'''
>>> import tensorflow as tf;tf.__version__
2021-11-07 23:53:04.980446: I tensorflow/stream_executor/platform/default/dso_loader.cc:48] Successfully opened dynamic library libcudart.so.10.1
'2.3.0'
>>> import tensorflow_datasets as tfds;tfds.__version__
'4.3.0'
>>>
'''
import tensorflow as tf
from tensorflow.keras import layers, Model
from tensorflow.keras.activations import relu
from tensorflow.keras.models import Sequential, load_model
from tensorflow.keras.callbacks import ModelCheckpoint, EarlyStopping
from tensorflow.keras.losses import BinaryCrossentropy
from tensorflow.keras.optimizers import RMSprop, Adam
from tensorflow.keras.metrics import binary_accuracy
import tensorflow_datasets as tfds
import numpy as np
import matplotlib.pyplot as plt
# load datasets
ds_train, ds_info = tfds.load('fashion_mnist', split='train', shuffle_files=True, with_info=True)
fig = tfds.show_examples(ds_info, ds_train)
batch_size = 200
image_shape = (28, 28, 1)
def preprocess(features):
image = tf.image.resize(features['image'], image_shape[:2])
image = tf.cast(image, tf.float32)
image = (image-127.5)/127.5
return image
ds_train = ds_train.map(preprocess)
ds_train = ds_train.cache() # put dataset into memory
ds_train = ds_train.shuffle(ds_info.splits['train'].num_examples)
ds_train = ds_train.batch(batch_size).repeat()
train_num = ds_info.splits['train'].num_examples
train_steps_per_epoch = round(train_num/batch_size)
print(train_steps_per_epoch)
class GAN():
def __init__(self, generator, discriminator):
# discriminator
self.D = discriminator
self.G = generator
self.bce = tf.keras.losses.BinaryCrossentropy()
self.d_loss = {}
self.g_loss = {}
self.accuracy = {}
self.g_gradients = []
def discriminator_loss(self, pred_fake, pred_real):
real_loss = self.bce(tf.ones_like(pred_real), pred_real)
fake_loss = self.bce(tf.zeros_like(pred_fake), pred_fake)
d_loss = 0.5*(real_loss + fake_loss)
return d_loss
def generator_loss(self, pred_fake):
g_loss = self.bce(tf.ones_like(pred_fake), pred_fake)
return g_loss
def train_step(self, g_input, real_input):
with tf.GradientTape() as g_tape,\
tf.GradientTape() as d_tape:
# Feed forward
fake_input = s
[GAN实战] DCGAN实现
最新推荐文章于 2023-12-27 20:43:26 发布
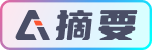