论文地址:http://papers.nips.cc/paper/4824-imagenet-classification-with-deep-convolutional-neural-networks.pdf
Fashion-MNIST数据集下载:https://github.com/zalandoresearch/fashion-mnist/tree/master/data/fashion
1.Fashion-MNIST数据集介绍
Fashion-MNIST是一个替代MNIST手写数字集的图像数据集。 它是由Zalando(一家德国的时尚科技公司)旗下的研究部门提供。其涵盖了来自10种类别的共7万个不同商品的正面图片。Fashion-MNIST的大小、格式和训练集/测试集划分与原始的MNIST完全一致。60000/10000的训练测试数据划分,28x28的灰度图片。你可以直接用它来测试你的机器学习和深度学习算法性能,且不需要改动任何的代码。
这个数据集的样子大致如下(每个类别占三行):
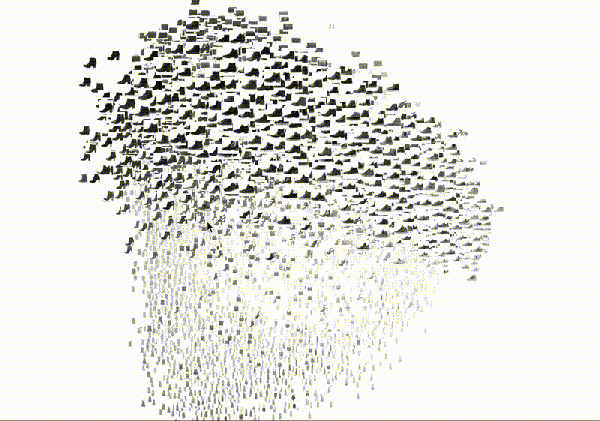
取代MNIST数据集的原因由如下几个:
- MNIST太简单了。 很多深度学习算法在测试集上的准确率已经达到99.6%!不妨看看我们基于scikit-learn上对经典机器学习算法的评测 和这段代码: "Most pairs of MNIST digits can be distinguished pretty well by just one pixel"(翻译:大多数MNIST只需要一个像素就可以区分开!)
- MNIST被用烂了。 参考:"Ian Goodfellow wants people to move away from mnist"(翻译:Ian Goodfellow希望人们不要再用MNIST了。)
- MNIST数字识别的任务不代表现代机器学习。 参考:"François Cholle: Ideas on MNIST do not transfer to real CV" (翻译:在MNIST上看似有效的想法没法迁移到真正的机器视觉问题上。)
1.1.获取数据
git clone git@github.com:zalandoresearch/fashion-mnist.git
1.2.类别标注
每个训练和测试样本都按照以下类别进行了标注:
2.AlexNet深度卷积神经网络
AlexNet是2012年ImageNet竞赛冠军获得者Hinton和他的学生Alex Krizhevsky设计的。也是在那年之后,更多的更深的神经网路被提出,比如优秀的vgg,GoogLeNet。 这对于传统的机器学习分类算法而言,已经相当的出色。
2.1.模型简介
AlexNet中包含了几个比较新的技术点,也首次在CNN中成功应用了ReLU、Dropout和LRN等Trick。同时AlexNet也使用了GPU进行运算加速。
AlexNet将LeNet的思想发扬光大,把CNN的基本原理应用到了很深很宽的网络中。AlexNet主要使用到的新技术点如下:
(1)成功使用ReLU作为CNN的激活函数,并验证其效果在较深的网络超过了Sigmoid,成功解决了Sigmoid在网络较深时的梯度弥散问题。虽然ReLU激活函数在很久之前就被提出了,但是直到AlexNet的出现才将其发扬光大。
(2)训练时使用Dropout随机忽略一部分神经元,以避免模型过拟合。Dropout虽有单独的论文论述,但是AlexNet将其实用化,通过实践证实了它的效果。在AlexNet中主要是最后几个全连接层使用了Dropout。
(3)在CNN中使用重叠的最大池化。此前CNN中普遍使用平均池化,AlexNet全部使用最大池化,避免平均池化的模糊化效果。并且AlexNet中提出让步长比池化核的尺寸小,这样池化层的输出之间会有重叠和覆盖,提升了特征的丰富性。
(4)提出了LRN层,对局部神经元的活动创建竞争机制,使得其中响应比较大的值变得相对更大,并抑制其他反馈较小的神经元,增强了模型的泛化能力。
(5)使用CUDA加速深度卷积网络的训练,利用GPU强大的并行计算能力,处理神经网络训练时大量的矩阵运算。AlexNet使用了两块GTX 580 GPU进行训练,单个GTX 580只有3GB显存,这限制了可训练的网络的最大规模。因此作者将AlexNet分布在两个GPU上,在每个GPU的显存中储存一半的神经元的参数。因为GPU之间通信方便,可以互相访问显存,而不需要通过主机内存,所以同时使用多块GPU也是非常高效的。同时,AlexNet的设计让GPU之间的通信只在网络的某些层进行,控制了通信的性能损耗。
(6)数据增强,随机地从256*256的原始图像中截取224*224大小的区域(以及水平翻转的镜像),相当于增加了2*(256-224)^2=2048倍的数据量。如果没有数据增强,仅靠原始的数据量,参数众多的CNN会陷入过拟合中,使用了数据增强后可以大大减轻过拟合,提升泛化能力。进行预测时,则是取图片的四个角加中间共5个位置,并进行左右翻转,一共获得10张图片,对他们进行预测并对10次结果求均值。同时,AlexNet论文中提到了会对图像的RGB数据进行PCA处理,并对主成分做一个标准差为0.1的高斯扰动,增加一些噪声,这个Trick可以让错误率再下降1%。
2.2.AlexNet特点
2.2.1.使用了Relu激活函数
基于ReLU的深度卷积网络比基于tanh和sigmoid的网络训练快数倍。
2.2.2.标准化
