Active Learning关注如何采样:select the most informative images for detector training训练阶段选择信息量最大的图像,通过观察实例级的不确定性
一个采样策略,在未标注数据中选择最具代表性图像,送去专家Oracle模型中打上标签。在目标检测中,要选择最不确定的实例
如何确定采样策略?
maximizing the prediction discrepancy = larger uncertainty
===============================
问题:the labeled sets are small
实例级的不确定性 & 图像不确定性,图像不确定性 = re-weighting*实例1不确定性 + re-weighting*实例2不确定性 + re-weighting*noise实例3不确定性
刚开始已经训练好的模型是纯净的,分类能力最强,然后选择最不确定的去打上标签
其利用在标记集上训练的两个对立实例分类器的差异来预测未标记集中实例不确定性。
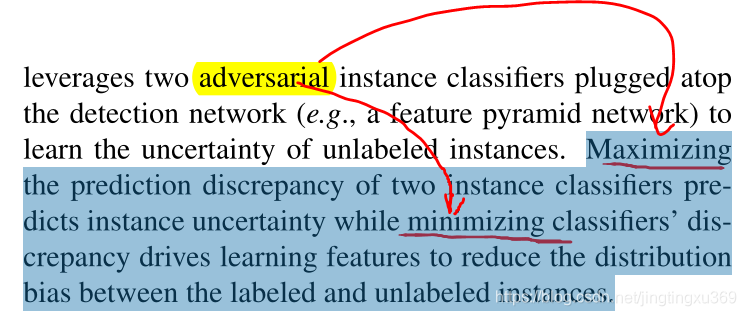
re-weighting相关描述:
performs instance uncertainty re-weighting (IUR) by evaluating instance appearance consistency across images. 通过评估图像间的实例外观一致性,执行实例不确定性重新加权(IUR)。re-weighting the instance uncertainty under the supervision of the image classification loss.
step1:uncertainty of the unlabeled instances
step2:estimate the image uncertainty while filtering out noisy instances 通过分别介绍两个学习模块解决两个问题