SiamRPN source code
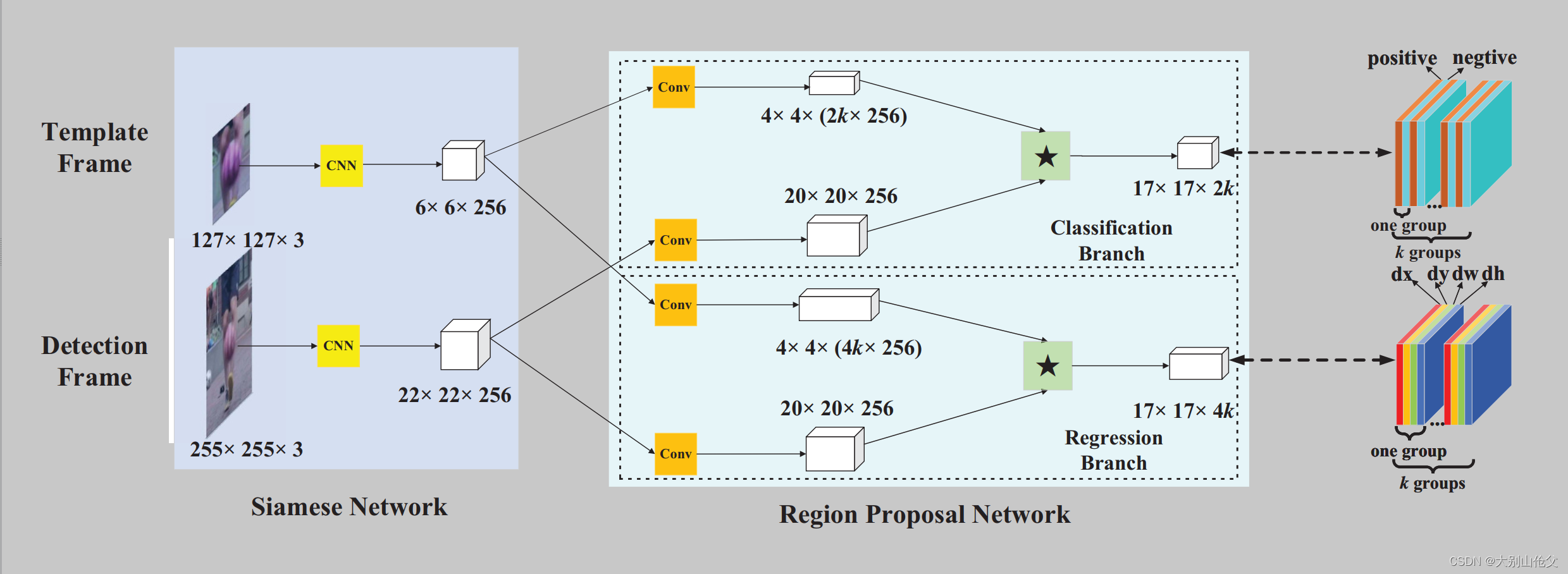
import torch
import torch.nn as nn
import torch.nn.functional as F
from torch.nn.modules.conv import Conv2d
class SiamRPN(nn.Module):
def __init__(self, anchor_num=5):
super(SiamRPN, self).__init__()
self.anchor_num = anchor_num
self.feature = nn.Sequential(
nn.Conv2d(3, 192, 11, 2),
nn.BatchNorm2d(192),
nn.ReLU(inplace=True),
nn.MaxPool2d(3, 2),
nn.Conv2d(192, 512, 5, 1),
nn.BatchNorm2d(512),
nn.ReLU(inplace=True),
nn.MaxPool2d(3, 2),
nn.Conv2d(512, 768, 3, 1),
nn.BatchNorm2d(768),
nn.ReLU(inplace=True),
nn.Conv2d(768, 768, 3, 1),
nn.BatchNorm2d(768),
nn.ReLU(inplace=True),
nn.Conv2d(768, 512, 3, 1),
nn.BatchNorm2d(512)
)
self.conv_reg_z = nn.Conv2d(512, 512*4*anchor_num, 3, 1)
self.conv_cls_z = nn.Conv2d(512, 512*2*anchor_num, 3, 1)
self.conv_reg_x = nn.Conv2d(512, 512, 3)
self.conv_cls_x = nn.Conv2d(512, 512, 3)
self.adjust_reg = nn.Conv2d(4*anchor_num, 4*anchor_num, 1)
def forward(self, z, x):
kernel_reg, kernel_cls = self.learn(z)
return self.inference(x, kernel_reg, kernel_cls)
def learn(self, z):
z = self.feature(z)
print("z.size is:",z.size())
kernel_reg = self.conv_reg_z(z)
kernel_cls = self.conv_cls_z(z)
print("Before view operation, kernel_reg size is:{}, kernel_cls size is:{}".format(kernel_reg.size(), kernel_cls.size()))
k = kernel_reg.size()[-1]
kernel_reg = kernel_reg.view(4*self.anchor_num, 512, k, k)
kernel_cls = kernel_cls.view(2*self.anchor_num, 512, k, k)
print("After view operation,kernel_reg size is:{}, kernel_cls size is:{}".format(kernel_reg.size(), kernel_cls.size()))
print("kernel_reg.size()[-1] is:", k)
return kernel_reg, kernel_cls
def inference(self, x, kernel_reg, kernel_cls):
x = self.feature(x)
print("x.size is:",x.size())
x_reg = self.conv_reg_x(x)
x_cls = self.conv_cls_x(x)
print("Before adjust operation, x_reg size is:{}, x_cls size is:{}".format(x_reg.size(), x_cls.size()))
out_reg = self.adjust_reg(F.conv2d(x_reg, kernel_reg))
out_cls = F.conv2d(x_cls, kernel_cls)
print("After adjust and F.conv2d() operation, x_reg size is:{}, x_cls size is:{}".format(out_reg.size(), out_cls.size()))
return out_reg, out_cls
Test code
net = SiamRPN()
z = torch.ones([1, 3, 127, 127])
x = torch.ones([1, 3, 256, 256])
output = net(z,x)
output is
z.size is: torch.Size([1, 512, 6, 6])
Before view operation, kernel_reg size is:torch.Size([1, 10240, 4, 4]), kernel_cls size is:torch.Size([1, 5120, 4, 4])
After view operation,kernel_reg size is:torch.Size([20, 512, 4, 4]), kernel_cls size is:torch.Size([10, 512, 4, 4])
kernel_reg.size()[-1] is: 4
x.size is: torch.Size([1, 512, 22, 22])
Before adjust operation, x_reg size is:torch.Size([1, 512, 20, 20]), x_cls size is:torch.Size([1, 512, 20, 20])
After adjust and F.conv2d() operation, x_reg size is:torch.Size([1, 20, 17, 17]), x_cls size is:torch.Size([1, 10, 17, 17])
msc code
- Viualize network structure while giving a input size tensor
from torchsummary import summary
class MultipleInputNetDifferentDtypes(nn.Module):
def __init__(self):
super().__init__()
self.fc1a = nn.Linear(300, 50)
self.fc1b = nn.Linear(50, 10)
self.fc2a = nn.Linear(300, 50)
self.fc2b = nn.Linear(50, 10)
def forward(self, x1, x2):
x1 = F.relu(self.fc1a(x1))
x1 = self.fc1b(x1)
x2 = x2.type(torch.float)
x2 = F.relu(self.fc2a(x2))
x2 = self.fc2b(x2)
x = torch.cat((x1, x2), 0)
return F.log_softmax(x, dim=1)
summary(model, [(1, 300), (1, 300)], dtypes=[torch.float, torch.long])