Wasserstein Distance
Optimal transport
1. Notations
Consider two probability measures µ and ν defined on measure spaces X and Y . In most applications X and Y are subsets of R d \mathbb{R}^d Rd and µ and ν have density functions which we denote by I 0 I_0 I0 and I 1 I_1 I1, d μ ( x ) = I 0 ( x ) d x d\mu(x)=I_0(x)dx dμ(x)=I0(x)dx and d v ( x ) = I 1 ( x ) d x dv(x) = I_1(x)dx dv(x)=I1(x)dx, (originally representing the height of a pile of soil/sand and the depth of an excavation).
2. Monge’s formulation
Monge’s optimal transportation problem is to find a measurable map f : X → Y that pushes µ onto ν and minimizes the following objective function,
M
(
μ
,
v
)
=
i
n
f
f
∈
M
P
∫
x
c
(
x
,
f
(
x
)
)
d
μ
(
x
)
M(\mu,v)=inf_{f\in MP}\int_xc(x,f(x))d\mu(x)
M(μ,v)=inff∈MP∫xc(x,f(x))dμ(x)
Where
c
:
X
×
Y
→
R
+
c:X\times Y\rightarrow \mathbb{R}^+
c:X×Y→R+, is the cost functional, and
M
P
=
{
f
:
X
→
Y
∣
f
#
μ
=
v
}
MP=\{f:X\rightarrow Y|f_\#\mu=v\}
MP={f:X→Y∣f#μ=v} represents the pushforward of measure µ and is characterized as,
∫
f
−
1
(
A
)
d
μ
(
x
)
=
∫
A
d
ν
(
y
)
\int_{f^{-1}(A)} d \mu(x)=\int_{A} d \nu(y)
∫f−1(A)dμ(x)=∫Adν(y) for any measurable
A
⊂
Y
A\subset Y
A⊂Y.
Simply put, the Monge formulation of the problem seeks the best pushforward map that rearranges measure µ into measure ν while minimizing a specific cost function.
Drawback:
- Nonlinear with respect to f(x)
- For certain measures the Monge’s formulation of the optimal transport problem is illposed; in the sense that there is no transport map to rearrange one measure to another. For instance, consider the case where µ is a Dirac mass while ν is not.
3. Kantorovich’s formulation
Kantorovich’s formulation alleviates this problem by finding the optimal transport plan as opposed to the transport map. Kantorovich formulated the transportation problem by optimizing over transportation plans, where a transport plan is a probability measure
γ
∈
P
(
X
×
Y
)
\gamma\in P(X \times Y)
γ∈P(X×Y) with marginals µ and ν. The quantity
γ
(
A
,
B
)
\gamma(A,B)
γ(A,B) tells us how much ‘mass’ in set A is being moved to set B. Let
Γ
(
μ
,
v
)
\Gamma(\mu,v)
Γ(μ,v) be the set of all such plans. Kantorovich’s formulation can then be written as,
K
(
μ
,
ν
)
=
min
γ
∈
Γ
(
μ
,
ν
)
∫
X
×
Y
c
(
x
,
y
)
d
γ
(
x
,
y
)
K(\mu, \nu)=\min _{\gamma \in \Gamma(\mu, \nu)} \int_{X \times Y} c(x, y) d \gamma(x, y)
K(μ,ν)=γ∈Γ(μ,ν)min∫X×Yc(x,y)dγ(x,y)
Note that unlike the Monge problem, in Kantorovich’s formulation the objective function and the constraints are linear with respect to
γ
(
x
,
y
)
\gamma (x,y)
γ(x,y). Moreover, Kantorovich’s formulation is in the form of a convex optimization problem.