使用ReLU 后,会发现激活函数之后的值没有了tanh、sigmoid函数那样有一个值域区间,所以一般在ReLU之后会做一个normalization,LRU就是稳重提出一种方法,在神经科学中有个概念叫“Lateral inhibition”,讲的是活跃的神经元对它周边神经元的影响。
2.2.3.Dropout
Dropout也是经常说的一个概念,能够比较有效地防止神经网络的过拟合。 相对于一般如线性模型使用正则的方法来防止模型过拟合,而在神经网络中Dropout通过修改神经网络本身结构来实现。对于某一层神经元,通过定义的概率来随机删除一些神经元,同时保持输入层与输出层神经元的个人不变,然后按照神经网络的学习方法进行参数更新,下一次迭代中,重新随机删除一些神经元,直至训练结束。
3.AlexNet的TensorFlow实现
3.1.预备工作
import tensorflow as tf
from tensorflow.examples.tutorials.mnist import input_data
# 1.载入数据集
mnist = input_data.read_data_sets('./data/MNIST_data/', one_hot=True)
# 2.定义超参数(学习率、迭代次数、批(batch)大小、显示打印数据step)
learning_rate = 0.001
training_iters = 200000
batch_size = 128
display_step = 10
# 3.定义网络参数(输入维度,输出种类,dropout概率参数)
n_input = 784
n_classes = 10
dropout = 0.75
# 4.定义占位符,等待喂数据
x = tf.placeholder(tf.float32, [None, n_input])
y = tf.placeholder(tf.float32, [None, n_classes])
keep_prob = tf.placeholder(tf.float32) # dropout参数的占位符
# 5.定义卷积操作
def conv2d(name, x, W, b, strides=1):
x = tf.nn.conv2d(x, W, strides=[1, strides, strides, 1], padding='SAME')
x = tf.nn.bias_add(x, b)
return tf.nn.relu(x, name=name)
# 6.定义池化操作
def maxpool2d(name, x, k=2):
return tf.nn.max_pool(x, ksize=[1, k, k, 1], strides=[1, k, k, 1], padding='SAME', name=name)
# 7.规范化操作
def norm(name, l_input, lsize=4):
return tf.nn.lrn(l_input, lsize, bias=1.0, alpha=0.001/9.0, beta=0.75, name=name)
3.2.初始化网络参数
根据论文中的网络结构图(下图)对网络参数进行定义:
# 8.定义所有的网络参数
weights = {
'wc1':tf.Variable(tf.random_normal([11, 11, 1, 96])),# 因为mnist数据集的图像channel为1,故卷积核为11*11*1,个数为96
'wc2':tf.Variable(tf.random_normal([5, 5, 96, 256])),# 因为论文中的卷积在两个GPU上进行计算,笔记本只有一个GPU,
'wc3':tf.Variable(tf.random_normal([3, 3, 256, 384])), # 所以每层卷积的数量为论文中的2倍。
'wc4':tf.Variable(tf.random_normal([3, 3, 384, 384])),
'wc5':tf.Variable(tf.random_normal([3, 3, 384, 256])),
'wd1':tf.Variable(tf.random_normal([4*4*256, 4096])), # 第一个全连接网络的输入为 4096
'wd2':tf.Variable(tf.random_normal([4096, 1024])),
'out':tf.Variable(tf.random_normal([1024, 10])) # 输出为 10 个类别
}
biases = {
'bc1':tf.Variable(tf.random_normal([96])),
'bc2':tf.Variable(tf.random_normal([256])),
'bc3':tf.Variable(tf.random_normal([384])),
'bc4':tf.Variable(tf.random_normal([384])),
'bc5':tf.Variable(tf.random_normal([256])),
'bd1':tf.Variable(tf.random_normal([4096])),
'bd2':tf.Variable(tf.random_normal([1024])),
'out':tf.Variable(tf.random_normal([n_classes]))
}
定义 AlexNet 的网络模型:
