支持向量机原理
Python数据科学 (Python Data Science)
The support vector machines (SVM) algorithm has applications for regression problems as well as for classification, both linear and non-linear. In addition, both continuous and categorical values may be used in SVM. This time around, we will take a look at SVM for classification.
支持向量机(SVM)算法可用于回归问题以及线性和非线性分类。 另外,连续值和分类值都可以在SVM中使用。 这次,我们将研究SVM的分类。
SVM can be used to predict whether a Beatles song was written by John or Paul, whether or not a news article is fake, or from what region a chili recipe originates. This article will explore:
SVM可用于预测甲壳虫乐队的歌曲是由约翰还是保罗撰写的,新闻文章是否是假的,或者辣椒食谱来自哪个地区。 本文将探讨:
- What is SVM? 什么是SVM?
- How do SVMs work? SVM如何工作?
- How to implement SVM in python. 如何在python中实现SVM。
支持向量机 (Support Vector Machines)

SVM supervised machine learning (ML) models separate classes by creating a hyperplane in multi-dimensional space. Key to the idea of SVM is finding a hyperplane (shown as a bold-type line, in the figure 1 illustration) that maximizes the margin between classes or between each class and the hyperplane that divides them.
SVM监督的机器学习(ML)通过在多维空间中创建超平面来对单独的类进行建模。 支持向量机的思想的关键是找到一个超平面(在图1中显示为粗体线),该平面可使类之间或每个类与划分它们的超平面之间的余量最大化。
支持向量 (Support vectors)
The points which are closest to the hyperplane are the support vectors. These points will make the decision boundary better by calculating the margins. These support vectors are the points most critical for the iterative calculations required to construct the classifier.
最靠近超平面的点是支持向量。 这些点将通过计算边距使决策边界更好。 这些支持向量是构造分类器所需的迭代计算最关键的点。
超飞机 (Hyperplane)
A decision plane which separates the classes. This plane will optimally be equidistant from the support vectors on either side.
分隔各个类的决策平面。 该平面最好与任一侧的支持向量等距。
保证金 (Margin)
A gap between the two lines supported by the closest distinct-class vectors (data points) is calculated as the perpendicular distance between the lines.
计算由最接近的独特类向量(数据点)支持的两条线之间的间隙作为线之间的垂直距离。
SVM的工作原理 (Workings of SVM)
The main objective of SVM is to divide the given data in the best possible way into different classes. The distance between the nearest data points of each class is known as the margin. The goal of SVM is to select a hyperplane with the maximum possible margin between support vectors in the given data. It does it in following ways:
SVM的主要目标是以最佳方式将给定数据划分为不同的类。 每个类别的最近数据点之间的距离称为边距。 SVM的目标是在给定数据中选择在支持向量之间具有最大可能余量的超平面。 它通过以下方式进行:
- Creates multiple decision boundaries that divide data into classes in the best way. 创建多个决策边界,以最佳方式将数据分为几类。
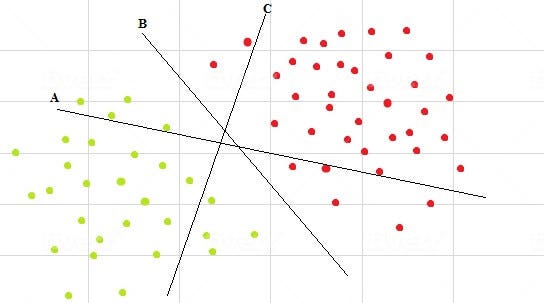
Now, there are multiple decision boundaries (figure 2) but which to select? Intuitively, if we select a hyperplane which is close to the data points of one class, then it might not generalize well. So the goal is to choose the hyperplane which is as far as possible from the data points of each category.
现在,存在多个决策边界(图2),但是要选择哪个呢? 直观地,如果我们选择一个超平面,该超平面接近一类的数据点,那么它可能无法很好地概括。 因此,目标是选择距每个类别的数据点尽可能远的超平面。
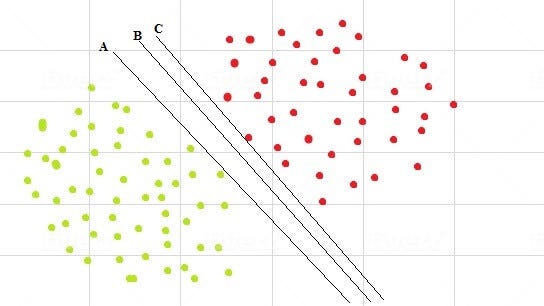
- Maximizing the distance between the classes and the hyperplane would result in an optimal separating hyperplane (indicated by line B, in figure 3). The goal of SVMs is to find the optimal hyperplane, because it not only classifies the existing dataset but also helps predict the class of unseen data. 使类与超平面之间的距离最大化将导致最佳的分离超平面(图3中的线B所示)。 SVM的目标是找到最佳的超平面,因为它不仅对现有数据集进行分类,而且还有助于预测看不见的数据的类别。
注意:SVM内核 (Note: SVM kernels)
We suggested earlier that SVM may be used for non-linearly separable classes. To make it work on non-linear data we use something called kernels.
前面我们建议将SVM用于非线性可分离类。 为了使它适用于非线性数据,我们使用了称为内核的东西。
A kernel transforms an input data space into the required space in which data can be linearly separated. There are different types of kernels, including linear, polynomial, and radial basis function (rbf). SVM uses a technique called the kernel trick, by which the kernel takes a low-dimensional input space and transforms it into a higher dimensional space.
