4 Neural Network acceleration: hardware
In this section, we introduce the hardware implementation of neural networks, from general-purpose processors to vanilla accelerators with sole hardware optimization and modern accelerators with algorithm-hardware codesign. Before presenting the details, we first explain the computation pattern of neural networks because it is the basis of the latter hardware design. Fig. 21 illustrates two typical workloads in running neural networks, that is, the Conv layer and the FC layer. The former features Conv operations while the latter features matrix–vector multiplications (MVMs). In the Conv operation, there is huge data reusability including both activations and weights; by contrast, the data cannot be reused in the MVM operation if without the batching technique. In fact, the computation of one sliding window in the Conv operation is equivalent to an MVM operation.
在本节中,我们将介绍神经网络的硬件实现,从通用处理器到具有单一硬件优化的普通加速器,以及具有算法-硬件协同设计的现代加速器。在详细介绍之前,我们首先说明神经网络的计算模式,因为它是后一种硬件设计的基础。图21为运行神经网络的两种典型工作方式,即Conv层和FC层。前者的特点是卷积运算,后者的特点是矩阵向量乘法(MVM)。在Conv操作中,存在大量的数据重用性,包括激活和权重;相比之下,如果没有批处理技术,数据就不能在MVM操作中重用。实际上,Conv操作中一个滑动窗口的计算相当于一个MVM操作。
A. Why Domain-Specific Accelerator for Neural Networks?
Besides innovative algorithms, increasing data resources, and easy-to-use programming tools, the rapid development of neural networks also heavily relies on the computing capability of the hardware. The general-purpose processors such as GPUs act as the mainstay in the deep learning era, especially on the cloud side. GPUs keep an ongoing pursuit of high throughput, whereas they pay the cost of huge resource overhead and energy consumption.
除了创新的算法、增加的数据资源和易于使用的编程工具外,神经网络的快速发展还严重依赖于硬件的计算能力。 GPU等通用处理器在深度学习时代起着中流砥柱的作用,特别是在云计算方面。 GPU一直追求高吞吐量,然而他们付出的代价是巨大的资源开销和能源消耗。
For the edge applications, the budget on resource and energy is usually very limited. How to minimize the latency, energy, and area has become an inevitable design concern. Although the use of on-GPU compression such as [201], [233], [250], and [251] can improve the performance, there is still a big gap far from our expectation due to the redundant design of general-purpose processors for flexible programmability and general applicability. This motivates the study of specialized accelerators tailored for neural networks. By sacrificing the flexibility to some extent, these accelerators focus on the specific pattern of neural networks to achieve satisfactory performance through the optimization of processing architecture, memory hierarchy, and dataflow mapping. Note that most neural network accelerators are intended for the inference phase and the CNN models due to their wide applications on the edge side. Although we can find a few ones for the training phase [252]–[254], RNN models [255] or both CNNs and RNNs [256]–[258], they are still not the mainstream. Therefore, the default neural network accelerators in this article indicate the CNN inference scenario unless otherwise specified. Due to the limited space, we just review the recent accelerators that can support large-scale neural networks and ignore the early ones [259], [260].
对于边缘应用,资源和能源的预算通常非常有限。如何使延迟、能量和面积最小化成为设计中不可避免的问题。虽然使用针对GPU的压缩技术[201]、[233]、[250]、[251]可以提高性能,但由于通用处理器的冗余设计,使得距具有灵活的可编程性和通用性,仍然有很大的差距,与我们的预期相距甚远。这激发了专门为神经网络设计的加速器的研究。这些加速器在一定程度上牺牲了灵活性,专注于特定的神经网络模式,通过优化处理体系结构、内存层次结构和数据流映射来实现令人满意的性能。请注意,大多数神经网络加速器用于推理阶段和CNN模型,因为它们在边缘方面的应用非常广泛。虽然我们可以找到一些用于训练阶段[252]-[254]、RNN模型[255]或CNN和RNN[256] -[258]的模型,但它们仍不是主流。因此,除非另有说明,本文中默认的神经网络加速器表示CNN推理场景。由于篇幅有限,我们只回顾了最近支持大规模神经网络的加速器,而忽略了早期的加速器[259],[260]。
B. Sole Hardware Optimization
1) Parallel Compute Units and Orchestrated Memory Hierarchy: Usually, neural network accelerators make efforts in two aspects: enhancing the compute parallelism and optimizing the memory hierarchy. For example, DaDianNao [8] distributed weight memory into multiple tiles for the better locality. The MAC operations are performed in parallel by these tiles and the intermediate activations of different tiles are exchanged through a central memory. By contrast, in other neural network accelerators (e.g., Eyeriss [261], TPU [9], and Thinker [257]), the architecture often has an array of processing elements (PEs) with a small local buffer for each and a global buffer to hide the off-chip DRAM access latency, as shown in Fig. 22. Double buffering technique can be used in the global buffer to prefetch data before layer computation [257].
