ai基因智能防御
The high frequency of successful large-scale cyber attacks points to gaps existing in conventional cybersecurity. Though attacks are often blamed on mistakes stemming from human factors, the problems of the current cyber situation go deeper. In this article, I argue that the limitations of the conventional defense lie in its simplistic and generic approach, which enables attackers to bypass them with ease and re-use the same attack strategy on multiple victims. I show how the adoption of Artificial Intelligence can change this scene, by personalizing the defence to the defender, forcing attackers to a different and harder situation.
成功进行大规模网络攻击的频率高,表明了常规网络安全中存在的漏洞。 尽管通常将攻击归咎于人为因素造成的错误,但当前网络形势的问题更加严重。 在本文中,我认为常规防御的局限性在于其简单且通用的方法,该方法使攻击者可以轻松绕开它们,并在多个受害者身上重用相同的攻击策略。 我展示了人工智能的采用如何通过使防御者个性化防御,将攻击者逼迫到另一个更困难的境地来改变这一局面。
The ever increasing spread of technology into almost all sectors of industry, as well as every aspect of our daily lives, has increased the complexity of our cyber footprint. This phenomenon has brought with it a new challenge to confront: to ensure our safety from cybercrimes. Cyber theft is the fastest-growing category of crime. Nation-state backed cybercriminals are expanding their targets to not only government institutions, but also businesses and industrial facilities.
技术在几乎所有行业以及我们日常生活的各个方面的不断增长,都增加了我们网络足迹的复杂性。 这种现象带来了新的挑战:确保我们免受网络犯罪的危害。 网络盗窃是犯罪增长最快的类别。 由民族国家支持的网络犯罪分子不仅将目标扩大到政府机构,而且还将其范围扩大到企业和工业设施。
Cybersecurity is now a determining factor in the success of organizations. Their reputation, return on investment (ROI), and customer satisfaction rates depend on it. In the given circumstances, detecting cyber threats and responding to them on time is a key performance indicator of any realistic cyber defense strategy.
网络安全现在是组织成功的决定因素。 他们的声誉,投资回报率(ROI)和客户满意度取决于此。 在给定的情况下,检测网络威胁并及时做出响应是任何现实的网络防御策略的关键绩效指标。
There is now a wave of discussions in the cyber defense community regarding the insufficiencies of the signature-based approach, and how attackers have overcome this defense strategy with increased creativity in their techniques. These discussions are usually accompanied by the proposal of the use of AI technologies to upgrade the capabilities of the signature-based approach and provide a proactive instead of a reactive defense.
关于基于签名的方法的不足,以及攻击者如何通过提高自己的技术创造力来克服这种防御策略的问题,网络防御界现在展开了一波讨论。 这些讨论通常伴随着使用AI技术的建议,以升级基于签名的方法的功能并提供主动防御而不是被动防御。
In this short essay, I am expanding on the contrast between the conventional signature-based or a rule-based defense and the novel AI-based approach. To do so, I am approaching the problem of cyber defense, specifically, of cyber threat detection from within the framework of anomaly detection. The framework of anomaly detection is a canvas on which the distinction between the conventional and AI-based approaches can be drawn with clarity.
在这篇简短的文章中,我将扩展传统的基于签名或基于规则的防御与新颖的基于AI的防御方法之间的对比。 为此,我正在从异常检测的框架内处理网络防御问题,尤其是网络威胁检测。 异常检测的框架是一个画布,在其上可以清晰地描绘出传统方法与基于AI的方法之间的区别。
异常检测 (Anomaly Detection)
An anomaly is something that deviates from what is standard, normal, or expected. Standards are defined pragmatically, with respect to the end-goal or the motivation. For example, an e-commerce company might have different expectations for the behavior of their IT systems than a government organization would have. Anomaly detection is the practice of imposing one’s expectations onto the observations and categorizing them into normal versus abnormal (Figures 1).
