目录
3. 分别使用nn.RNNCell、nn.RNN实现SRN编辑
5. 实现“Character-Level Language Models”源代码(必做)
1. 使用Numpy实现SRN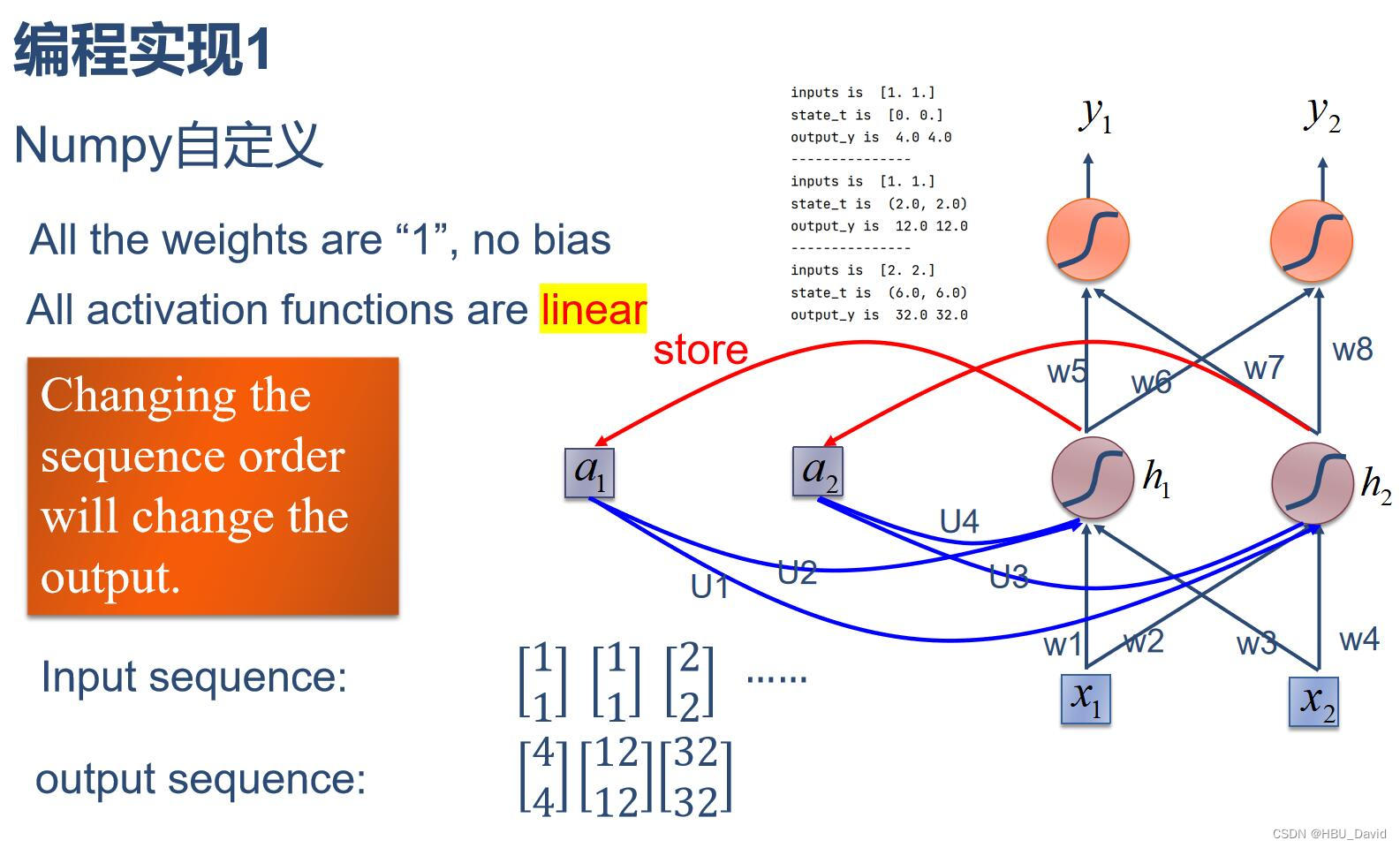
import numpy as np
inputs = np.array([[1., 1.],
[1., 1.],
[2., 2.]]) # 初始化输入序列
print('inputs is ', inputs)
state_t = np.zeros(2, ) # 初始化存储器
print('state_t is ', state_t)
w1, w2, w3, w4, w5, w6, w7, w8 = 1., 1., 1., 1., 1., 1., 1., 1.
U1, U2, U3, U4 = 1., 1., 1., 1.
print('--------------------------------------')
for input_t in inputs:
print('inputs is ', input_t)
print('state_t is ', state_t)
in_h1 = np.dot([w1, w3], input_t) + np.dot([U2, U4], state_t)
in_h2 = np.dot([w2, w4], input_t) + np.dot([U1, U3], state_t)
state_t = in_h1, in_h2
output_y1 = np.dot([w5, w7], [in_h1, in_h2])
output_y2 = np.dot([w6, w8], [in_h1, in_h2])
print('output_y is ', output_y1, output_y2)
print('---------------')
得到以下结果:
C:\Users\DELL\.conda\envs\pytorch\python.exe C:/Users/DELL/PycharmProjects/pythonProject/CSDN/作业8.py
inputs is [[1. 1.]
[1. 1.]
[2. 2.]]
state_t is [0. 0.]
--------------------------------------
inputs is [1. 1.]
state_t is [0. 0.]
output_y is 4.0 4.0
---------------
inputs is [1. 1.]
state_t is (2.0, 2.0)
output_y is 12.0 12.0
---------------
inputs is [2. 2.]
state_t is (6.0, 6.0)
output_y is 32.0 32.0
---------------
进程已结束,退出代码为 0
2. 在1的基础上,增加激活函数tanh
import numpy as np
inputs = np.array([[1., 1.],
[1., 1.],
[2., 2.]]) # 初始化输入序列
print('inputs is ', inputs)
state_t = np.zeros(2, ) # 初始化存储器
print('state_t is ', state_t)
w1, w2, w3, w4, w5, w6, w7, w8 = 1., 1., 1., 1., 1., 1., 1., 1.
U1, U2, U3, U4 = 1., 1., 1., 1.
print('--------------------------------------')
for input_t in inputs:
print('inputs is ', input_t)
print('state_t is ', state_t)
in_h1 = np.tanh(np.dot([w1, w3], input_t) + np.dot([U2, U4], state_t))
in_h2 = np.tanh(np.dot([w2, w4], input_t) + np.dot([U1, U3], state_t))
state_t = in_h1, in_h2
output_y1 = np.dot([w5, w7], [in_h1, in_h2])
output_y2 = np.dot([w6, w8], [in_h1, in_h2])
print('output_y is ', output_y1, output_y2)
print('---------------')
得到以下结果:
C:\Users\DELL\.conda\envs\pytorch\python.exe C:/Users/DELL/PycharmProjects/pythonProject/CSDN/作业8.py
inputs is [[1. 1.]
