Heart Rate1
1. Traditional HR measurements
- Relay on contact monitors(ECG) -> cause inconvenience and discomfort
- Contact photoplethysmography( cPPG )based sensors
2. Recently, remote HR estimation from face videos, allows HR estimation from the skin. i.e. the face area
2.1 Drawback
- most of them focus on well-controlled scenarios, the generalization ability into less-constrained scenarios are not known
- Lack large-scale HR databases
2.2 Existing video-based HR estimation methods
2.2.1 Remote photoplethysmography( rPPG) based approaches
- Introduction: Aim to extract HR signals from the color changes caused by variations in volume and oxygen saturation of the blood in the vessels due to heart beats
- Principle: Color space transformation and signal decomposition
- Disadvantage: limited observed video framse, the requirement of speed
Because the continuous HR measurement requires not only high accuracy but also fast response, and an estimate should be made given a small number of video frames. Inaddition, rPPG signals can be influenced by face movement and illumination lighting variations
2.2.2 Improved Remote photoplethysmography( rPPG) based approaches 2
- Introduction
- Reliable detection and tracking for region of interest on the face
- Efficient cardiac cycle signal extraction and enhancement
- Ability to handle temporal subtle changes
- How to overcome the issues
- Address these issues from the perspectives of ROI selection, chrominance feature generation, filtering, and heart arte distribution learning.
- New rPPG algorithms (The essential difference is how the combine RGB-signals into a pulse-signal).
- Blind Source seperation
- CHROM, combinesthe chrominance signals
- PBV
- 2SR
2.2.3 Ballistocardiograph(BCG) based approaches.
- Extract HR signals from head movements arising from the periodic ejections of blood into the great vessels along with each heartbeat
Eye Tracking3
1. Traditional methods
- Electro-oculographic techniques, for example, relied on electrodes mounted on the skin around the eye that could measure differences in electric potential so as to detect eye movements.
- Required the wearing of large contact lenses that covered the cornea (the clear membrane covering the front of the eye) and sclera (the white of the eye that is seen from the outside), with a metal coil embedded around the edge of the lens; eye movements were then measured by fluctuations in an electromagnetic field when the metal coil moved along with the eyes (Duchowski, 2003).
2. Most commercial eye-tracking systems available today - “corneal-reflection/pupil-centre” method (Goldberg & Wichansky, 2003).
2.1. Procedures
- In operation, infrared light from an LED embedded in the infrared camera is first directed into the eye to create strong reflections in target eye features to make them easier to track (infrared light is used to avoid dazzling the user with visible light). The light enters the retina and a large proportion of it is reflected back, making the pupil appear as a bright, well defined disc (known as the “bright pupil” effect). The corneal reflection (or first Purkinje image) is also generated by the infrared light, appearing as a small, but sharp, glint.
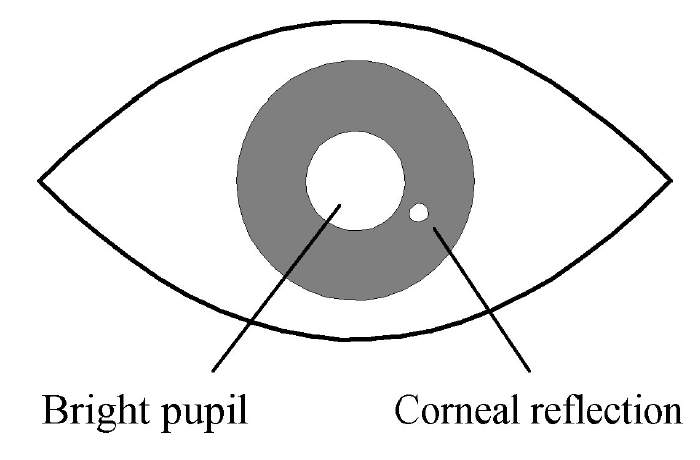
Figure 1. Corneal reflection and bright pupil as seen in the infrared camera image
- Once the image processing software has identified the centre of the pupil and the location of the corneal reflection, the vector between them is measured, and, with further trigonometric calculations, point-of-regard can be found.
3. Pupil detection based eye tracking4
3.1 Six state-of-art pupil detection methods
- EISe
- ExCuSe
- Pupil Labs
- SET
- Starurst
- Swirski
3.2 Introduction
- Probably the most popular algorithm in this realm is Starburst.
- But EISe shows superior performance and proves to be the most robust algorithm when employed in outdoor scenarios.
- Note that we do not report performance measures related to the gaze position on the scene, since this also depends on the calibration. We focus on the pupil center position on the eye images, where the first source of noise occurs.
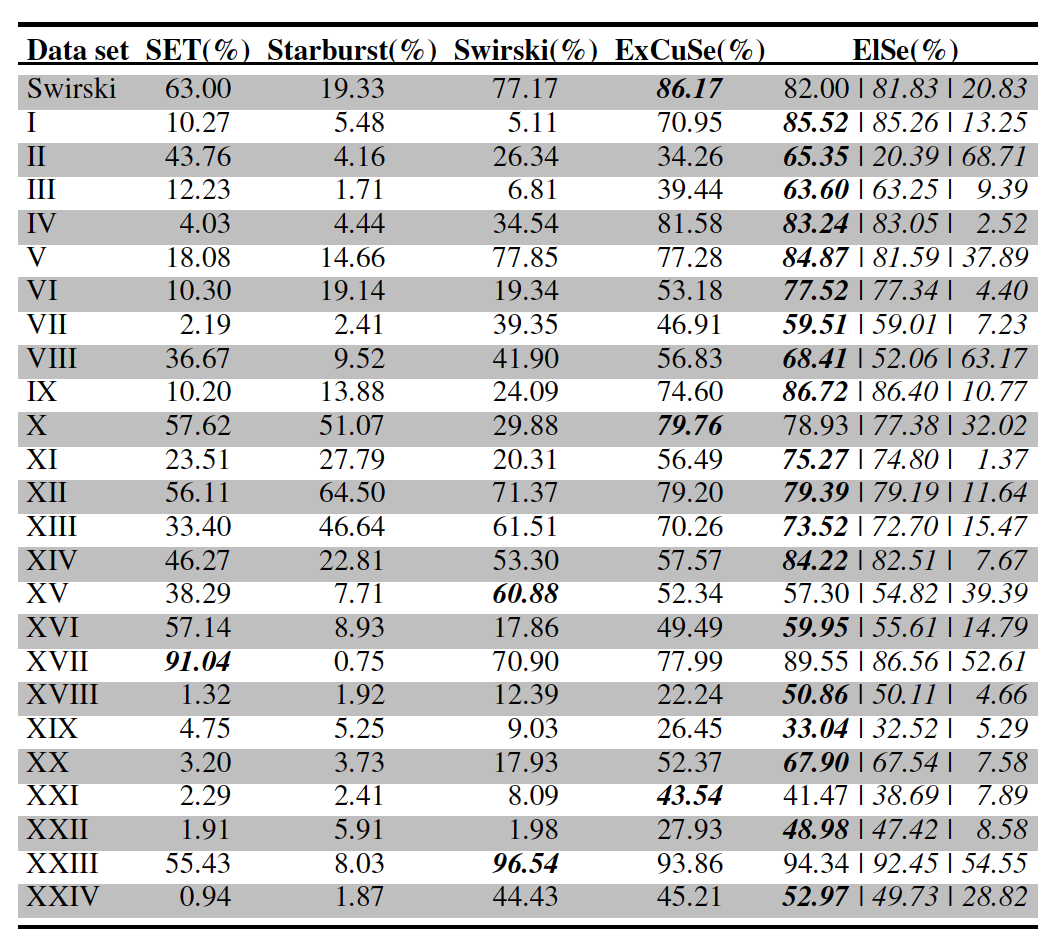
Figure 2. Comparsion between different algorithms. This table don't report performance measures related to the gaze position, since this also depends on the calibration. It focus the pupil center position on the eye images, where the first source of noise occurs.
3.3 EISe 5
- Introduction: Ellipse Selector (ElSe for short) based on edge filtering, ellipse evaluation, and pupil validation.
- Procedures
- First estimates a likely location candidate and then refines this position.