异常是偏离标准,正常或预期的东西。 关于最终目标或动机,务实地定义标准。 例如,一个电子商务公司可能对他们的IT系统行为的期望与政府组织不同。 异常检测是一种将期望值强加到观察值上并将其分类为正常值和异常值的实践(图1)。

Pragmatic reasons impose a hierarchy of importance on assets, where importance is determined by the impact on the achievement of the end-goals. It is where a threat is differentiated from an anomaly. The higher the affected asset in the hierarchy of importance, the more likely an anomaly is to end up being a threat (Figure 2). Although it is easy to quantify how much surprise a given event arises through its anomaly score, it is not always straightforward to determine the position of the affected asset in the importance hierarchy, especially in a complex and deeply intermingled IT infrastructure. For instance, an account depending on its complex relationship with other accounts, assets, and processes could be crucial, but go unnoticed until a successful attack is launched from that seemingly innocent starting point.
务实的原因对资产施加了重要性等级,其中重要性取决于对实现最终目标的影响。 在这里,可以将威胁与异常区分开来。 重要等级中受影响的资产越高,异常最终成为威胁的可能性就越大(图2)。 尽管很容易通过异常得分量化给定事件产生多少惊喜,但要确定受影响资产在重要性层次结构中的位置并不总是那么容易,尤其是在复杂且深度混杂的IT基础架构中。 例如,一个取决于其与其他帐户,资产和流程的复杂关系的帐户可能至关重要,但是直到从那个看似无害的起点发起成功的攻击之前,该帐户才被注意到。
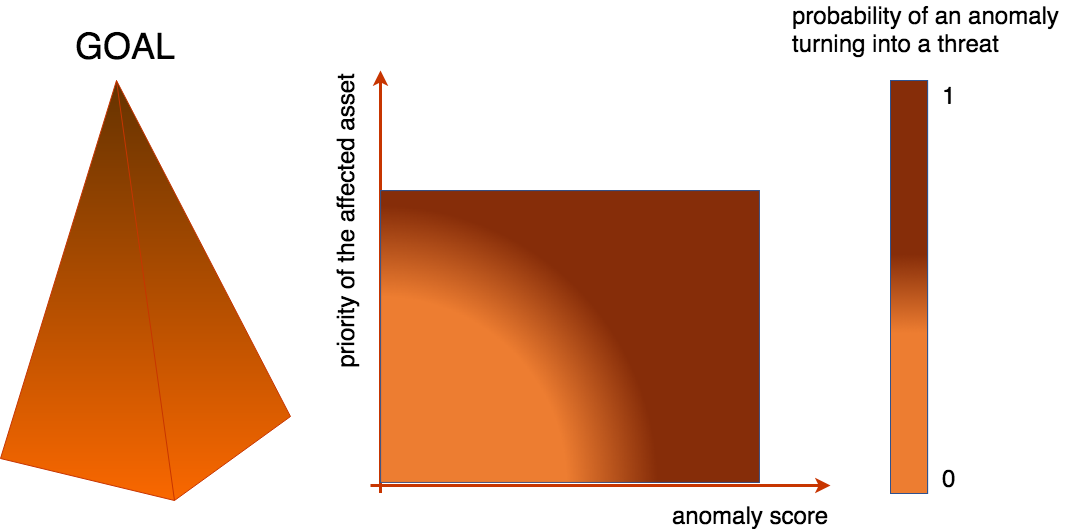
Goals come in layers. In a given organization, let’s say a bank, the highest level goals are the business goals. The business goals require IT goals. The IT goals, in turn, require IT security goals. IT security carries the burden of the previous two layers. IT security goals give rise to standards and norms, whether explicit or implicit, that define the anomaly detection map. Therefore, every IT security team, generally speaking, establishes norms with respect to the IT security goals (cascaded down from the high-level goals), collects the relevant pieces of information (ex. audit logs), detects anomalies, quantifies their severity level (impact to the goals) and responds to them on time with minimal side effects.
