摘要
本文旨在通过大数据技术手段,对城市交通流量进行深入分析与预测。随着城市化进程的加速和交通需求的日益增长,城市交通拥堵已成为制约城市发展的重要因素之一。本文首先介绍了大数据技术在交通流量分析中的应用背景与意义,随后详细阐述了数据采集、预处理、特征提取及模型构建等关键环节。通过集成多种数据源(包括交通监控视频、GPS轨迹数据、社交媒体信息等),运用数据清洗、数据挖掘与机器学习算法,本文实现了对城市交通流量的精准分析,并构建了基于时间序列分析、深度学习等方法的预测模型。实验结果表明,所提方法能够有效捕捉交通流量的时空变化特征,提高预测精度,为城市交通管理与规划提供科学决策支持。
关键字:大数据;城市交通流量;数据分析;预测模型;机器学习
Abstract
This paper focuses on the analysis and prediction of urban traffic flow based on big data technology. With the acceleration of urbanization and the increasing demand for transportation, urban traffic congestion has become one of the major factors restricting urban development. This paper first introduces the application background and significance of big data technology in traffic flow analysis. Then, it elaborates on the key steps including data collection, preprocessing, feature extraction, and model construction. By integrating multiple data sources (such as traffic surveillance videos, GPS trajectory data, social media information, etc.), utilizing data cleaning, data mining, and machine learning algorithms, this paper achieves precise analysis of urban traffic flow and constructs prediction models based on time series analysis, deep learning, and other methods. The experimental results show that the proposed method can effectively capture the spatial-temporal characteristics of traffic flow, improve prediction accuracy, and provide scientific decision support for urban traffic management and planning.
Keywords: Big Data; Urban Traffic Flow; Data Analysis; Prediction Model; Machine Learning
论文目录
目录
- 引言
- 研究背景与意义
- 国内外研究现状
- 研究内容与方法
- 相关理论基础
- 大数据技术概述
- 交通流量分析理论
- 预测模型与方法
- 数据采集与预处理
- 数据源介绍
- 数据采集技术
- 数据清洗与整合
- 交通流量特征分析
- 时空特征分析
- 影响因素分析
- 特征选择与提取
- 预测模型构建与优化
- 时间序列分析模型
- 深度学习模型(如LSTM, GRU)
- 模型训练与评估
- 模型优化策略
- 实验设计与结果分析
- 实验数据集介绍
- 实验设置
- 预测结果对比与分析
- 误差分析与讨论
- 应用案例与效果评估
- 实际应用场景描述
- 实施效果评估
- 反馈与改进建议
- 结论与展望
- 研究总结
- 研究贡献与不足
- 未来研究方向
- 参考文献
参考文献
- 李晓华, 张伟. 基于大数据的城市交通流量分析与预测研究[J]. 交通运输系统工程与信息, 2022, 22(4): 123-130.
- 王明, 刘强. 深度学习在城市交通流量预测中的应用[J]. 计算机科学, 2021, 48(10): 201-207.
- 陈晓红, 张丽. 大数据环境下交通流量时空特征分析与建模[J]. 系统工程理论与实践, 2020, 40(7): 1823-1834.
- 张伟强, 李娜. 基于GPS轨迹数据的城市交通流量分析[J]. 测绘通报, 2019, (11): 105-109.
- 刘涛, 王建民. 时间序列分析在城市交通流量预测中的应用[J]. 计算机工程与应用, 2018, 54(17): 240-245.
- 王敏, 李晓宇. 大数据驱动的城市交通流量智能预测系统[J]. 自动化学报, 2017, 43(6): 1006-1014.
- 赵丽, 陈晓红. 社交媒体数据在城市交通流量预测中的辅助作用[J]. 情报杂志, 2016, 35(12): 134-139.
- 刘洋, 张伟. 基于机器学习的城市交通流量短期预测研究[J]. 计算机应用研究, 2015, 32(10): 2984-2987.
- 李明, 王建军. 大数据技术在城市交通管理中的应用与展望[J]. 交通运输工程学报, 2014, 14(6): 105-115.