- Afterward, the image is convolved with two different filters separately:
- (1). a surface difference filter to claculate the area difference between an inner circle and a surrounding box
- (2). a mean filter
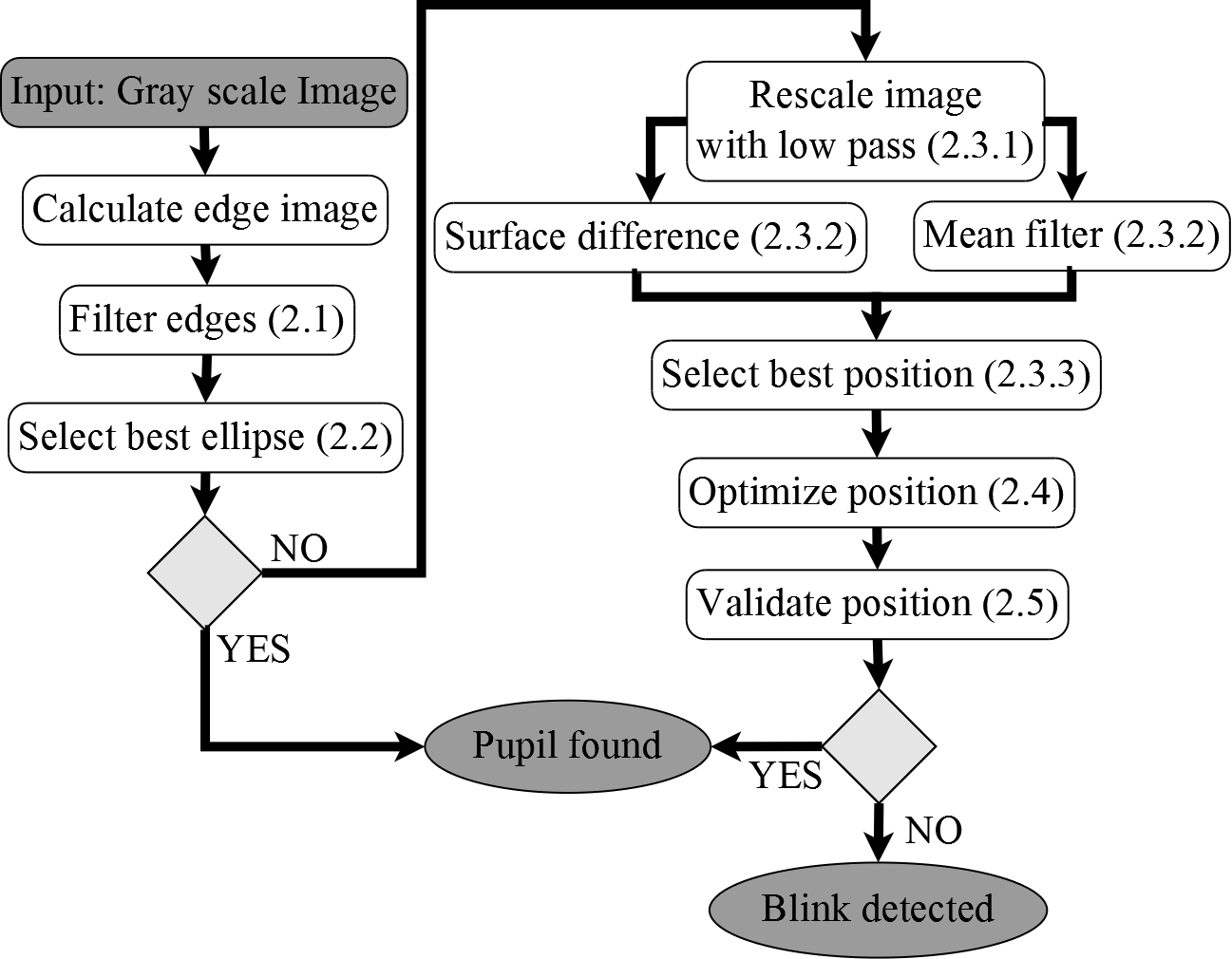
Figure 3. Flowchart of the proposed algorithm. Light gray boxes represent decisions, dark gray ellipses termination points, and white boxes represent processing steps.
Skin Conductance(Galavic Skin Response or Electro Dermal Response)
Studying stress using Skin Conductance 6
1. Basic Introduction
- Thirty-one participants were shown 5 Scenarios respectively. During the demonstration, the skin conductance data of the experimenters were recorded. After the experiment, the participants need to score the emotions of 1-7 for the five segments of experience in order to subjectively score.
- The collected skin conductance signals were first preprocessed and then used to train seven popular ma-chine learning classifiers to automatically detect the two emotional classes (stress – no stress) from skin conductance.
2. Setting and Equipment
- Skin conductance was recorded at 5Hz using a Mindfield eSense sensor, the skin conductance sensor was place on participants’ non dominant hand in the middle and ring finger respectively.
3. Analysis and Results
- The collected signals were smoothed using hanning window function. Smoothing window w