# 定义整个网络
def alex_net(x, weights, biases, dropout):
x = tf.reshape(x, shape=[-1, 28, 28, 1])
# 第一层卷积
conv1 = conv2d('conv1', x, weights['wc1'], biases['bc1'])
# 最大池化——下采样
pool1 = maxpool2d('pool1', conv1, k=2)
# 规范化——数据归一化
norm1 = norm('norm1', pool1, lsize=4)
# 第二层卷积
conv2 = conv2d('conv2', norm1, weights['wc2'], biases['bc2'])
# 最大池化——下采样
pool2 = maxpool2d('pool2', conv2, k=2)
# 规范化——数据归一化
norm2 = norm('norm2', pool2, lsize=4)
# 第三层卷积
conv3 = conv2d('conv3', norm2, weights['wc3'], biases['bc3'])
pool3 = maxpool2d('pool3', conv3, k=2)
norm3 = norm('norm3', pool3, lsize=4)
# 第四层卷积
conv4 = conv2d('conv4', norm3, weights['wc4'], biases['bc4'])
# 第五层卷积
conv5 = conv2d('conv5', conv4, weights['wc5'], biases['bc5'])
pool5 = maxpool2d('pool5', conv5, k=2)
norm5 = norm('norm5', pool5, lsize=4)
# 全卷积层 1
fc1 = tf.reshape(norm5, [-1, weights['wd1'].get_shape().as_list()[0]])
fc1 = tf.add(tf.matmul(fc1, weights['wd1']), biases['bd1'])
fc1 = tf.nn.relu(fc1)
# 使用Dropout技术
fc1 = tf.nn.dropout(fc1, dropout)
# 全卷积层 2
fc2 = tf.reshape(fc1, [-1, weights['wd2'].get_shape().as_list()[0]])
fc2 = tf.add(tf.matmul(fc2, weights['wd2']), biases['bd2'])
fc2 = tf.nn.relu(fc2)
fc2 = tf.nn.dropout(fc2, dropout)
# 输出
out = tf.add(tf.matmul(fc2, weights['out']), biases['out'])
return out
构建模型,定义损失函数和优化器,并构建评估函数
# 1.构建模型
pred = alex_net(x, weights, biases, keep_prob)
# 2.定义损失函数和优化器
cost = tf.reduce_mean(tf.nn.softmax_cross_entropy_with_logits(logits=pred, labels=y))
optimizer = tf.train.AdamOptimizer(learning_rate=learning_rate).minimize(cost)
# 3.评估函数
correct_pred = tf.equal(tf.arg_max(pred, 1), tf.arg_max(y, 1))
accuracy = tf.reduce_mean(tf.cast(correct_pred, tf.float32))
训练模型和评估模型
# 初始化变量
init = tf.global_variables_initializer()
with tf.Session() as sess:
sess.run(init)
step = 1
while step * batch_size < training_iters:
batch_x, batch_y = mnist.train.next_batch(batch_size)
sess.run(optimizer, feed_dict={x: batch_x, y: batch_y, keep_prob: dropout})
if step % display_step == 0:
loss,acc = sess.run([cost,accuracy], feed_dict={x: batch_x, y: batch_y, keep_prob: 1.})
print("Iter"+str(step*batch_size)+",Minibatch Loss="+"{:.6f}".format(loss)+",Training Accuracy="+"{:.5f}".format(acc))
step += 1
print('Optimization Finishion!')
print("Testing Accuracy:",sess.run(accuracy, feed_dict={x: mnist.test.images[:256],y: mnist.test.labels[:256],keep_prob: 1.}))
结果显示:
.......
