ABSTRACT
problem:
Extracting relations in social media posts is challenging when
sentences lack of contexts.
solution:
images related to these
sentences can supplement such missing contexts and help to
identify relations precisely.
1. INTRODUCTION
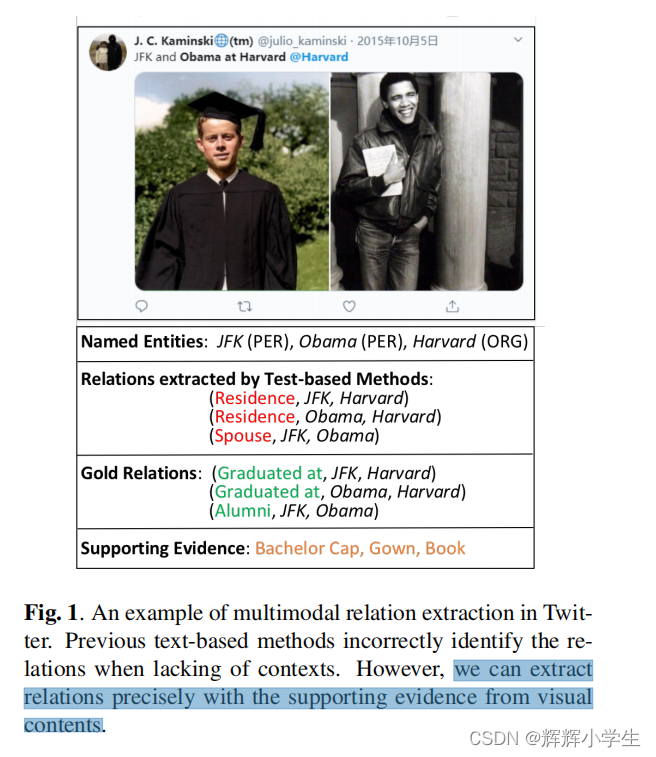
introduce a new task called multimodal relation extraction and a human-annotated multimodal dataset
propose several multimodal baselines
provide an in-depth and thorough analysis for different cases
2. RELATED WORK
2.1. Relation Extraction in Social Media
2.2. Multimodal Dataset
there are fewer datasets focusing on text-intensive tasks
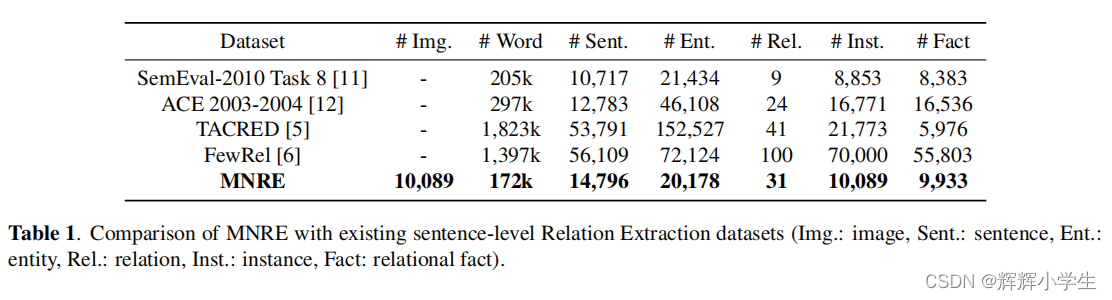
3. MNRE DATASET
3.1. Dataset Collection
sources: two available
multimodal named entity recognition datasets - Twitter15
and Twitter17 and crawling data from Twitter.
3.2. Twitter Name Tagging
3.3. Human Annotation
3.4. Dataset Statistics
3.5. Case Analysis
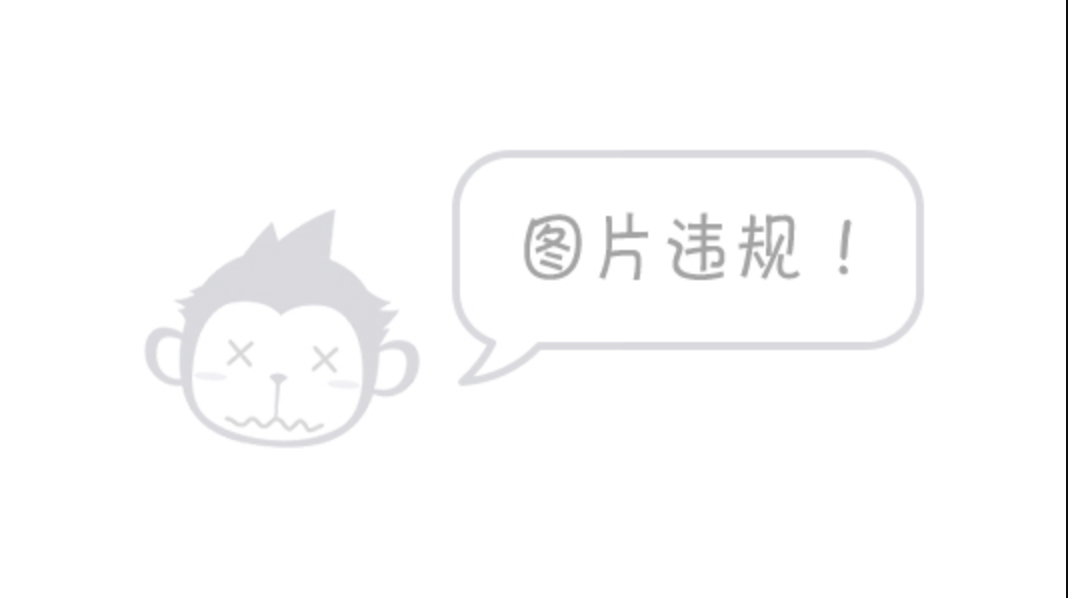
4. EXPERIMENTAL RESULT AND ANALYSIS
4.1. Problem Defifinition
function to predict relations:
F : (
e
1
,
e
2
,
S
,
V
)
→ Y
e1,e2: pre-extracted
named entities
S
= (
w
1
, w
2
, ..., wn): a given sentence(marked entities
e
1,
e
2)
V
= (
v
1
, v
2
, ..., v
n): visual contents
Y: the corresponding rela
tion tag
4.2. Baselines of Relation Extraction
choose models from three aspects: CNN-based method;pre
-trained language model based method;distantly super
vised method.