目标是分层的。 在给定的组织中,例如一家银行,最高级别的目标是业务目标。 业务目标需要IT目标。 反过来,IT目标需要IT安全目标。 IT安全承担着前两层的负担。 IT安全目标产生了定义异常检测图的标准和规范(无论是显式的还是隐式的)。 因此,一般而言,每个IT安全团队都会建立有关IT安全目标的规范(从高层目标中降级),收集相关信息(例如审核日志),检测异常情况,量化其严重性级别(对目标有影响),并能以最小的副作用及时做出React。
常规防御 (Conventional defense)
The classic approach to IT security is to establish norms in regards to goals beforehand and monitor systems to ensure these norms are obeyed. The expectations take the form of specific rules, policies to be obeyed (e.g. firewall, proxy laws, thresholds on failed login attempts, etc.), and signatures of malicious files in the wild. Rules, policies, and signatures are subject to change in the light of a new vulnerability exposure, or a new cyberattack campaign. In the majority of cases, an event that breaks the policy, rule, or signature can be automatically blocked. If not blocked, then an alarm with severity level given apriori to the broken policy or the affected asset is generated and taken to the attention of the security officer.
IT安全性的经典方法是事先建立有关目标的规范,并监视系统以确保遵守这些规范。 期望采取特定规则,要遵循的策略(例如,防火墙,代理法律,失败的登录尝试阈值等)以及恶意文件签名的形式。 规则,策略和签名可能会根据新的漏洞披露或新的网络攻击活动而更改。 在大多数情况下,可以自动阻止违反策略,规则或签名的事件。 如果未阻止,则会生成具有严重级别的警报,警报的严重程度将优先于已损坏的策略或受影响的资产,并引起安全人员的注意。
The approach above is an explicit formulation of norms. Here, explicit means that all the expectations are written down somewhere and are expressed precisely and shortly (e.g. block if more than 5 failed attempts have been made to log in). However, there are always unwritten rules, not written down by anyone, operating behind the scenes that govern all sorts of processes. These rules are implicit, and it is impossible to define them apriori since they emerge as things happen. For example, society operates based on explicitly defined rules (traffic rules, law system, human rights, and other similar protocols) and as well as implicitly defined rules (moral codes, human instinct, and traditions passed down from generations before that are encoded in action only).
上面的方法是对规范的明确表述。 在这里,显式表示将所有期望记录在某个地方,并准确而简短地表达出来(例如,如果尝试登录失败超过5次,则阻止该期望)。 但是,总是存在着不成文的规则,任何人都没有写下来,而是在幕后操纵着各种过程。 这些规则是隐式的,并且不可能先定义它们,因为它们会随着事情的发生而出现。 例如,社会基于明确定义的规则(交通规则,法律制度,人权和其他类似协议)以及隐含定义的规则(道德规范,人类的本能和传承于世世代代的传统)进行运作仅动作)。
Explicit rules are easy to change and define. Ease of modification is a crucial factor in keeping up to date with the highly dynamic nature of the modern cyber landscape. Drawn from the shared cybersecurity knowledge pool, these rules tend to be generic, meaning they are not unique to the organization in question. The collective nature of conventional defense across organizations can facilitate universal security standards and protocols.
显式规则易于更改和定义。 易于修改是与时俱进的关键因素,以适应现代网络环境的高度动态性。 从共享的网络安全知识库中提取的这些规则通常是通用的,这意味着它们并非所讨论的组织所独有。 跨组织的常规防御的集体性质可以促进通用安全标准和协议。
Explicitly defined rules, taking into account a limited number of factors at a time, cannot capture the full complexity of a cyber event. In other words, they treat cyber events atomically, oblivious to their inter-relationships. This results in a high rate of false alarms. An example policy of ‘block if the transaction occurs outside the country of residence’ prevents some fraudulent transactions. However, it comes at the cost of client dissatisfaction when their cards are blocked during their trips. A credit card transaction event has other nuances to it. Answers to “How often the client travels?”, “How much time has passed since the last transaction in the country of residence?”, “At which merchant is the transaction happening?” and other similar questions could have led to a smarter decision, therefore reducing the false alarm rate. Adding these nuances, however, takes us to the domain of unwritten rules where we lose the advantages of explicitness and easy maintenance. What is more, discovering such relevant nuances is a hard task on its own.