1) 并行计算单元和灵活组合内存层次结构:通常,神经网络加速器从两个方面展开:增强计算的并行性和优化内存层次。例如,DaDianNao[8]将权重内存分配到多个块中,以获得更好的性能。MAC操作是由这些块和不同的中间激活并行执行的拼接通过一个中央存储器交换。相比之下,在其他神经网络加速器(如Eyeriss[261]、TPU[9]和Thinker[257])中,该体系结构通常有一组处理元素(PE),每个处理元素都有一个小的本地缓冲区,还有一个全局缓冲区来隐藏片外DRAM访问延迟,如图22所示。在全局缓冲区中可以使用双缓冲技术,在层计算之前预取数据[257]。
The PE array features a dataflow processing fashion with an orchestrated network-on-chip (NoC) enabling direct message passing between PEs. Three types of data, input activation, weight, and partial sum (psum) of output activation, flow through the PE array when performing a Conv or MVM operation, which increases the data reuse thus decreasing the requirement for memory bandwidth. Furthermore, the dataflow pattern can be variable in a different design. We use Fig. 23 to briefly explain it. As depicted in Fig. 23(a), the output psum is stationary in each PE, and the input activations and weights propagate across PEs along the row and column directions, respectively. In this way, the inputs and weights can be reused by multiple PEs, which can reduce the memory access. Besides the output stationary dataflow [257], we can also see architectures with input stationary dataflow [262] or weight stationary dataflow [9], as shown in Fig. 23(b) and (c). Eyeriss [261] uses another dataflow called row stationary dataflow that is illustrated in Fig. 23(d). Specifically, each PE performs the Conv operation between one weight row and one input row, and the PEs in the same column generates one output row. The weights and psums propagate across PEs along the row and column directions, respectively, whereas the input activations propagate along the diagonal direction that is different from other dataflow solutions mentioned above.
PE阵列采用了一种数据流处理方式,通过设计的片上网络(NoC)实现PE之间的直接消息传递。当执行Conv或MVM操作时,PE阵列中有三种类型的数据:输入激活、权重和输出激活的部分和(psum),这增加了数据的重用性,从而降低了对内存带宽的要求。此外,数据流模式在不同的设计中可以是可变的。我们用图23来简单解释一下。如图23(a)所示,输出psum在每个PE中是平稳的,而输入激活和权值分别沿着行方向和列方向在PE中传播。这样,输入和权值可以被多个PE重用,从而减少了内存访问。除了输出固定数据流[257],我们也可以看到在图23(b)和(c)中有输入平稳数据流[262]和权重平稳数据流[9]的架构。Eyeriss[261]使用了另一种称为行平稳数据流的数据流,如图23(d)所示。具体来说,每个PE在一个权重行和一个输入行之间执行Conv操作,同一列的PE生成一个输出行。权重和pme分别沿行方向和列方向在PE之间传播,而输入激活则沿不同于上述其他数据流解决方案的对角线方向传播。
Combining the weight distribution [8] with the inter-PE data passing [9], [257], Tianjic [264], [265] and Simba [266], [267] adopt a scalable many-core/many-chip architecture where all cores (i.e., PEs) work in a decentralized manner without the global off-chip memory. Compared with the above accelerators, this emerging architecture is more spatial, as presented in Fig. 24. The weights are preloaded into each PE and remain stationary during the entire inference, and the activations propagate across intrachip and interchip cores.