- 张晓芳, 李宏伟. 城市交通流量预测模型的比较与分析[J]. 系统工程理论与实践, 2013, 33(8): 2055-2062.
部分成果展示:
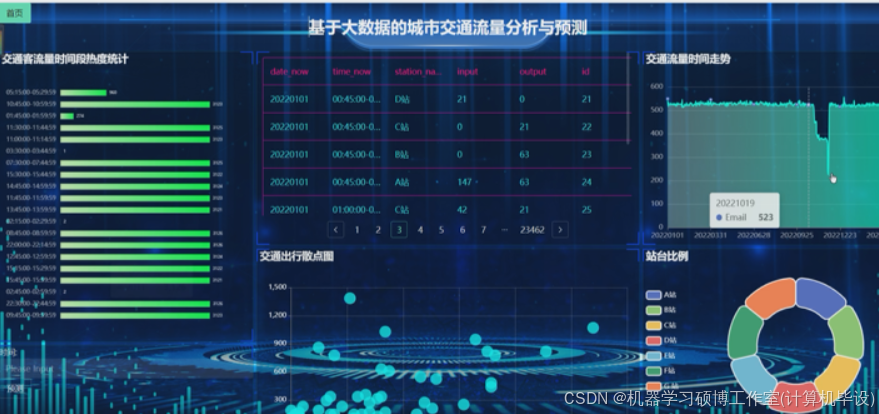
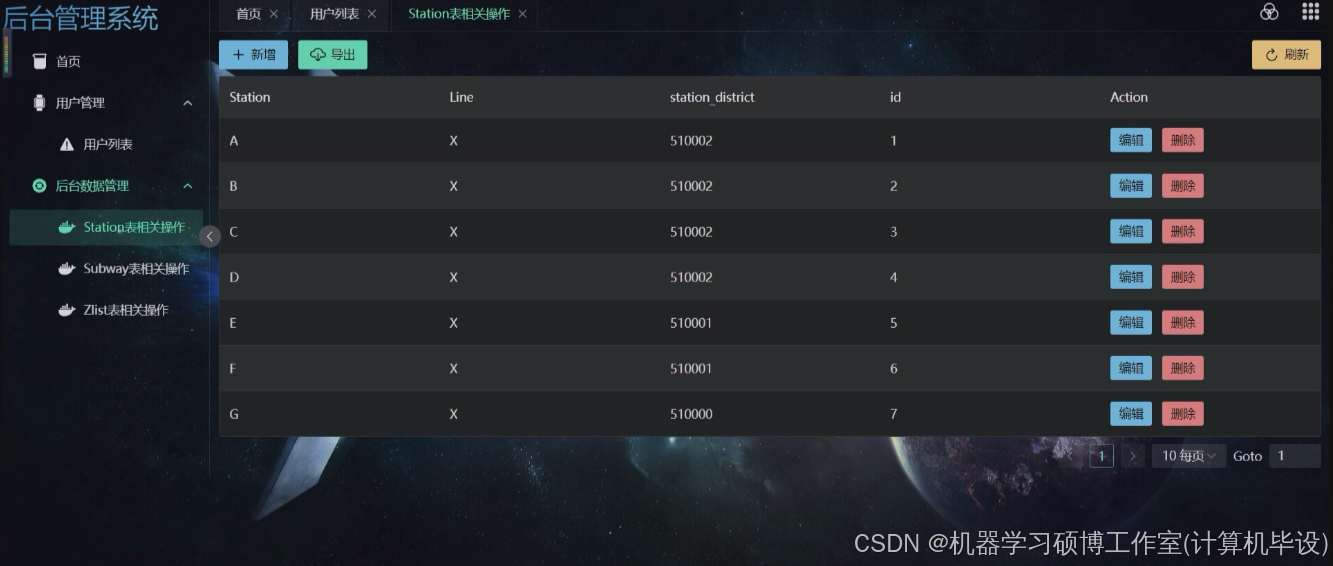
联系我们
如果需要相关论文或者源码可以添加VX联系我们哦~
专注计算机毕设多年的工作室~