明确定义的规则,一次考虑到有限的因素, 不能捕获网络事件的全部复杂性。 换句话说,他们原子地对待网络事件,而忽略了它们之间的相互关系。 这导致较高的误报率。 “如果交易发生在居住国之外则阻止”的示例策略可防止某些欺诈性交易。 但是,如果在旅行途中卡被遮挡,则会以客户不满意为代价。 信用卡交易事件还有其他细微差别。 回答“客户旅行的频率如何?”,“自上次居住国上次交易以来经过了多少时间?”,“交易发生在哪个商人?” 和其他类似问题可能会导致做出更明智的决策,从而降低了误报率。 但是,添加这些细微差别会使我们进入不成文规则的领域,在这里我们失去了明确性和易于维护的优势。 而且,发现这样的细微差别本身就是一项艰巨的任务。
To go under the radar, one needs to know about the radar. The radars, in the form of signatures, rules, and policies, as a result of being explicitly defined, are not complex. The attacker, therefore, does not have much to learn and he can bypass them with minimal effort. What is more, the barriers in one place are quite close to barriers in another place, therefore allowing attackers to re-use, and even sell their techniques to be used against different victims.
要进入雷达之下,需要了解雷达。 由于被明确定义,以签名,规则和策略形式出现的雷达并不复杂。 因此,攻击者无需学习太多知识,并且可以轻松地绕过它们。 而且,一个地方的障碍与另一地方的障碍非常接近,因此攻击者可以重复使用,甚至出售其技术以对付不同的受害者。
新型防御 (Novel Defense)
The rule-based approach treats cyber events atomically, ignoring the context within which they exist. The collective detection logic lets attackers hit multiple birds with a single stone. The remedy to the conventional defense is to contextualize conventional rules so that they are more nuanced. This contextualization can be done by extending the reach of collective rules to unwritten rules specific to the organizations, which can lead to unique, personalized defense logic to each place, creating a web as in Figure 3.
基于规则的方法以原子方式对待网络事件,而忽略了它们存在的上下文。 集体检测逻辑使攻击者可以用一块石头击中多只鸟。 常规防御的补救措施是将常规规则置于上下文中,以使它们更具细微差别。 可以通过将集体规则的范围扩展到组织特定的未成文规则来实现这种上下文关系,这可以导致每个地方都有独特的个性化防御逻辑,如图3所示。
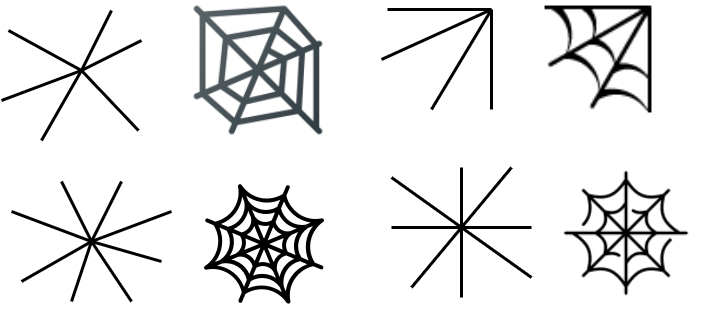
Unwritten rules are not defined apriori, as in the conventional case. They manifest themselves in experience only, or in our cybersecurity context, in the events themselves. The unwritten rules are holistic, meaning they inter-twine multiple factors spread both in time (patterns emerging in a given duration, for instance in the form of a seasonality), and space (patterns emerging across events that are coincidental with one another, similar in the credit card transaction case described above). To extract unwritten rules one needs to analyze event logs. Since such an analysis is an immense undertaking to be done manually, it needs to be automated.
