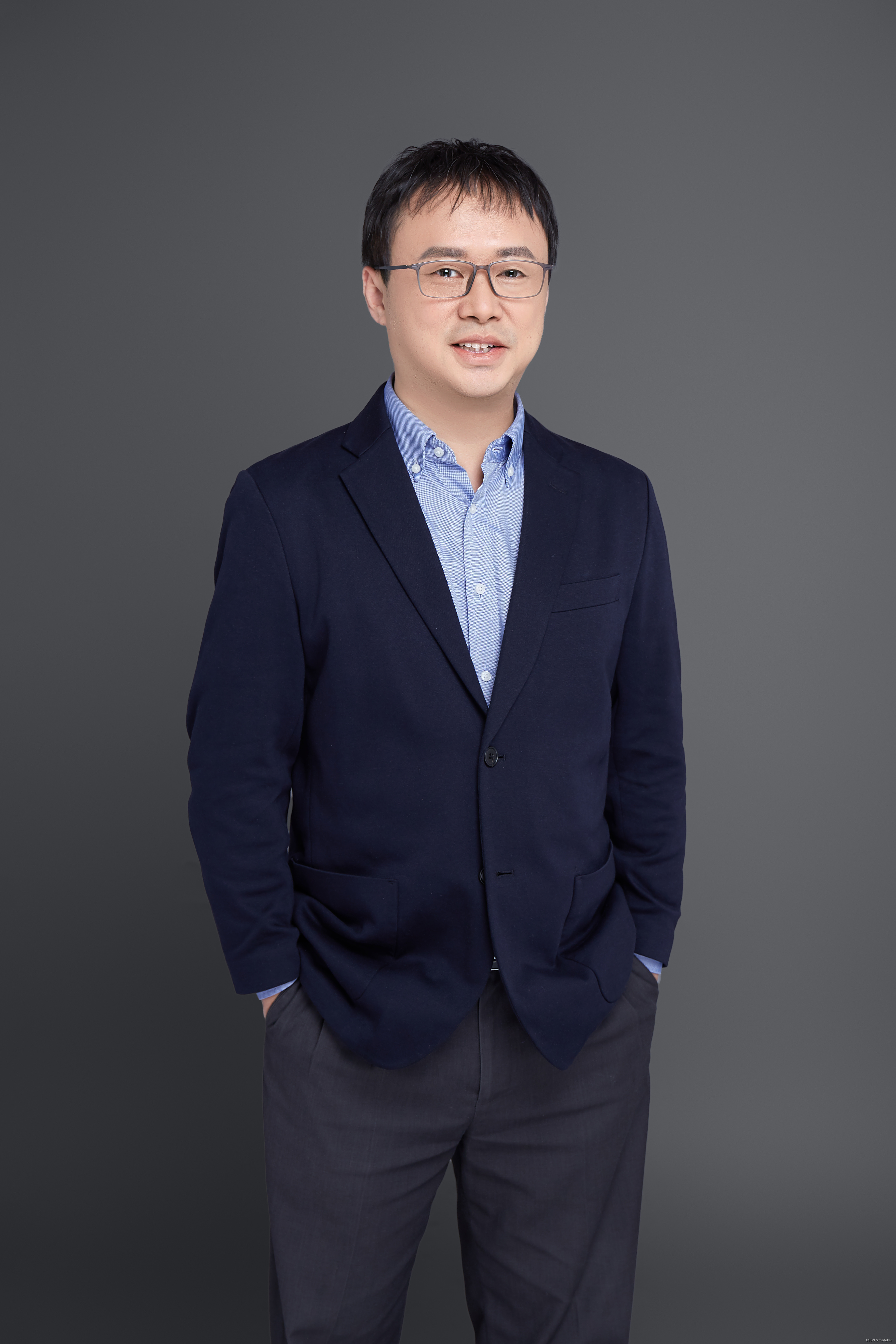
Q: How do you understand AIGA (Generative Automation)?
Li Zhe: The soil in which AIGA and AIGC grow and the nutrients they get are not equal. AIGC can get a lot of nutrition from the public domain in the Internet environment to feed its model and results. And AIGA in the B2B and B2C two major business areas, at least in the B2B business area, the growth soil is somewhat poor. AIGA is based on GPT or other models, which requires a lot of data to train. In fact, the model is fixed. The most important part is pre-trained, which requires a large amount of data for the model to practice. In the field of B2B business, a large amount of data is actually in the hands of B2B enterprises themselves, which is private, not open, and cannot be invested in training. From the perspective of productivity and production relations, this means of production is equivalent to data, which is mastered by various enterprises but not released, and producers cannot deal with it. The large model is not trained, and the results are not close to the actual application scenarios, so it can not meet the needs of users.
Q: Would it be possible for companies to contribute their own data for training?
If each enterprise trains itself, it faces another problem, many enterprises do not have enough data, far from reaching the magnitude of big data, which is not enough for training. I once worked with an AI expert and obtained a lot of public data such as business statistics and tried to train. I provide two sets of data. One set of data has been validated and identified as MQL and SQL for the training of the model. The other is a large batch of unlabeled data that is used to validate the model. Results The data distribution of validation group was very uniform, and the accuracy of anchoring sales leads was not ideal. Experts suggest that the result is a lack of data dimension and volume. We were talking about collaborative models, and from my point of view, a lot of dimensions and data had been released; Other B2B companies may not be so open. However, it is not feasible to use general algorithms to score sales leads. It's really a matter of not having enough data ready.
If we learn from the B2C industry, the data needs to reach the 100 million level to produce valuable results. The data dimension is also important. Different dimensions, especially business people's perception of the industry, can be presented digitally, which is also a particularly challenging work. These things actually need to be trained by the model.
Even in many of the organizations I've talked to, the digitalization is not so thorough, and many business-level knowhow is not preserved in a digital way. In such cases, AI may not be able to play a large enough role. Of course, the direction of AIGA is very attractive, but compared to AIGC, there are challenges. How to share data among enterprises, train and feed together is a topic that needs to be discussed.
Q: In the application of marketing scenarios, what revolutionary changes will AI marketing have compared with traditional marketing methods? What disruption will it bring to marketing techniques and tools?
Li Zhe: We do MA now based on human cognition. Domestic enterprises, whether foreign-funded or private enterprises, are using the traditional idea of the leader from top to bottom management, the vision and cognition of the leader determines the layout of the business, of course, the leader can decide the rules and strategies of MA according to the experience of a small number of subordinates. Most of the time, MA planning is rule-based, that is, rule-based mode. From the perspective of algorithm, it is not a rule-based but data-driven model, which constantly uses previous data to optimize iteration.
Of course, we say that the leader is also based on the previous report and other data analysis, but after all, the processing speed is slow compared to the machine, even Excel speed is limited. However, artificial intelligence can complete the calculation of massive data instantly, and the efficiency will become very high.
Of course, the difficulty of AIGA is also greater, at the beginning we promoted MA to drive traditional marketing, just let marketers accept and learn this technology, and when MA develops to AIGA, the supply of "raw materials" is very important, there must be enough means of production to make productivity burst out, otherwise there is no oil engine, the car can not move forward in any case. Therefore, AIGA is necessarily the direction of MA, provided that the data dilemma is solved.