class GetAttentionHiddens(nn.Module):
def __init__(self, input_size, attention_hidden_size, similarity_attention = False):
super(GetAttentionHiddens, self).__init__()
self.scoring = AttentionScore(input_size, attention_hidden_size, similarity_score=similarity_attention)
def forward(self, x1, x2, x2_mask, x3=None, scores=None, return_scores=False, drop_diagonal=False):
"""
Using x1, x2 to calculate attention score, but x1 will take back info from x3.
If x3 is not specified, x1 will attend on x2.
x1: [batch, len1, x1_input_size]
x2: [batch, len2, x2_input_size]
x2_mask: [batch, len2]
x3: [batch, len2, x3_input_size]
"""
if x3 is Non
一组向量 和 一组向量 的attention计算,pytorch实现
最新推荐文章于 2024-04-15 12:18:20 发布
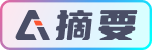