Iter 373760, Minibatch Loss= 7115.613770, Training Accuracy= 0.81641
Iter 375040, Minibatch Loss= 5161.097656, Training Accuracy= 0.85547
Iter 376320, Minibatch Loss= 8036.062988, Training Accuracy= 0.81250
Iter 377600, Minibatch Loss= 5098.235352, Training Accuracy= 0.85547
Iter 378880, Minibatch Loss= 6332.221680, Training Accuracy= 0.85547
Iter 380160, Minibatch Loss= 8099.681641, Training Accuracy= 0.81641
Iter 381440, Minibatch Loss= 7120.978516, Training Accuracy= 0.81250
Iter 382720, Minibatch Loss= 8811.558594, Training Accuracy= 0.80469
Iter 384000, Minibatch Loss= 7238.435547, Training Accuracy= 0.83594
Iter 385280, Minibatch Loss= 6391.473633, Training Accuracy= 0.82812
Iter 386560, Minibatch Loss= 5670.675781, Training Accuracy= 0.80859
Iter 387840, Minibatch Loss= 4547.402832, Training Accuracy= 0.83984
Iter 389120, Minibatch Loss= 8333.980469, Training Accuracy= 0.77734
Iter 390400, Minibatch Loss= 5622.814453, Training Accuracy= 0.83203
Iter 391680, Minibatch Loss= 6032.568359, Training Accuracy= 0.83594
Iter 392960, Minibatch Loss= 7194.363770, Training Accuracy= 0.83203
Iter 394240, Minibatch Loss= 5317.426270, Training Accuracy= 0.85938
Iter 395520, Minibatch Loss= 6857.503418, Training Accuracy= 0.80078
Iter 396800, Minibatch Loss= 6782.902344, Training Accuracy= 0.82031
Iter 398080, Minibatch Loss= 6809.053711, Training Accuracy= 0.82812
Iter 399360, Minibatch Loss= 6525.162109, Training Accuracy= 0.82031
Optimization Finished!
Testing Accuracy: 0.82421875
完整代码:
# -*- coding: utf-8 -*-
# @Time : 2019/3/11 13:12
# @Author : Chaucer_Gxm
# @Email : gxm4167235@163.com
# @File : AlexNet.py
# @GitHub : https://github.com/Chaucergit/Code-and-Algorithm
# @blog : https://blog.csdn.net/qq_24819773
# @Software: PyCharm
import tensorflow as tf
# 输入数据
from tensorflow.examples.tutorials.mnist import input_data
mnist = input_data.read_data_sets("./data/MNIST_data", one_hot=True)
# 定义网络的超参数
learning_rate = 0.0001
training_iters = 400000
batch_size = 256
display_step = 5
# 定义网络的参数
n_input = 784 # 输入的维度 (img shape: 28*28)
n_classes = 10 # 标记的维度 (0-9 digits)
dropout = 0.5 # Dropout的概率,输出的可能性
# 输入占位符
x = tf.placeholder(tf.float32, [None, n_input])
y = tf.placeholder(tf.float32, [None, n_classes])
keep_prob = tf.placeholder(tf.float32) # dropout (keep probability)
# 定义卷积操作
def conv2d(name, x, W, b, strides=1):
# Conv2D wrapper, with bias and relu activation
x = tf.nn.conv2d(x, W, strides=[1, strides, strides, 1], padding='SAME')
x = tf.nn.bias_add(x, b)
return tf.nn.relu(x, name=name) # 使用relu激活函数
# 定义池化层操作
def maxpool2d(name, x, k=2):
# MaxPool2D wrapper
return tf.nn.max_pool(x, ksize=[1, k, k, 1], strides=[1, k, k, 1],
padding='SAME', name=name)
# 规范化操作
def norm(name, l_input, lsize=4):
return tf.nn.lrn(l_input, lsize, bias=1.0, alpha=0.001 / 9.0,
beta=0.75, name=name)
# 定义所有的网络参数
weights = {
'wc1': tf.Variable(tf.random_normal([11, 11, 1, 96])),
'wc2': tf.Variable(tf.random_normal([5, 5, 96, 256])),
'wc3': tf.Variable(tf.random_normal([3, 3, 256, 384])),
'wc4': tf.Variable(tf.random_normal([3, 3, 384, 384])),
'wc5': tf.Variable(tf.random_normal([3, 3, 384, 256])),
'wd1': tf.Variable(tf.random_normal([4*4*256, 4096])),
'wd2': tf.Variable(tf.random_normal([4096, 4096])),
'out': tf.Variable(tf.random_normal([4096, n_classes]))
}
biases = {
'bc1': tf.Variable(tf.random_normal([96])),
'bc2': tf.Variable(tf.random_normal([256])),
'bc3': tf.Variable(tf.random_normal([384])),
'bc4': tf.Variable(tf.random_normal([384])),
'bc5': tf.Variable(tf.random_normal([256])),
'bd1': tf.Variable(tf.random_normal([4096])),
'bd2': tf.Variable(tf.random_normal([4096])),
'out': tf.Variable(tf.random_normal([n_classes]))
}
# 定义整个网络
def alex_net(x, weights, biases, dropout):
# 向量转为矩阵 Reshape input picture
x = tf.reshape(x, shape=[-1, 28, 28, 1])
# 第一层卷积
# 卷积
conv1 = conv2d('conv1', x, weights['wc1'], biases['bc1'])
# 下采样
pool1 = maxpool2d('pool1', conv1, k=2)
# 规范化
norm1 = norm('norm1', pool1, lsize=4)
# 第二层卷积
# 卷积
conv2 = conv2d('conv2', norm1, weights['wc2'], biases['bc2'])
# 最大池化(向下采样)
pool2 = maxpool2d('pool2', conv2, k=2)
# 规范化
norm2 = norm('norm2', pool2, lsize=4)
# 第三层卷积
# 卷积
conv3 = conv2d('conv3', norm2, weights['wc3'], biases['bc3'])
# 规范化
norm3 = norm('norm3', conv3, lsize=4)
# 第四层卷积
conv4 = conv2d('conv4', norm3, weights['wc4'], biases['bc4'])
# 第五层卷积
conv5 = conv2d('conv5', conv4, weights['wc5'], biases['bc5'])
# 最大池化(向下采样)
pool5 = maxpool2d('pool5', conv5, k=2)
# 规范化
norm5 = norm('norm5', pool5, lsize=4)
# 全连接层1
fc1 = tf.reshape(norm5, [-1, weights['wd1'].get_shape().as_list()[0]])
fc1 =tf.add(tf.matmul(fc1, weights['wd1']), biases['bd1'])
fc1 = tf.nn.relu(fc1)
# dropout
fc1 = tf.nn.dropout(fc1, dropout)
# 全连接层2
fc2 = tf.reshape(fc1, [-1, weights['wd2'].get_shape().as_list()[0]])
fc2 = tf.add(tf.matmul(fc2, weights['wd2']), biases['bd2'])
fc2 = tf.nn.relu(fc2)
# dropout
fc2=tf.nn.dropout(fc2, dropout)
# 输出层
out = tf.add(tf.matmul(fc2, weights['out']), biases['out'])
return out
# 构建模型
pred = alex_net(x, weights, biases, keep_prob)
# 定义损失函数和优化器
cost = tf.reduce_mean(tf.nn.softmax_cross_entropy_with_logits(logits=pred, labels=y))
optimizer = tf.train.AdamOptimizer(learning_rate=learning_rate).minimize(cost)
# 评估函数
correct_pred = tf.equal(tf.argmax(pred, 1), tf.argmax(y, 1))
accuracy = tf.reduce_mean(tf.cast(correct_pred, tf.float32))
# 初始化变量
init = tf.global_variables_initializer()
import os
ckpt_dir = './tmp/ckpt_dir'
if not os.path.exists(ckpt_dir):
os.makedirs(ckpt_dir)
# 定义一个提取器
saver = tf.train.Saver()
# 开启一个训练
with tf.Session() as sess:
sess.run(init)
step = 1
# 开始训练,直到达到training_iters,即200000
while step * batch_size < training_iters:
# 获取批量数据
batch_x, batch_y = mnist.train.next_batch(batch_size)
sess.run(optimizer, feed_dict={x: batch_x, y: batch_y, keep_prob: dropout})
if step % display_step == 0:
# 计算损失值和准确度,输出
loss, acc = sess.run([cost, accuracy], feed_dict={x: batch_x, y: batch_y, keep_prob: 1.})
print("Iter " + str(step*batch_size) + ", Minibatch Loss= " + "{:.6f}".format(loss) + ", Training Accuracy= " + "{:.5f}".format(acc))
step += 1
print("Optimization Finished!")
# 计算测试集的精确度
print("Testing Accuracy:", sess.run(accuracy, feed_dict={x: mnist.test.images[:256], y: mnist.test.labels[:256], keep_prob: 1.}))
saver.save(sess, './tmp/ckpt_dir/model.ckpt')
参考文献:
[1].Tensorflow实战[M] 黄文坚, 唐源
[2].Tensorflow技术解析与实战[M] 李嘉璇
[3].https://baike.baidu.com/item/AlexNet/22689612?fr=aladdin
[4].Tensorflow实战Google深度学习框架[M].郑泽宇,梁博文,顾思宇