Squeeze-and-Excitation Attention模块
链接:Squeeze-and-Excitation Networks
模型结构图
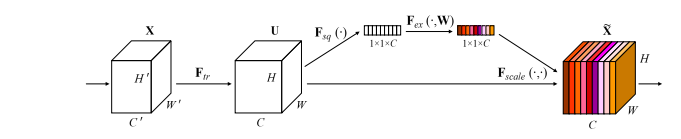
论文主要核心思想
Squeeze-and-Excitation Networks(SENets)是一种网络架构,旨在改善网络的特征计算。该架构包括两个主要组件:Squeeze(压缩)和Excitation(激发)。Squeeze组件负责通过学习分析每个通道的全局信息,从而提取出每个通道的有用特征。这是通过让每个通道的特征向量通过一个全局池化层,从而将特征向量压缩成一个固定维度的特征表示。Excitation组件负责改变每个通道的特征表示。这是通过将Squeeze组件输出的特征表示与每个通道的特征向量做乘法,从而加权每个通道的特征向量,从而改变每个通道的特征表示。
总的来说,SENets的核心思想是,通过将每个通道的特征向量通过Squeeze组件进行压缩,并且通过Excitation组件来加权每个通道的特征表示,从而提高网络的特征计算能力。
import numpy as np
import torch
from torch import nn
from torch.nn import init
# 定义一个SEAttention类,继承自nn.Module
class SEAttention(nn.Module):
# 初始化函数,设置默认参数channel=512,reduction=16
def __init__(self, channel=512,reduction=16):
super().__init__()
# 定义一个自适应平均池化层,使用1x1的池化核
self.avg_pool = nn.AdaptiveAvgPool2d(1)
#定义全连接层,包括两个线性层,一个ReLu激活函数和一个Sigmoid激活函数
self.fc = nn.Sequential(
nn.Linear(channel, channel // reduction, bias=False),
nn.ReLU(inplace=True),
nn.Linear(channel // reduction, channel, bias=False),
nn.Sigmoid()
)
#定义一个初始化权重的函数
def init_weights(self):
for m in self.modules():
if isinstance(m, nn.Conv2d):
init.kaiming_normal_(m.weight, mode='fan_out')
if m.bias is not None:
init.constant_(m.bias, 0)
elif isinstance(m, nn.BatchNorm2d):
init.constant_(m.weight, 1)
init.constant_(m.bias, 0)
elif isinstance(m, nn.Linear):
init.normal_(m.weight, std=0.001)
if m.bias is not None:
init.constant_(m.bias, 0)
def forward(self, x):
b, c, _, _ = x.size()
#全局平均池化,并获得通道注意力权重
y = self.avg_pool(x).view(b, c)
y = self.fc(y).view(b, c, 1, 1)
return x * y.expand_as(x)
if __name__ == '__main__':
# 定义一个随机输入
input=torch.randn(50,512,7,7)
se = SEAttention(channel=512,reduction=8)
output=se(input)
print(output.shape)