[1. 1.]
[2. 2.]]
state_t is [0. 0.]
--------------------------------------
inputs is [1. 1.]
state_t is [0. 0.]
output_y is 1.9280551601516338 1.9280551601516338
---------------
inputs is [1. 1.]
state_t is (0.9640275800758169, 0.9640275800758169)
output_y is 1.9984510891336251 1.9984510891336251
---------------
inputs is [2. 2.]
state_t is (0.9992255445668126, 0.9992255445668126)
output_y is 1.9999753470497836 1.9999753470497836
---------------
进程已结束,退出代码为 0
3. 分别使用nn.RNNCell、nn.RNN实现SRN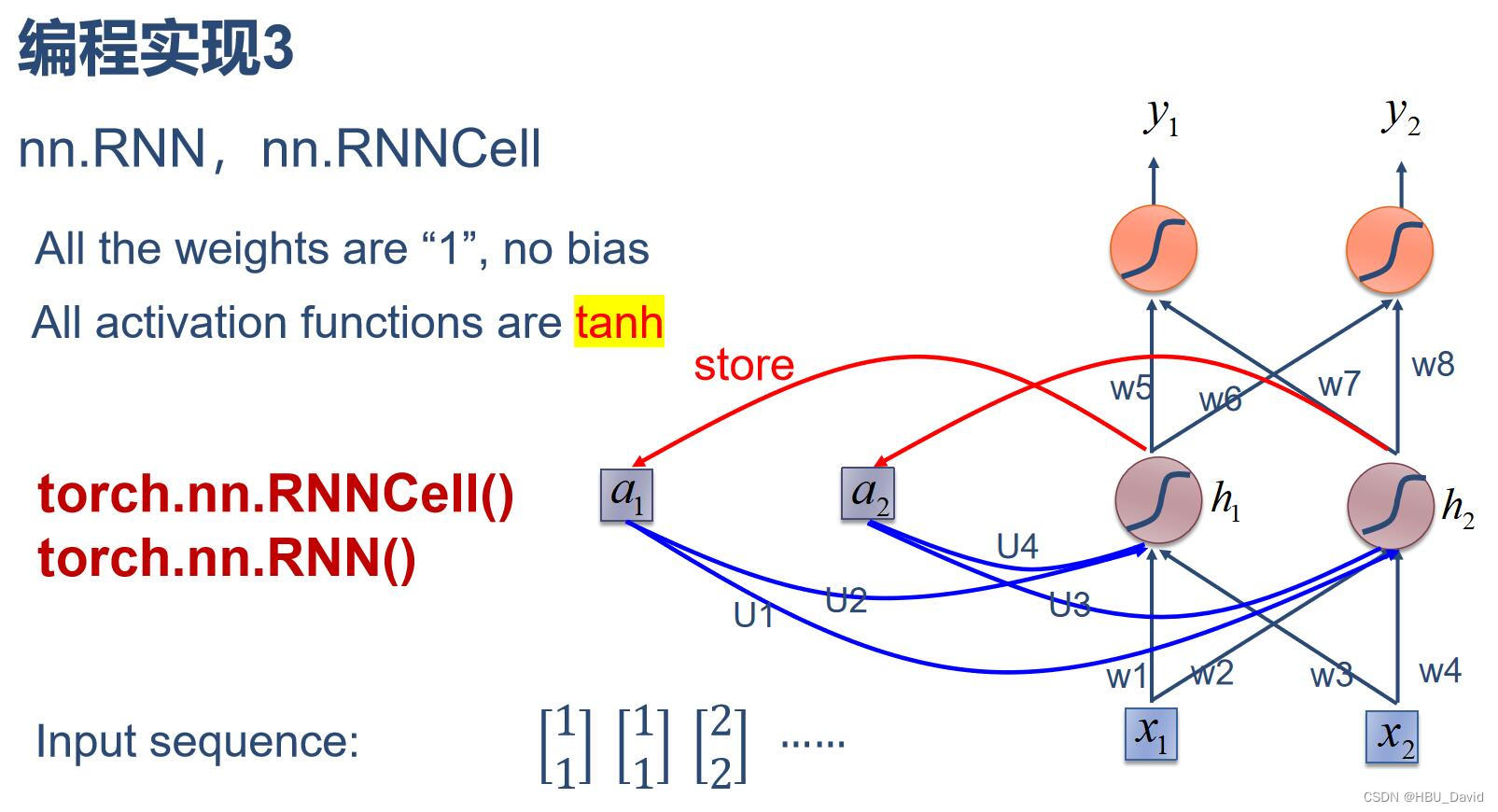
nn.RNNcell:
import torch
batch_size = 1
seq_len = 3 # 序列长度
input_size = 2 # 输入序列维度
hidden_size = 2 # 隐藏层维度
output_size = 2 # 输出层维度
# RNNCell
cell = torch.nn.RNNCell(input_size=input_size, hidden_size=hidden_size)
# 初始化参数 https://zhuanlan.zhihu.com/p/342012463
for name, param in cell.named_parameters():
if name.startswith("weight"):
torch.nn.init.ones_(param)
else:
torch.nn.init.zeros_(param)
# 线性层
liner = torch.nn.Linear(hidden_size, output_size)
liner.weight.data = torch.Tensor([[1, 1], [1, 1]])
liner.bias.data = torch.Tensor([0.0])
seq = torch.Tensor([[[1, 1]],
[[1, 1]],
[[2, 2]]])
hidden = torch.zeros(batch_size, hidden_size)
output = torch.zeros(batch_size, output_size)
for idx, input in enumerate(seq):
print('=' * 20, idx, '=' * 20)
print('Input :', input)
print('hidden :', hidden)
hidden = cell(input, hidden)
output = liner(hidden)
print('output :', output)
得到以下结果:
C:\Users\DELL\.conda\envs\pytorch\python.exe C:/Users/DELL/PycharmProjects/pythonProject/CSDN/作业8.py
==================== 0 ====================
Input : tensor([[1., 1.]])
hidden : tensor([[0., 0.]])
output : tensor([[1.9281, 1.9281]], grad_fn=<AddmmBackward0>)
==================== 1 ====================
Input : tensor([[1., 1.]])
