Lecture 1: Introduction of Machine / Deep Learning
文章目录
Chapter 1 Introduction
Machine Learning ≈ \approx ≈ Looking for Function
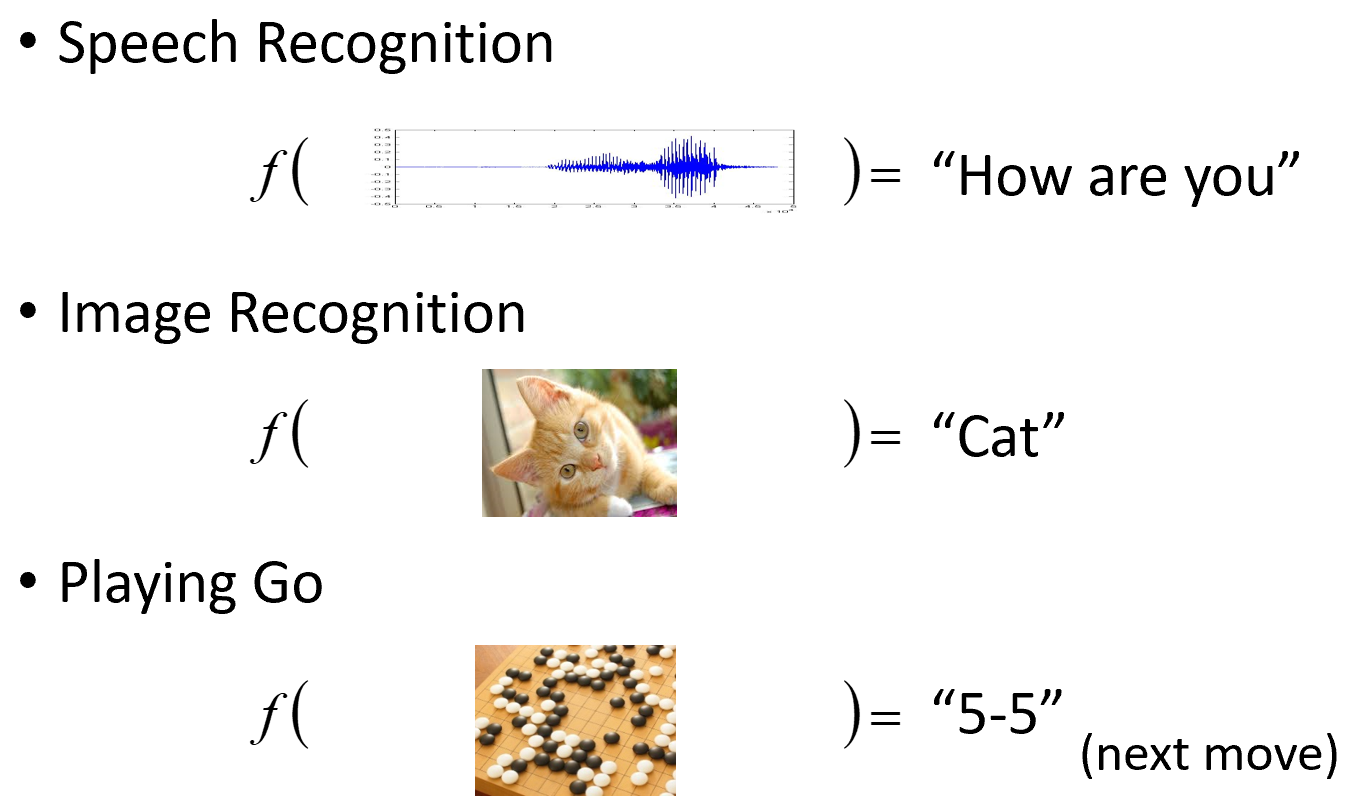
Different types of Functions
Regression
The function outputs a scalar.
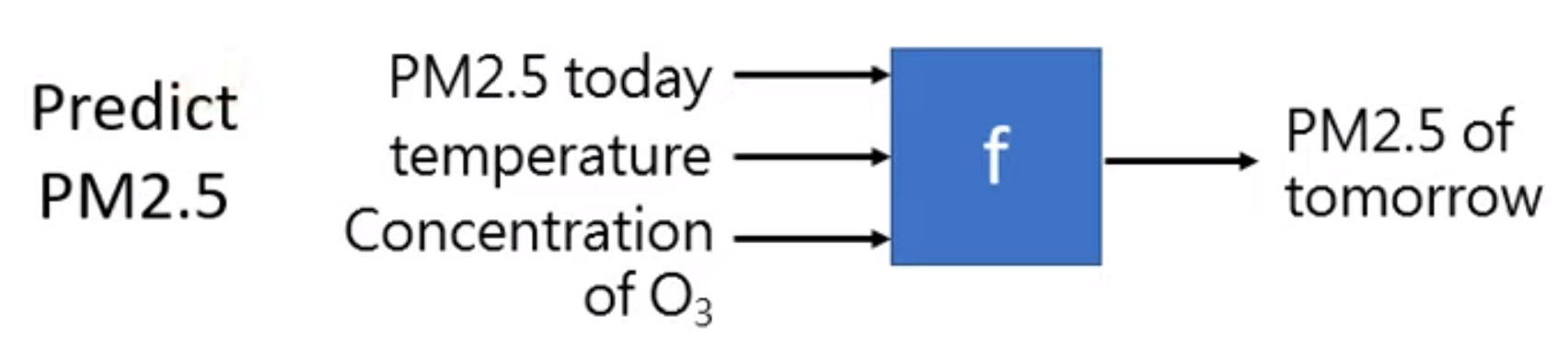
Classification
Given options (classes), the function outputs the correct one.

Structured Learning
create something with structure (image, document) 让机器学会输出一些有特定结构的东西(例如,图片、文档等)
