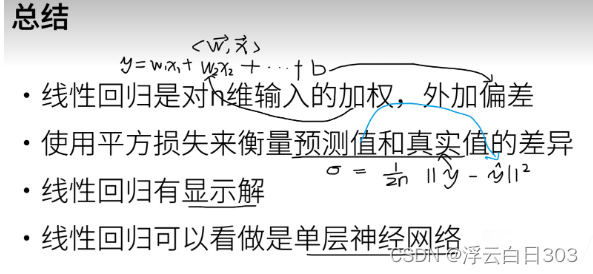
梯度下降:以最快速度逼近最优解
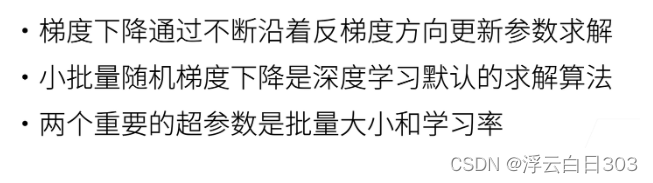
import random
import torch
from d2l import torch as d2l
def zaosheng(w,b,yangbenshu):
x= torch.normal(0,1,(yangbenshu,len(w)))
#torch.normal可分为三种情况:(1)torch.normal(means,std, out=None)中means和std都是Tensor,两者的形状可以不必相同,但Tensor内的元素数量必须相同,一一对应的元素作为输出的各元素的均值和标准差;(2)torch.normal(mean=0.0, std, out=None)中mean是一个可定义的float,各个元素共享该均值;(3)torch.normal(means,std=1.0, out=None)中std是一个可定义的float,各个元素共享该标准差。
y = torch.matmul(x,w)+b#张量相乘
y += torch.normal(0,0.01,y.shape)
return x,y.reshape((-1,1))
shiji_w = torch.tensor([2,-3.4])
shiji_b = 4.2
feature,lable = zaosheng(shiji_w,shiji_b,1000)
d2l.set_figsize()
d2l.plt.scatter(feature[:,1].detach().numpy(),lable.detach().numpy())#第一列
#d2l.plt.show()#显示出图样
#取小批量数据集
def data_ji(batch,feature,label):
yanbenshu = len(feature)
zliebiao= list(range(yanbenshu))
random.shuffle(zliebiao)#打乱列表顺序
for i in range (0,yanbenshu,batch):
shoujipiliang = torch.tensor(zliebiao[i:min((i+batch),yanbenshu)])
yield feature[shoujipiliang],label[shoujipiliang]#近似于return
#<https://blog.csdn.net/qq_41554005/article/details/119940983?ops_request_misc=%257B%2522request%255Fid%2522%253A%2522168078543116800213041866%2522%252C%2522scm%2522%253A%252220140713.130102334..%2522%257D&request_id=168078543116800213041866&biz_id=0&utm_medium=distribute.pc_search_result.none-task-blog-2~all~top_positive~default-1-119940983-null-null.142^v81^koosearch_v1,201^v4^add_ask,239^v2^insert_chatgpt&utm_term=yield%E5%87%BD%E6%95%B0%E6%80%8E%E4%B9%88%E7%94%A8&spm=1018.2226.3001.4187>
batch = 10
# for x,y in data_ji(batch,feature,lable):
# print(x,'\\n',y)
# break
#定义初始化模型
w = torch.normal(0,0.01,size=(2,1),requires_grad=True)#x(1000,2) w(2,1)可以进行向量积
b= torch.zeros(1,requires_grad=True)
#定义线性回归模型
def xianxing(x,w,b):
return torch.matmul(x,w)+b
#定义损失函数 均方损失
def junfang_loss(y,shi_y):
return (y-shi_y.reshape(y.shape))**2/2
#定义优化算法
def youhua(zongcan,xuexilv,batch):#zongcan包括W B
#小批量随机梯度下降
with torch.no_grad():
for gecan in zongcan:
gecan -= xuexilv*gecan.grad/batch#弥补上面的求平均 梯度下降
gecan.grad.zero_()
xuexilv = 0.03
saomiaocishu = 3
net = xianxing
loss = junfang_loss
for cishu in range(saomiaocishu):
for x,y in data_ji(batch,feature,lable):
l=loss(net(x,w,b),y)
l.sum().backward()
youhua([w,b],xuexilv,batch)
with torch.no_grad():
xunlian = loss(net(feature,w,b),lable)
print(f'cishu {cishu + 1},loss {float(xunlian.mean()):f}')
print(shiji_w-w.reshape(shiji_b.shape))
print(shiji_b-b)
线性回归简化实现
#线性回归的简洁实现
import numpy as np
import torch
from torch.utils import data
from d2l import torch as d2l
from torch import nn
shi_w = torch.tensor([2,-3.4])
shi_b = 4.2
feature,lable = d2l.synthetic_data(shi_w,shi_b,1000)
##构造一个数据迭代器
def zonshuju(data_list,batch,is_train=True):
dataset = data.TensorDataset(*data_list)
return data.DataLoader(dataset,batch,shuffle=is_train)
batch = 10
shujuji = zonshuju((feature,lable),batch)
#next() 返回迭代器的下一个项目
#<https://blog.csdn.net/weixin_42782150/article/details/109315355?ops_request_misc=&request_id=&biz_id=102&utm_term=next(iter()>)&utm_medium=distribute.pc_search_result.none-task-blog-2~all~sobaiduweb~default-2-109315355.nonecase&spm=1018.2226.3001.4187
#print(next(iter(shujuji)))
## Sequential一个有序的容器,神经网络模块将按照在传入构造器的顺序依次被添加到计算图中执行,
# 同时以神经网络模块为元素的有序字典也可以作为传入参数。
#linear 是全连接层
net = nn.Sequential(nn.Linear(2,1))
###初始化模型参数
net[0].weight.data.normal_(0,0.01)
net[0].bias.data.fill_(0)
##损失
loss = nn.MSELoss()
##优化
train = torch.optim.SGD(net.parameters(),lr=0.03)
saomiancishu = 3
for saomiao in range(saomiancishu):
for x,y in shujuji:
l= loss(net(x),y)
train.zero_grad()#清零梯度
l.backward()#计算与图中叶子结点有关的当前张量的梯度
train.step()#模型更新
l =loss(net(feature),lable)
print(float(l))