将权重分配[8]与PE间数据传递[9]、[257]、Tianjic[264]、[265]和Simba[266]相结合,采用可扩展的多核/多芯片体系结构,其中所有核(即PE)都以分散的方式工作,而不需要全局片外存储器。 与上述加速器相比,这种新兴的体系结构更具空间性,如图所示 24. 权重被预加载到每个PE中,并在整个推理过程中保持不变,并且激活在芯片内和芯片间传输。
2) Processing-in-Memory (PIM) Architecture: In conventional digital neural network accelerators, an MVM operation is split into many MAC operations to perform cycle by cycle. To improve the efficiency of performing MVM the PIM architecture based on emerging nonvolatile memory (eNVM) technologies has been widely studied. Taking memristor (e.g., RRAM [268], PCRAM [252]) as an example, the MVM can be performed in the analog domain. Each column of the crossbar obeys =
, where
is the input voltage of the ith row,
is the output current of the j th column, and
is the memristor device conductance at the (i,j)th crosspoint. The weights are prestored as G, and the input and output activations correspond to V and I, respectively. The entire MVM can be processed in the analog domain with only one cycle, which is ultrafast. Nevertheless, ADCs (ADCs and DACs) are usually needed, which causes extra overhead. For the current to voltage (I2V) converters, they can be implemented either explicitly [258], [268] or implicitly [269] in different designs. The complete architecture is similar to the spatial architectures in [264]–[267] (see Fig. 24), where each MVM engine is a weight-stationary PE and a communication infrastructure helps the activation passing between PEs. Besides the MVM operation, some other operations such as scalar and vector operations should be additionally supported