这部分的机器学习任务占比更高。
How to find a function? A Case Study
Function with Unknown Parameters
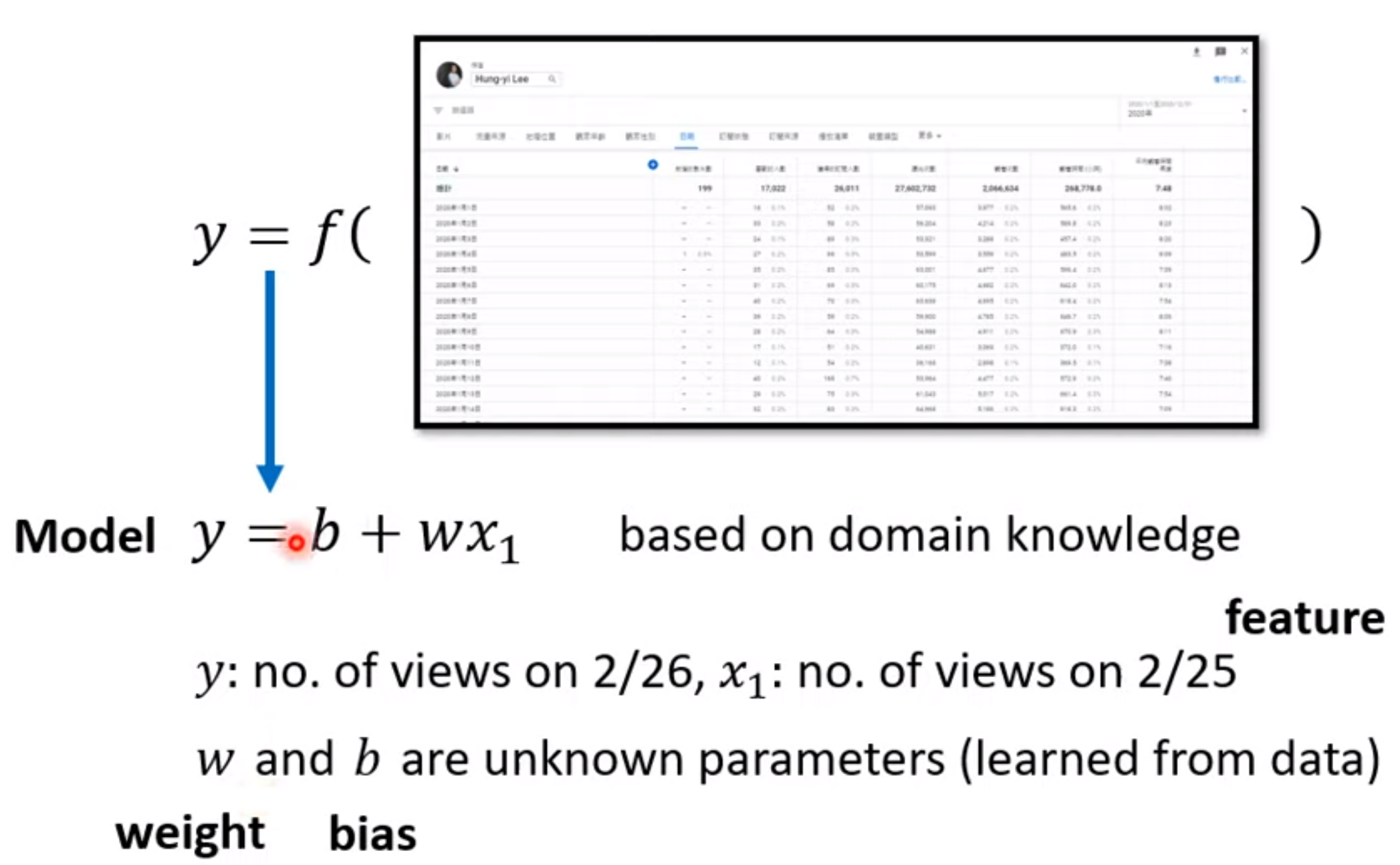
Define Loss from Training Data
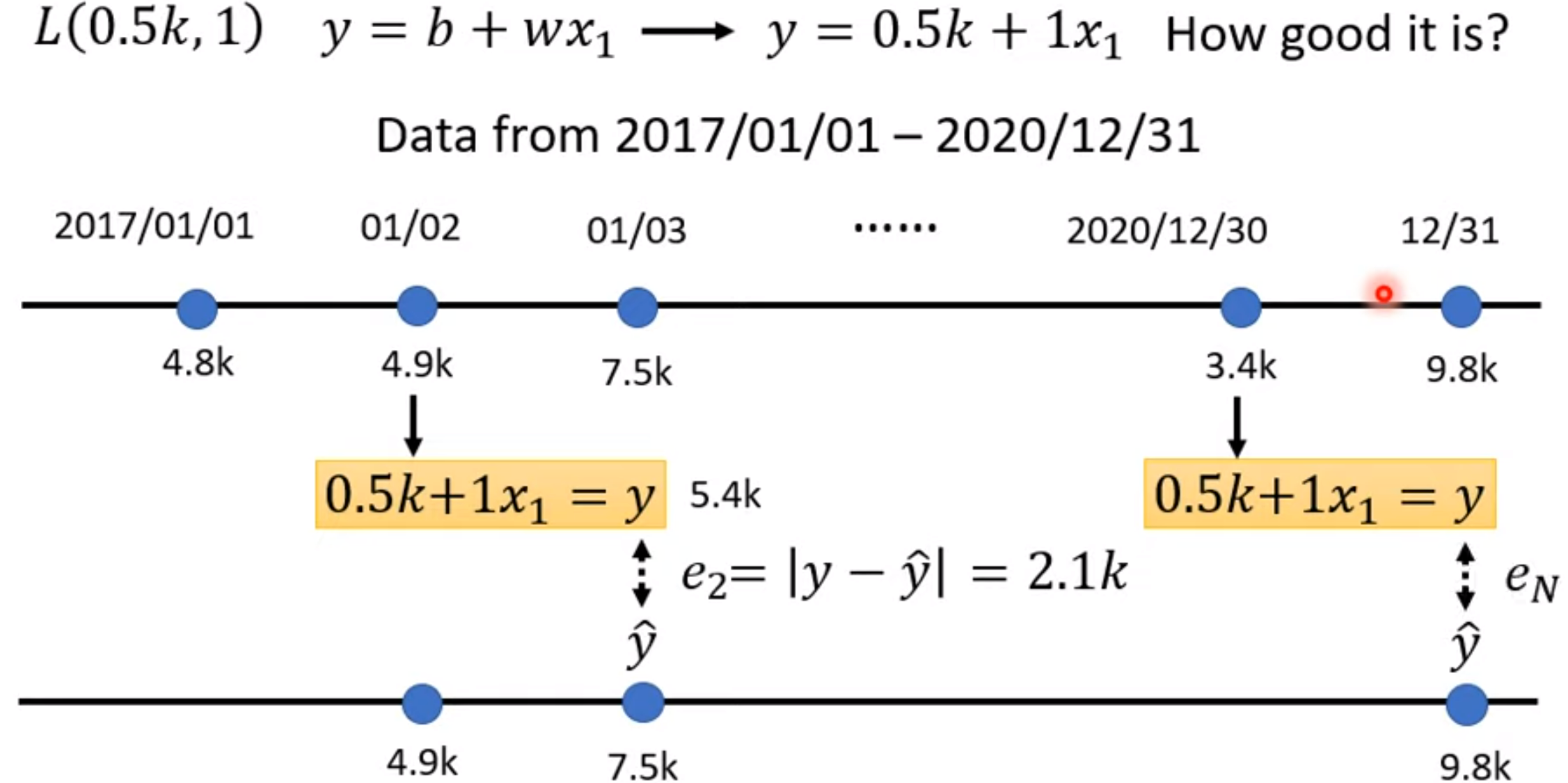
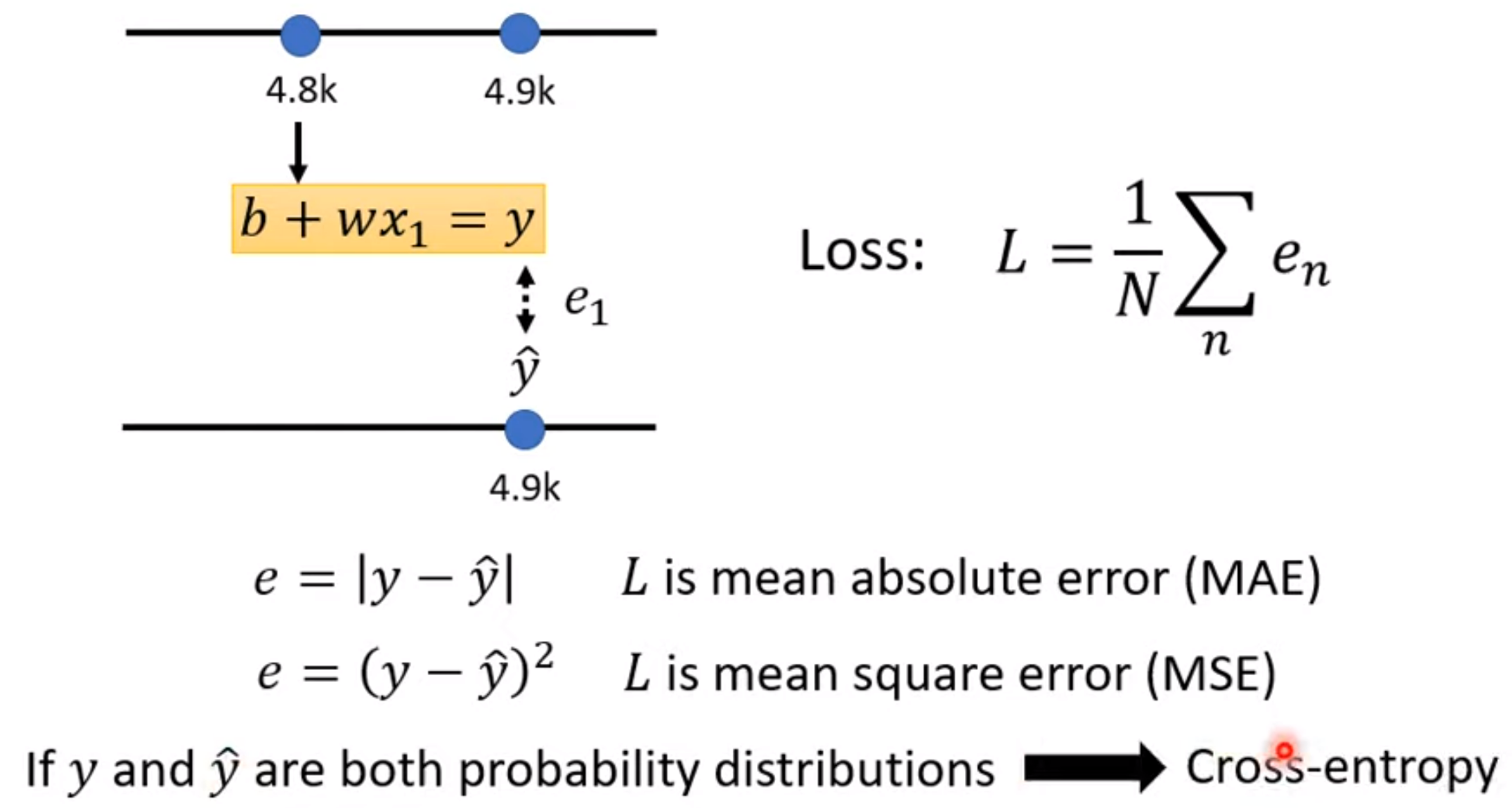
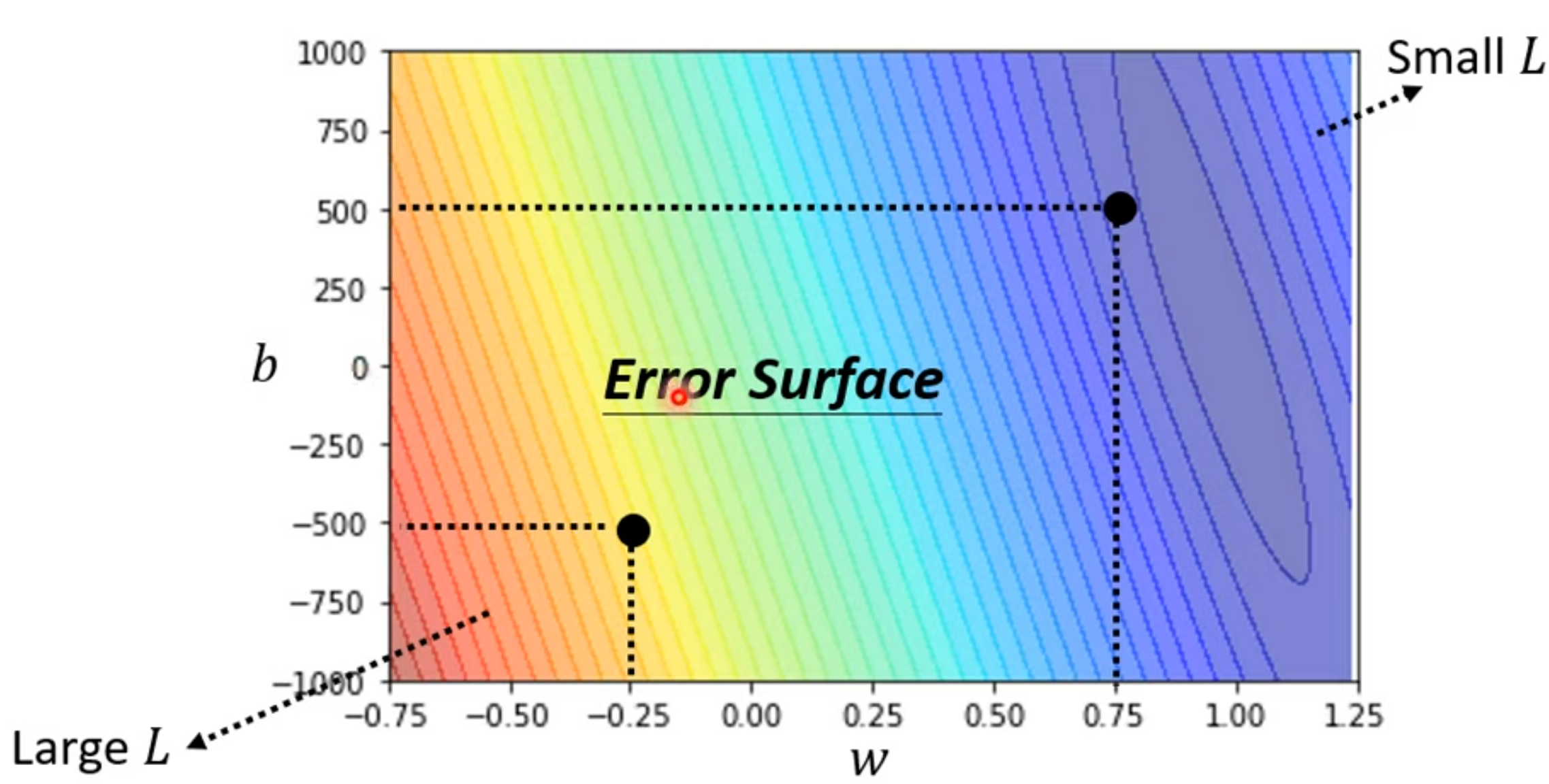
Optimization
w ∗ , b ∗ = arg min w , b L w^*,b^*=\arg \min_{w,b} L w∗,b∗=argw,bminL
Gradient Descent
单个参数做梯度下降,比如将 b b b 作为常数处理
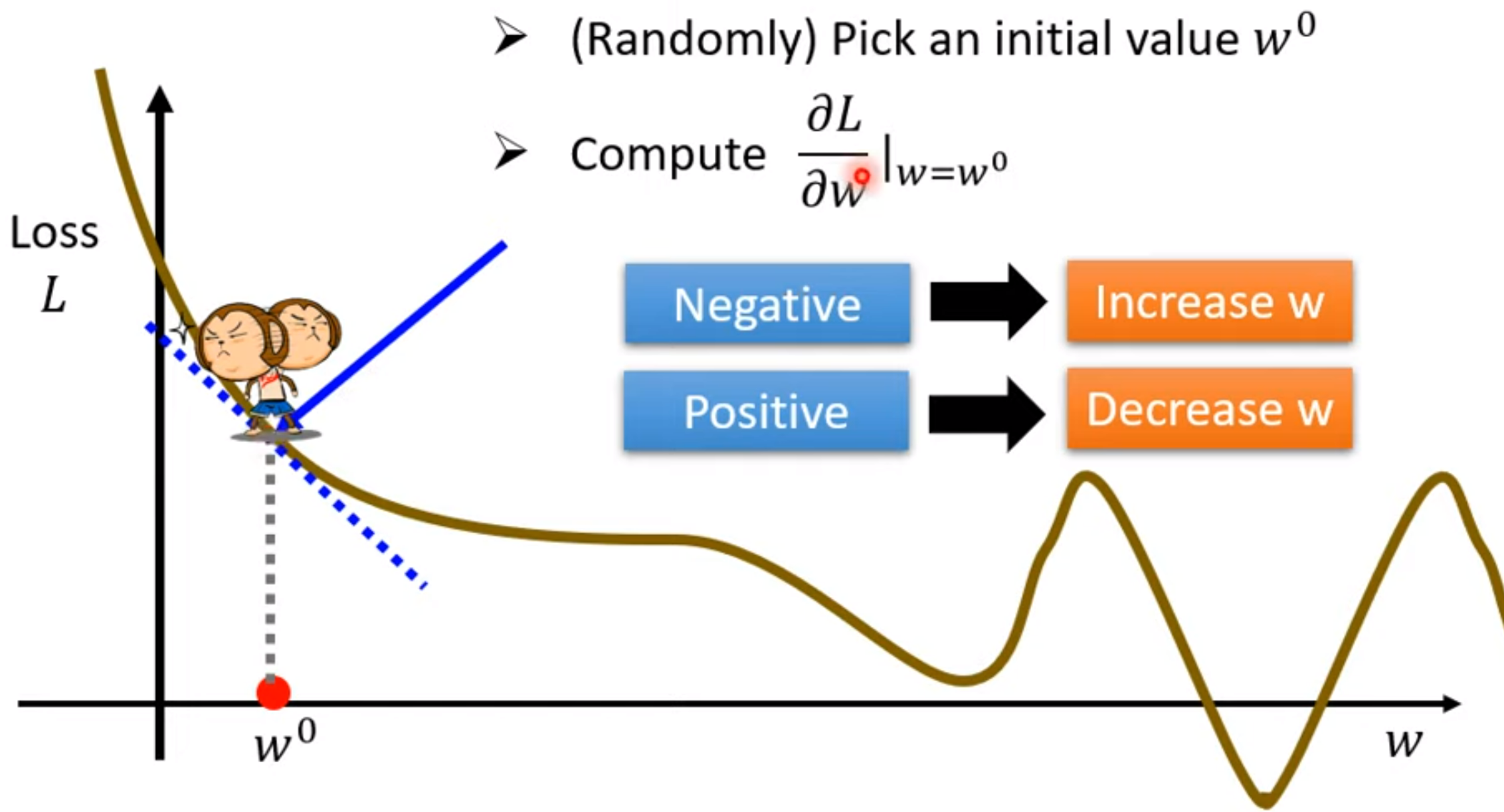
