一、pytorch官网中torch.nn的相关简介
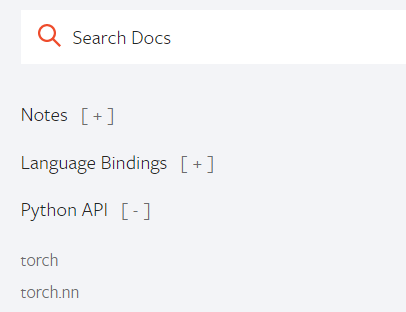
可以看到torch.nn中有许多模块:
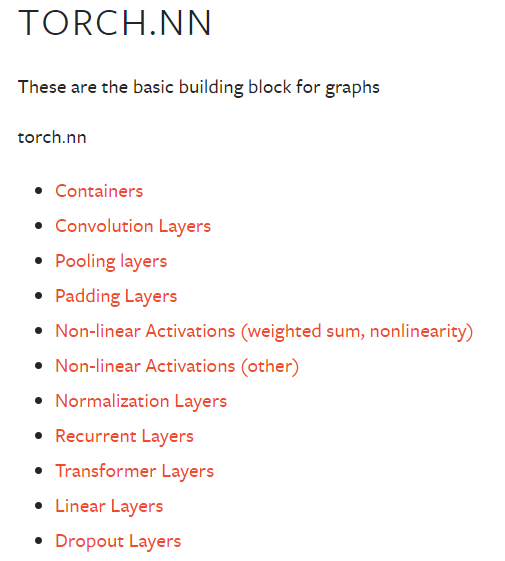
二、Containers模块
1、MODULE(CLASS : torch.nn.Module)
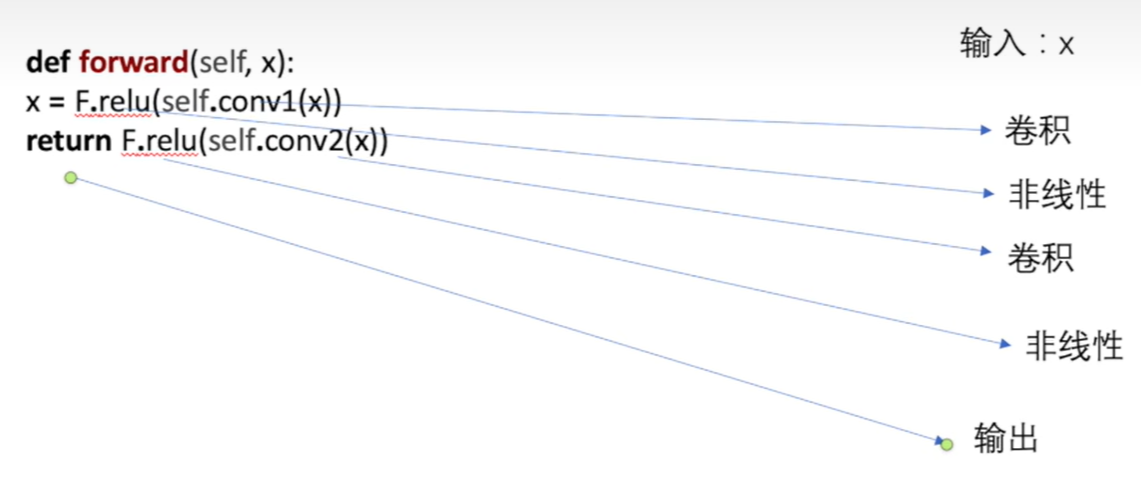
import torch.nn as nn
import torch.nn.functional as F
class Model(nn.Module):#nn.Module---所有神经网络模块的基类。
def __init__(self): #初始化
super(Model, self).__init__()
self.conv1 = nn.Conv2d(1, 20, 5)
self.conv2 = nn.Conv2d(20, 20, 5)
def forward(self, x): #前向计算
x = F.relu(self.conv1(x))
return F.relu(self.conv2(x))
forward(*input)
Defines the computation performed at every call. Should be overridden by all subclasses.
2、搭建神经网络模型
import torch
import torch.nn as nn
import torch.nn.functional as F
# 定义自己的神经网络模板
class Lemon(nn.Module):
def __init__(self) -> None:
super().__init__()
def forward(self,input):
output = input + 1
return output
# 创建神经网络
lemon = Lemon()
x = torch.tensor(1.0)
output = lemon(x)
print(output)
三、Convolution Layers 卷积层
nn.Conv1d/nnCon2d
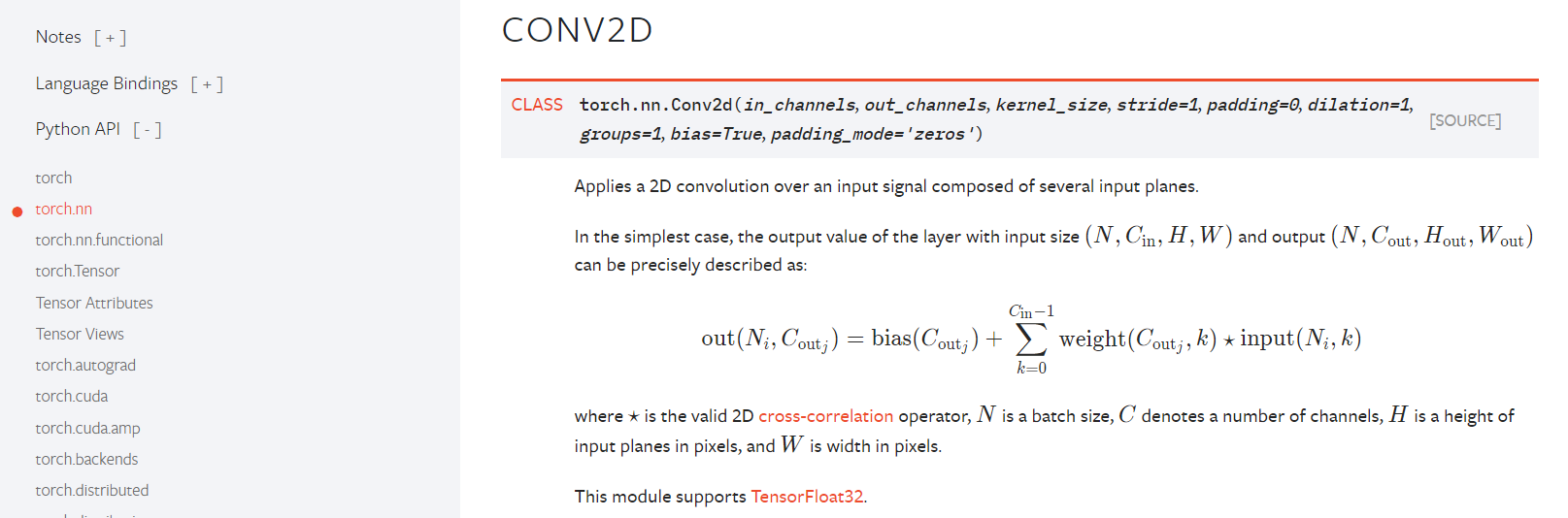
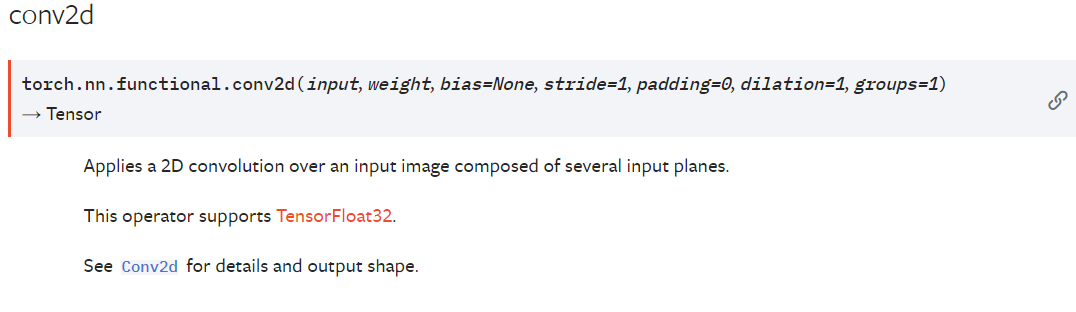
input – input tensor of shape (minibatch,in_channels,iH,iW)输入
weight – filters of shape (out_channels,groupsin_channels,kH,kW)权重/卷积核
bias – optional bias tensor of shape (out_channels). Default: None偏置
stride – the stride of the convolving kernel. Can be a single number or a tuple (sH, sW). Default: 1步进/长 SH和SW分别控制横向的步进和纵向的步进
padding – implicit paddings on both sides of the input. Can be a single number or a tuple (padH, padW). Default: 0
dilation – the spacing between kernel elements. Can be a single number or a tuple (dH, dW). Default: 1
groups – split input into groups, in_channelsin_channels should be divisible by the number of groups. Default: 1
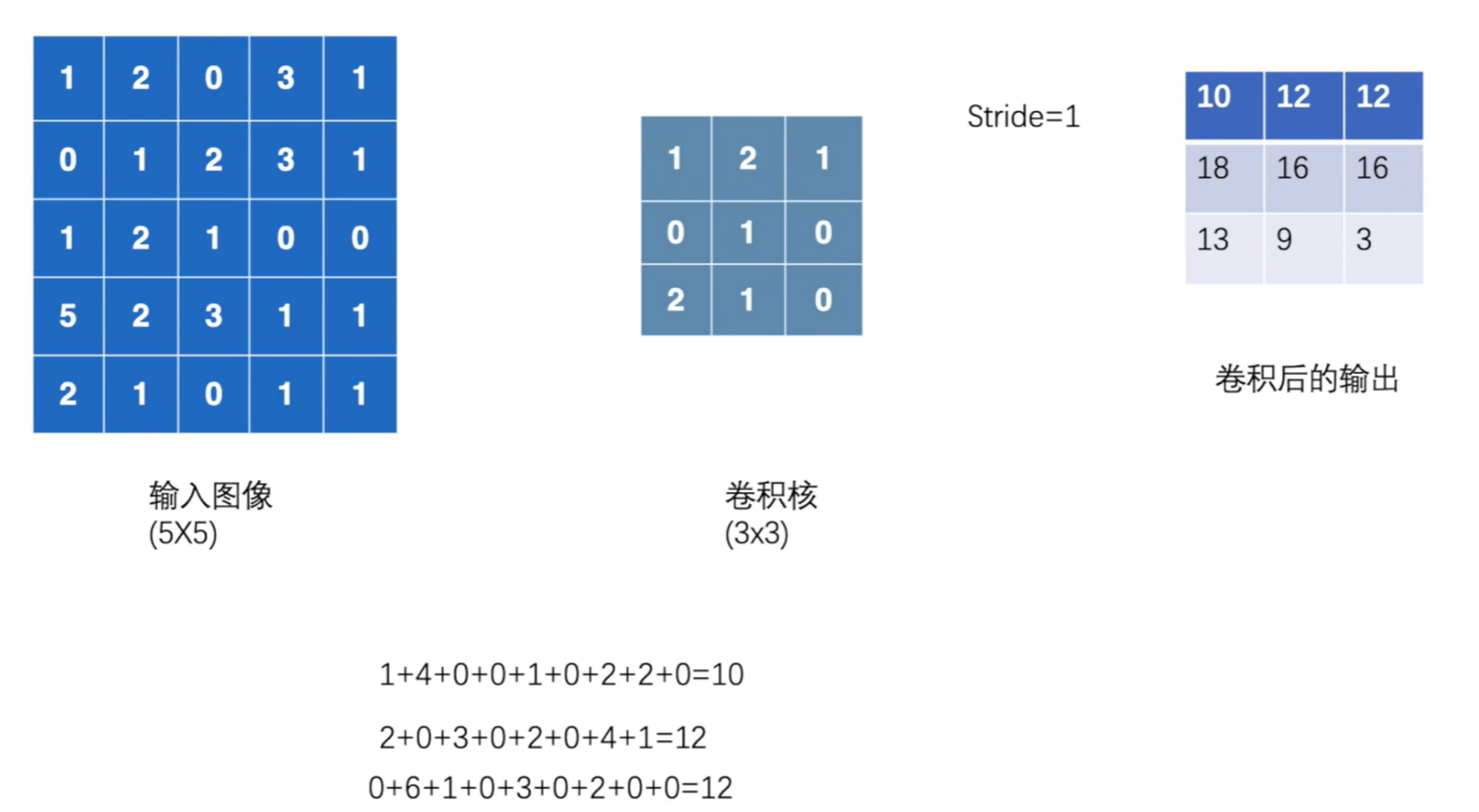
import torch
import torch.nn.functional as F
# 输入
input = torch.tensor([[1,2,0,3,1],
[0,1,2,3,1],
[1,2,1,0,0],
[5,2,3,1,1],
[2,1,0,1,1]])
# 卷积核
kernel = torch.tensor([[1,2,1],
[0,1,0],
[2,1,0]])
print(input.shape) #torch.Size([5, 5])
print(kernel.shape) #torch.Size([3, 3])
#官方文档中输入input和卷积核weight需要四个参数——>input tensor of shape (minibatch,in_channels,iH,iW)
#所以可以使用reshape二参变四参
input = torch.reshape(input,(1,1,5,5)) #torch.Size([1, 1, 5, 5])
kernel = torch.reshape(kernel,(1,1,3,3)) #torch.Size([1, 1, 3, 3])
print(input.shape) #torch.Size([5, 5])
print(kernel.shape) #torch.Size([3, 3])
output = F.conv2d(input,kernel,stride=1)
print(output)
一般来讲,输出的维度 = 输入的维度 - 卷积核大小/stride + 1
padding =1,为上下左右各填充一行,空的地方默认为0
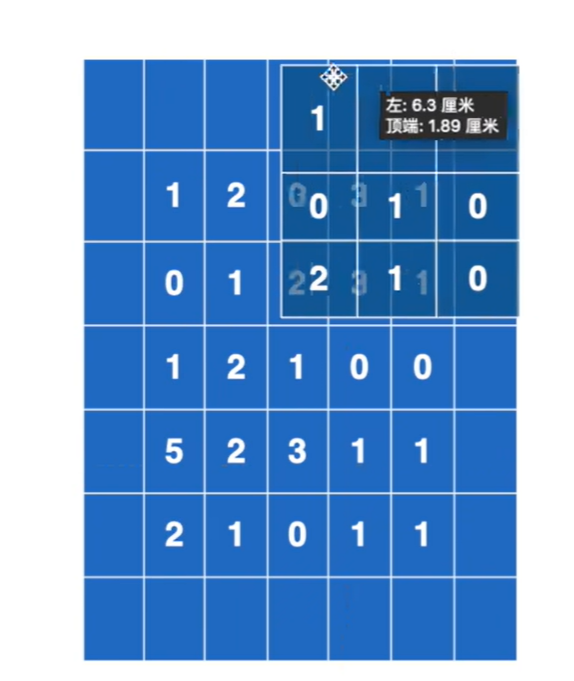
torch.nn.Conv2d(in_channels, out_channels, kernel_size, stride=1, padding=0, dilation=1, groups=1, bias=True, padding_mode='zeros')
bias:偏置,卷积后的结果是否加减一个常数
padding_mode:填充模式
in_channels (int) – Number of channels in the input image # 输入图像的通道数,彩色一般是三通道
out_channels (int) – Number of channels produced by the convolution# 输出的通道数
kernel_size (int or tuple) – Size of the convolving kernel #定义卷积核大小 3---3*3 (1,2) #卷积核不需要自己给定,是在训练过程中不断完善的。
in_channel和out_channel的实际解释:
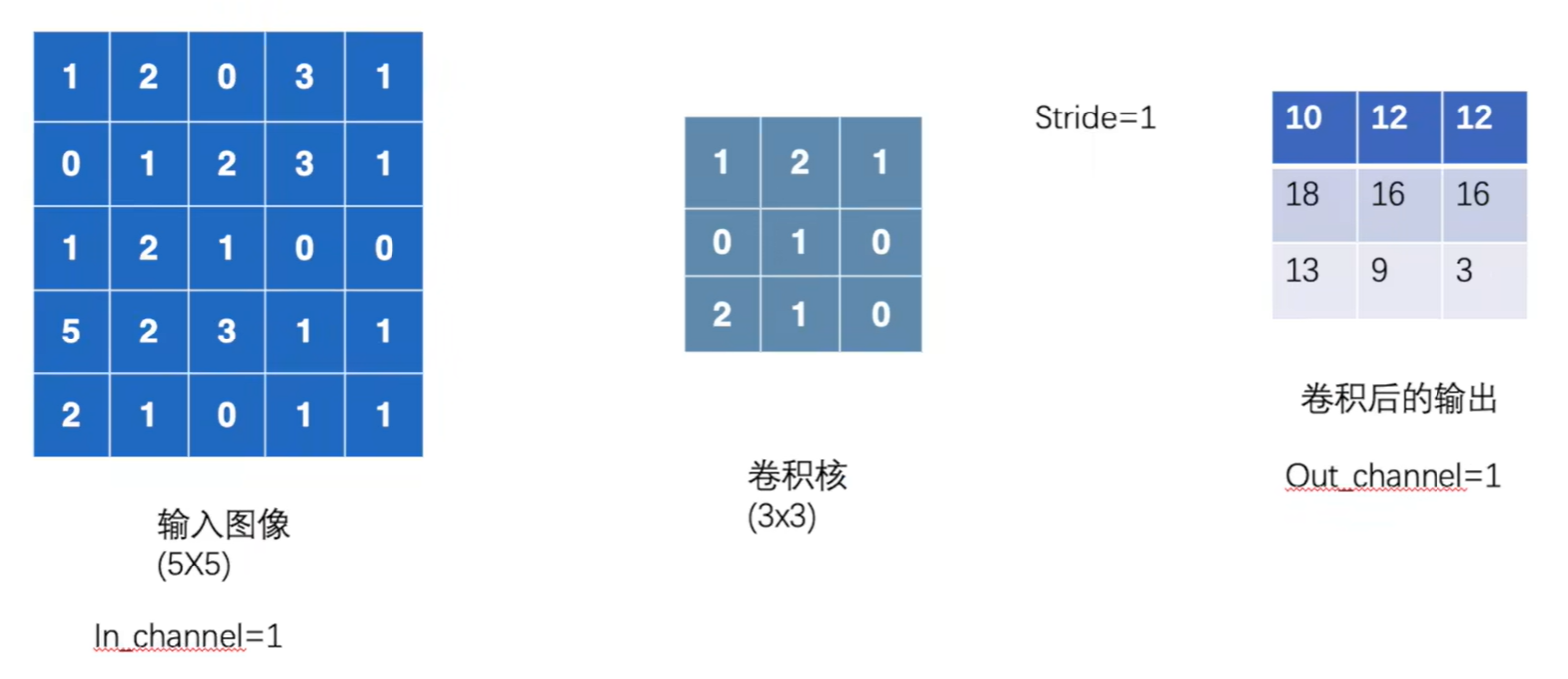
若outchannel=2时,卷积层就会生成两个卷积核【输出通道数就是卷积核的个数】
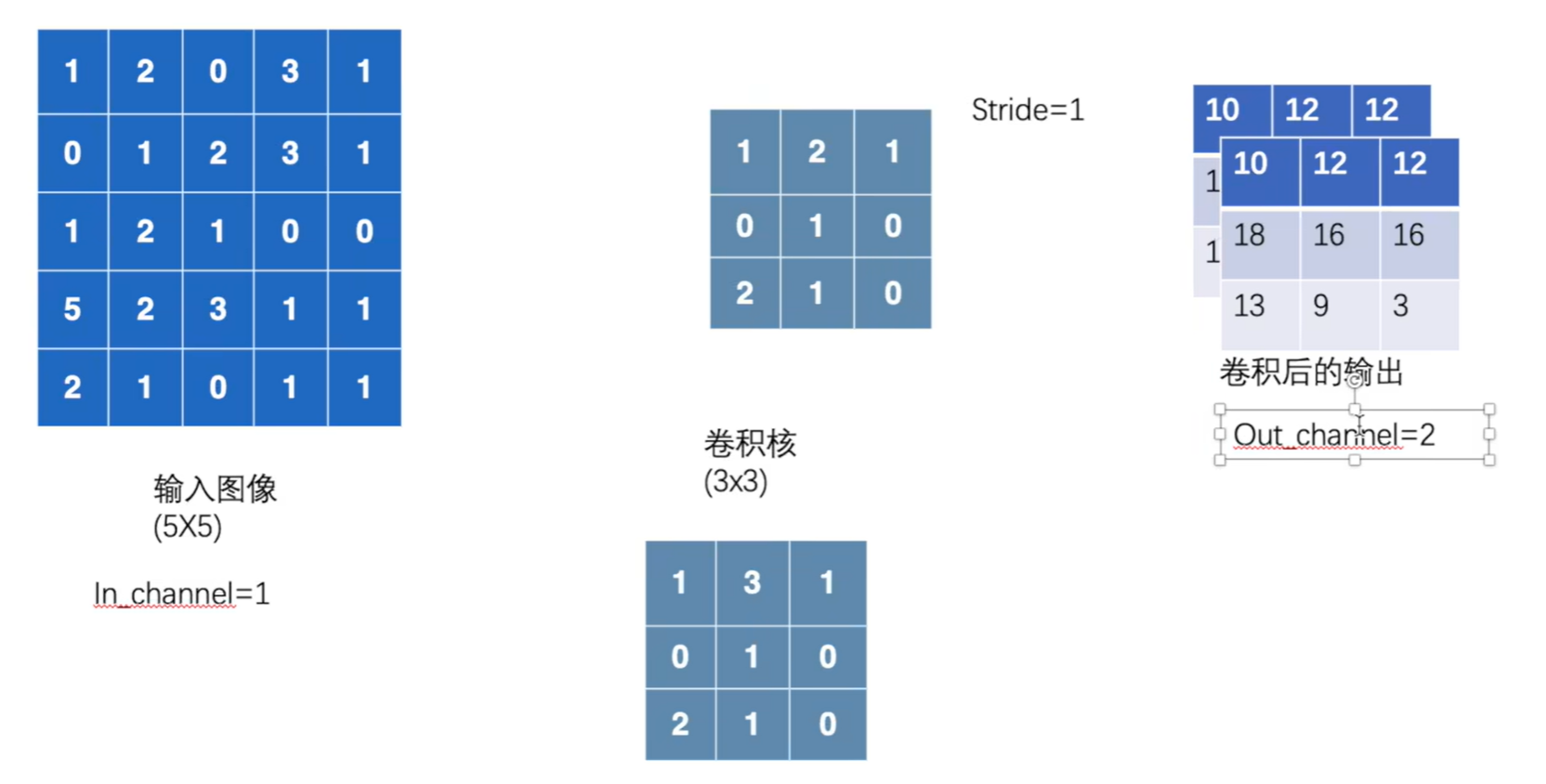
torch.nn.MaxPool2d(kernel_size, stride=None, padding=0, dilation=1, return_indices=False, ceil_mode=False)
kernel_size:表示做最大池化的窗口大小,可以是单个值,也可以是tuple元组
stride:步长,默认是kernel_size大小
dilation:空洞卷积
ceil_mode :布尔类型,为True,用向上取整的方法,计算输出形状;默认是向下取整。
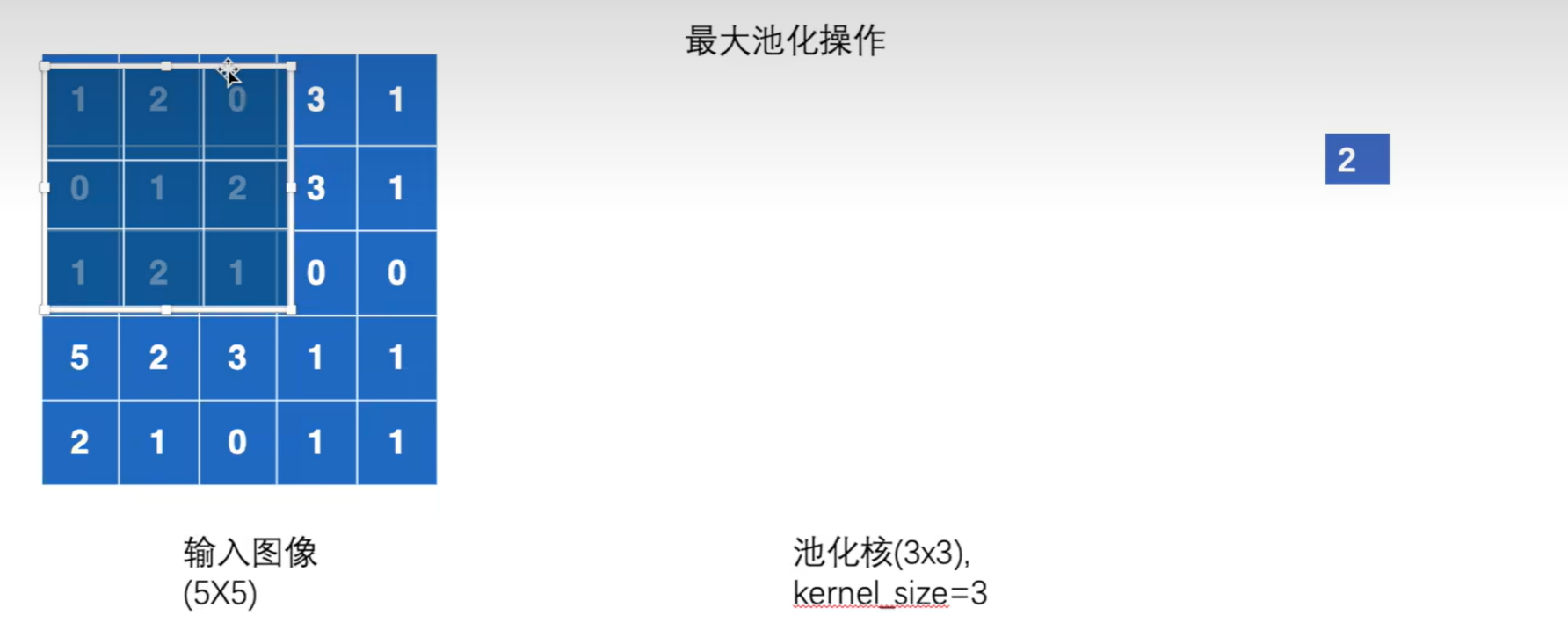
默认的步长是池化核的大小(3)
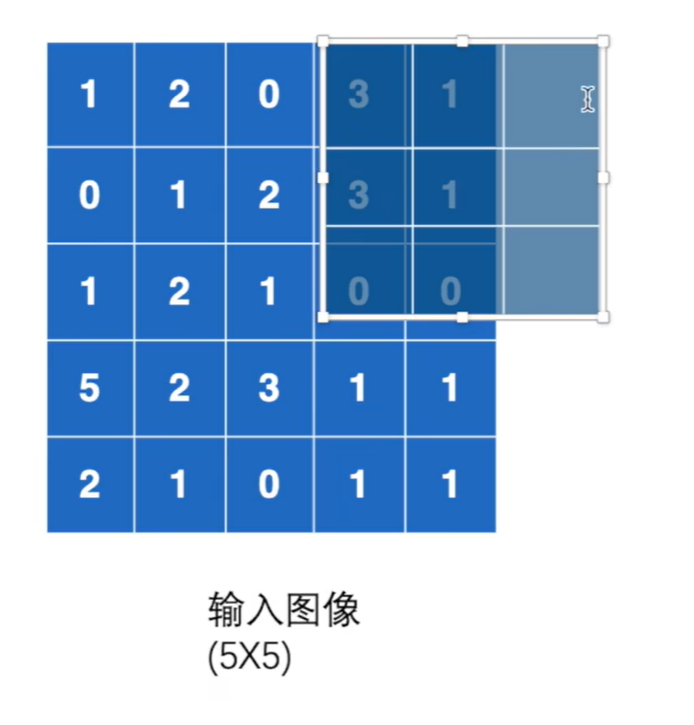
Ceil_mode=True时候,向上取整,保留6个数 。False时不保留
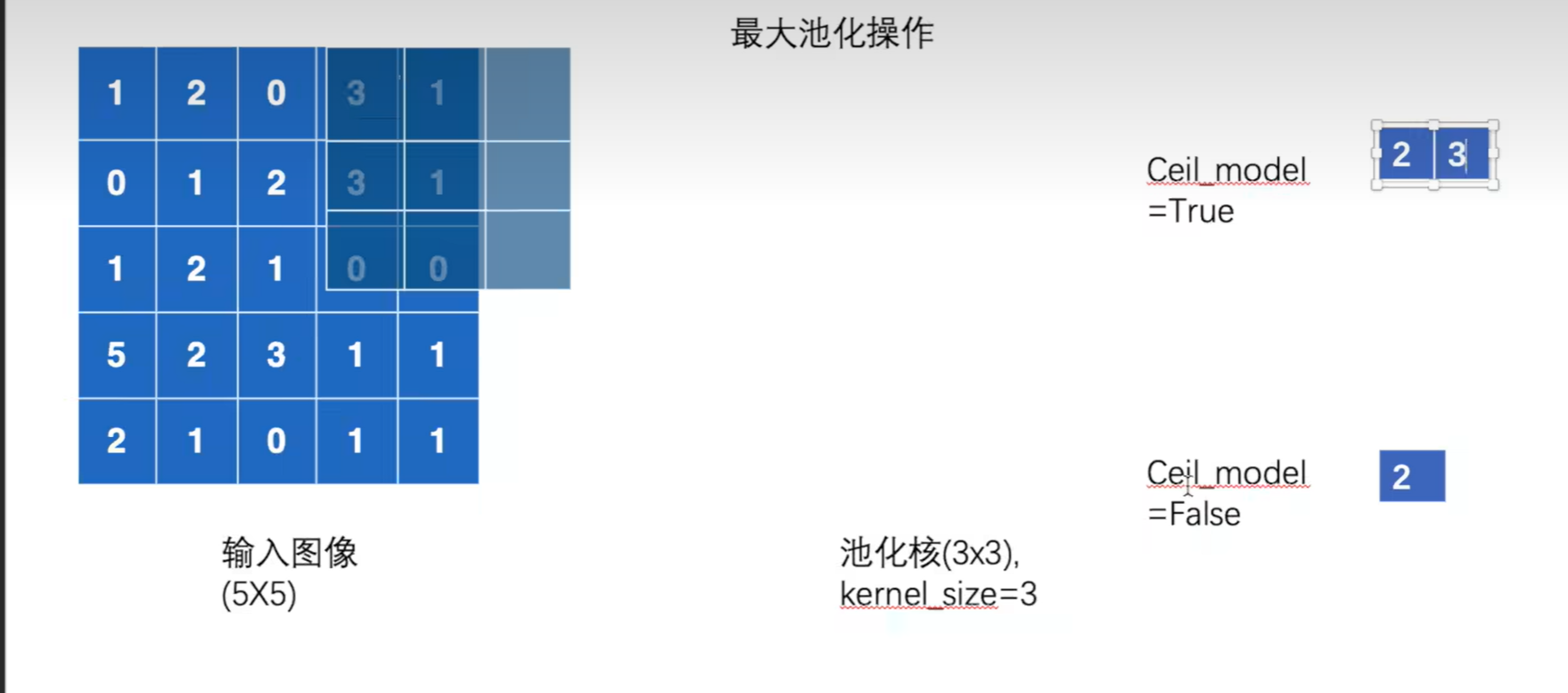
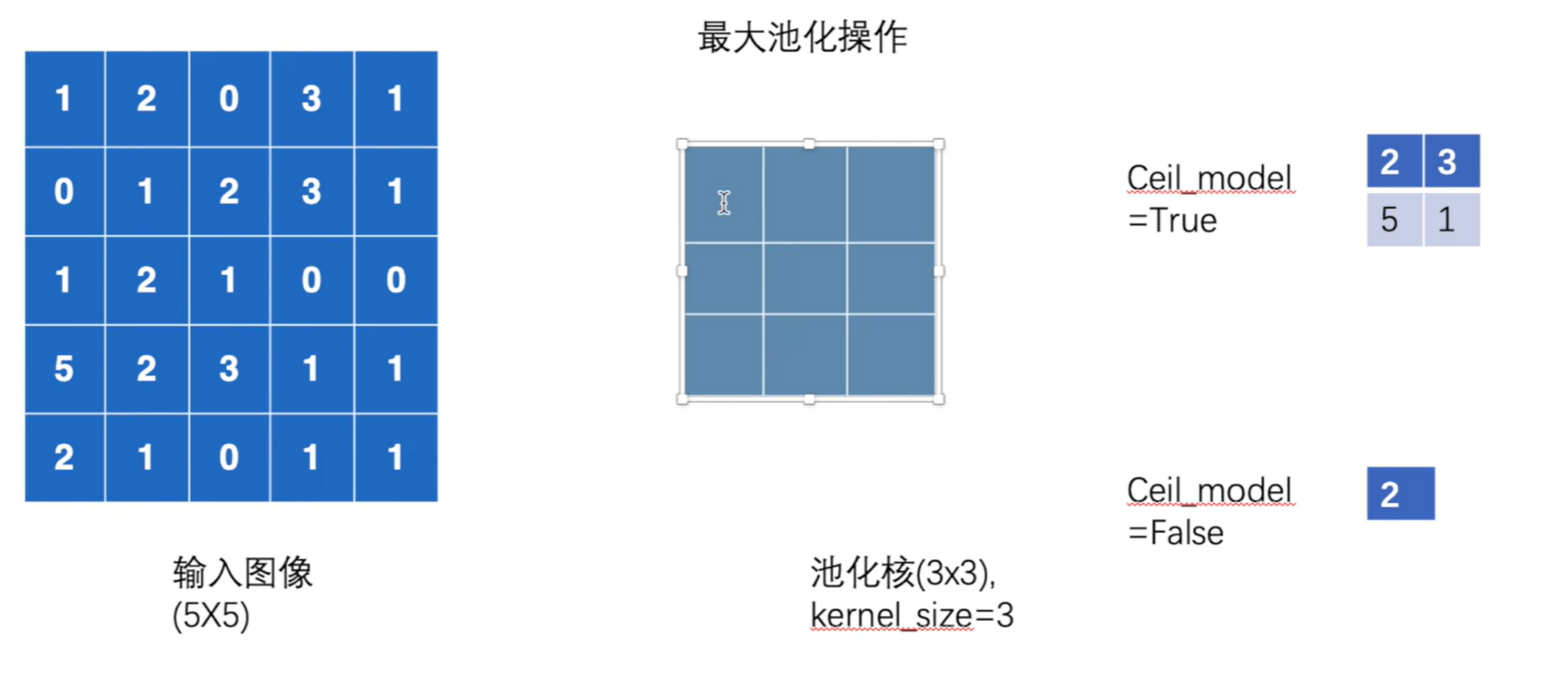
import torch
from torch import nn
from torch.nn import MaxPool2d
input = torch.tensor([[1,2,0,3,1],
[0,1,2,3,1],
[1,2,1,0,0],
[5,2,3,1,1],
[2,1,0,1,1]], dtype=torch.float)
# input:(N,C,Hin,Win) N->batch-size C->通道数
input = torch.reshape(input,(-1,1,5,5))
print(input.shape)#torch.Size([1, 1, 5, 5])
class Lemon(nn.Module):
def __init__(self):
super(Lemon,self).__init__()
self.maxpool1 = MaxPool2d(kernel_size=3,ceil_mode=True)
def forward(self,input):
output = self.maxpool1(input)
return output
lemon = Lemon()
output = lemon(input)
print(output)
最大池化的目的就是保留输入的特征,同时减少数据量。(卷积的作用是提取特征,池化的作用是降低特征的数据量)
import torch
import torchvision
from torch import nn
from torch.nn import MaxPool2d
from torch.utils.data import DataLoader
from torch.utils.tensorboard import SummaryWriter
dataset = torchvision.datasets.CIFAR10("../data",train=False,download=True,transform=torchvision.transforms.ToTensor())
dataloader = DataLoader(dataset,batch_size=64)
class Lemon(nn.Module):
def __init__(self):
super(Lemon,self).__init__()
self.maxpool1 = MaxPool2d(kernel_size=3,ceil_mode=False)
def forward(self,input):
output = self.maxpool1(input)
return output
lemon = Lemon()
writer = SummaryWriter("../logs_maxpool")
step=0
for data in dataloader:
imgs, targets = data
writer.add_images("input",imgs,step)
print(imgs.shape)
output = lemon(imgs) #torch.Size([64, 3, 32, 32])
writer.add_images("output",output,step)
print(output.shape) #torch.Size([64, 3, 10, 10])
step = step + 1
writer.close()
非线性激活是为了给神经网络中引入一些非线性的特质,相当于两层神经元之间的关系函数,上层的输出被激活函数作用得到下层的输入。

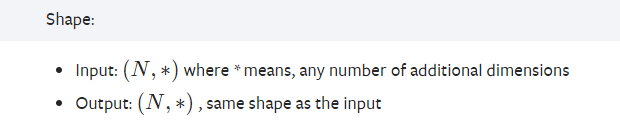
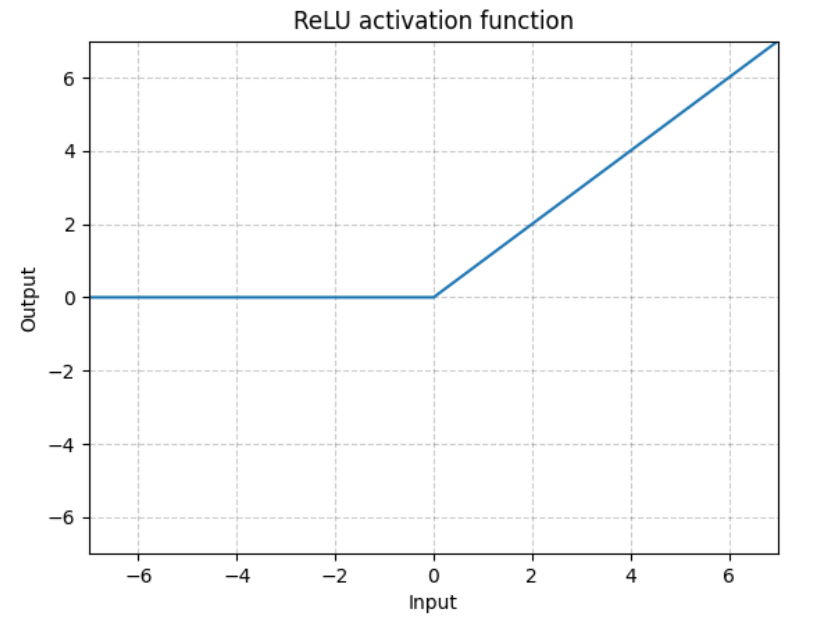
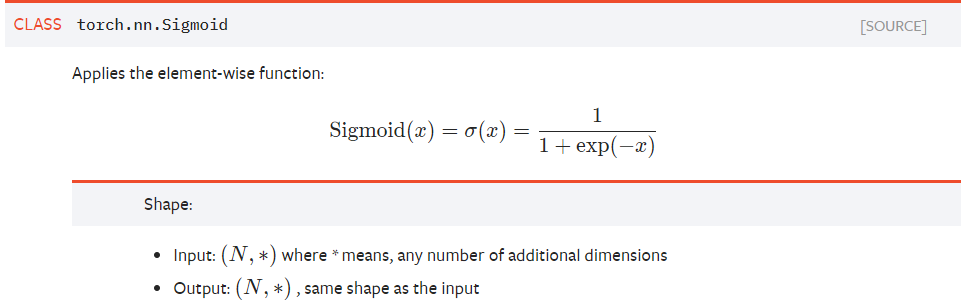
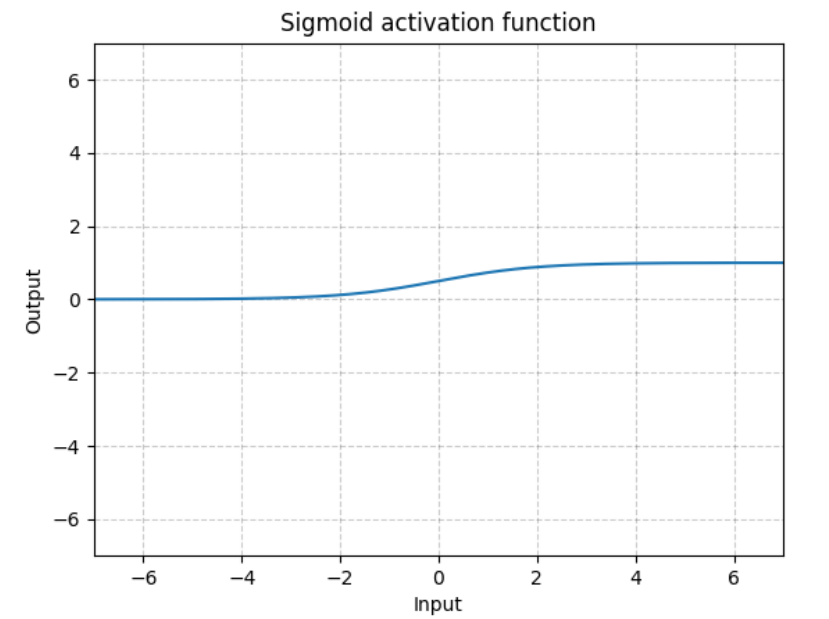
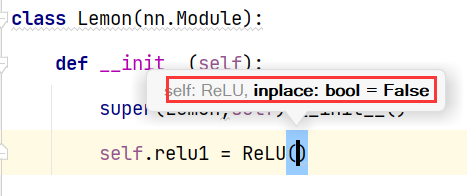

import torch
from torch import nn
from torch.nn import ReLU
input = torch.tensor([[1,-0.5],
[-1,3]])
input = torch.reshape(input,(-1,1,2,2)) #batch-size自己算 1维 2*2
print(input.shape) #torch.Size([1, 1, 2, 2])
class Lemon(nn.Module):
def __init__(self):
super(Lemon,self).__init__()
self.relu1 = ReLU()#inplace:bool=True直接在input上进行替换,默认为False
def forward(self,input):
output = self.relu1(input)
return output
lemon = Lemon()
output = lemon(input)
print(output)
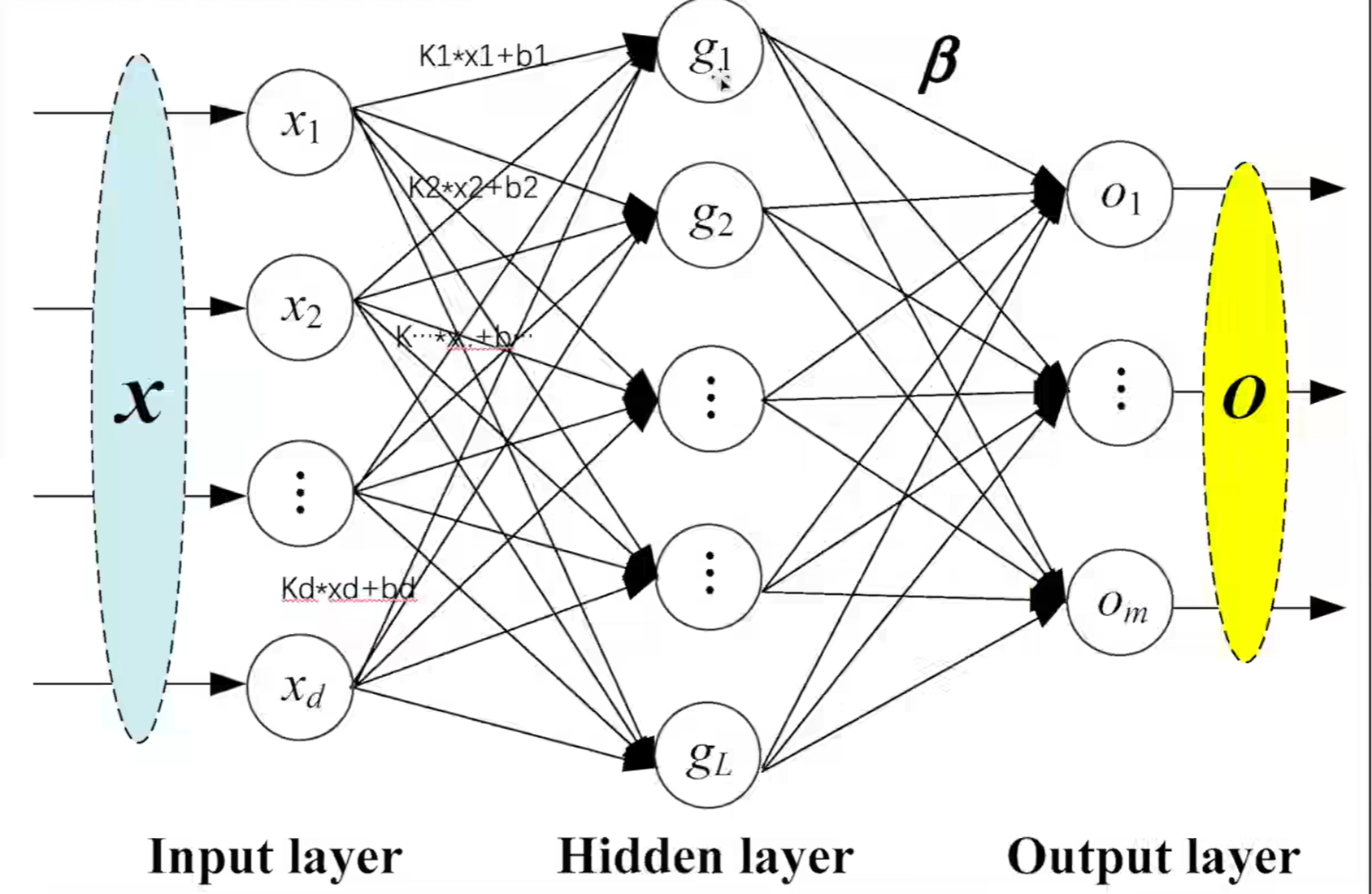
torch.nn.Linear(in_features, out_features, bias=True)
# in_features就是输入的个数d, out_features就是输出的个数l

weight与bias都是从上述分布中进行采样,进行初始化的。

# vgg16 moedel
import torch
import torchvision
from torch import nn
from torch.nn import Linear
from torch.utils.data import DataLoader
dataset = torchvision.datasets.CIFAR10("../data",train=False,transform=torchvision.transforms.ToTensor(),download=True)
dataloader = DataLoader(dataset,batch_size=64,drop_last=True)#doop_last默认为False,保留了最后16张图,会报错
class Lemon(nn.Module):
def __init__(self):
super(Lemon,self).__init__()
self.linear1 = Linear(in_features=196608,out_features=10)
def forward(self,input):
output = self.linear1(input)
return output
lemon = Lemon()
for data in dataloader:
imgs,targets = data # imgs.shape-->torch.Size([64, 3, 32, 32])
print(imgs.shape)
input = torch.reshape(imgs,(1,1,1,-1)) # output.shape-->torch.Size([1, 1, 1, 196608]) 每个batch里面只有一个 单通道 1行n列的矩阵 (batch_size,channel,height,weight)
print(input.shape)
output = lemon(input) #torch.Size([1, 1, 1, 10])
print(output.shape)