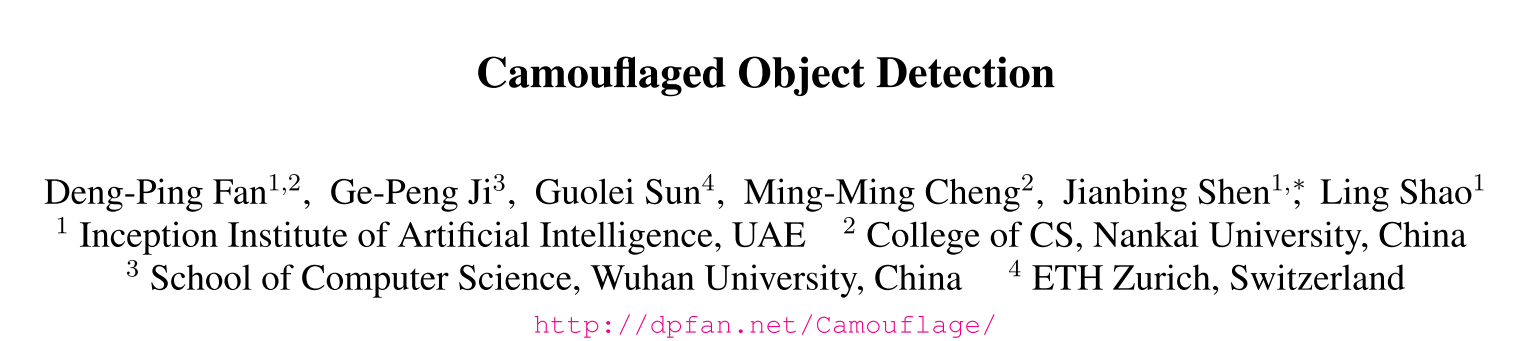
1. Motivation
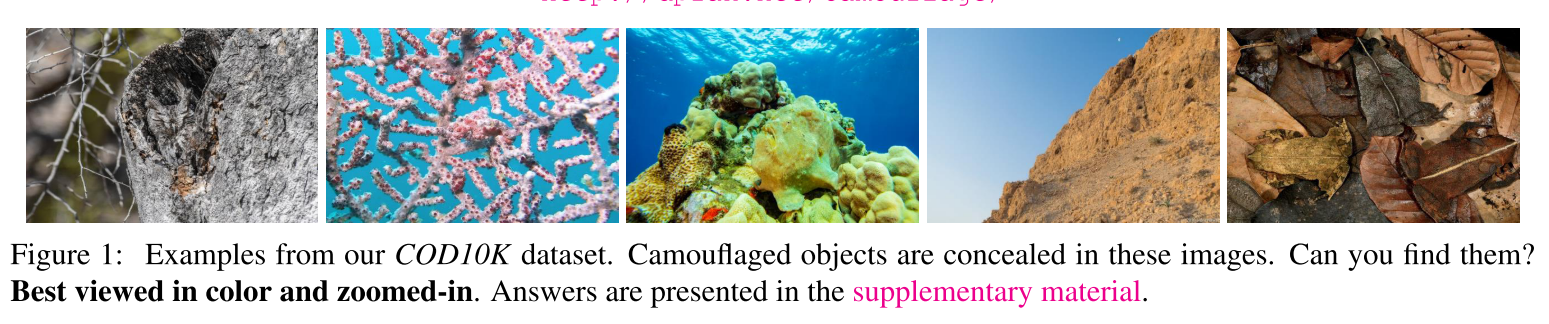
本文研究的领域为COD(Camouflaged object detection),伪装物体检测的定义:
Camouflaged object detection(COD) aims to identify objects that are ‘seamlessly’ embedded in their surroundings.
由于在COD中的target和background具有intrinsic similarities,因此相对于传统的目标检测来说,COD更具有挑战性。
The high intrinsic similarities between the target object and the background make COD far more challenging than the traditional object detection task.
COD的适用性:
COD is also beneficial for applications in the fields of computer vision , medical image segmentation , agriculture and art.
COD领域数据集缺乏。
Currently, camouflaged object detection is not well studied due to the lack of a sufficiently large dataset.