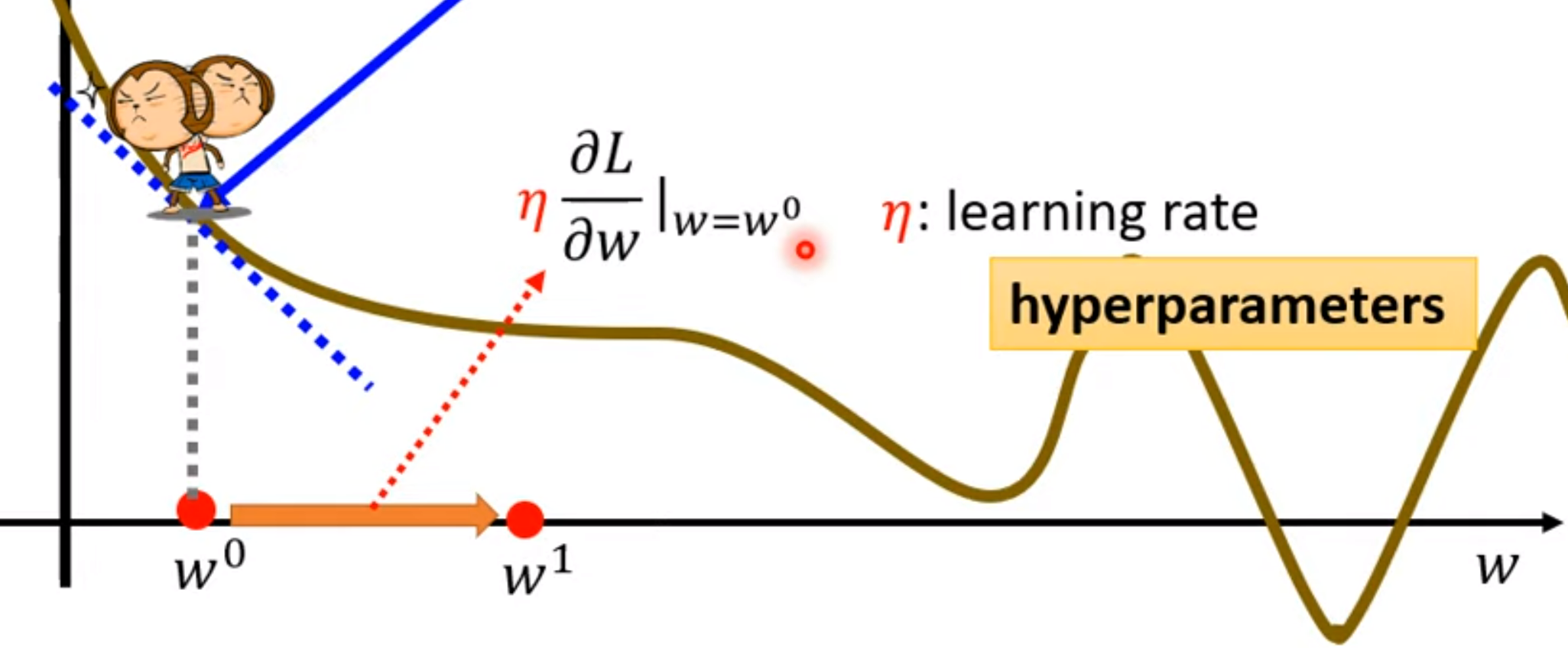
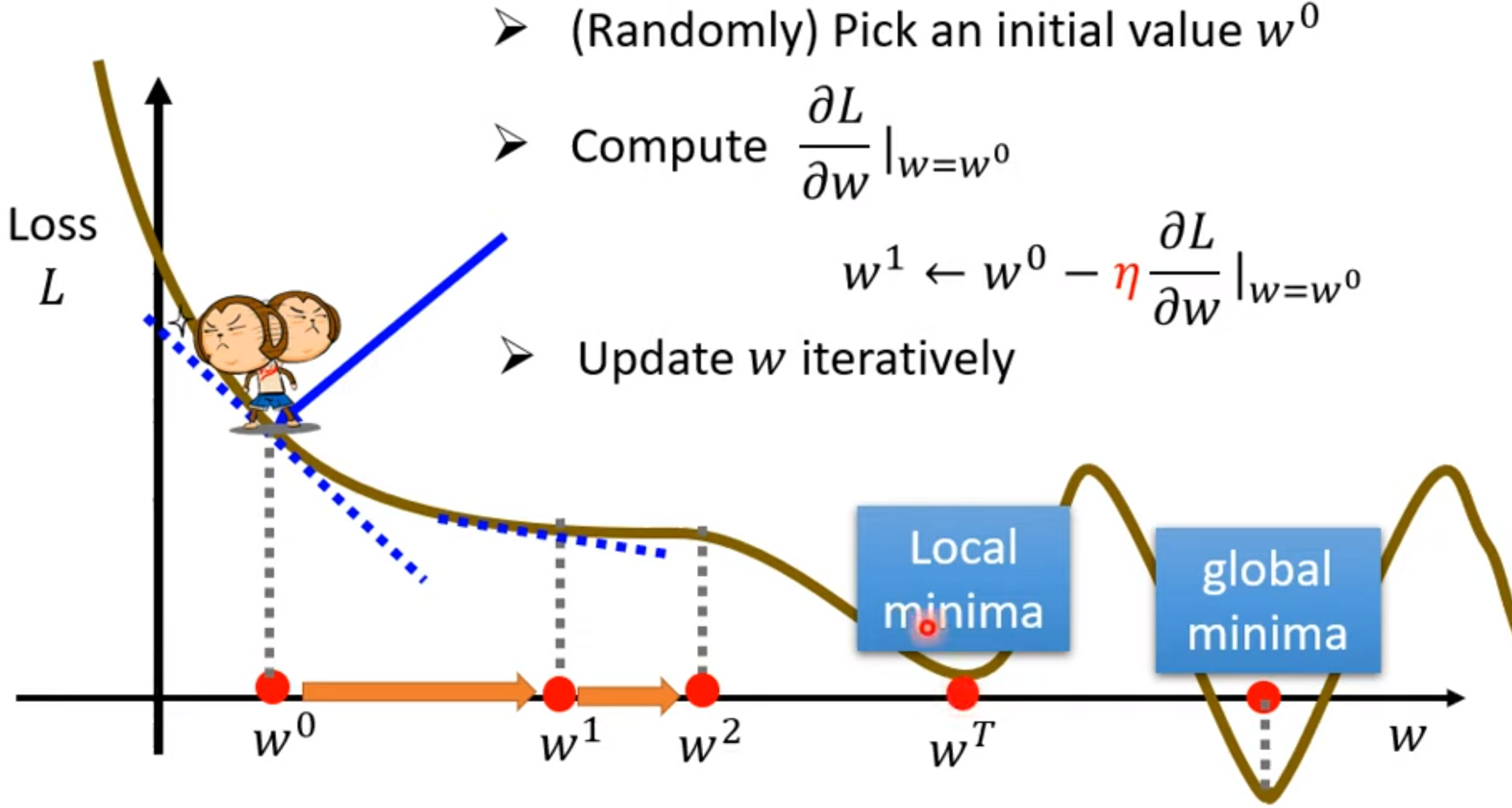
两个参数做梯度下降
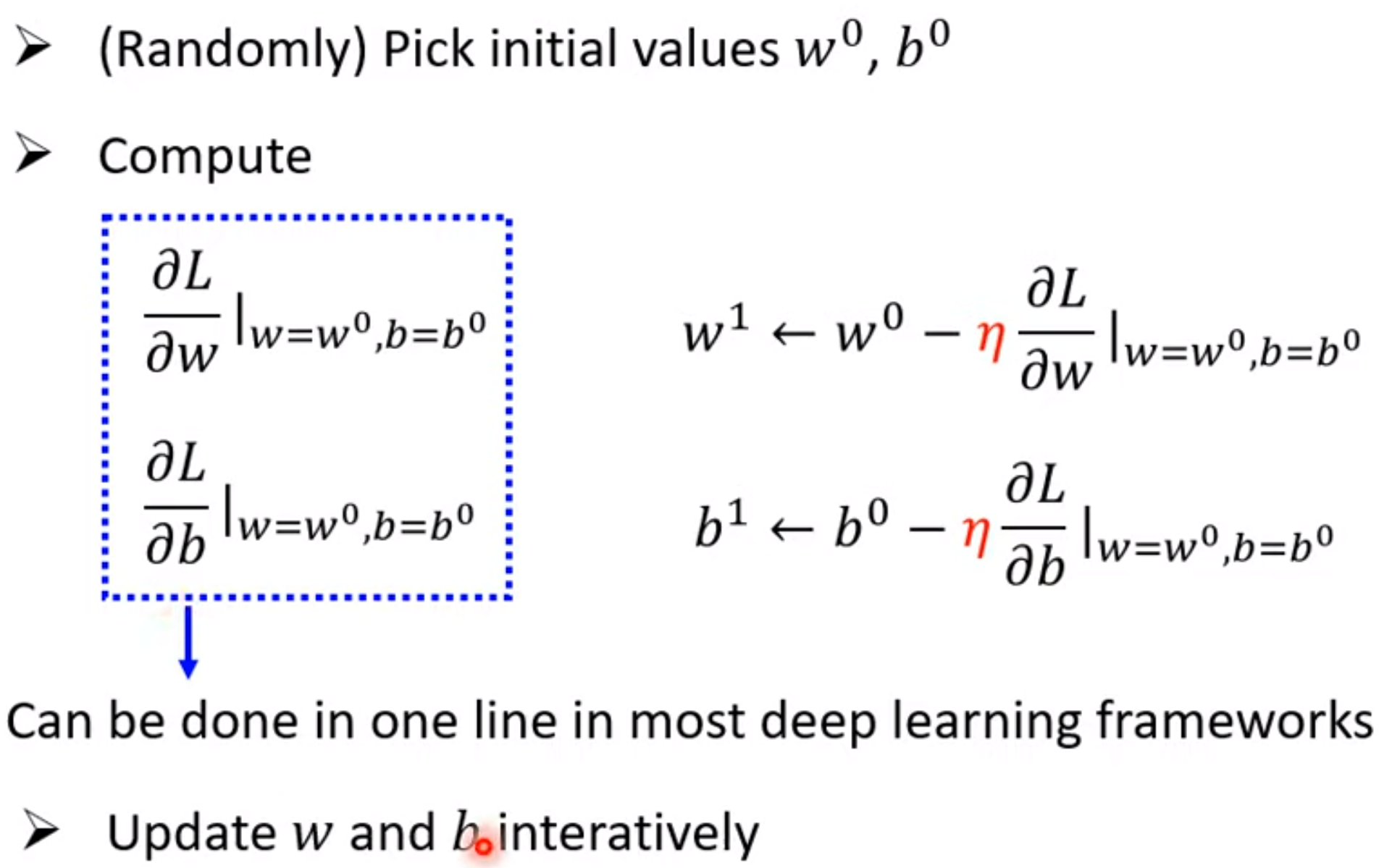
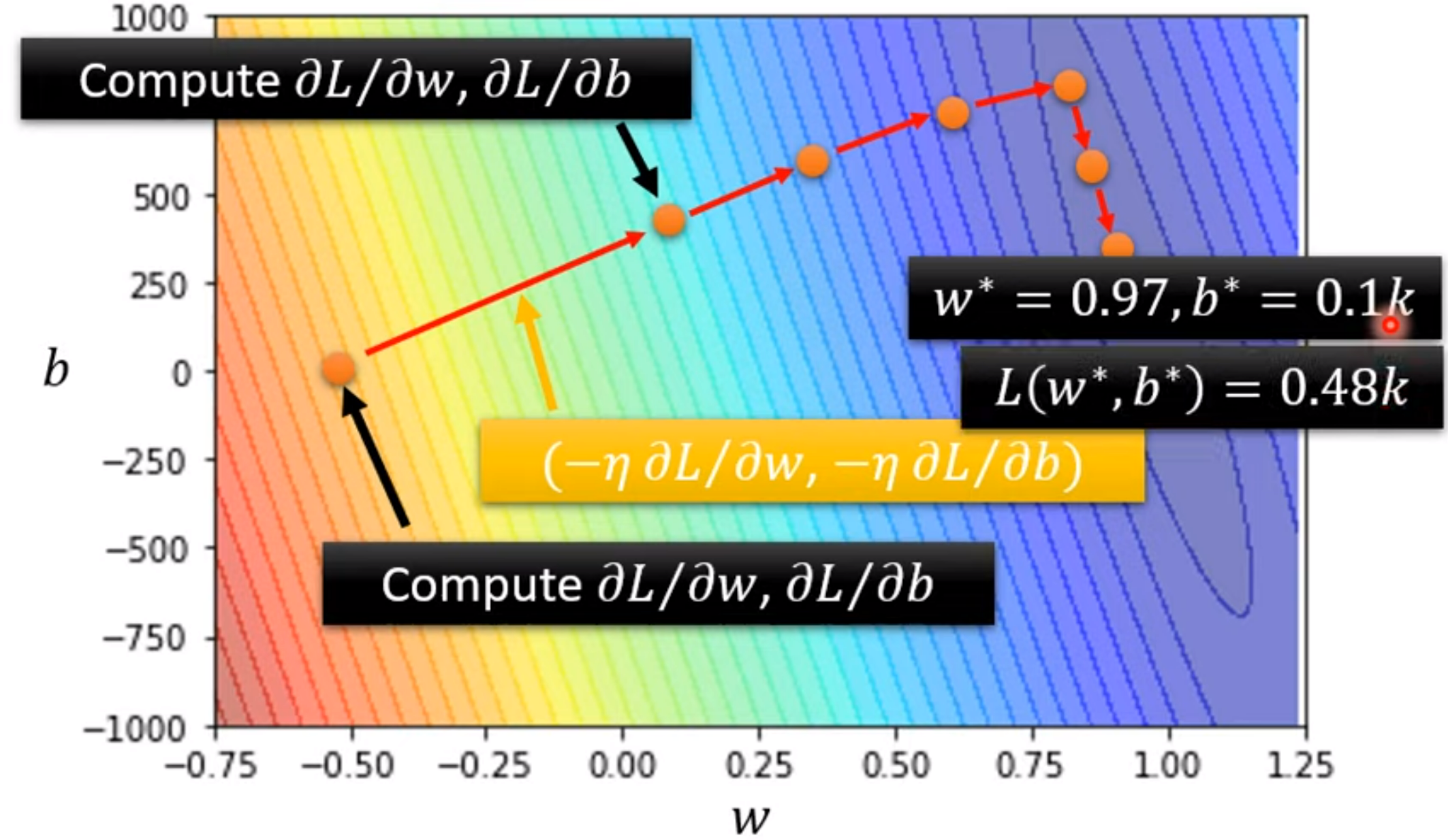
Training Procedure of Machine Learning
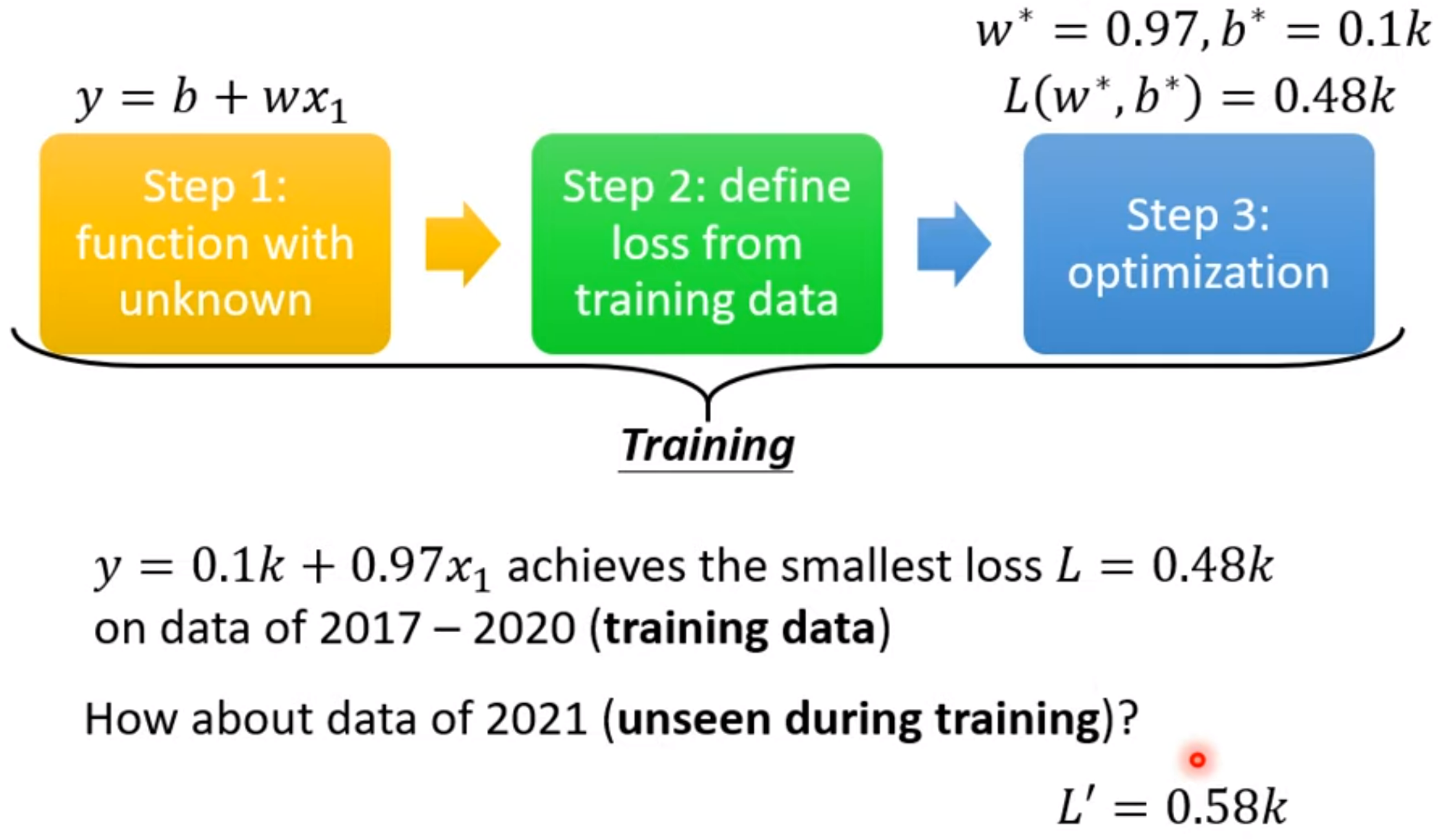
Linear models are too simple, we need more sophisticated modes.
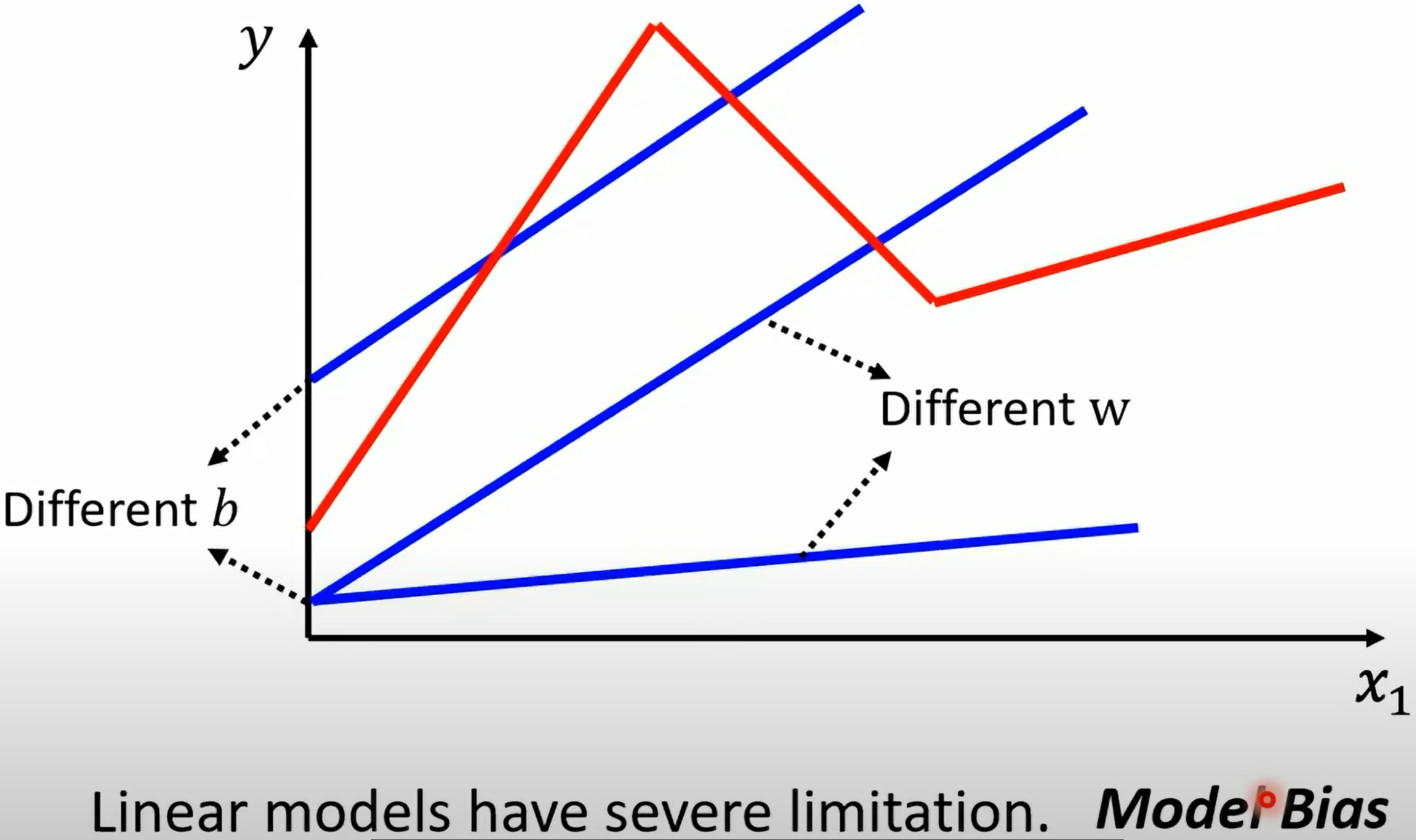
Sigmoid Function
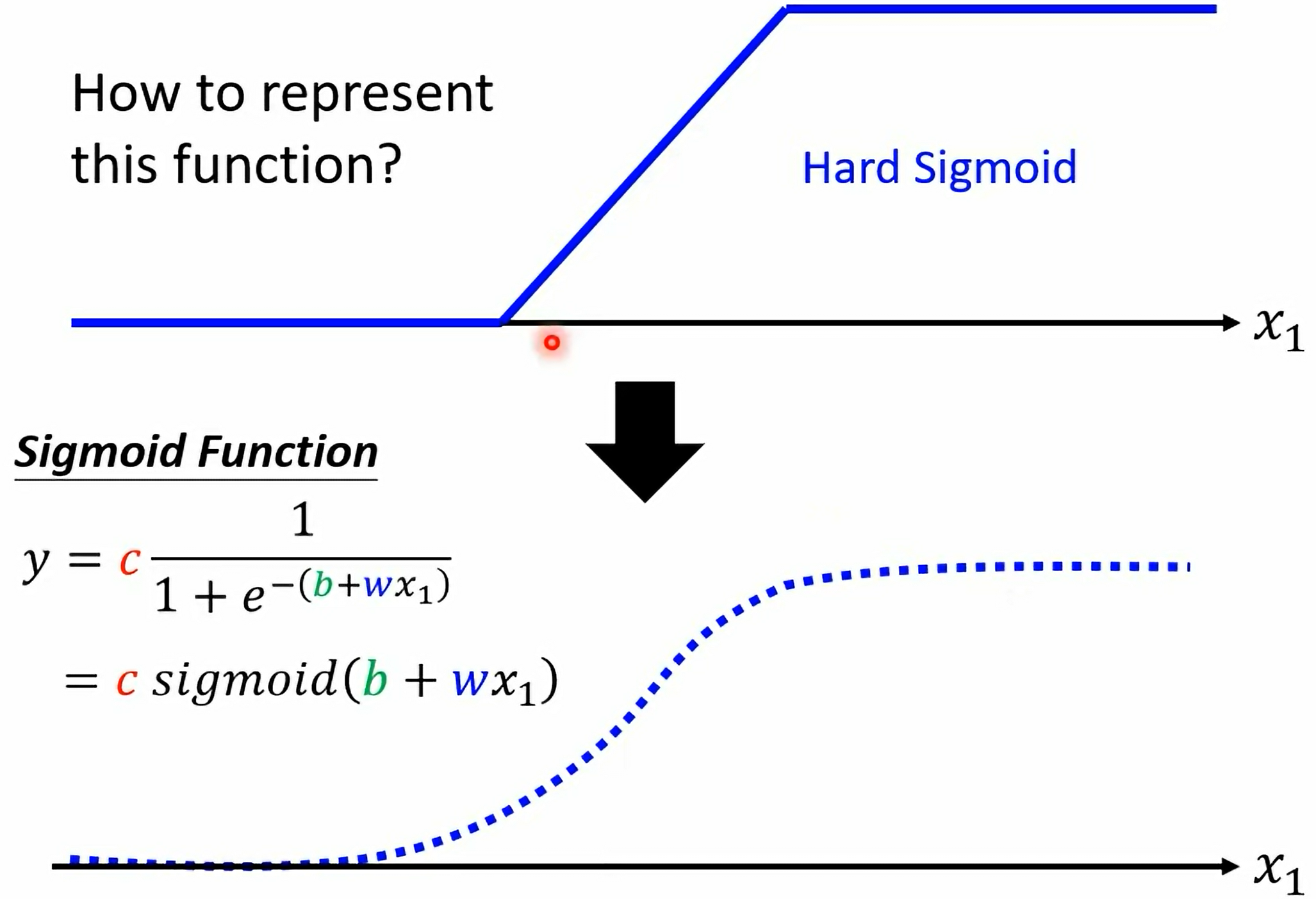
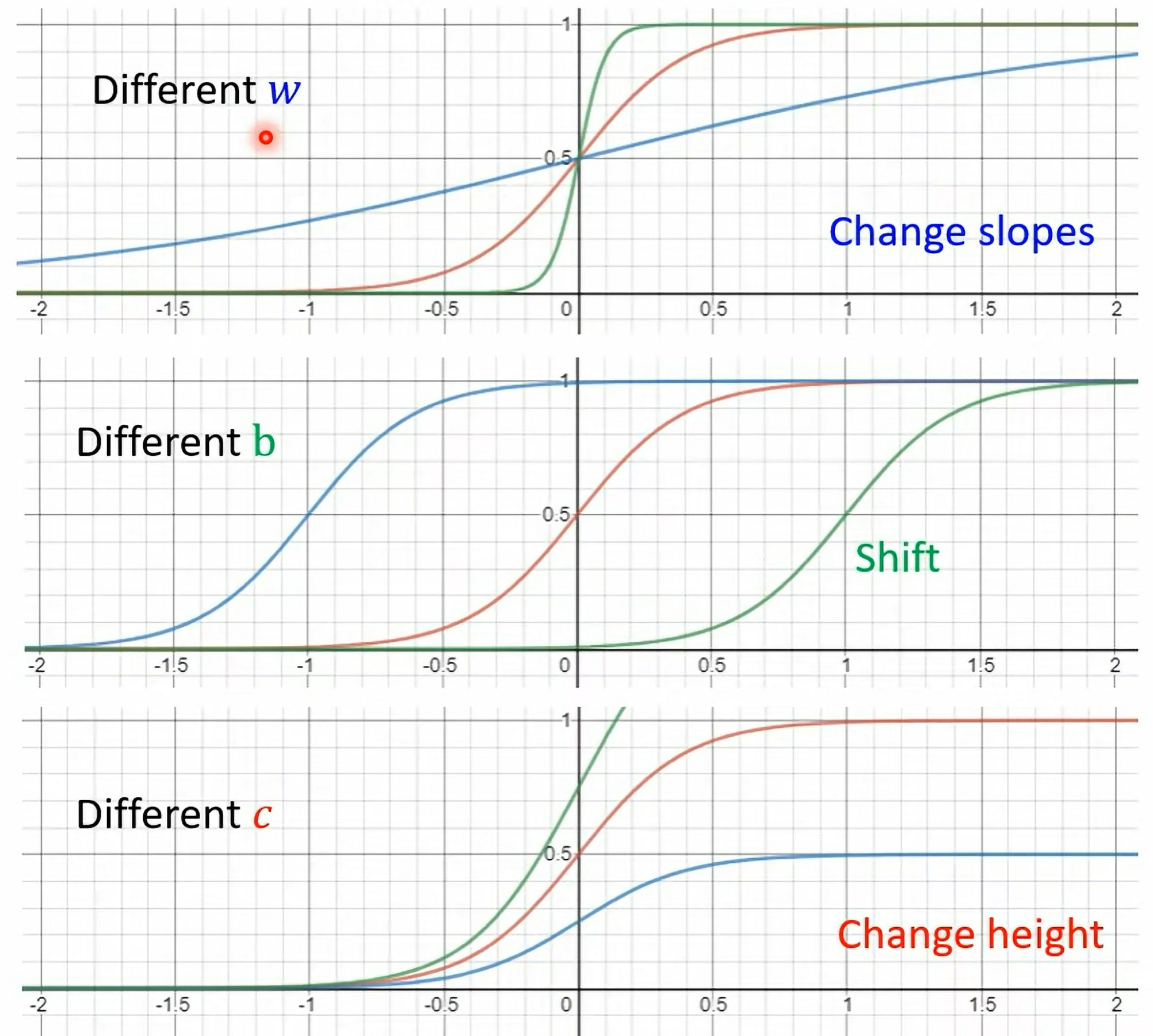
Training Procedure of Machine Learning
Step 1 Function
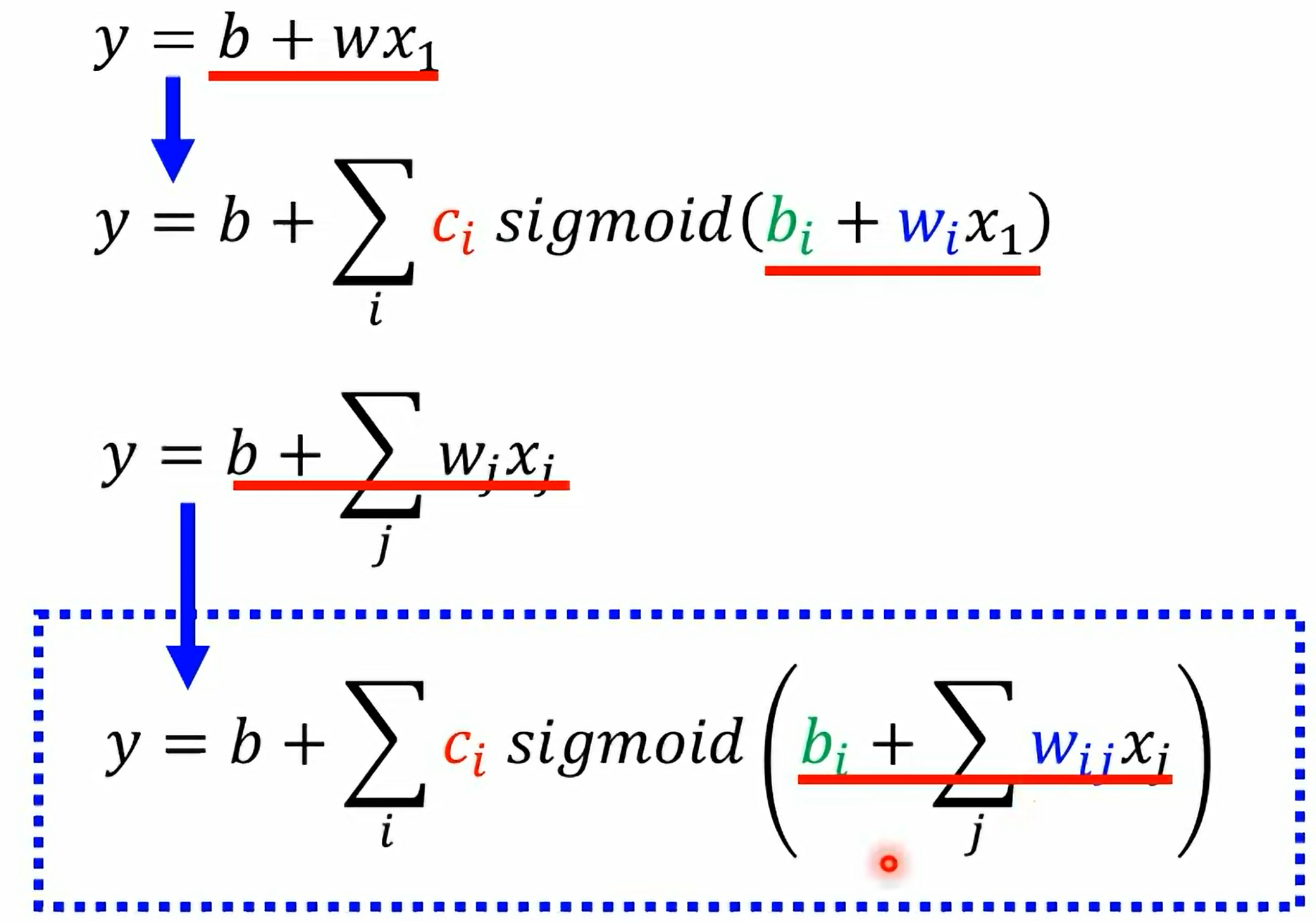
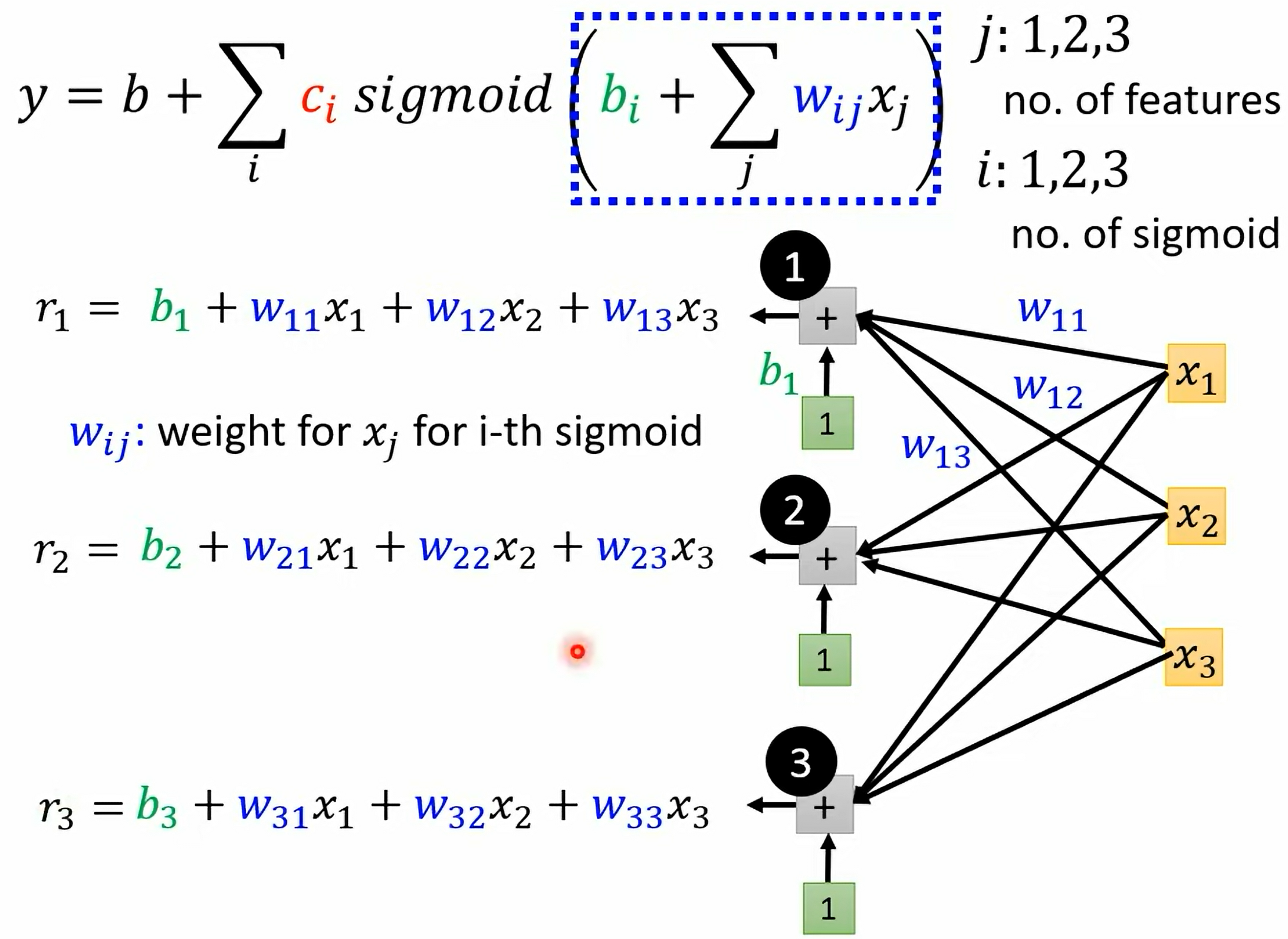
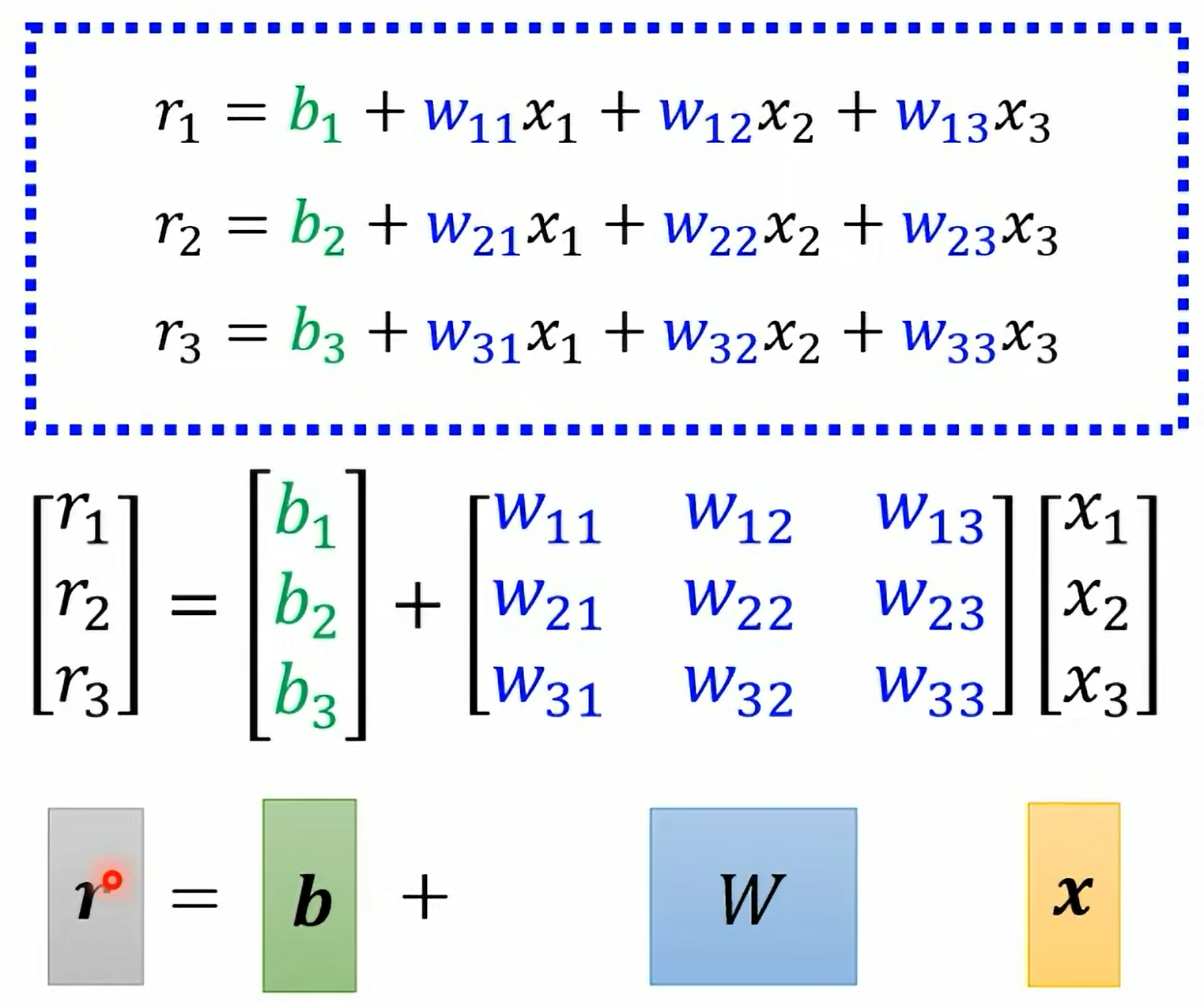
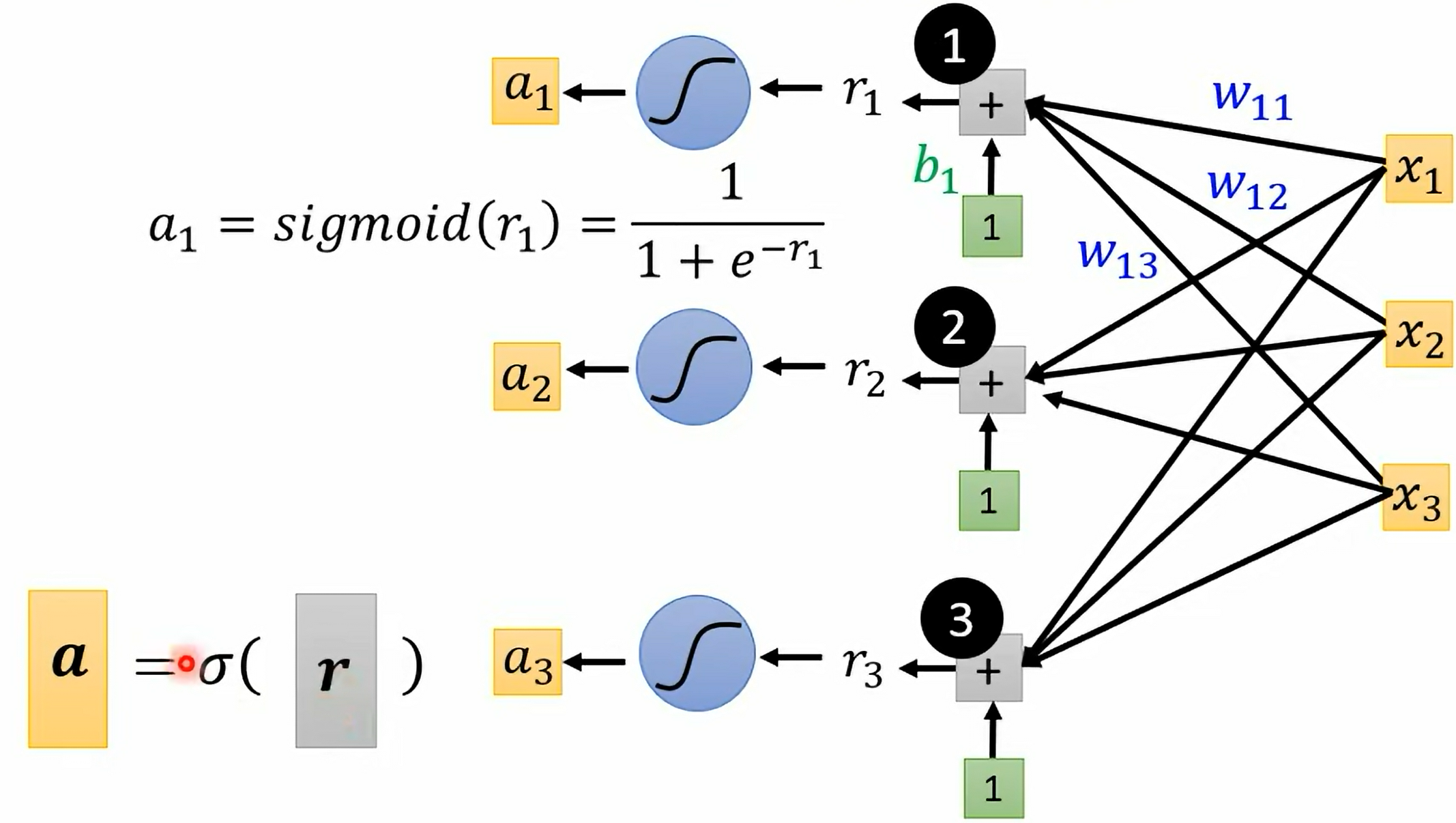
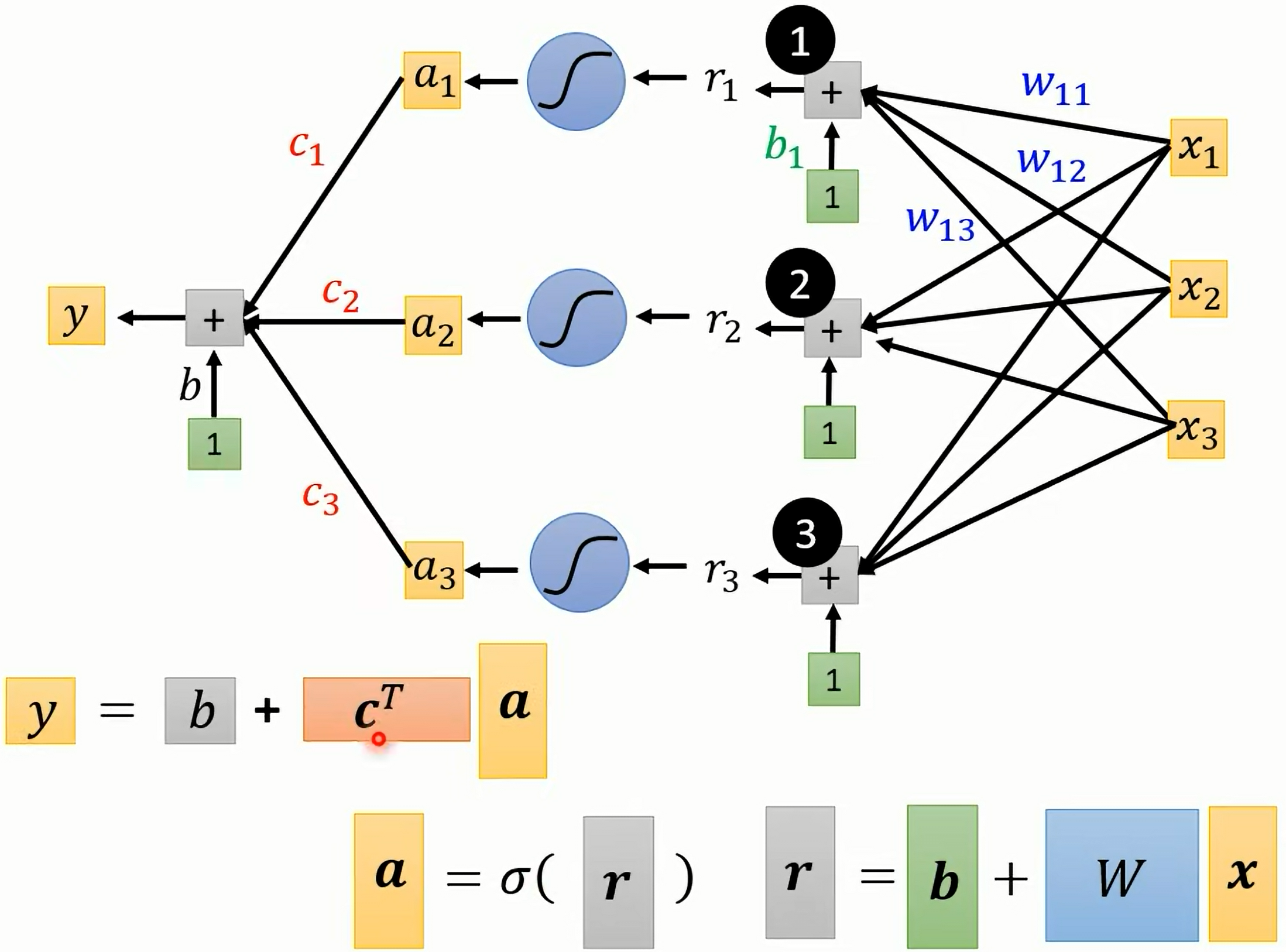
未知参数都统称为 θ \theta θ
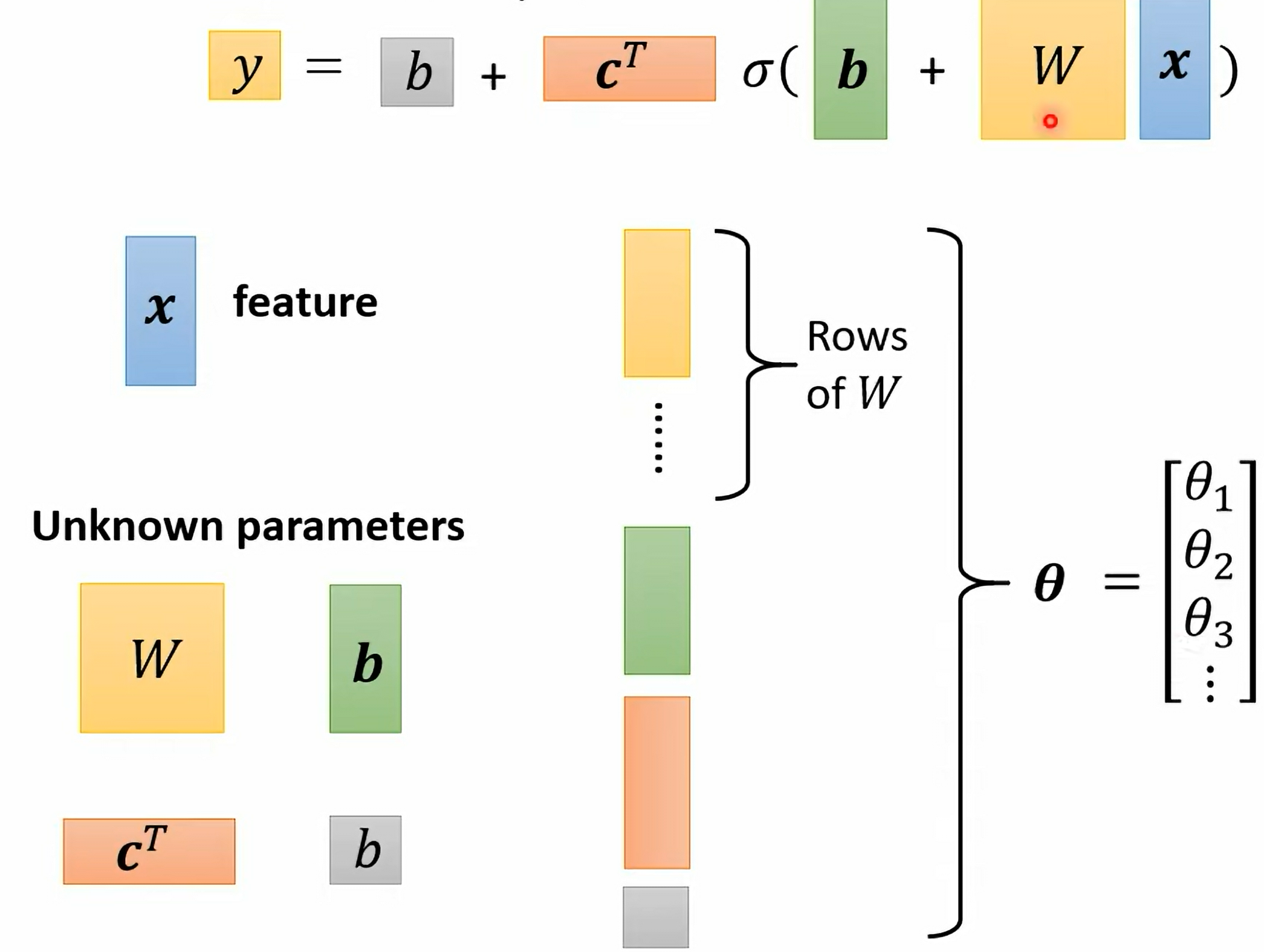
Step 2 Loss
- Loss is a function of parameters L ( θ ) L(\theta) L(θ);
- Loss means how good a set of value is.
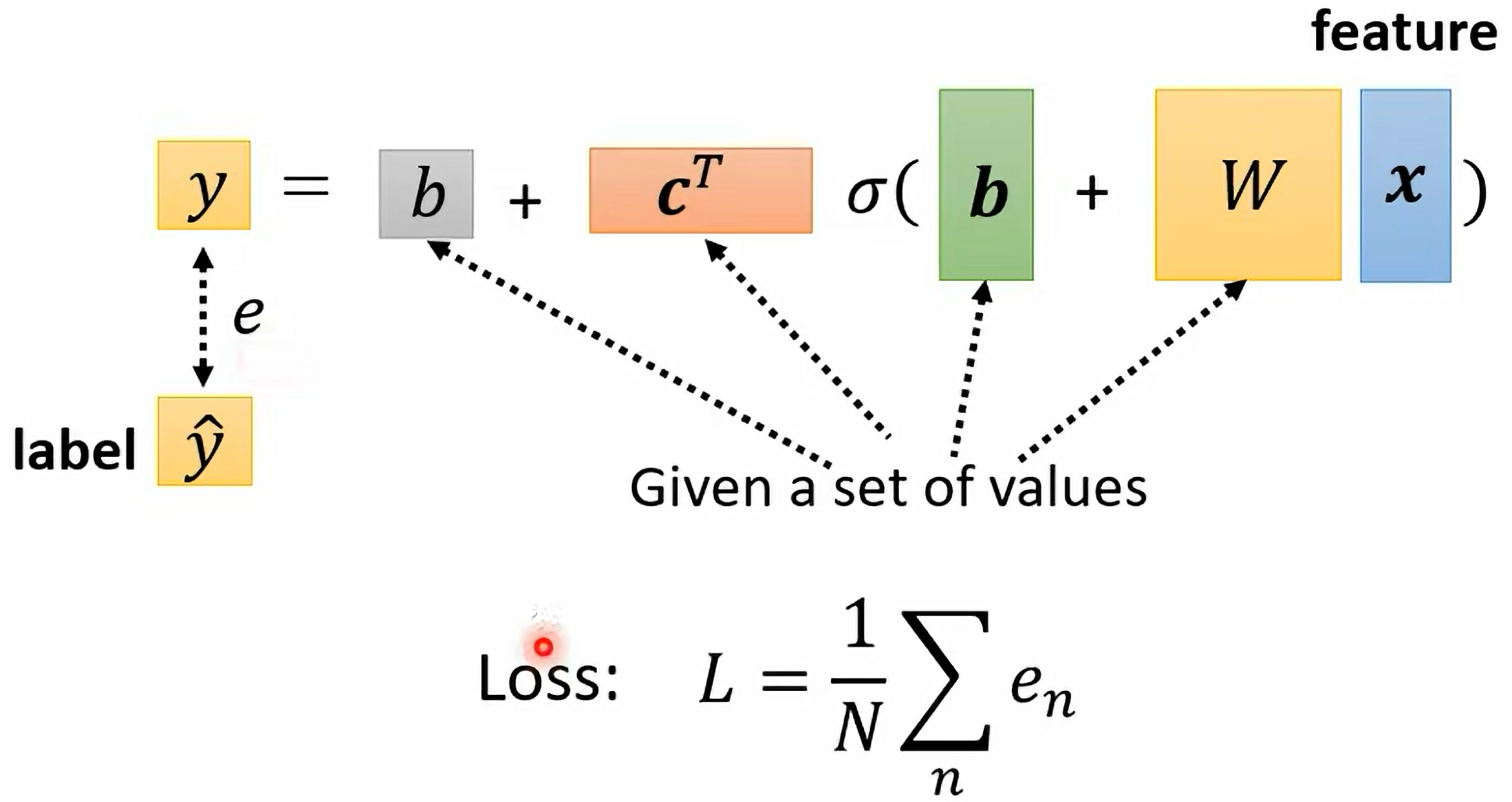
Step 3 Optimization
θ
∗
=
arg
min
θ
L
,
θ
=
[
θ
1
θ
2
θ
3
⋮
]
.
\theta^*=\arg \min_{\theta}L,\ \theta= \begin{bmatrix} \theta_1\\ \theta_2\\ \theta_3\\ \vdots \end{bmatrix}.
θ∗=argθminL, θ=⎣
⎡θ1θ2θ3⋮⎦
⎤.
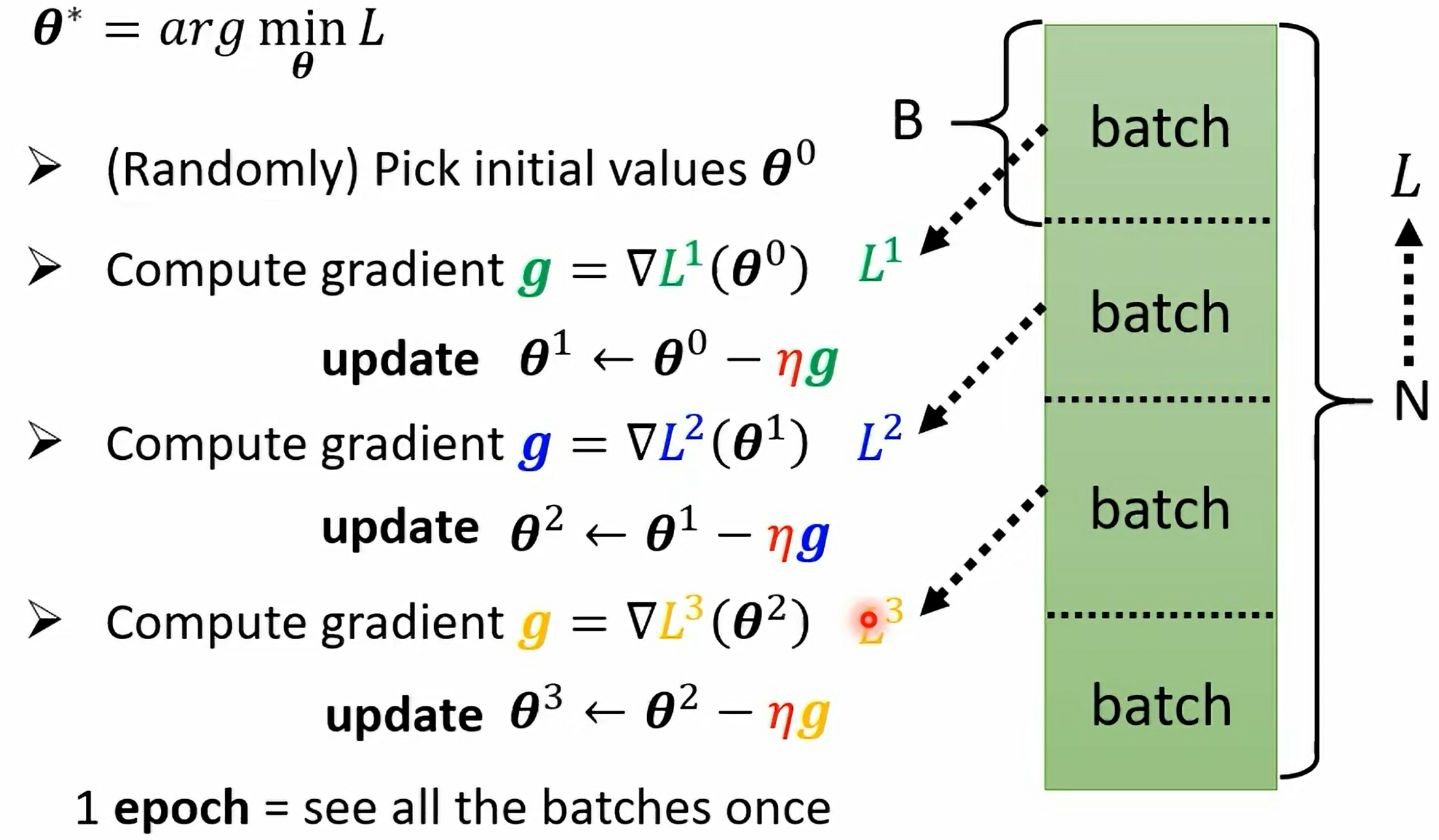
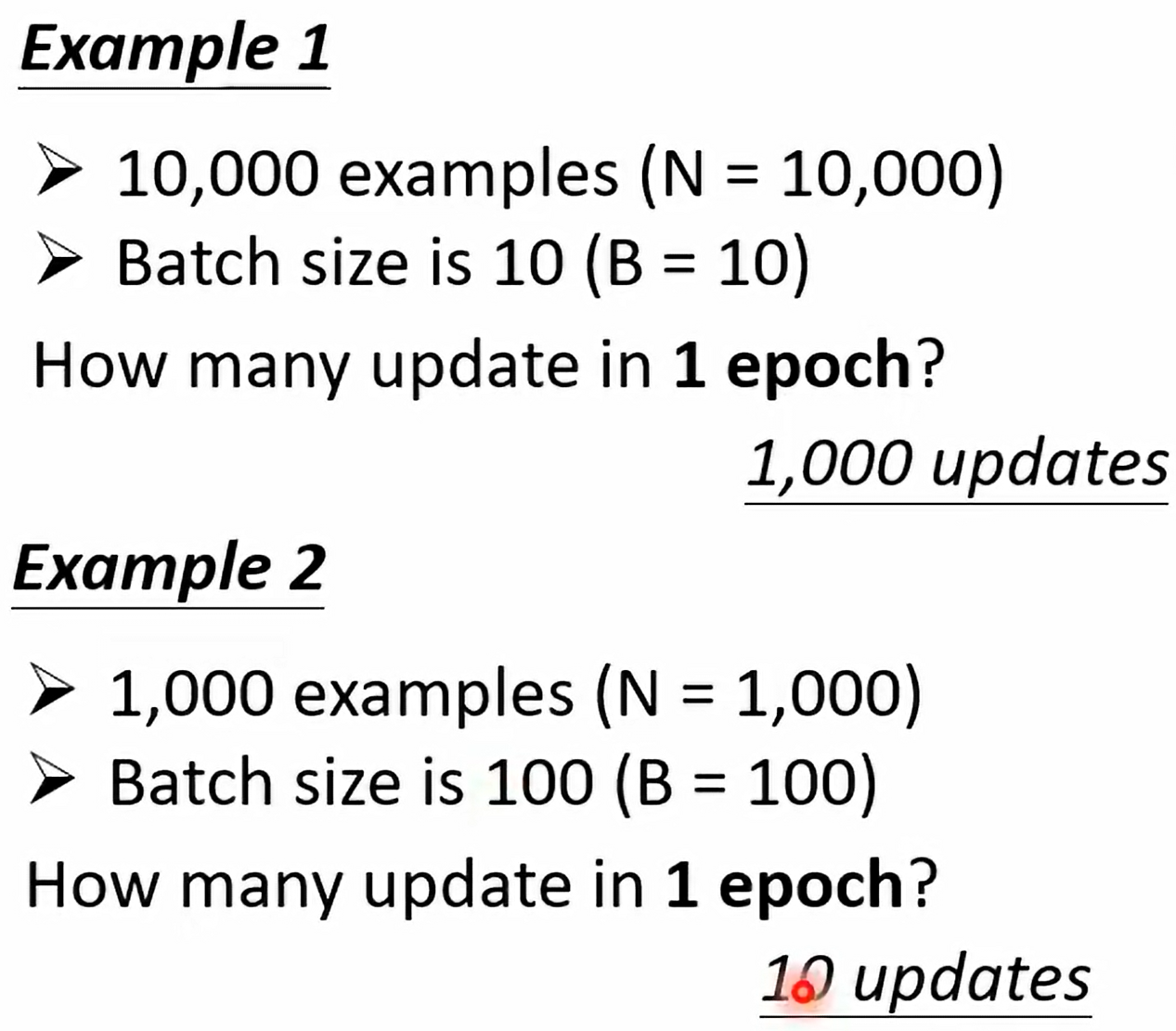
ReLU (Rectified Linear Unit)
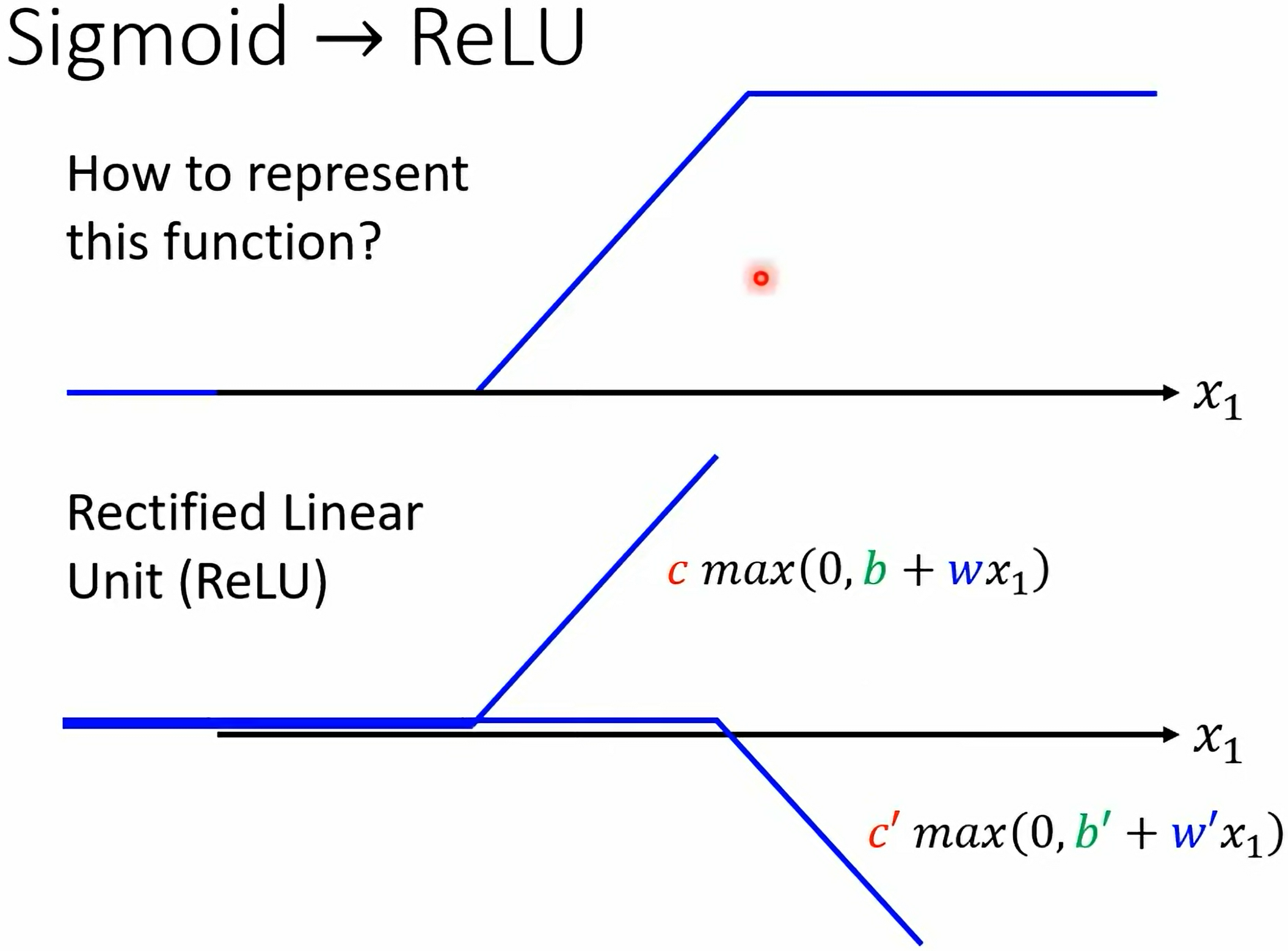
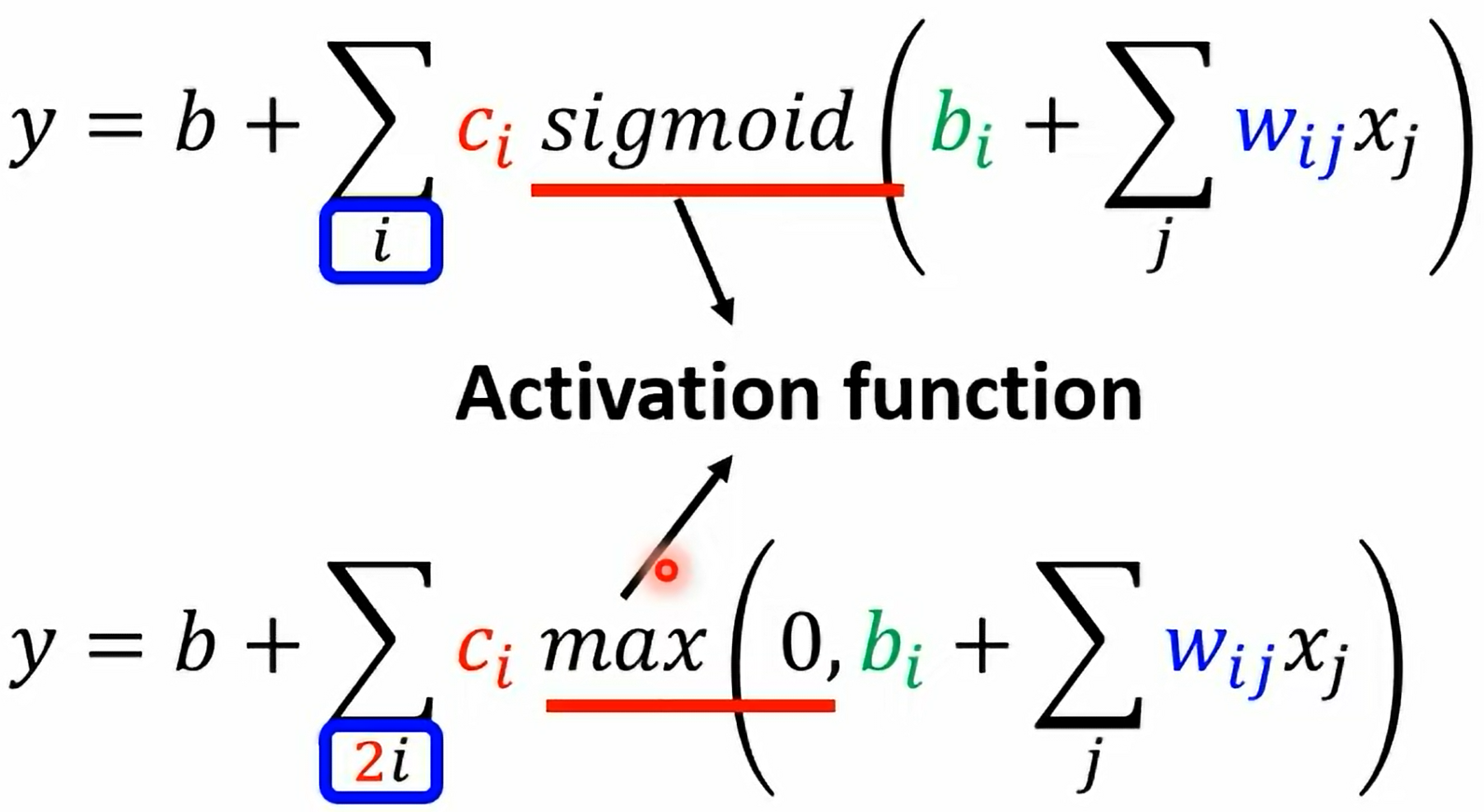
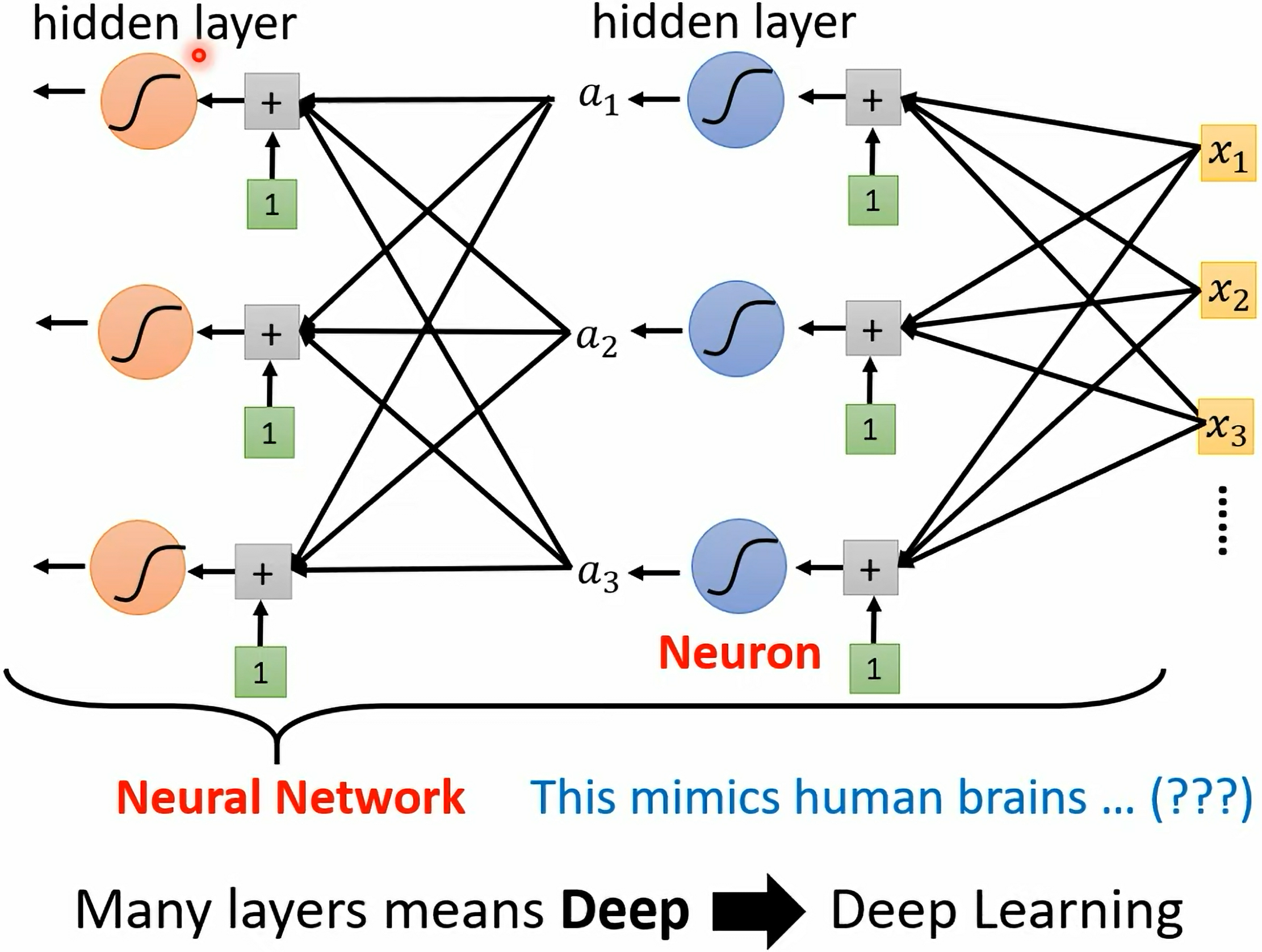
Backpropagation
Gradient Descent
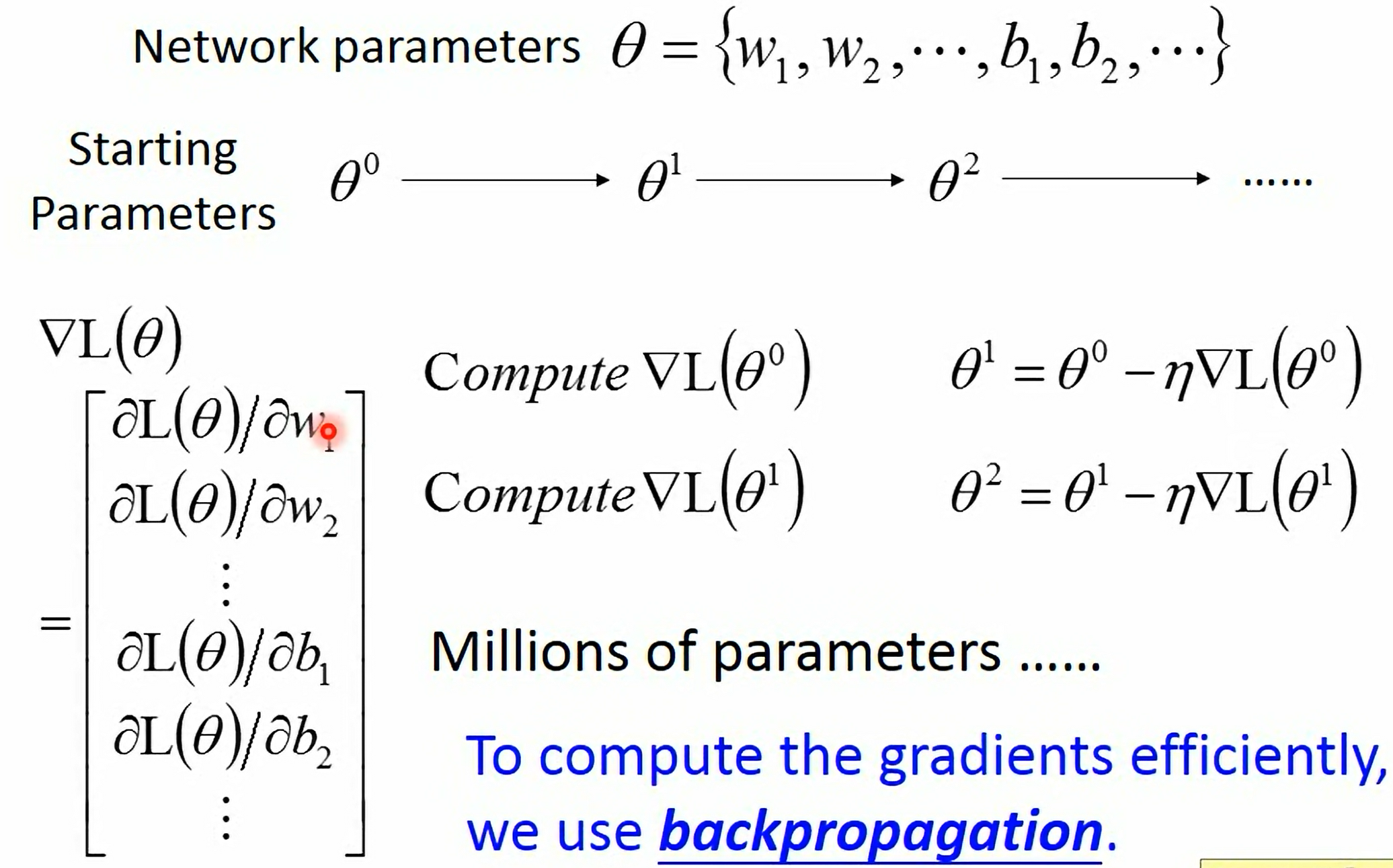
