Human intelligence can retrieve any person according to
both visual and language descriptions. However, the current computer vision community studies specific person reidentification (ReID) tasks in different scenarios separately,
which limits the applications in the real world. This paper
strives to resolve this problem by proposing a new instructReID task that requires the model to retrieve images according to the given image or language instructions. Our
instruct-ReID is a more general ReID setting, where existing 6 ReID tasks can be viewed as special cases by designing different instructions. We propose a large-scale OmniReID benchmark and an adaptive triplet loss as a baseline method to facilitate research in this new setting. Experimental results show that the proposed multi-purpose ReID
model, trained on our OmniReID benchmark without finetuning, can improve +0.5%, +0.6%, +7.7% mAP on Market1501, MSMT17, CUHK03 for traditional ReID, +6.4%,
+7.1%, +11.2% mAP on PRCC, VC-Clothes, LTCC for
clothes-changing ReID, +11.7% mAP on COCAS+ real2
for clothes template based clothes-changing ReID when using only RGB images, +24.9% mAP on COCAS+ real2
for our newly defined language-instructed ReID, +4.3% on
LLCM for visible-infrared ReID, +2.6% on CUHK-PEDES
for text-to-image ReID. The datasets, the model, and code
will be available at https://github.com/hwz-zju/InstructReID.
人工智能可以根据视觉和语言描述检索任何人. 然而,当前计算机视觉界分别研究不同场景下的特定人员重新识别(ReID)任务,
这限制了人员重识别在现实世界中的应用。 这篇论文努力通过提出一个新的instructReID任务来解决这个问题,该任务要求模型根据给定的图像或语言指令检索图像。 我们的
instruct-ReID 是一种更通用的 ReID 设置,通过设计不同的指令,可以将现有的 6 个 ReID 任务视为特殊情况。 我们提出了大规模 OmniReID 基准和自适应三元组损失作为基线方法,以促进这一新环境中的研究。 实验结果表明,所提出的多用途 ReID
模型在我们的 OmniReID 基准上进行训练,无需进行微调,可以将传统 ReID 在 Market1501、MSMT17、CUHK03 上的 mAP 提高 +0.5%、+0.6%、+7.7%、+6.4%、
PRCC、VC-Clothes、LTCC 的 mAP +7.1%、+11.2%
换衣服 ReID,COCAS+ real2 上的 mAP +11.7%
对于基于衣服模板的换衣 ReID,仅使用 RGB 图像时,COCAS+ real2 上的 mAP +24.9%
对于我们新定义的语言指导的 ReID,+4.3%
用于可见红外 ReID 的 LLCM,CUHK-PEDES +2.6%
用于文本到图像的 ReID。 数据集、模型和代码
将在 https://github.com/hwz-zju/InstructReID 上提供。
论文-REid
最新推荐文章于 2024-07-25 12:21:29 发布
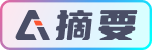