像常规情况一样,未事先定义未成文的规则。 他们只是在事件本身或我们的网络安全环境中表现自己。 不成文的规则是整体的,这意味着它们将多个因素(时间上出现的模式(在给定的持续时间内出现,例如以季节性形式出现))和空间(时间上相互一致的模式出现)之间相互缠绕在上述信用卡交易中)。 要提取不成文的规则,需要分析事件日志。 由于这种分析是手动完成的一项艰巨任务,因此需要自动化。
The recent success of AI algorithms, especially in image and audio tasks, is due to their ability to extract patterns from the empirical training data and use the extracted patterns as a basis to classify or predict the test data. The same technique can be applied to extract unwritten rules from cyber event logs. These unwritten rules then can be used to enrich the conventional rules, policies, and signatures and build defense barriers that are unique to the organization.
AI算法最近的成功,尤其是在图像和音频任务中,是由于它们具有从经验训练数据中提取模式并将提取的模式用作分类或预测测试数据的基础的能力。 可以应用相同的技术从网络事件日志中提取未编写的规则。 然后,这些不成文的规则可以用来丰富常规规则,策略和签名,并为组织建立独特的防御屏障。
An AI learning algorithm is not a blank slate that one can throw onto data hoping it will learn useful patterns in it; we do not have such general artificial intelligence yet. An algorithm has a built-in logic imputed by the programmer. No knowledge is possible without bias. To know something starts with the observing of a thing, and observation requires a tool. The tool has a particular structure that processes the incoming raw information in accordance with that structure, adding the first layer of bias. An additional bias is introduced in the interpretation of the observation. To put it in another way, every observation presupposes some kind of an expectation, a hypothesis or a guess, even the change in one’s hypothesis requires an initial hypothesis to start from. A bias introduced into an AI algorithm is of two kinds: hard and soft. The hard bias does not alter in an encounter with data, while the soft one is flexible and re-configures itself in response to data. The soft bias is what does the actual learning. The hard bias is more of an interpretative filter that feeds the soft bias with the interpreted data. The development of a functional AI-based threat detection tool is an iterative process of converging to the most optimal bias (hard and soft) until the success criteria are satisfied.
人工智能学习算法并不是一个空白,它可以让人们希望它能够学习有用的模式,而不会丢给数据。 我们还没有这样的通用人工智能。 算法具有由程序员估算的内置逻辑。 没有偏见就不可能有知识。 要了解事物,首先要观察事物,而观察则需要工具。 该工具具有特定的结构,该结构根据该结构处理传入的原始信息,从而增加了第一层偏差。 在观察结果的解释中引入了额外的偏差。 换句话说,每个观察都以某种期望,假设或猜测为前提,即使一个人的假设发生变化也需要一个初始的假设作为起点。 引入AI算法的偏差有两种:硬性和软性。 硬偏见在遇到数据时不会改变,而软偏见则很灵活,可以根据数据进行重新配置。 软偏差是实际学习的内容。 硬偏差更多是一种解释性过滤器,它将解释的数据提供给软偏差。 功能性基于AI的威胁检测工具的开发是一个迭代过程,逐步收敛到最佳偏差(硬性和软性),直到满足成功标准为止。
In the case of supervised learning, training data comes with labels, and success can be measured using those labels. In an unsupervised setting, however, such labels are not available, and success criteria remain not as formalized. Labeled data sets are not in abundance in the cybersecurity domain, so the majority of AI development here happens in the unsupervised setting. In the context of threat detection, the quality of the trained model is inferred from the quality of its detected anomalies that are assessed by the end-user of the tool, a security specialist.