Glove+CNN:classic CNN-based model for re
BertNRE: pre-trained language model
Bert+CNN: ablation model(
to demon
strate that the image features are more adaptive to CNN-based
methods
)
PCNN: distantly supervised re model(also CNN-based)
4.3. Visual Feature Extraction
three methods to incorporate visual information:
Image Labels
Visual Objects Visual Attention
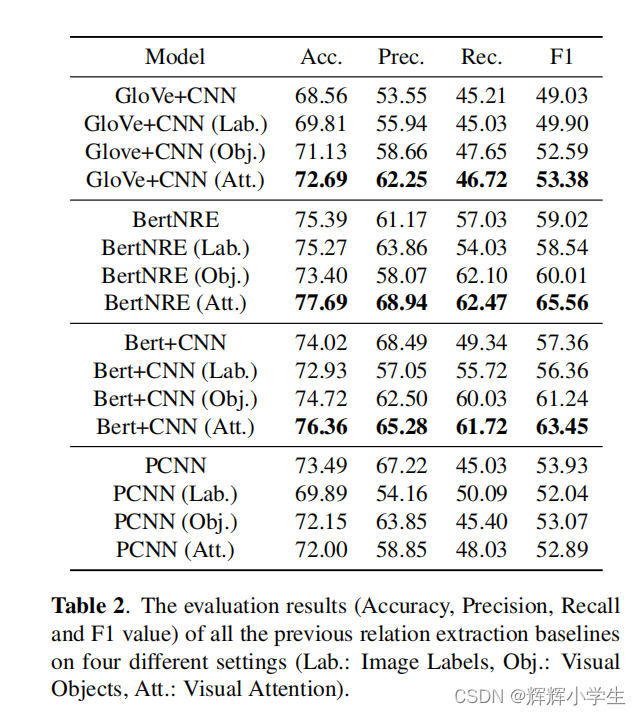
4.4. General Results
4.5. Error Analysis
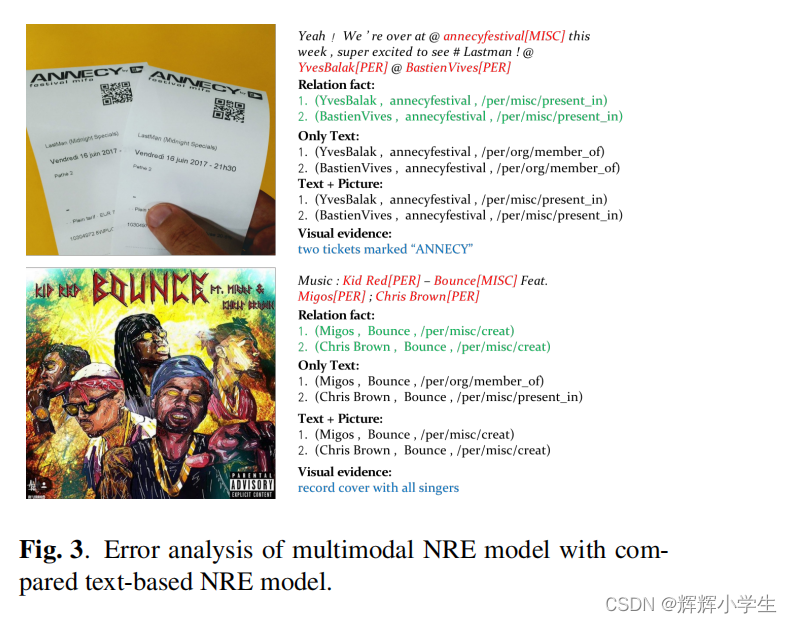
5. CONCLUSION