The Kantorovich problem is especially interesting in a discrete setting, that is for probability measures of the form
μ
=
∑
i
=
1
M
p
i
δ
x
i
\mu=\sum_{i=1}^{M} p_{i} \delta_{x_{i}}
μ=∑i=1Mpiδxi and
v
=
∑
j
=
1
N
q
j
δ
x
j
v=\sum_{j=1}^{N} q_{j} \delta_{x_{j}}
v=∑j=1Nqjδxj, where
δ
x
i
\delta_{x_i}
δxi is a dirac measure centered at
x
i
x_i
xi. the Kantorovich problem can be written as,
K
(
μ
,
ν
)
=
min
γ
∑
i
∑
j
c
(
x
i
,
y
j
)
γ
i
j
s.t.
∑
j
γ
i
j
=
p
i
,
∑
i
γ
i
j
=
q
j
γ
i
j
≥
0
,
i
=
1
,
…
,
M
,
j
=
1
,
…
,
N
\begin{aligned} K(\mu, \nu)=& \min _{\gamma} \sum_{i} \sum_{j} c\left(x_{i}, y_{j}\right) \gamma_{i j} \\ \text { s.t. } & \sum_{j} \gamma_{i j}=p_{i}, \sum_{i} \gamma_{i j}=q_{j} \\ & \gamma_{i j} \geq 0, i=1, \ldots, M, j=1, \ldots, N \end{aligned}
K(μ,ν)= s.t. γmini∑j∑c(xi,yj)γijj∑γij=pi,i∑γij=qjγij≥0,i=1,…,M,j=1,…,N
where
γ
i
j
\gamma_{ij}
γij identifies how much of the mass particle
m
i
m_i
mi at
x
i
x_i
xi needs to be moved to
y
i
y_i
yi.
4. Wasserstein Distance
Let
Ω
\Omega
Ω be a subset of
R
d
\mathbb{R}^d
Rd on which the measures we consider are defined. In most applications
Ω
\Omega
Ω is the domain where the signal is defined and thus bounded. Let
P
p
(
Ω
)
P_p(\Omega)
Pp(Ω) be the set of Borel probability measures on
Ω
\Omega
Ω, with finite p’th moment, that is the set of probability measures
μ
\mu
μ on
R
d
\mathbb{R}^d
Rd such that
∫
Ω
∣
x
∣
p
d
μ
(
x
)
<
∞
\int_{\Omega}|x|^{p} d \mu(x)<\infty
∫Ω∣x∣pdμ(x)<∞ The p-Wasserstein distance(metric),
W
p
W_p
Wp , for
p
>
=
1
p>=1
p>=1 on
P
p
(
Ω
)
P_p(\Omega)
Pp(Ω) is then defined as using the Kantorovich’s formulation with the cost function
c
(
x
,
y
)
=
∣
x
−
y
∣
p
c(x,y)=|x-y|^p
c(x,y)=∣x−y∣p, for
μ
\mu
μ and
v
v
v in
P
p
(
Ω
)
P_p(\Omega)
Pp(Ω) ,
W
p
(
μ
,
ν
)
=
(
inf
γ
∈
Γ
(
μ
,
ν
)
∫
Ω
×
Ω
∣
x
−
y
∣
p
d
γ
(
x
,
y
)
)
1
p
W_{p}(\mu, \nu)=\left(\inf _{\gamma \in \Gamma(\mu, \nu)} \int_{\Omega \times \Omega}|x-y|^{p} d \gamma(x, y)\right)^{\frac{1}{p}}
Wp(μ,ν)=(γ∈Γ(μ,ν)inf∫Ω×Ω∣x−y∣pdγ(x,y))p1
For any
p
≥
0
p \ge0
p≥0,
W
p
W_p
Wp is a metric on
P
p
(
Ω
)
P_p(\Omega)
Pp(Ω).The metric space
(
P
p
(
Ω
)
,
W
p
)
(P_p(\Omega),W_p)
(Pp(Ω),Wp)is referred to as the p-Wasserstein space.