hidden : tensor([[0.9640, 0.9640]], grad_fn=<TanhBackward0>)
output : tensor([[1.9985, 1.9985]], grad_fn=<AddmmBackward0>)
==================== 2 ====================
Input : tensor([[2., 2.]])
hidden : tensor([[0.9992, 0.9992]], grad_fn=<TanhBackward0>)
output : tensor([[2.0000, 2.0000]], grad_fn=<AddmmBackward0>)
进程已结束,退出代码为 0
nn.RNN:
import torch
batch_size = 1
seq_len = 3
input_size = 2
hidden_size = 2
num_layers = 1
output_size = 2
cell = torch.nn.RNN(input_size=input_size, hidden_size=hidden_size, num_layers=num_layers)
for name, param in cell.named_parameters(): # 初始化参数
if name.startswith("weight"):
torch.nn.init.ones_(param)
else:
torch.nn.init.zeros_(param)
# 线性层
liner = torch.nn.Linear(hidden_size, output_size)
liner.weight.data = torch.Tensor([[1, 1], [1, 1]])
liner.bias.data = torch.Tensor([0.0])
inputs = torch.Tensor([[[1, 1]],
[[1, 1]],
[[2, 2]]])
hidden = torch.zeros(num_layers, batch_size, hidden_size)
out, hidden = cell(inputs, hidden)
print('Input :', inputs[0])
print('hidden:', 0, 0)
print('Output:', liner(out[0]))
print('--------------------------------------')
print('Input :', inputs[1])
print('hidden:', out[0])
print('Output:', liner(out[1]))
print('--------------------------------------')
print('Input :', inputs[2])
print('hidden:', out[1])
print('Output:', liner(out[2]))
得到以下结果:
C:\Users\DELL\.conda\envs\pytorch\python.exe C:/Users/DELL/PycharmProjects/pythonProject/CSDN/作业8.py
Input : tensor([[1., 1.]])
hidden: 0 0
Output: tensor([[1.9281, 1.9281]], grad_fn=<AddmmBackward0>)
--------------------------------------
Input : tensor([[1., 1.]])
hidden: tensor([[0.9640, 0.9640]], grad_fn=<SelectBackward0>)
Output: tensor([[1.9985, 1.9985]], grad_fn=<AddmmBackward0>)
--------------------------------------
Input : tensor([[2., 2.]])
hidden: tensor([[0.9992, 0.9992]], grad_fn=<SelectBackward0>)
Output: tensor([[2.0000, 2.0000]], grad_fn=<AddmmBackward0>)
进程已结束,退出代码为 0
4. 分析“二进制加法” 源代码(选做)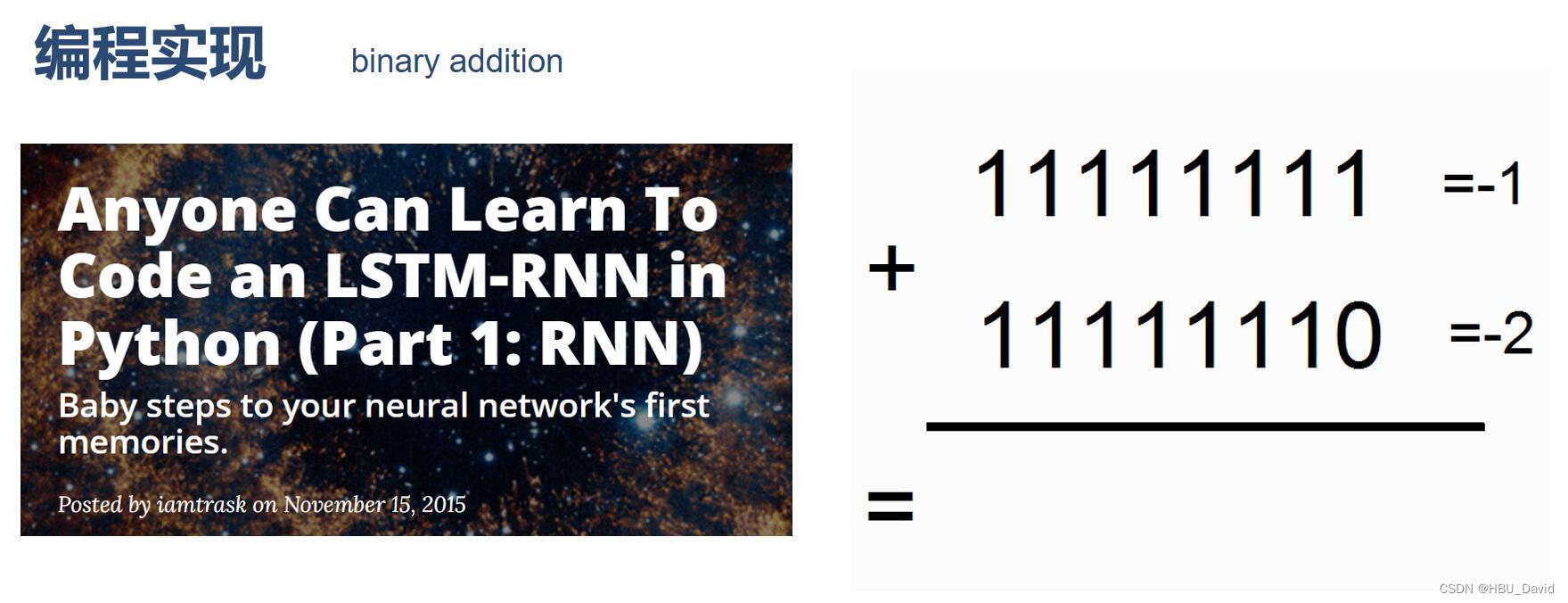
import copy, numpy as np
np.random.seed(0)
# 定义sigmoid函数
def sigmoid(x):
output = 1 / (1 + np.exp(-x))
return output
# 计算sigmoid函数的导数
def sigmoid_output_to_derivative(output):
return output * (1 - output)
# 生成要计算的二进制数据
int2binary = {} # 用于将输入的整数转为计算机可运行的二进制数用
binary_dim = 8 # 定义了二进制数的长度=8
largest_number = pow(2, binary_dim) # 二进制数最大能取的数就=256喽
binary = np.unpackbits(
np.array([range(largest_number)], dtype=np.uint8).T, axis=1)
for i in range(largest_number): # 将二进制数与十进制数做个一一对应的字典
int2binary[i] = binary[i]
# 初始参数
alpha = 0.1 # 反向传播时参数w更新的速度
input_dim = 2 # 输入数据的维度,程序是实现两个数相加的
hidden_dim = 16 # 隐藏层神经元个数=16
output_dim = 1 # 输出结果值是1维的
# 初始化神经网络的权重参数
synapse_0 = 2 * np.random.random((input_dim, hidden_dim)) - 1 # 输入层权值,维度为2X16,取值约束在[-1,1]间
synapse_1 = 2 * np.random.random((hidden_dim, output_dim)) - 1 # 隐层权值,维度为16X1,取值约束在[-1,1]间
synapse_h = 2 * np.random.random((hidden_dim, hidden_dim)) - 1 # 循环层,维度为16X16,取值约束在[-1,1]间
synapse_0_update = np.zeros_like(synapse_0) # 初始化增量矩阵
synapse_1_update = np.zeros_like(synapse_1)
synapse_h_update = np.zeros_like(synapse_h)
# training logic
for j in range(10000): # 模型迭代次数,可自行更改
# 随机生成相加的数,并将其转换为二进制数
# a_int 为十进制 且小于128, a为二进制
a_int = np.random.randint(largest_number / 2)
a = int2binary[a_int]
b_int = np.random.randint(largest_number / 2)
b = int2binary[b_int]
# c 为实际值
c_int = a_int + b_int # 真实和
c = int2binary[c_int]
# d 为预测值
d = np.zeros_like(c)
overallError = 0 # 打印显示误差
layer_2_deltas = list() # 反向求导用
layer_1_values = list()
# 先对隐藏层前一时刻状态初始化为 [0,0,0,,,,*16]
layer_1_values.append(np.zeros(hidden_dim))
# 前向传播;二进制求和,低位在右,高位在左 以此方向为正向
for position in range(binary_dim):
# 从最右边的数开始求和,所以索引要倒着写(从第七个开始求和)
X = np.array([[a[binary_dim - position - 1], b[binary_dim - position - 1]]])
# 输入的a与b(二进制形式) 1*2
y = np.array([[c[binary_dim - position - 1]]]).T # 真实label值 二进制
# 隐层输出 1*2 * 2*16 + 1*16 * 16*16 = 1*16
layer_1 = sigmoid(np.dot(X, synapse_0) + np.dot(layer_1_values[-1], synapse_h)) # X*w0+RNN前一时刻状态值*wh
# 输出层 1*16 * 16*1 = 1*1
layer_2 = sigmoid(np.dot(layer_1, synapse_1))
# 求误差
layer_2_error = y - layer_2
# 将layer_2_deltas 算出来 并存入列表( y - y_p )*f'(z) 其结果是一个数
layer_2_deltas.append((layer_2_error) * sigmoid_output_to_derivative(layer_2))
overallError += np.abs(layer_2_error[0]) # 误差,打印显示用
# a[7]+b[7]=d[7] 预测的和 循环结束后就会得到完整的二进制加法结果
d[binary_dim - position - 1] = np.round(layer_2[0][0])
# 深拷贝,将前向传播隐层输出保存起来
layer_1_values.append(copy.deepcopy(layer_1))
# 给记忆细胞赋初值 1*16 个0
future_layer_1_delta = np.zeros(hidden_dim)
# 反向传播,计算从左到右,即二进制高位到低位
for position in range(binary_dim):
X = np.array([[a[position], b[position]]]) # a[0],b[0]
# 因为从右往左是正向,所以此时拿前向传播中的隐层中第七位的值
layer_1 = layer_1_values[-position - 1]
# 拿到前向传播中的前一个值 layer_1_+1 便于后面对循环层的矩阵进行跟新
prev_layer_1 = layer_1_values[-position - 2]
# 拿出第七位的 layer_2_delta ,用于计算 layer_1_delta
layer_2_delta = layer_2_deltas[-position - 1]
# 计算 layer_1_delta , future_layer_1_delta初始值为0 与 Whh 相乘
layer_1_delta = (future_layer_1_delta.dot(synapse_h.T) + layer_2_delta.dot(
synapse_1.T)) * sigmoid_output_to_derivative(layer_1)
# 跟新权值增量 (atleast_2d 避免列向量导致无法计算的问题)
synapse_1_update += np.atleast_2d(layer_1).T.dot(layer_2_delta) # 对w1进行更新
synapse_h_update += np.atleast_2d(prev_layer_1).T.dot(layer_1_delta) # 对wh进行更新
synapse_0_update += X.T.dot(layer_1_delta) # 对w0进行更新
# 跟新记忆细胞中的值
future_layer_1_delta = layer_1_delta
# 跟新权值
synapse_0 += synapse_0_update * alpha
synapse_1 += synapse_1_update * alpha
synapse_h += synapse_h_update * alpha
synapse_0_update *= 0
synapse_1_update *= 0
synapse_h_update *= 0
# print out progress
if (j % 1000 == 0): # 每1000次打印结果
print("Error:" + str(overallError))
print("Pred:" + str(d))
print("True:" + str(c))
out = 0
for index, x in enumerate(reversed(d)):
out += x * pow(2, index)
print(str(a_int) + " + " + str(b_int) + " = " + str(out))
print("------------")
得到以下结果:
C:\Users\DELL\.conda\envs\pytorch\python.exe C:/Users/DELL/PycharmProjects/pythonProject/CSDN/作业8.py
Error:[3.45638663]
Pred:[0 0 0 0 0 0 0 1]
True:[0 1 0 0 0 1 0 1]
9 + 60 = 1
------------
Error:[3.63389116]
Pred:[1 1 1 1 1 1 1 1]
True:[0 0 1 1 1 1 1 1]
28 + 35 = 255
------------
Error:[3.91366595]
Pred:[0 1 0 0 1 0 0 0]
True:[1 0 1 0 0 0 0 0]
116 + 44 = 72
------------
Error:[3.72191702]
Pred:[1 1 0 1 1 1 1 1]
True:[0 1 0 0 1 1 0 1]
4 + 73 = 223
------------
Error:[3.5852713]
Pred:[0 0 0 0 1 0 0 0]
True:[0 1 0 1 0 0 1 0]
71 + 11 = 8
------------
Error:[2.53352328]
Pred:[1 0 1 0 0 0 1 0]
True:[1 1 0 0 0 0 1 0]
81 + 113 = 162
------------
Error:[0.57691441]
Pred:[0 1 0 1 0 0 0 1]
True:[0 1 0 1 0 0 0 1]
81 + 0 = 81
------------
Error:[1.42589952]
Pred:[1 0 0 0 0 0 0 1]
True:[1 0 0 0 0 0 0 1]
4 + 125 = 129
------------
Error:[0.47477457]
Pred:[0 0 1 1 1 0 0 0]
True:[0 0 1 1 1 0 0 0]
39 + 17 = 56
------------
Error:[0.21595037]
Pred:[0 0 0 0 1 1 1 0]
True:[0 0 0 0 1 1 1 0]
11 + 3 = 14
------------
进程已结束,退出代码为 0
5. 实现“Character-Level Language Models”源代码(必做)
翻译Character-Level Language Models 相关内容
字符级语言模型
好的,所以我们有一个关于RNN是什么,为什么它们超级令人兴奋的,以及它们是如何工作的。现在,我们将在一个有趣的应用程序中进行验证:我们将训练 RNN 字符级语言模型。也就是说,我们将给 RNN 一大块文本,并要求它对给定先前字符序列的序列中下一个字符的概率分布进行建模。然后,这将允许我们一次生成一个字符的新文本。
作为一个工作示例,假设我们只有四个可能的字母“helo”的词汇表,并且想在训练序列“hello”上训练一个RNN。这个训练序列实际上是 4 个独立训练示例的来源:1. 给定 “h” 上下文时,“e”的概率应该可能,2. “l”应该在“he”的上下文中出现,3. “l”也应该在给定“hel”的上下文中,最后是 4。“o”应该可能被赋予“地狱”的上下文。
具体来说,我们将使用 1-of-k 编码将每个字符编码到一个向量中(即除了词汇表中字符索引处的单个字符之外的所有字符均为零),并使用 step 函数一次将它们馈送到 RNN 中。然后,我们将观察一个 4 维输出向量序列(每个字符一个维度),我们将其解释为 RNN 当前分配给序列中下一个字符的置信度。下图如下:
具有 4 维输入和输出层的示例 RNN,以及 3 个单元(神经元)的隐藏层。此图显示了当 RNN 被输入字符“hell”时正向传递中的激活。输出层包含 RNN 为下一个字符分配的置信度(词汇表为 “h,e,l,o”);我们希望绿色数字高,红色数字低。
一个具有4维输入和输出层的RNN例子,以及一个由3个单元(神经元)组成的隐藏层。该图显示了当RNN被输入字符 "hell "时,在前向通道中的激活情况。输出层包含RNN为下一个字符(词汇为 "h,e,l,o")分配的置信度;我们希望绿色数字为高,红色数字为低。
例如,我们看到,在第一个时间步骤中,当RNN看到字符 "h "时,它给下一个字母 "h "分配了1.0的信心,给字母 "e "分配了2.2的信心,给 "l "分配了3.0的信心,给 "o "分配了4.1的信心。由于在我们的训练数据(字符串 "hello")中,下一个正确的字符是 "e",我们希望增加它的信心(绿色),减少所有其他字母的信心(红色)。同样,我们在4个时间步骤中的每一个步骤都有一个理想的目标字符,我们希望网络能赋予它更大的信心。由于RNN完全由可微调的操作组成,我们可以运行反向传播算法(这只是微积分中链式规则的递归应用),以找出我们应该向哪个方向调整它的每一个权重,以增加正确目标的分数(绿色粗体数字)。然后我们可以进行参数更新,在这个梯度方向上对每个权重进行微小的调整。如果我们在参数更新后给RNN提供相同的输入,我们会发现正确的字符(例如第一个时间步骤中的 "e")的得分会略高(例如2.3而不是2.2),而错误的字符的得分会略低。然后,我们多次重复这个过程,直到网络收敛,其预测结果最终与训练数据一致,即正确的字符总是被预测在下一个。
一个更技术性的解释是,我们在每个输出向量上同时使用标准的Softmax分类器(也通常被称为交叉熵损失)。RNN是用小型批次的随机梯度下降法训练的,我喜欢用RMSProp或Adam(每参数自适应学习率方法)来稳定更新。
还请注意,第一次输入字符 "l "时,目标是 "l",但第二次输入时,目标是 "o"。因此,RNN不能仅仅依靠输入,必须使用其递归连接来跟踪上下文以实现这一任务。
在测试时,我们将一个字符送入RNN,得到一个关于下一个可能出现的字符的分布。我们从这个分布中取样,并将其直接送回以获得下一个字母。重复这个过程,你就可以对文本进行取样了! 现在让我们在不同的数据集上训练一个RNN,看看会发生什么。
为了进一步说明问题,出于教育目的,我还用Python/numpy写了一个最小的字符级RNN语言模型。它只有大约100行,如果你更擅长阅读代码而不是文字的话,希望它能对上述内容做一个简明、具体和有用的总结。我们现在将深入研究用更有效的Lua/Torch代码库产生的例子结果。
import numpy as np
import jieba
# data I/O
data = open('input.txt', 'rb').read() # should be simple plain text file
data = data.decode('gbk')
data = list(jieba.cut(data, cut_all=False))
chars = list(set(data))
data_size, vocab_size = len(data), len(chars)
print('data has %d characters, %d unique.' % (data_size, vocab_size))
char_to_ix = {ch: i for i, ch in enumerate(chars)}
ix_to_char = {i: ch for i, ch in enumerate(chars)}
# hyperparameters
hidden_size = 200 # size of hidden layer of neurons
seq_length = 25 # number of steps to unroll the RNN for
learning_rate = 1e-1
# model parameters
Wxh = np.random.randn(hidden_size, vocab_size) * 0.01 # input to hidden
Whh = np.random.randn(hidden_size, hidden_size) * 0.01 # hidden to hidden
Why = np.random.randn(vocab_size, hidden_size) * 0.01 # hidden to output
bh = np.zeros((hidden_size, 1)) # hidden bias
by = np.zeros((vocab_size, 1)) # output bias
def lossFun(inputs, targets, hprev):
"""
inputs,targets are both list of integers.
hprev is Hx1 array of initial hidden state
returns the loss, gradients on model parameters, and last hidden state
"""
xs, hs, ys, ps = {}, {}, {}, {}
hs[-1] = np.copy(hprev) # hprev 中间层的值, 存作-1,为第一个做准备
loss = 0
# forward pass
for t in range(len(inputs)):
xs[t] = np.zeros((vocab_size, 1)) # encode in 1-of-k representation
xs[t][inputs[t]] = 1 # x[t] 是一个第t个输入单词的向量
# 双曲正切, 激活函数, 作用跟sigmoid类似
# h(t) = tanh(Wxh*X + Whh*h(t-1) + bh) 生成新的中间层
hs[t] = np.tanh(np.dot(Wxh, xs[t]) + np.dot(Whh, hs[t - 1]) + bh) # hidden state tanh
# y(t) = Why*h(t) + by
ys[t] = np.dot(Why, hs[t]) + by # unnormalized log probabilities for next chars
# softmax regularization
# p(t) = softmax(y(t))
ps[t] = np.exp(ys[t]) / np.sum(np.exp(ys[t])) # probabilities for next chars, 对输出作softmax
# loss += -log(value) 预期输出是1,因此这里的value值就是此次的代价函数,使用 -log(*) 使得离正确输出越远,代价函数就越高
loss += -np.log(ps[t][targets[t], 0]) # softmax (cross-entropy loss) 代价函数是交叉熵
# 将输入循环一遍以后,得到各个时间段的h, y 和 p
# 得到此时累积的loss, 准备进行更新矩阵
# backward pass: compute gradients going backwards
dWxh, dWhh, dWhy = np.zeros_like(Wxh), np.zeros_like(Whh), np.zeros_like(Why) # 各矩阵的参数进行
dbh, dby = np.zeros_like(bh), np.zeros_like(by)
dhnext = np.zeros_like(hs[0]) # 下一个时间段的潜在层,初始化为零向量
for t in reversed(range(len(inputs))): # 把时间作为维度,则梯度的计算应该沿着时间回溯
dy = np.copy(ps[t]) # 设dy为实际输出,而期望输出(单位向量)为y, 代价函数为交叉熵函数
dy[targets[t]] -= 1 # backprop into y. see http://cs231n.github.io/neural-networks-case-study/#grad if confused here
dWhy += np.dot(dy, hs[t].T) # dy * h(t).T h层值越大的项,如果错误,则惩罚越严重。反之,奖励越多(这边似乎没有考虑softmax的求导?)
dby += dy # 这个没什么可说的,与dWhy一样,只不过h项=1, 所以直接等于dy
dh = np.dot(Why.T, dy) + dhnext # backprop into h z_t = Why*H_t + b_y H_t = tanh(Whh*H_t-1 + Whx*X_t), 第一阶段求导
dhraw = (1 - hs[t] * hs[t]) * dh # backprop through tanh nonlinearity 第二阶段求导,注意tanh的求导
dbh += dhraw # dbh表示传递 到h层的误差
dWxh += np.dot(dhraw, xs[t].T) # 对Wxh的修正,同Why
dWhh += np.dot(dhraw, hs[t - 1].T) # 对Whh的修正
dhnext = np.dot(Whh.T, dhraw) # h层的误差通过Whh不停地累积
for dparam in [dWxh, dWhh, dWhy, dbh, dby]:
np.clip(dparam, -5, 5, out=dparam) # clip to mitigate exploding gradients
return loss, dWxh, dWhh, dWhy, dbh, dby, hs[len(inputs) - 1]
def sample(h, seed_ix, n):
"""
sample a sequence of integers from the model
h is memory state, seed_ix is seed letter for first time step
"""
x = np.zeros((vocab_size, 1))
x[seed_ix] = 1
ixes = []
for t in range(n):
h = np.tanh(np.dot(Wxh, x) + np.dot(Whh, h) + bh) # 更新中间层
y = np.dot(Why, h) + by # 得到输出
p = np.exp(y) / np.sum(np.exp(y)) # softmax
ix = np.random.choice(range(vocab_size), p=p.ravel()) # 根据softmax得到的结果,按概率产生下一个字符
x = np.zeros((vocab_size, 1)) # 产生下一轮的输入
x[ix] = 1
ixes.append(ix)
return ixes
n, p = 0, 0
mWxh, mWhh, mWhy = np.zeros_like(Wxh), np.zeros_like(Whh), np.zeros_like(Why)
mbh, mby = np.zeros_like(bh), np.zeros_like(by) # memory variables for Adagrad
smooth_loss = -np.log(1.0 / vocab_size) * seq_length # loss at iteration 0
while True:
# prepare inputs (we're sweeping from left to right in steps seq_length long)
if p + seq_length + 1 >= len(data) or n == 0: # 如果 n=0 或者 p过大
hprev = np.zeros((hidden_size, 1)) # reset RNN memory 中间层内容初始化,零初始化
p = 0 # go from start of data # p 重置
inputs = [char_to_ix[ch] for ch in data[p:p + seq_length]] # 一批输入seq_length个字符
targets = [char_to_ix[ch] for ch in data[p + 1:p + seq_length + 1]] # targets是对应的inputs的期望输出。
# sample from the model now and then
if n % 100 == 0: # 每循环100词, sample一次,显示结果
sample_ix = sample(hprev, inputs[0], 200)
txt = ''.join(ix_to_char[ix] for ix in sample_ix)
print('----\n %s \n----' % (txt,))
# forward seq_length characters through the net and fetch gradient
loss, dWxh, dWhh, dWhy, dbh, dby, hprev = lossFun(inputs, targets, hprev)
smooth_loss = smooth_loss * 0.999 + loss * 0.001 # 将原有的Loss与新loss结合起来
if n % 100 == 0: print('iter %d, loss: %f' % (n, smooth_loss)) # print progress
# perform parameter update with Adagrad
for param, dparam, mem in zip([Wxh, Whh, Why, bh, by],
[dWxh, dWhh, dWhy, dbh, dby],
[mWxh, mWhh, mWhy, mbh, mby]):
mem += dparam * dparam # 梯度的累加
param += -learning_rate * dparam / np.sqrt(mem + 1e-8) # adagrad update 随着迭代次数增加,参数的变更量会越来越小
p += seq_length # move data pointer
n += 1 # iteration counter, 循环次数
6. 分析“序列到序列”源代码(选做)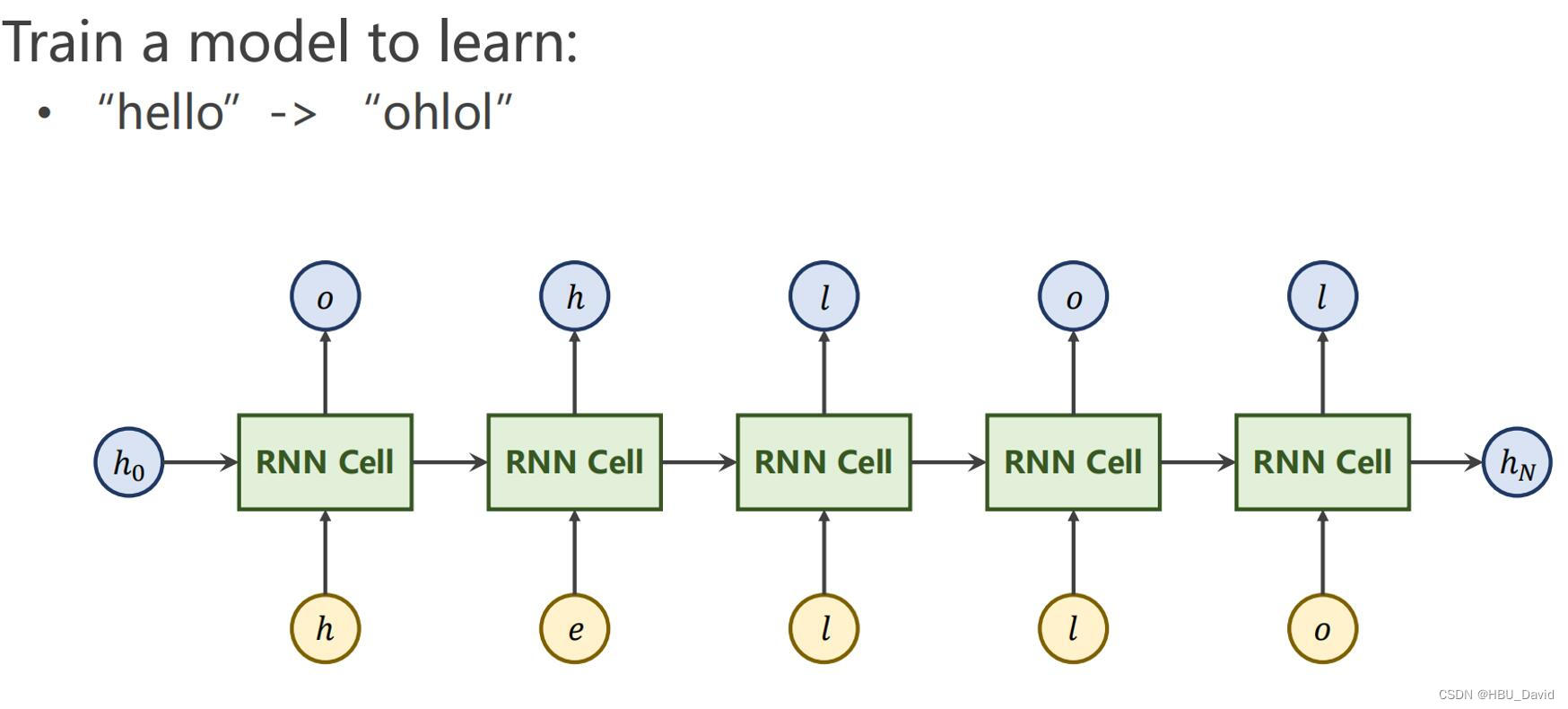
# Model
class Seq2Seq(nn.Module):
def __init__(self):
super(Seq2Seq, self).__init__()
self.encoder = nn.RNN(input_size=n_class, hidden_size=n_hidden, dropout=0.5) # encoder
self.decoder = nn.RNN(input_size=n_class, hidden_size=n_hidden, dropout=0.5) # decoder
self.fc = nn.Linear(n_hidden, n_class)
def forward(self, enc_input, enc_hidden, dec_input):
# enc_input(=input_batch): [batch_size, n_step+1, n_class]
# dec_inpu(=output_batch): [batch_size, n_step+1, n_class]
enc_input = enc_input.transpose(0, 1) # enc_input: [n_step+1, batch_size, n_class]
dec_input = dec_input.transpose(0, 1) # dec_input: [n_step+1, batch_size, n_class]
# h_t : [num_layers(=1) * num_directions(=1), batch_size, n_hidden]
_, h_t = self.encoder(enc_input, enc_hidden)
# outputs : [n_step+1, batch_size, num_directions(=1) * n_hidden(=128)]
outputs, _ = self.decoder(dec_input, h_t)
model = self.fc(outputs) # model : [n_step+1, batch_size, n_class]
return model
model = Seq2Seq().to(device)
criterion = nn.CrossEntropyLoss().to(device)
optimizer = torch.optim.Adam(model.parameters(), lr=0.001)
#下面是训练,由于输出的 pred 是个三维的数据,所以计算 loss 需要每个样本单独计算,因此就有了下面 for 循环的代码
for epoch in range(5000):
for enc_input_batch, dec_input_batch, dec_output_batch in loader:
# make hidden shape [num_layers * num_directions, batch_size, n_hidden]
h_0 = torch.zeros(1, batch_size, n_hidden).to(device)
(enc_input_batch, dec_intput_batch, dec_output_batch) = (enc_input_batch.to(device), dec_input_batch.to(device), dec_output_batch.to(device))
# enc_input_batch : [batch_size, n_step+1, n_class]
# dec_intput_batch : [batch_size, n_step+1, n_class]
# dec_output_batch : [batch_size, n_step+1], not one-hot
pred = model(enc_input_batch, h_0, dec_intput_batch)
# pred : [n_step+1, batch_size, n_class]
pred = pred.transpose(0, 1) # [batch_size, n_step+1(=6), n_class]
loss = 0
for i in range(len(dec_output_batch)):
# pred[i] : [n_step+1, n_class]
# dec_output_batch[i] : [n_step+1]
loss += criterion(pred[i], dec_output_batch[i])
if (epoch + 1) % 1000 == 0:
print('Epoch:', '%04d' % (epoch + 1), 'cost =', '{:.6f}'.format(loss))
optimizer.zero_grad()
loss.backward()
optimizer.step()
7. “编码器-解码器”的简单实现(必做)
# code by Tae Hwan Jung(Jeff Jung) @graykode, modify by wmathor
import torch
import numpy as np
import torch.nn as nn
import torch.utils.data as Data
device = torch.device('cuda' if torch.cuda.is_available() else 'cpu')
# S: Symbol that shows starting of decoding input
# E: Symbol that shows starting of decoding output
# ?: Symbol that will fill in blank sequence if current batch data size is short than n_step
letter = [c for c in 'SE?abcdefghijklmnopqrstuvwxyz']
letter2idx = {n: i for i, n in enumerate(letter)}
seq_data = [['man', 'women'], ['black', 'white'], ['king', 'queen'], ['girl', 'boy'], ['up', 'down'], ['high', 'low']]
# Seq2Seq Parameter
n_step = max([max(len(i), len(j)) for i, j in seq_data]) # max_len(=5)
n_hidden = 128
n_class = len(letter2idx) # classfication problem
batch_size = 3
def make_data(seq_data):
enc_input_all, dec_input_all, dec_output_all = [], [], []
for seq in seq_data:
for i in range(2):
seq[i] = seq[i] + '?' * (n_step - len(seq[i])) # 'man??', 'women'
enc_input = [letter2idx[n] for n in (seq[0] + 'E')] # ['m', 'a', 'n', '?', '?', 'E']
dec_input = [letter2idx[n] for n in ('S' + seq[1])] # ['S', 'w', 'o', 'm', 'e', 'n']
dec_output = [letter2idx[n] for n in (seq[1] + 'E')] # ['w', 'o', 'm', 'e', 'n', 'E']
enc_input_all.append(np.eye(n_class)[enc_input])
dec_input_all.append(np.eye(n_class)[dec_input])
dec_output_all.append(dec_output) # not one-hot
# make tensor
return torch.Tensor(enc_input_all), torch.Tensor(dec_input_all), torch.LongTensor(dec_output_all)
'''
enc_input_all: [6, n_step+1 (because of 'E'), n_class]
dec_input_all: [6, n_step+1 (because of 'S'), n_class]
dec_output_all: [6, n_step+1 (because of 'E')]
'''
enc_input_all, dec_input_all, dec_output_all = make_data(seq_data)
class TranslateDataSet(Data.Dataset):
def __init__(self, enc_input_all, dec_input_all, dec_output_all):
self.enc_input_all = enc_input_all
self.dec_input_all = dec_input_all
self.dec_output_all = dec_output_all
def __len__(self): # return dataset size
return len(self.enc_input_all)
def __getitem__(self, idx):
return self.enc_input_all[idx], self.dec_input_all[idx], self.dec_output_all[idx]
loader = Data.DataLoader(TranslateDataSet(enc_input_all, dec_input_all, dec_output_all), batch_size, True)
# Model
class Seq2Seq(nn.Module):
def __init__(self):
super(Seq2Seq, self).__init__()
self.encoder = nn.RNN(input_size=n_class, hidden_size=n_hidden, dropout=0.5) # encoder
self.decoder = nn.RNN(input_size=n_class, hidden_size=n_hidden, dropout=0.5) # decoder
self.fc = nn.Linear(n_hidden, n_class)
def forward(self, enc_input, enc_hidden, dec_input):
# enc_input(=input_batch): [batch_size, n_step+1, n_class]
# dec_inpu(=output_batch): [batch_size, n_step+1, n_class]
enc_input = enc_input.transpose(0, 1) # enc_input: [n_step+1, batch_size, n_class]
dec_input = dec_input.transpose(0, 1) # dec_input: [n_step+1, batch_size, n_class]
# h_t : [num_layers(=1) * num_directions(=1), batch_size, n_hidden]
_, h_t = self.encoder(enc_input, enc_hidden)
# outputs : [n_step+1, batch_size, num_directions(=1) * n_hidden(=128)]
outputs, _ = self.decoder(dec_input, h_t)
model = self.fc(outputs) # model : [n_step+1, batch_size, n_class]
return model
model = Seq2Seq().to(device)
criterion = nn.CrossEntropyLoss().to(device)
optimizer = torch.optim.Adam(model.parameters(), lr=0.001)
for epoch in range(5000):
for enc_input_batch, dec_input_batch, dec_output_batch in loader:
# make hidden shape [num_layers * num_directions, batch_size, n_hidden]
h_0 = torch.zeros(1, batch_size, n_hidden).to(device)
(enc_input_batch, dec_intput_batch, dec_output_batch) = (enc_input_batch.to(device), dec_input_batch.to(device), dec_output_batch.to(device))
# enc_input_batch : [batch_size, n_step+1, n_class]
# dec_intput_batch : [batch_size, n_step+1, n_class]
# dec_output_batch : [batch_size, n_step+1], not one-hot
pred = model(enc_input_batch, h_0, dec_intput_batch)
# pred : [n_step+1, batch_size, n_class]
pred = pred.transpose(0, 1) # [batch_size, n_step+1(=6), n_class]
loss = 0
for i in range(len(dec_output_batch)):
# pred[i] : [n_step+1, n_class]
# dec_output_batch[i] : [n_step+1]
loss += criterion(pred[i], dec_output_batch[i])
if (epoch + 1) % 1000 == 0:
print('Epoch:', '%04d' % (epoch + 1), 'cost =', '{:.6f}'.format(loss))
optimizer.zero_grad()
loss.backward()
optimizer.step()
# Test
def translate(word):
enc_input, dec_input, _ = make_data([[word, '?' * n_step]])
enc_input, dec_input = enc_input.to(device), dec_input.to(device)
# make hidden shape [num_layers * num_directions, batch_size, n_hidden]
hidden = torch.zeros(1, 1, n_hidden).to(device)
output = model(enc_input, hidden, dec_input)
# output : [n_step+1, batch_size, n_class]
predict = output.data.max(2, keepdim=True)[1] # select n_class dimension
decoded = [letter[i] for i in predict]
translated = ''.join(decoded[:decoded.index('E')])
return translated.replace('?', '')
print('test')
print('man ->', translate('man'))
print('mans ->', translate('mans'))
print('king ->', translate('king'))
print('black ->', translate('black'))
print('up ->', translate('up'))
得到以下结果:
Epoch: 1000 cost = 0.002094
Epoch: 1000 cost = 0.002035
Epoch: 2000 cost = 0.000448
Epoch: 2000 cost = 0.000436
Epoch: 3000 cost = 0.000135
Epoch: 3000 cost = 0.000137
Epoch: 4000 cost = 0.000046
Epoch: 4000 cost = 0.000046
Epoch: 5000 cost = 0.000016
Epoch: 5000 cost = 0.000016
test
man -> women
mans -> women
king -> queen
black -> white
up -> down
在卷积神经网络中,图片先经过卷积层,然后再经过线性层,最终输出分类结果。其中卷积层用于特征提取,而线性层用于结果预测。从另一个角度来看,可以把特征提取看成一个编码器,将原始的图片编码成有利于机器学习的中间表达形式,而解码器就是把中间表示转换成另一种表达形式。
总结
在前馈神经网络中,信息的传递是单向的,因此前馈神经网络难以处理时序序列这种会依赖于过去一段时间输出的的任务,如视频、文本、语音等。而且前馈神经网络的输入尺寸是固定的,但时序序列是不固定的,此时循环神经网络应运而生。
循环神经网络是一类具有短期记忆能力的神经网络.在循环神经网络中,神经元不但可以接受其他神经元的信息,也 可以接受自身的信息,形成具有环路的网络结构。
从应用方面上来看,CNN用到做图像识别比较多,而RNN在做到语言处理多一点,如果拿来比喻的话,CNN如同眼睛一样,正是目前机器用来识别对象的图像处理器。相应地,RNN则是用于解析语言模式的数学引擎,就像耳朵和嘴巴。
参考链接: