1.Neuronal model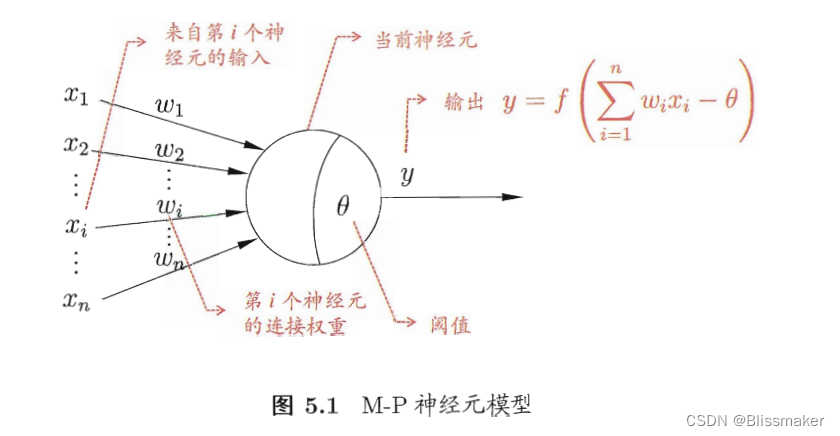
Multiple neuronal models form a feedforward neural network.
Most of the transmission principles are described in the article:
Learning:Get started with neural networks-CSDN博客
The θ is the threshold(阈值), according to which the output can be judged when the prediction probability is greater than or less than the threshold.
for example:
The ideal binary activation function is the step function described above, but it is not smooth and discontinuous, and has many drawbacks.
Therefore, we use the sigmoid function instead, and make the following judgment while continuing: when the output value is greater than 0.5, the output is 1, and when the output value is less than 0.5, the output is 0, and 0.5 is the threshold.
2.Multi-layer feedforward neural networks
It spreads forward in the following manner:
The summary is as follows:
The order in which these formulas are arranged is their order of operation (propagation order).
3.BP algorithm
The so-called bp algorithm adjusts the model parameters by obtaining the final loss function E_k through forward propagation:
Therefore, the parameter adjustment strategy in the model given above is as follows:
First of all, we want to propose a good property of the sigmoid function:
The proof is as follows:
Let's calculate the expressions of these four parameters separately, and the core idea is the chain rule of partial derivatives in mathematical analysis.
For example:
Inside:
Thus:
The detailed calculation process of the four parameters is given below, which uses the derivative operation of the matrix, which can be referred to in the article:
Parameter updates
(1)Layer 2 parameter w update
(2)Layer 2 parameter θ update
(3)Layer 1 parameter v update
(4)Layer 1 parameter γ update
In total:
In this way, we have completed the gradient descent method in neural networks, i.e., the backpropagation algorithm.
Learning rate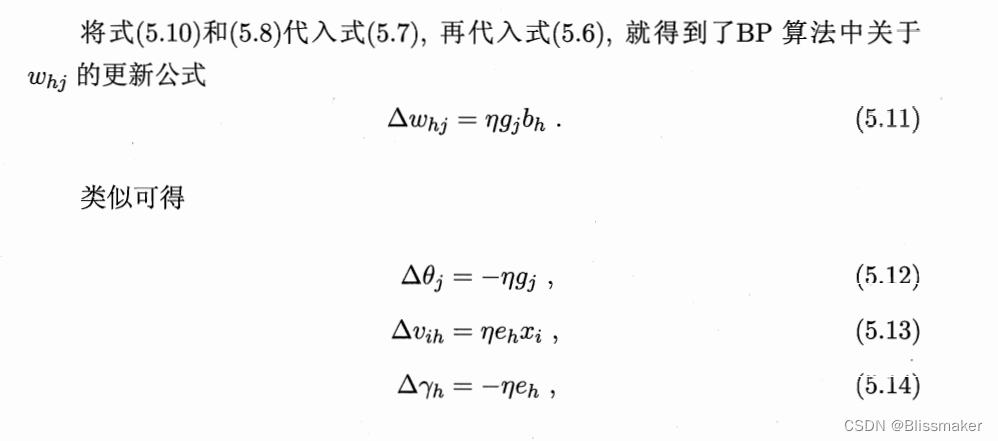
In other words, the learning rate of different layers can be set to different values.
Algorithmic flow
This bp algorithm is for only one input, for example:
4.Accumulation BP algorithm
The accumulation BP algorithm minimizes the accumulation error of the entire training set, that is, the minimum value of the loss function is found as follows:
Since the loss function values of all the training examples need to be calculated, the parameter update frequency becomes very slow.
Regularization
To prevent overfitting issues, we can still use a regularization approach: