very beginning of exploring using Deep Learning in games, and we look forward to you continuing with us on this journey.
在接下来的几周内,我们将发布一个界面,其中包含一组算法和示例项目,以允许在Unity游戏和模拟中训练类似的Deep Reinforcement Learning代理。 要一窥这些工具的功能,可以 在此处 查看视频链接 。 虽然此初始发行版将受到限制,并且主要针对从事研究,行业和游戏QA测试的人员,但我们对Unity使用现代深度学习方法学习游戏行为所带来的可能性感到兴奋。 我们希望随着这项工作的成熟,将激发人们在游戏中使用ML来控制复杂的NPC行为,游戏动态性等的兴趣。 我们正处于探索在游戏中使用深度学习的开始之初,我们期待您继续我们的旅程。
翻译自: https://blogs.unity3d.com/2017/08/22/unity-ai-reinforcement-learning-with-q-learning/
unity3d ai学习