2. Contribution
-
本文提出了COD10K 数据集。
To address this issue, we elaborately collect a novel dataset, called COD10K, which comprises 10,000 images covering camouflaged objects in various natural scenes, over 78 object categories.
-
本文构建了用于COD的Search Identification Network (SINet)框架。
In addition, we develop a simple but effective framework for COD, termed Search Identifi-cation Network (SINet).
其中COD10K与现有的COD数据集的区别有以下3点:
-
COD10K包含了10K,78个类别,水陆地两栖。
It contains 10K images covering 78 camouflaged object categories, such as aquatic, flying, amphibians, and terrestrial, etc.
-
COD10K的annotation信息,并且可以用于多任务中。
All the camouflaged images are hierarchically annotated with category, bounding-box, object-level, and instance-level labels, facilitating many vision tasks, such as localization, object proposal, semantic edge detection [42], task transfer learning [69], etc.
-
高质量的annotation促进算法的性能
Each camouflaged image is assigned with challenging attributes found in the real-world and matting-level [73] labeling (requiring ∼60 minutes per image). These high-quality annotations could help with providing deeper insight into the performance of algorithms.
图1是COD10K与之前COD任务中的2个数据集的summary的对比。
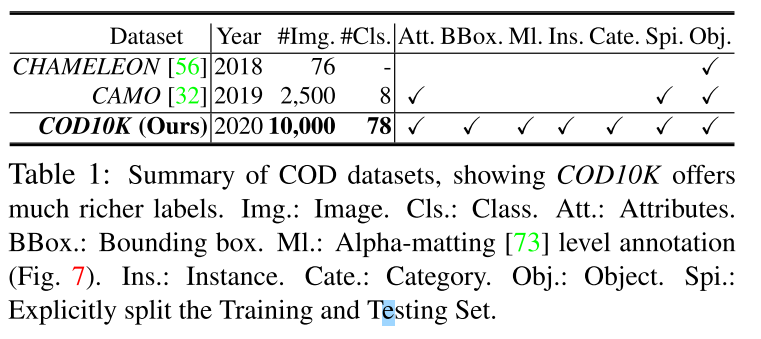
3. Relation Work
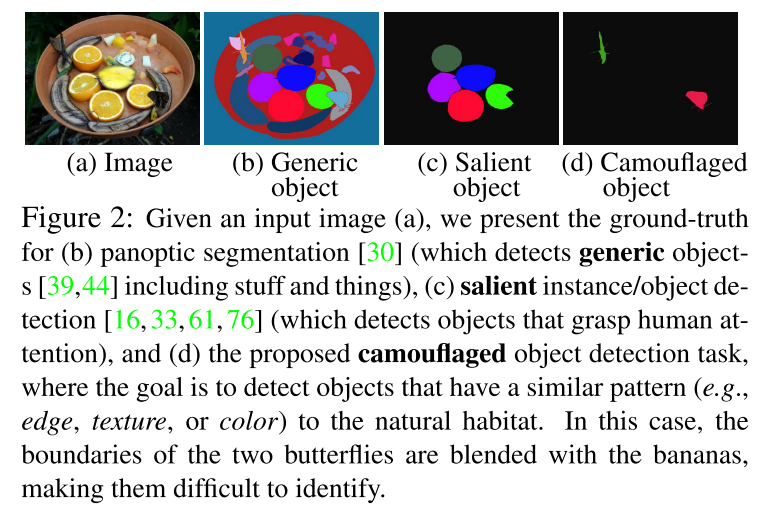
图2,分为了GOD,SOD,以及COD。
3.1 Generic and Salient Object Detection
其中GOD是最流行的视觉研究方向,用于研究语义分割和全景分割。
One of the most popular directions in computer vision is generic object detection.
Typical GOD tasks include semantic segmentation and panoptic segmentation
SOD是一张图片中最引人注意的物体。与salient相反的就是camouflaged。
That is, positive samples (images containing a salient object) can be utilized as the negative samples in a COD dataset.