Chain Rule
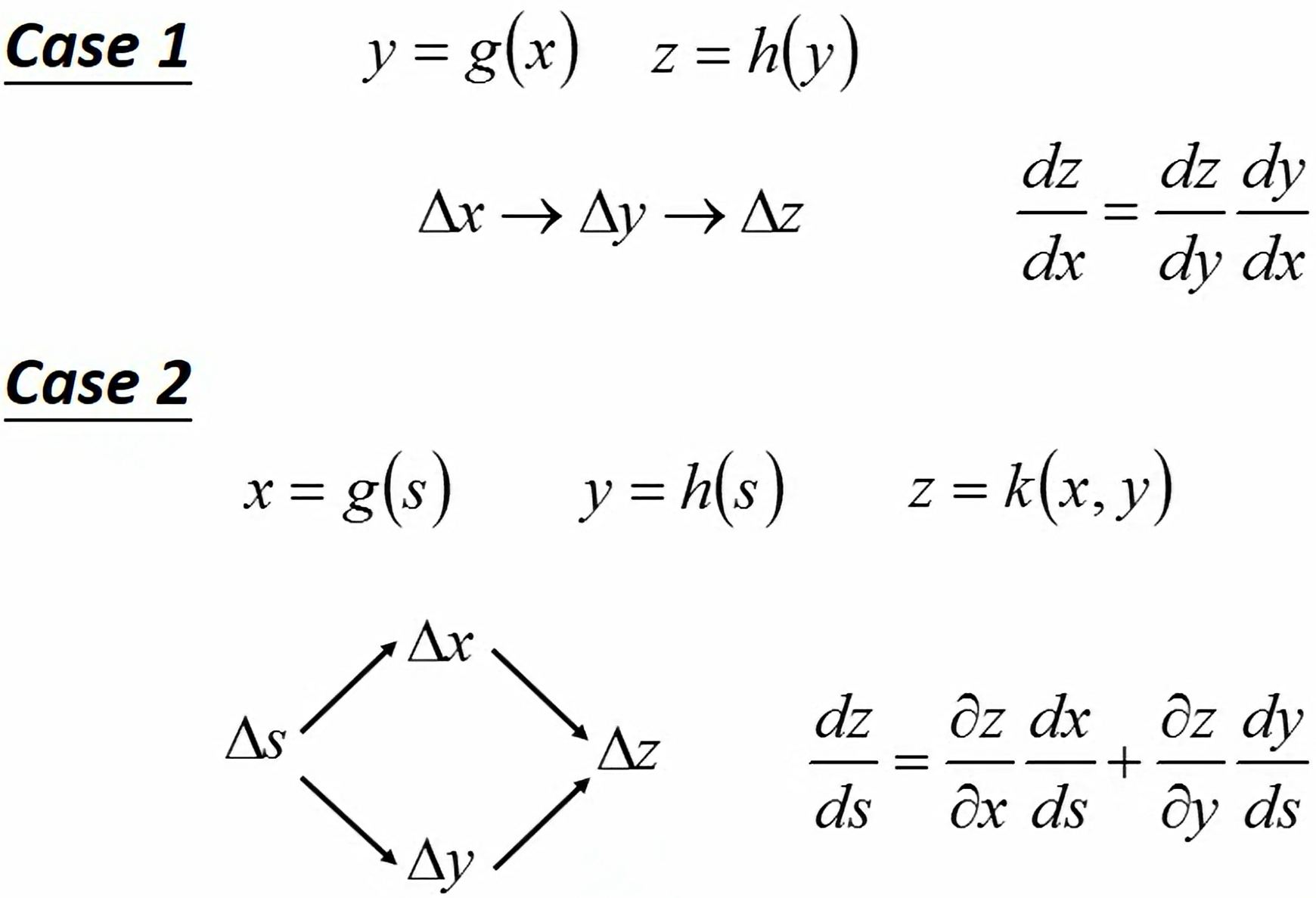
Forward and Backward Pass
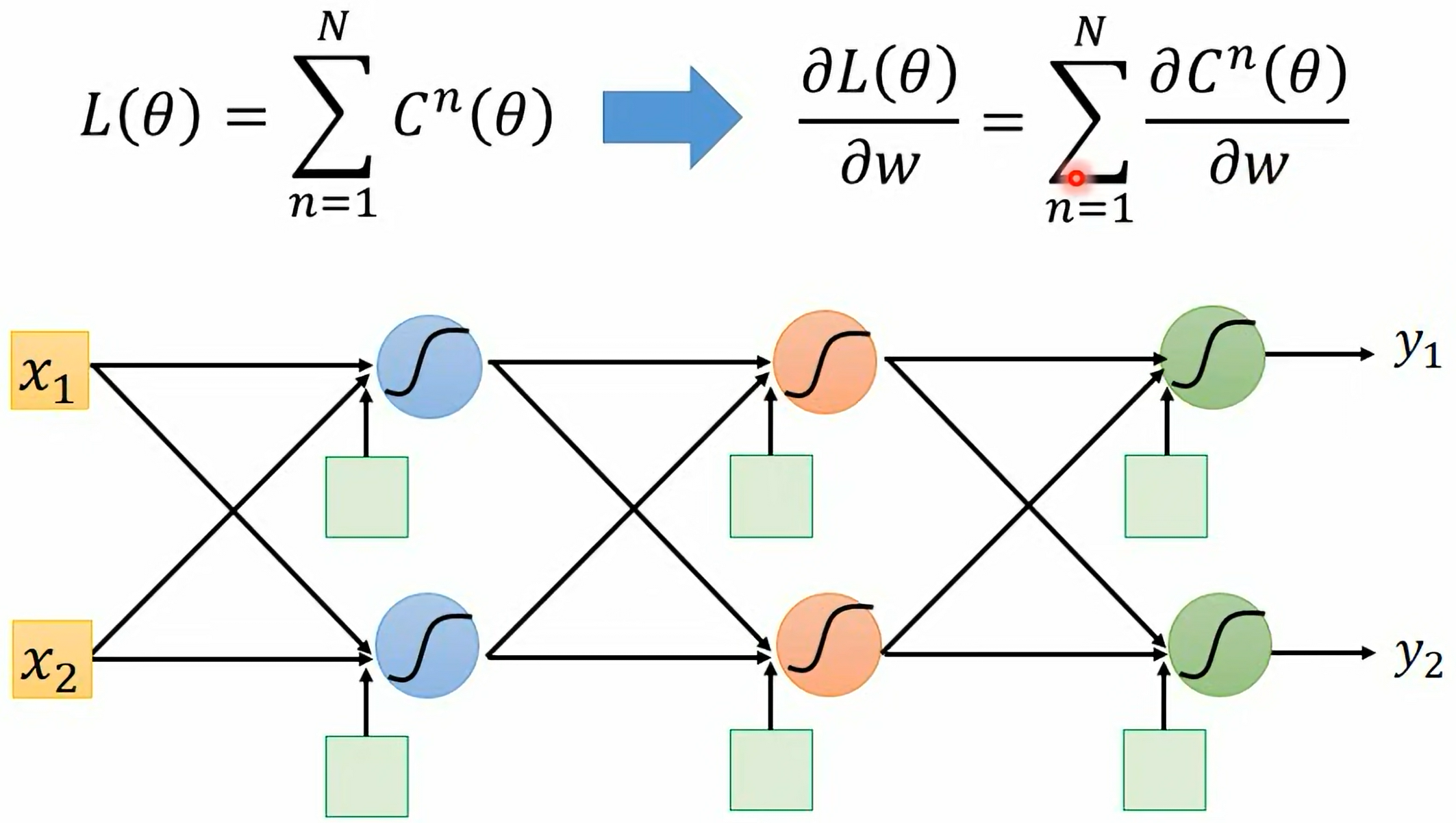
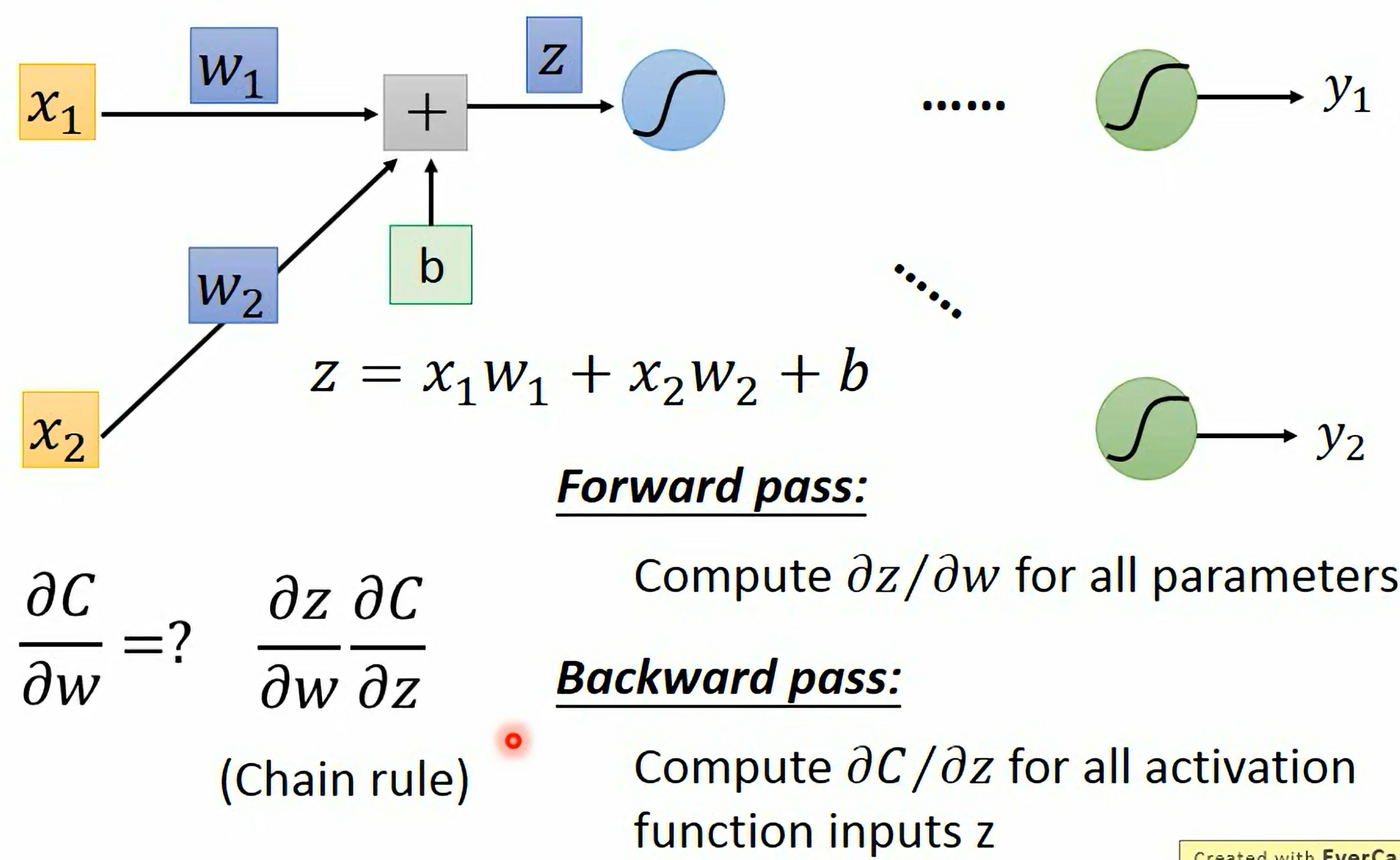
Forward Pass

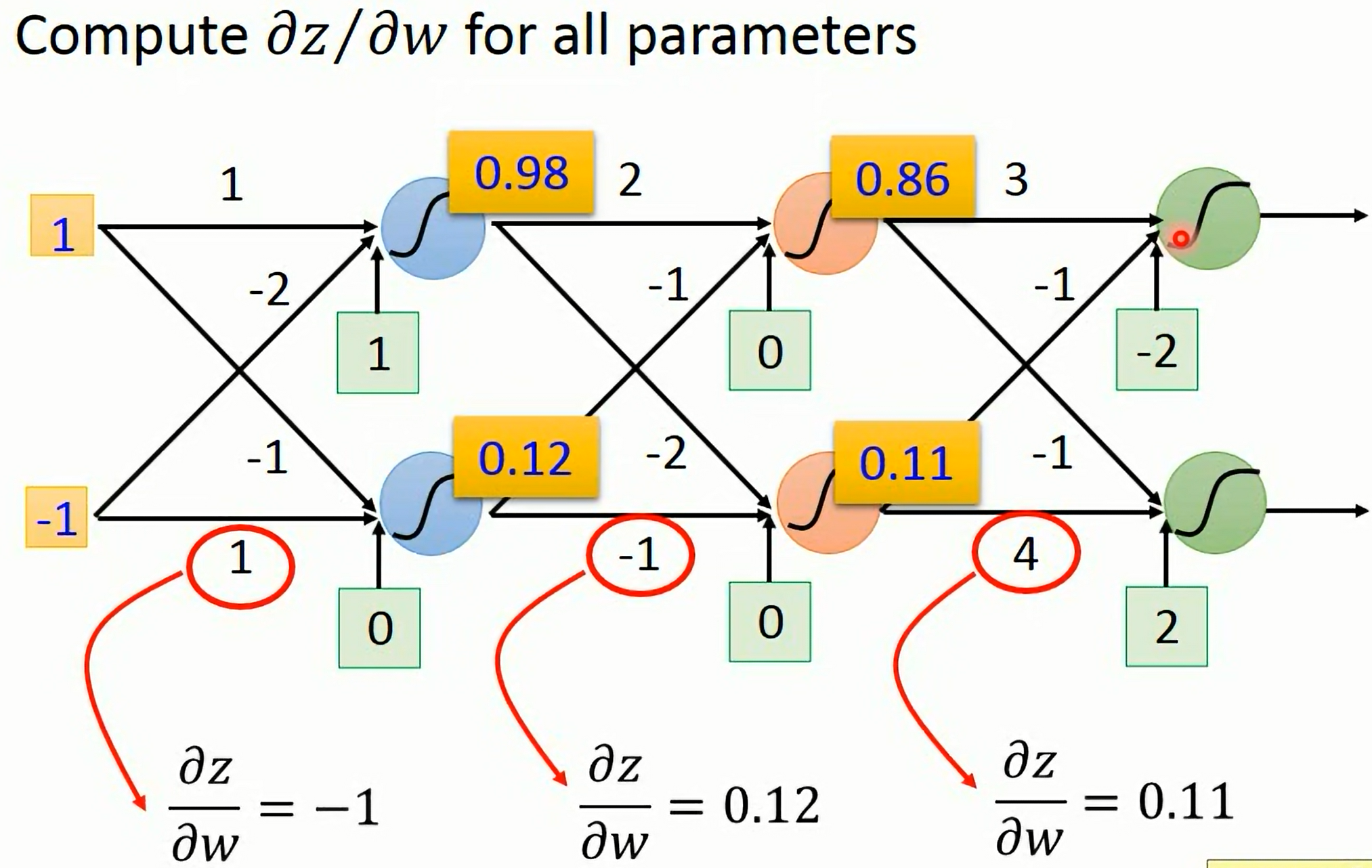
Backward Pass
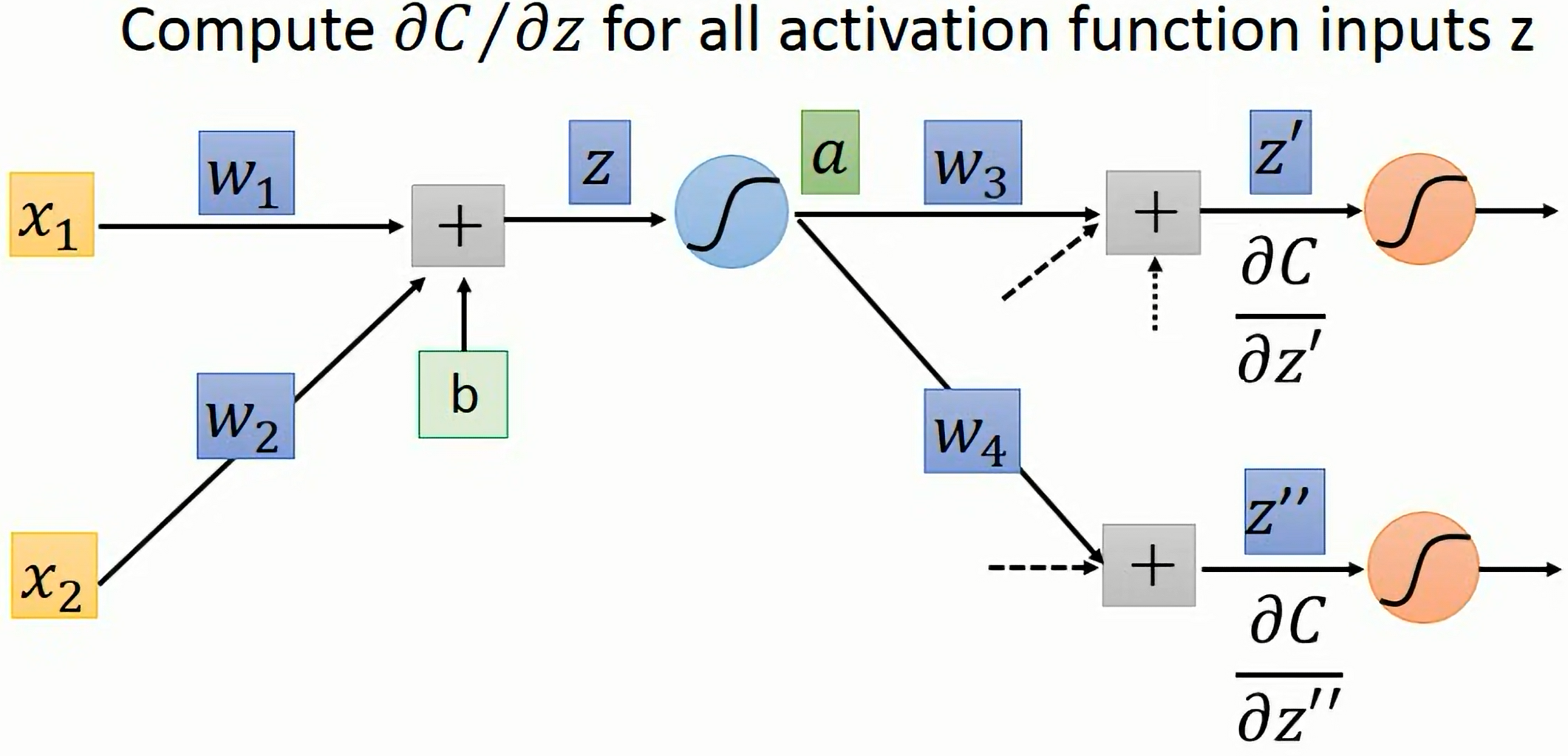
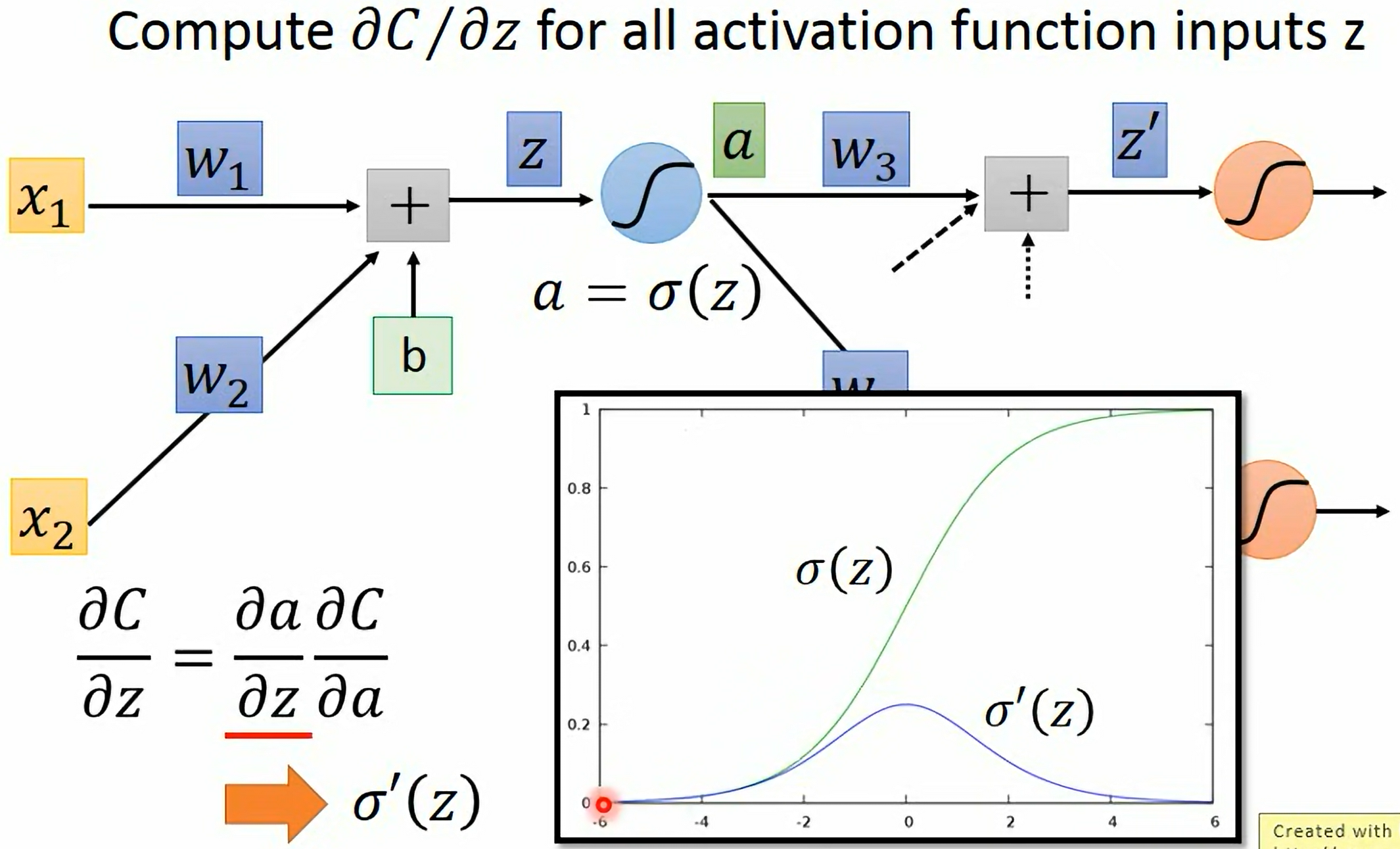
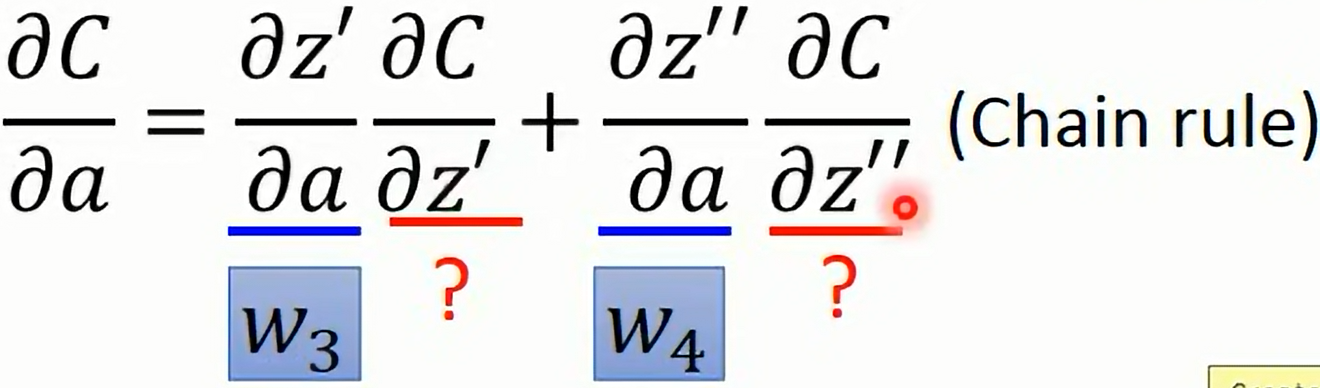
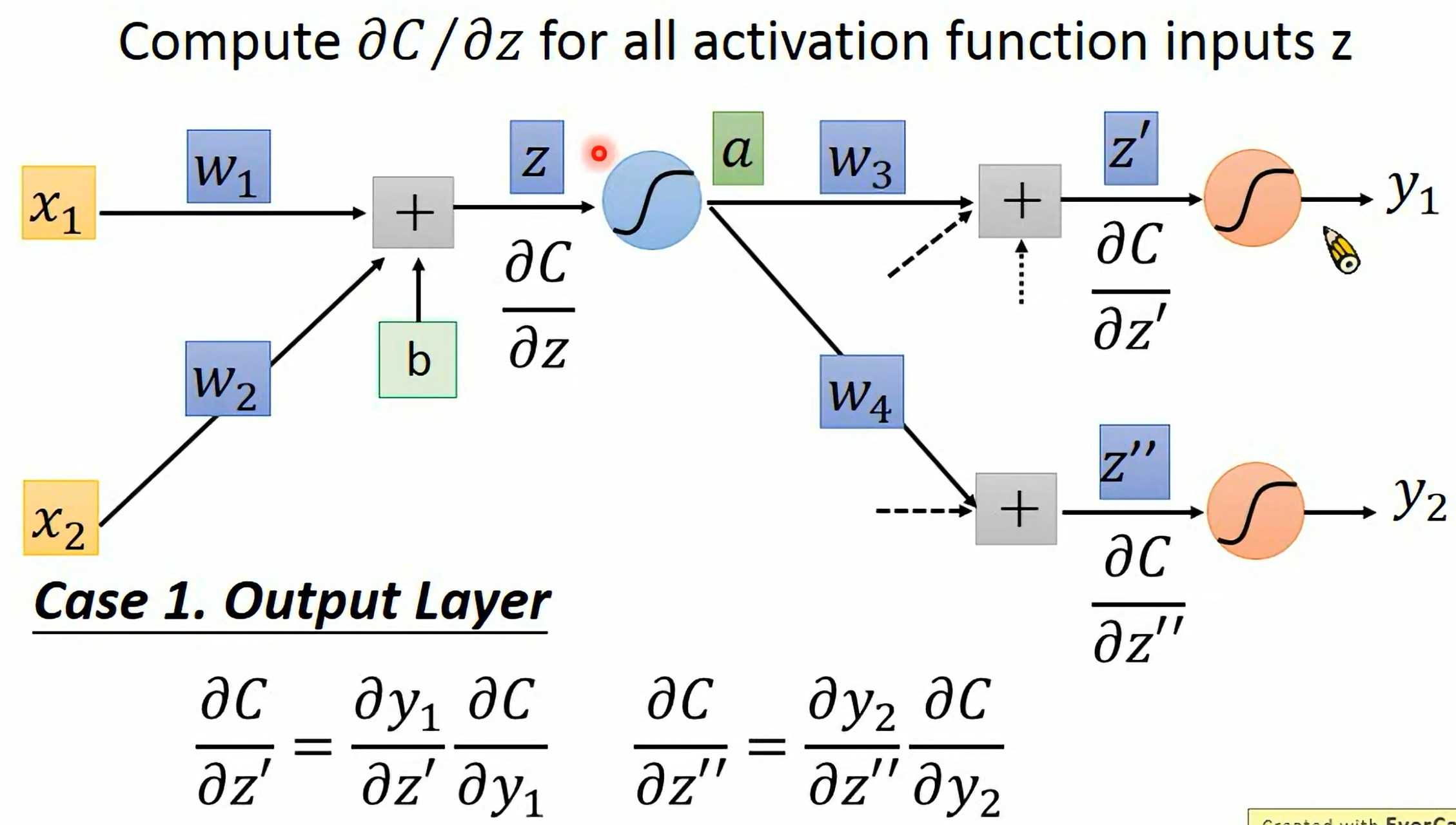
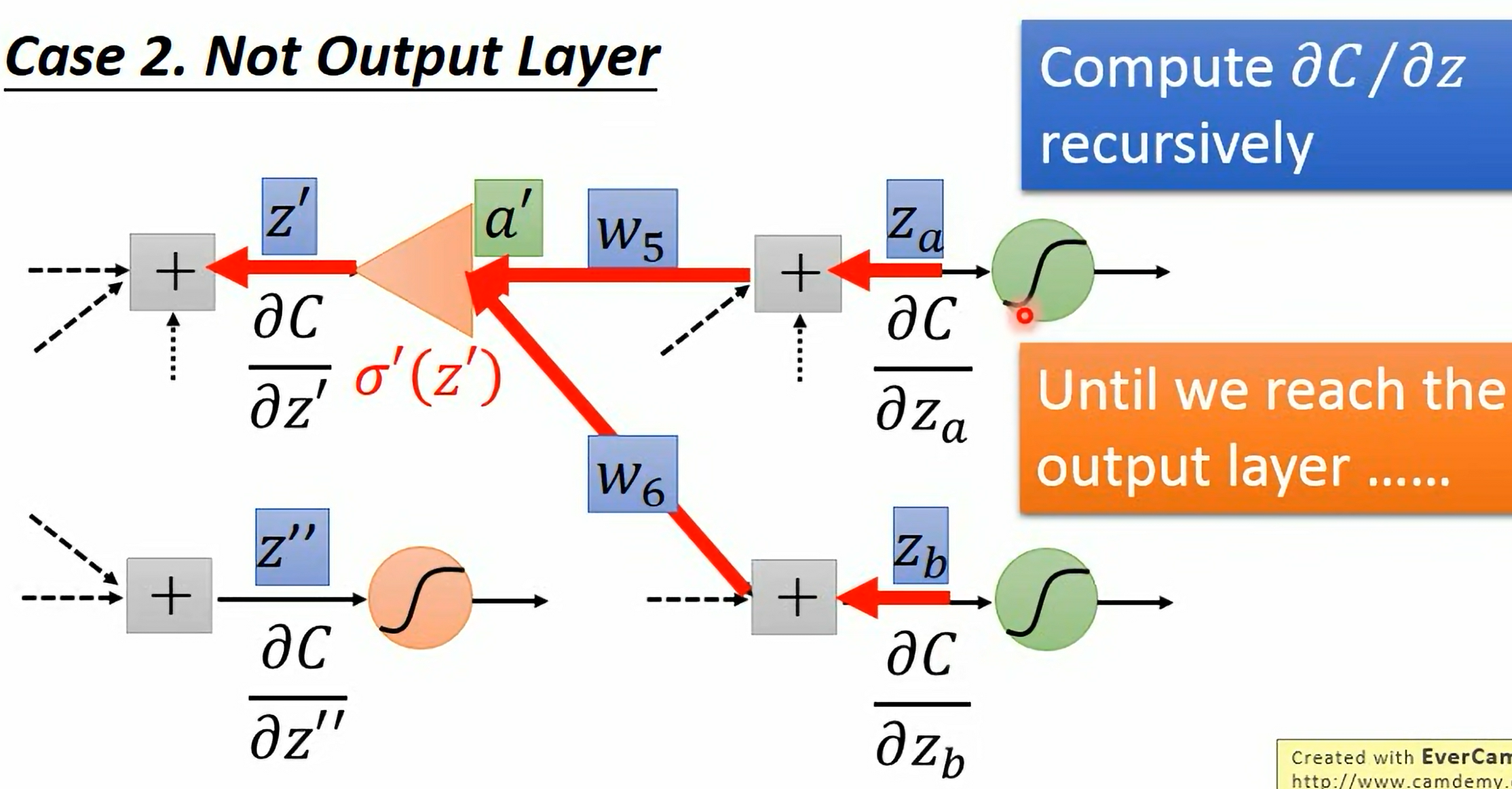
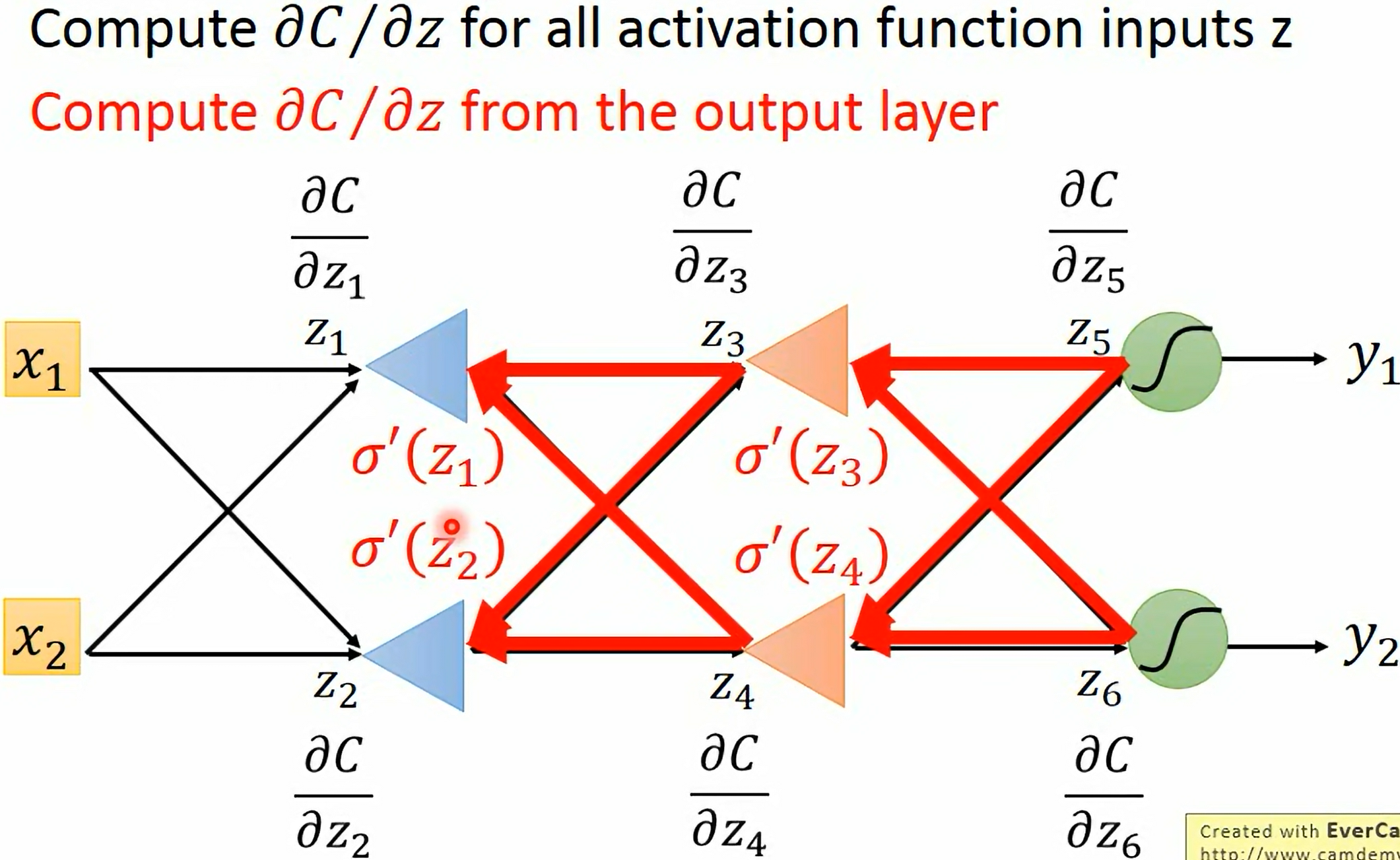
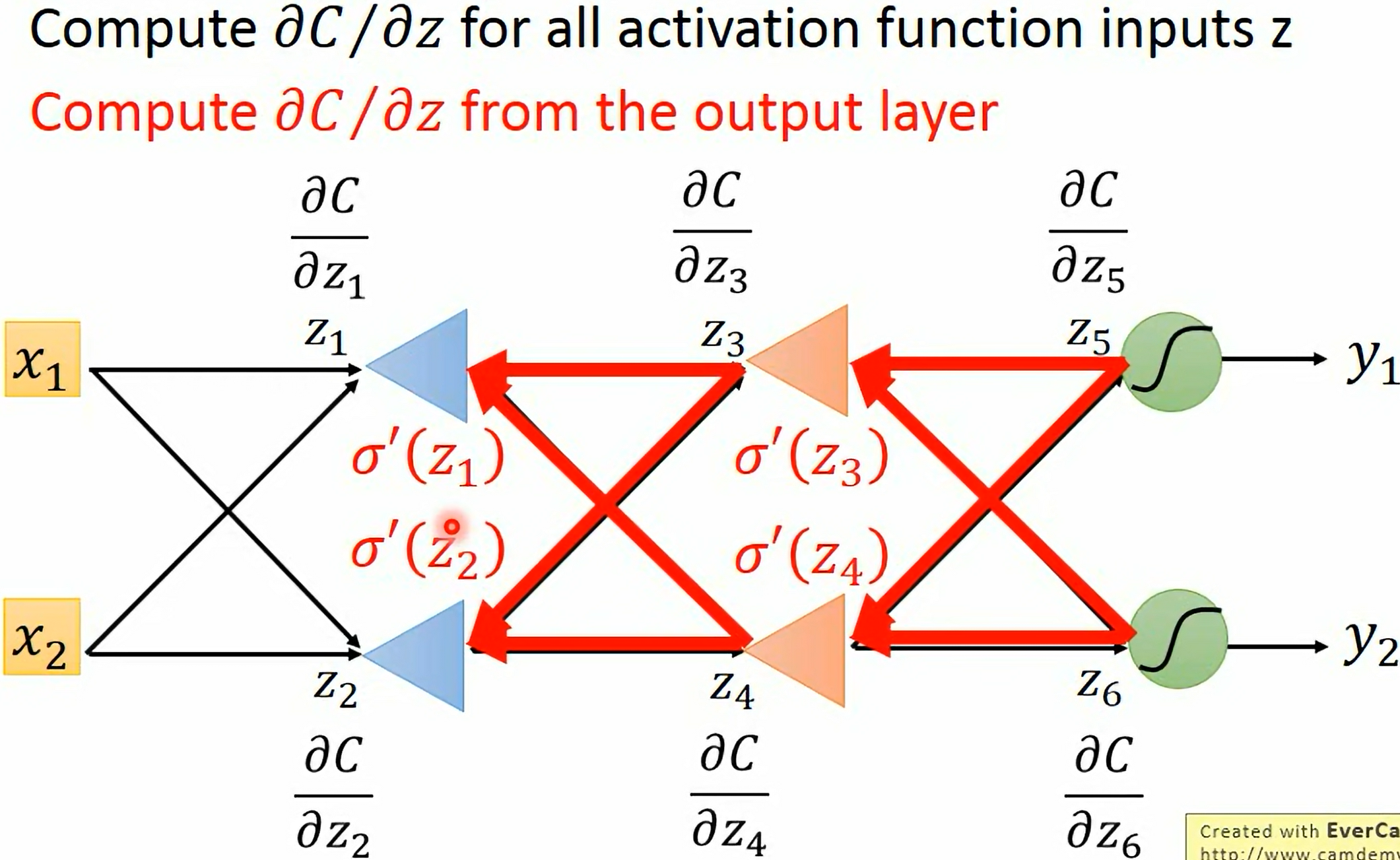
Summary
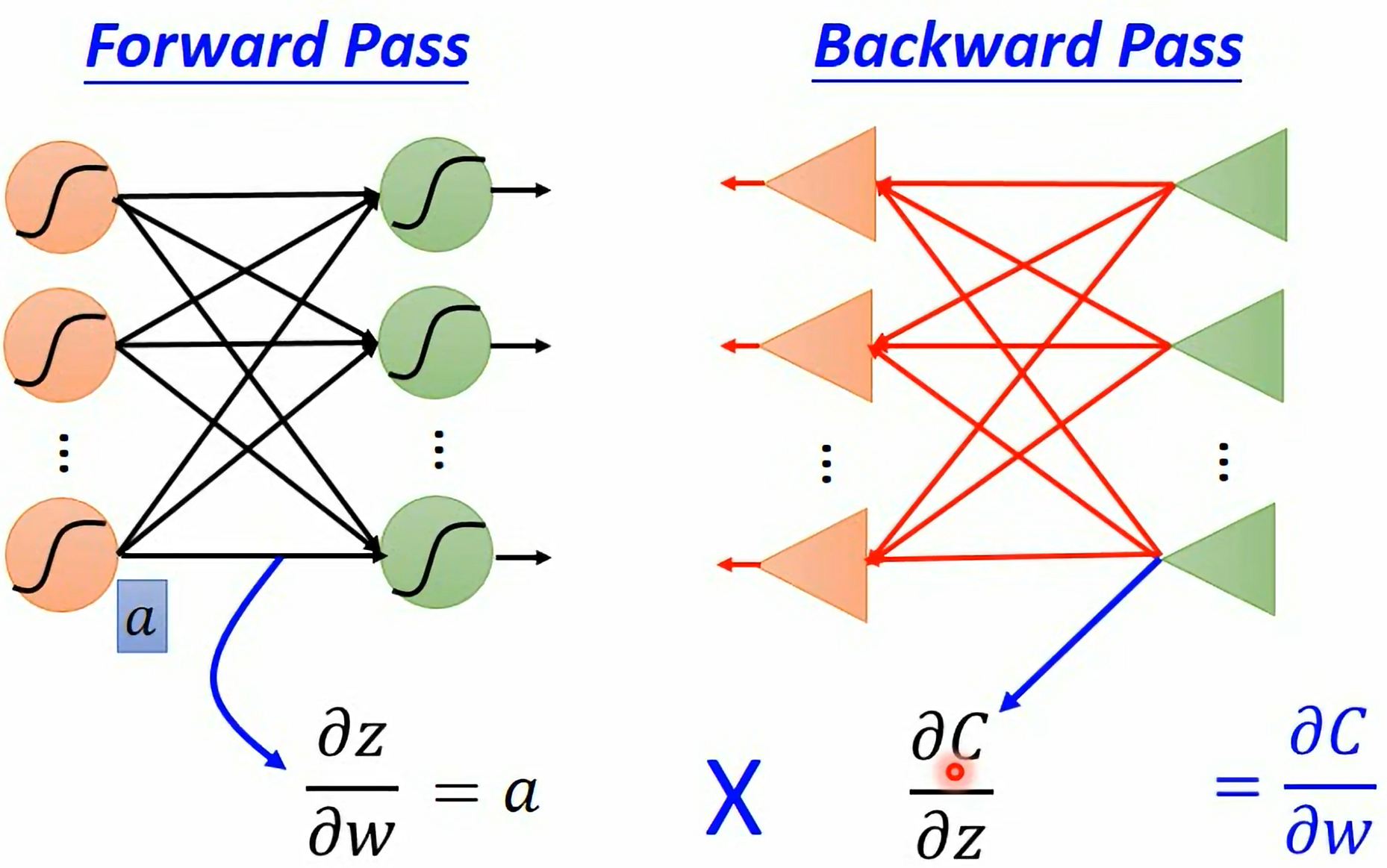