by PEs since they are necessary for some neural network models such as RNNs but cannot be handled by the memristor array efficiently [258]. Besides eNVM devices, traditional memories such as SRAM [270],DRAM [271], and Flash [272] can also be modified to support the PIM-fashion processing of neural networks. However, only small-scale prototype chips have been taped out due to the difficulty in fabrication, therefore they are not widely used in industry although they are very hot in academia.
2)内存中处理(PIM)体系结构:在传统的数字神经网络加速器中,一个MVM操作被分割成多个MAC操作,一个周期一个周期地执行。为了提高MVM的执行效率,基于新兴非易失性内存(eNVM)技术的PIM体系结构得到了广泛的研究。以忆阻器(如RRAM [268], PCRAM[252])为例,MVM可以在模拟域进行。交叉开关的每一列都服从=
,其中
为第i行输入电压,
为第j列输出电流,
为第(i,j)个交点处的忆阻器件电导。权值预存储为G,输入和输出激活分别对应V和I。整个MVM可以在模拟域内处理,只需一个周期,速度非常快。然而,通常需要ADC (ADC和DAC),这造成了额外的开销。对于电流-电压(I2V)转换器,它们可以在不同的设计中显式实现[258]、[268]或隐式实现[269]。完整的架构类似于[264]-[267]中的空间架构(见图24),其中每个MVM引擎是一个权重固定的PE,通信基础设施帮助激活PE之间的传递.除了MVM操作,一些其他的操作,如标量和向量操作,PEs需要额外支持,因为这些操作对于一些神经网络模型(如RNNs)是必需的,但忆阻器阵列不能有效地处理[258]。除了eNVM设备外,传统的存储器如SRAM[270]、DRAM[271]、Flash[272]也可以被修改以支持神经网络的PIM方式处理。然而,由于制造的困难,目前只有小规模的芯片原型,因此尽管在学术界非常热门,但在工业上还没有广泛的应用。
C. Algorithm and Hardware Codesign
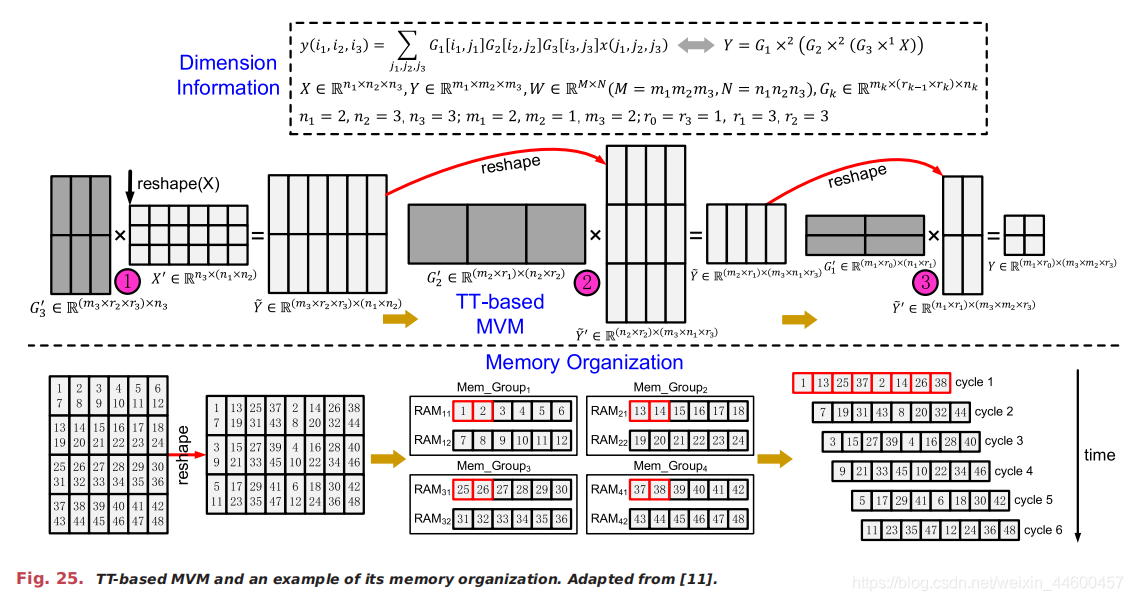
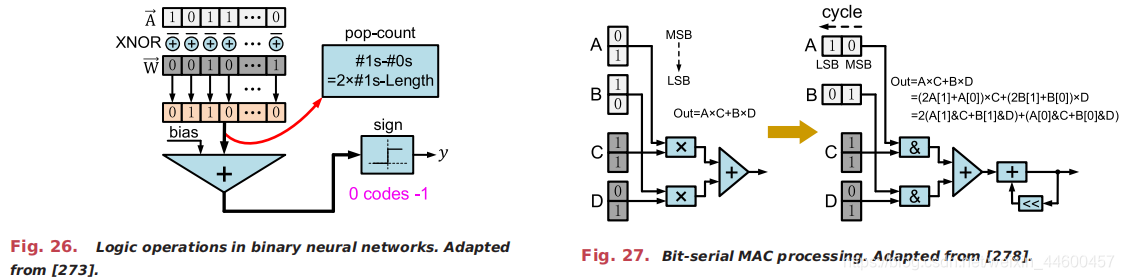
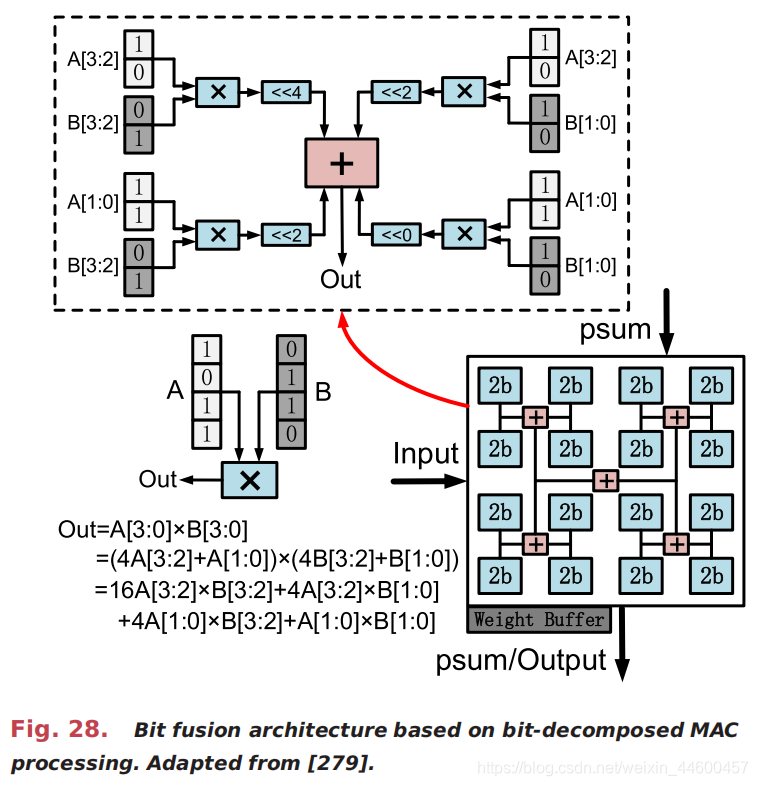
为此,图18中提到的结构化稀疏模式得到了进一步的开发。例如,利用块加权稀疏来优化通用处理器[13],[220],[234]的运行性能,在加速器设计中使用了更激进的对角加权矩阵[282]。在这些体系结构中,所需的索引可以大大减少,每个PE中的MAC数量变得更加平衡,内存组织/访问可以更加高效。图30显示了在收缩阵列中利用矢量稀疏性的示例[263]。多列上的非零权重(例如,本例中相邻的两列)被组合在一起,只保留每行上绝对最大的元素(其余的被修剪)。实际上,为了保证准确性,采用了贪婪列组合和迭代训练的方法。每个PE用一个列索引缓冲非零权重,并且额外集成一个多路复用器来选择正确的权重按权重列索引输入。