Note that the p-Wasserstein metric can equivalently be defined using the dual Kantorovich problem,
W
p
(
μ
,
ν
)
=
(
sup
ϕ
{
∫
Ω
ϕ
(
x
)
d
μ
(
x
)
−
∫
Ω
ϕ
c
(
y
)
d
ν
(
y
)
}
)
1
p
W_{p}(\mu, \nu)=\left(\sup _{\phi}\left\{\int_{\Omega} \phi(x) d \mu(x)-\int_{\Omega} \phi^{c}(y) d \nu(y)\right\}\right)^{\frac{1}{p}}
Wp(μ,ν)=(ϕsup{∫Ωϕ(x)dμ(x)−∫Ωϕc(y)dν(y)})p1
Where
ϕ
c
(
y
)
=
inf
x
{
ϕ
(
x
)
−
∣
x
−
y
∣
p
}
\phi^{c}(y)=\inf _{x}\left\{\phi(x)-|x-y|^{p}\right\}
ϕc(y)=infx{ϕ(x)−∣x−y∣p}. For the specific case of p = 1 the p-Wasserstein metricis also known as the Monge–Rubinstein metric, or the earth mover distance.
Advantage:
- 能够很自然地度量离散分布和连续分布之间的距离;
- 不仅给出了距离的度量,而且给出如何把一个分布变换为另一分布的方案;
- 能够连续地把一个分布变换为另一个分布,在此同时,能够保持分布自身的几何形态特征;(Geodesic) and (Barycenters)
Disadvantage:
- 计算复杂度高,大多数情况下没有闭式解(除了一维和高斯分布)
The p-Wasserstein metric for one-dimensional probability measures is specifically interesting due to its simple and unique characterization.
W
p
(
μ
,
ν
)
=
(
∫
X
∣
x
,
F
ν
−
1
(
F
μ
(
x
)
)
∣
p
d
μ
(
x
)
)
1
p
=
(
∫
0
1
∣
F
μ
−
1
(
z
)
,
F
ν
−
1
(
z
)
∣
p
d
z
)
1
p
\begin{aligned} W_{p}(\mu, \nu) &=\left(\int_{X}|x, F_{\nu}^{-1}\left(F_{\mu}(x)\right)|^p d \mu(x)\right)^{\frac{1}{p}} \\ &=\left(\int_{0}^{1} |F_{\mu}^{-1}(z), F_{\nu}^{-1}(z)|^p d z\right)^{\frac{1}{p}} \end{aligned}
Wp(μ,ν)=(∫X∣x,Fν−1(Fμ(x))∣pdμ(x))p1=(∫01∣Fμ−1(z),Fν−1(z)∣pdz)p1
The closed-form solution of the p-Wasserstein distance in one dimension is an attractive property, as it alleviates the need for optimization. This property was employed in the Sliced Wasserstein distance as defined below.
5. Sliced Wasserstein distance
- Radom Transform
简单来说,Radom Transform 阐述了一幅分布与其在各个角度下投影的具体表示。
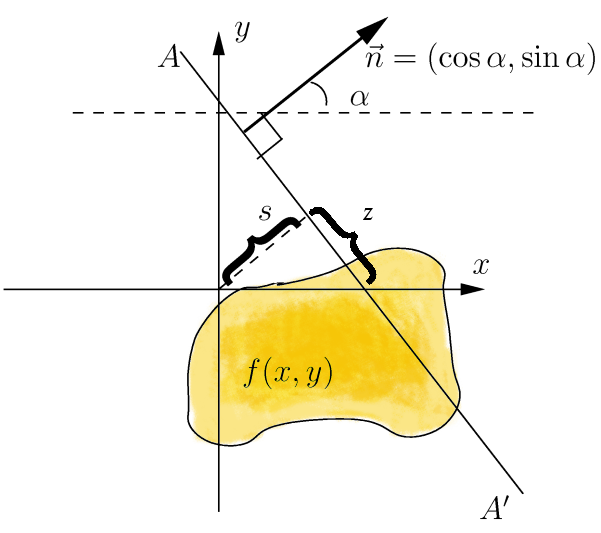
将分布 f ( x , y ) f(x,y) f(x,y)映射到 f ( α , s ) f(\alpha,s) f(α,s).下图也是一个radom transfrom例子:
-
Sliced Wasserstein distance
The idea behind the sliced p-Wasserstein distance is to first, obtain a family of one-dimensional representations for a higher-dimensional probability distribution through linear projections (via the Radon transform), and then, calculate the distance between two input distributions as a functional on the p-Wasserstein distance of their one-dimensional representations. The sliced p-Wasserstein distance between I μ I_\mu Iμ and I v I_v Iv is then formally defined as:
S W p ( I μ , I ν ) = ( ∫ S d − 1 W p p ( R I μ ( . , θ ) , R I ν ( . , θ ) ) d θ ) 1 p S W_{p}\left(I_{\mu},I_{\nu}\right)=\left(\int_{\mathbb{S}^{d-1}} W_{p}^{p}\left(\mathcal{R} I_{\mu}(., \theta), \mathcal{R} I_{\nu}(., \theta)\right) d \theta\right)^{\frac{1}{p}} SWp(Iμ,Iν)=(∫Sd−1Wpp(RIμ(.,θ),RIν(.,θ))dθ)p1
This is indeed a distance function as it satisfifies positive definiteness, symmetry and the triangle inequality. -
Maximum sliced p-Wasserstein (max-SW)
max − S W p ( I μ , I ν ) = max θ ∈ S d − 1 W p ( R I μ ( ⋅ , θ ) , R I ν ( ⋅ , θ ) ) \max -S W_{p}\left(I_{\mu}, I_{\nu}\right)=\max _{\theta \in \mathbb{S}^{d-1}} W_{p}\left(\mathcal{R} I_{\mu}(\cdot, \theta), \mathcal{R} I_{\nu}(\cdot, \theta)\right) max−SWp(Iμ,Iν)=θ∈Sd−1maxWp(RIμ(⋅,θ),RIν(⋅,θ))
6. Generalized Sliced-Wasserstein Distances
The GSW distance is obtained using the same procedure as for the SW distance, except that here, the one-dimensional representations are acquired through nonlinear projections.
-
Generalized Radon Transform
G I ( t , θ ) = ∫ R d I ( x ) δ ( t − g ( x , θ ) ) d x \mathcal{G} I(t, \theta)=\int_{\mathbb{R}^{d}} I(x) \delta(t-g(x, \theta)) d x GI(t,θ)=∫RdI(x)δ(t−g(x,θ))dx -
Generalized Sliced-Wasserstein and Maximum Generalized Sliced-Wasserstein Distances
Following the defifinition of the SW distance, we define the generalized sliced p-Wasserstein distance using the generalized Radon transform as:
G S W p ( I μ , I ν ) = ( ∫ Ω θ W p p ( G I μ ( ⋅ , θ ) , G I ν ( ⋅ , θ ) ) d θ ) 1 p G S W_{p}\left(I_{\mu}, I_{\nu}\right)=\left(\int_{\Omega_{\theta}} W_{p}^{p}\left(\mathcal{G} I_{\mu}(\cdot, \theta), \mathcal{G} I_{\nu}(\cdot, \theta)\right) d \theta\right)^{\frac{1}{p}} GSWp(Iμ,Iν)=(∫ΩθWpp(GIμ(⋅,θ),GIν(⋅,θ))dθ)p1
Maximum Generalized Sliced-Wasserstein Distances:
max − G S W p ( I μ , I ν ) = max θ ∈ Ω θ W p ( G I μ ( ⋅ , θ ) , G ν ( ⋅ , θ ) ) \max -G S W_{p}\left(I_{\mu}, I_{\nu}\right)=\max _{\theta \in \Omega_{\theta}} W_{p}\left(\mathcal{G} I_{\mu}(\cdot, \theta), \mathcal{G}_{\nu}(\cdot, \theta)\right) max−GSWp(Iμ,Iν)=θ∈ΩθmaxWp(GIμ(⋅,θ),Gν(⋅,θ)) -
Algorithm