3.2 Camouflaged Object Detection
3.2.1 Types of Camouflage
camouflaged 图片可以分为2种类型: 自然伪装以及人造伪装。
自然伪装是动物使用的;人造的伪装发生在产品中(所有的defect缺陷),或者在游戏用用于隐藏信息。
Camouflaged images can be roughly split into two types: those containing natural camouflage and those with artificial camouflage
3.2.2 COD Formulation
与class-dependent的语义分割不同,COD是class-independent task。COD formulation:给定一张图片,每个像素都有一个confidence p, p i ∈ [ 0 , 1 ] p_i \in [0, 1] pi∈[0,1],如果pixels的值为0,表示它不属于camouflaged objects,如果是1表明pixel表示camouflagd objects。
Given an image, the task requires a camouflaged object detection approach to assign each pixel i a confidence pi ∈ [0,1], where pi denotes the probability score of pixel i.
A score of 0 is given to pixels that don’t belong to the camouflaged objects, while a score of 1 indicates that a pixel is fully assigned to the camouflaged objects.
3.2.3 Evaluation Metrics.
不适用原本的MAE。
本文使用3个metrics:
- a human visual perception based E-measure ( E ϕ E_{\phi} Eϕ),which simultaneously evaluates the pixel-level matching and image-level statistics
- Since camouflaged objects often contain complex shapes, COD also requires a metric that can judge structural similarity. We utilize the S-measure ( S α S_\alpha Sα) [12] as our alternative metric.
- the weighted F-measure ( F β w F^w_{\beta} Fβw) [43] can provide more reliable evaluation
4. Dataset
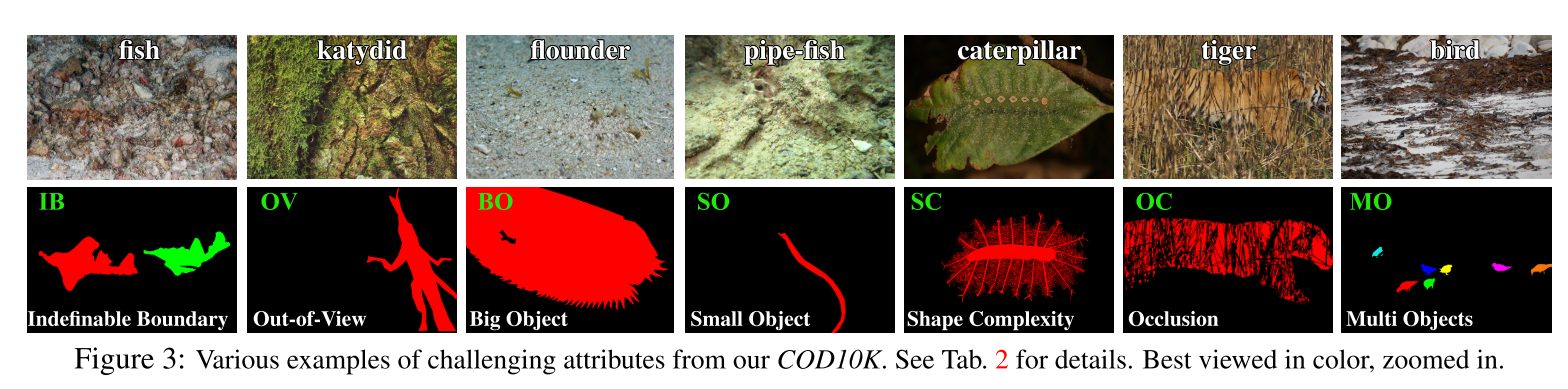
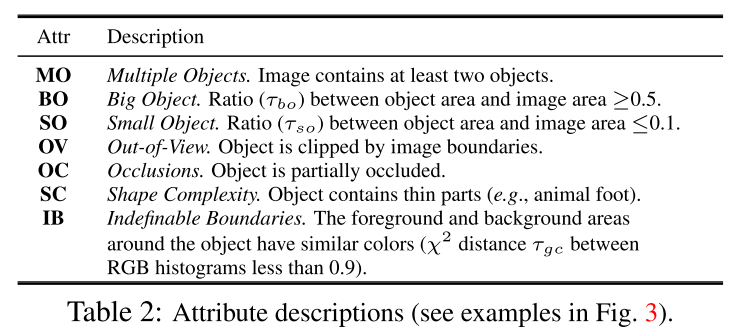
our goals for studying and d