内核将输入数据空间转换为所需的空间,在其中可以线性分离数据。 内核有不同类型,包括线性,多项式和径向基函数(rbf)。 SVM使用一种称为内核技巧的技术,内核通过该技术获取低维输入空间并将其转换为高维空间。
使用Python的SVM实现 (SVM Implementation Using Python)
Let’s take a look at how the sklearn library supports SVM modeling. We start by importing the necessary modules.
让我们看一下sklearn库如何支持SVM建模。 我们首先导入必要的模块。
from sklearn import datasets
from sklearn.model_selection import train_test_split
from sklearn import svm
from sklearn.metrics import accuracy_score
数据集 (The Dataset)
We will be working with the breast cancer dataset provided by sklearn.
我们将使用sklearn提供的乳腺癌数据集。
# loading the dataset
cancer = datasets.load_breast_cancer()
Exploring the dataset
探索数据集
# printing the names of the 13 features
print("Features: ", cancer.feature_names)# printing the label type of cancer('malignant' 'benign'), i.e., 2 classes
print("\nLabels: ", cancer.target_names)
The following description is output:
输出以下描述:
Features: ['mean radius' 'mean texture' 'mean perimeter' 'mean area'
'mean smoothness' 'mean compactness' 'mean concavity'
'mean concave points' 'mean symmetry' 'mean fractal dimension'
'radius error' 'texture error' 'perimeter error' 'area error'
'smoothness error' 'compactness error' 'concavity error'
'concave points error' 'symmetry error' 'fractal dimension error'
'worst radius' 'worst texture' 'worst perimeter' 'worst area'
'worst smoothness' 'worst compactness' 'worst concavity'
'worst concave points' 'worst symmetry' 'worst fractal dimension']Labels: ['malignant' 'benign']
The is a binary classification dataset. Let’s view the target data.
是二进制分类数据集。 让我们查看目标数据。
# printing the first 50 labels (0:malignant, 1:benign)
print(cancer.target[:50]
[0 0 0 0 0 0 0 0 0 0 0 0 0 0 0 0 0 0 0 1 1 1 0 0 0 0 0 0 0 0 0 0 0 0 0 0 0 1 0 0 0 0 0 0 0 0 1 0 1 1]}]}]
[0 0 0 0 0 0 0 0 0 0 0 0 0 0 0 0 0 0 1 1 1 0 0 0 0 0 0 0 0 0 0 0 0 0 0 0 0 0 0 0 0 0 0 0 0 0 1 1]}]}]
We will split data into training and testing sets.
我们将数据分为训练和测试集。
# splitting the dataset into training set and test set
X_train, X_test, y_train, y_test = train_test_split(cancer.data, cancer.target, test_size=0.25)
建立模型 (Creating a model)
Let’s start building our SVM model. Sklearn makes it possible to build a classifier and train a model, in only two lines of code.
让我们开始构建我们的SVM模型。 Sklearn使得仅用两行代码即可构建分类器和训练模型。
# creating an SVM Classifier
clf = svm.SVC(kernel='linear') # Linear Kernel# training the model using the training sets
clf.fit(X_train, y_train)
We can predict on test data:
我们可以根据测试数据进行预测:
# predicting the response for test dataset
y_pred = clf.predict(X_test)
性能 (Performance)
Measuring the performance of our model is accomplished by comparing predictions with the data’s original labels.
通过将预测与数据的原始标签进行比较,可以衡量模型的性能。
# reporting model accuracy
print("Accuracy:", round(accuracy_score(y_test, y_pred)*100),'%')Accuracy: 94.0 %
Our model achieved an accuracy of 94 %.
我们的模型达到了94%的精度。
结论 (Conclusion)
In this article, we discussed the SVM algorithm for ML classification. We defined the characteristics of SVM, explained and illustrated how they work for linear models, and coded a linear example in python.
在本文中,我们讨论了用于ML分类的SVM算法。 我们定义了SVM的特性,解释和说明了它们如何用于线性模型,并在python中编写了线性示例。
SVM classification has a number of practical applications, from identifying cancerous cells, to predicting wildfires for particular geographies, to deep learning object segmentation. We focused on simple SVM, to develop a linear model for binary classification.
SVM分类具有许多实际应用,从识别癌细胞到预测特定地区的野火,再到深度学习对象分割。 我们专注于简单的SVM,以开发用于二进制分类的线性模型。
For data that is not linearly separable, kernel SVM may be used to map data onto higher dimensional space. With the kernel trick, a variety of non-linear functions may be tested to determine the optimal hyperplane.
对于不可线性分离的数据,可以使用内核SVM将数据映射到更高维的空间。 利用内核技巧,可以测试各种非线性函数以确定最佳超平面。
Scoring modules available in sklearn may be used to compare models developed with the various functions. Since SVM is a supervised ML algorithm, the ultimate decision for which function is most appropriate will be yours and will depend on your particular use case.
sklearn中可用的评分模块可用于比较使用各种功能开发的模型。 由于SVM是一种监督型ML算法,因此哪个功能最合适的最终决定权取决于您,并且取决于您的特定用例。
翻译自: https://medium.com/the-innovation/support-vector-machines-7398c5fc7160
支持向量机原理