在监督学习的情况下,培训数据带有标签,并且可以使用这些标签来评估成功。 但是,在无人监督的情况下,此类标签不可用,并且成功标准也没有那么正式。 标记的数据集在网络安全领域并不丰富,因此此处的大多数AI开发都发生在无人监督的环境中。 在威胁检测的上下文中,从该工具的最终用户(安全专家)评估的检测到的异常的质量中推断出训练后模型的质量。
The AI-based approach solves the false alarm problem since rules are more nuanced, and anomalies that would arise in the conventional case are accounted for by referring to the relevant context. The AI-based approach takes away the burden of manual maintenance since an AI algorithm will pick up the changes and reconfigure itself to the new situation automatically. An AI erected radar, analogous to fingerprint-based security systems, is built upon unwritten rules specific and personal to the defender, forcing the attacker to play a harder game. The attacker now needs more detailed in-depth intelligence about the victim, his metaphorical fingerprint, for a successful attack.
基于AI的方法解决了虚假警报问题,因为规则更加细微,并且通过参考相关上下文来解决常规情况下可能出现的异常。 基于AI的方法免除了手动维护的负担,因为AI算法将自动进行更改并重新配置为新情况。 类似于基于指纹的安全系统,竖立的AI雷达建立在防御者特有的,不成文的规则基础上,迫使攻击者进行更艰苦的游戏。 攻击者现在需要有关受害者的更详细的深入情报,即他的隐喻指纹,才能成功进行攻击。
In advanced use cases, one can infer the priority of the assets, users, other entities purely from their behavior patterns. This way, one can identify those seemingly unimportant points in a complex environment and monitor them more closely.
在高级用例中,一个人可以纯粹根据其行为模式来推断资产,用户和其他实体的优先级。 这样,就可以识别复杂环境中那些看似不重要的点,并对其进行更密切的监视。
The Achilles’ heel of using AI is that extracted patterns are usually not expressible enough for humans to understand because AI analyzes and correlates more data points than a human operator can handle (AI black box problem). To understand what an AI tool is trying to convey, the operator must know the environment very well. It is harder to take action on the findings generated by an AI tool than policy-based ones. Contrary to policy-based tools where it is precisely clear what rule is broken when the alarm goes off, with an AI detection tool, the root cause of alarm might be tricky to understand since there is a multitude of data points that contribute to the alarm. These inherent constraints make it crucial that these AI tools are operated by human specialists, who have an in-depth understanding of the organization they are working for.
使用AI的致命弱点是,提取的模式通常无法表达给人类理解,因为AI分析和关联的数据点多于操作员无法处理的( AI黑匣子问题 )。 要了解AI工具试图传达的内容,操作员必须非常了解环境。 与基于策略的结果相比,对人工智能工具产生的结果采取行动更加困难。 与基于策略的工具相反,后者可以精确地确定警报响起时违反了什么规则,而使用AI检测工具,可能很难理解警报的根本原因,因为有许多数据点可构成警报。 这些固有的约束条件使这些AI工具由人类专家操作至关重要,因为他们对所工作的组织有深入的了解。
结论 (Conclusion)
The conventional defense strategy relies on linear reasoning with its explicitly defined, precise rules and policies. Application of AI brings about a non-linear reasoning to defense, by learning holistic, unwritten rules that manifest themselves in action. The adoption of AI is a promising way to cope with the creativity of cyberattacks and detect them in action even when they manage to bypass the conventional layer of defense.
常规防御策略依靠线性推理及其明确定义的精确规则和策略。 通过学习在行动中表现出来的整体,不成文的规则,人工智能的应用为防御带来了非线性推理 。 采用AI是应对网络攻击的创造力并在实际操作中发现它们的一种有前途的方法,即使它们设法绕过常规防御层也是如此。
翻译自: https://medium.com/soter-ai/changing-the-game-of-cyber-defence-with-ai-8c77e3799f39
ai基因智能防御