EfficientNet简记
主要问题
- 作者发现,Model Scaling(放缩)通常有三种方法(depth、width、img size),然而以往的Model Scaling大多只用了一种或者两种,比如ResNet是深度,后来有加上了img size而没有width维度上的Scaling,于是作者提出:
- 是否有一种原则性的方法来拓展ConvNet,以获得更好的准确率?(depth、width、img size)
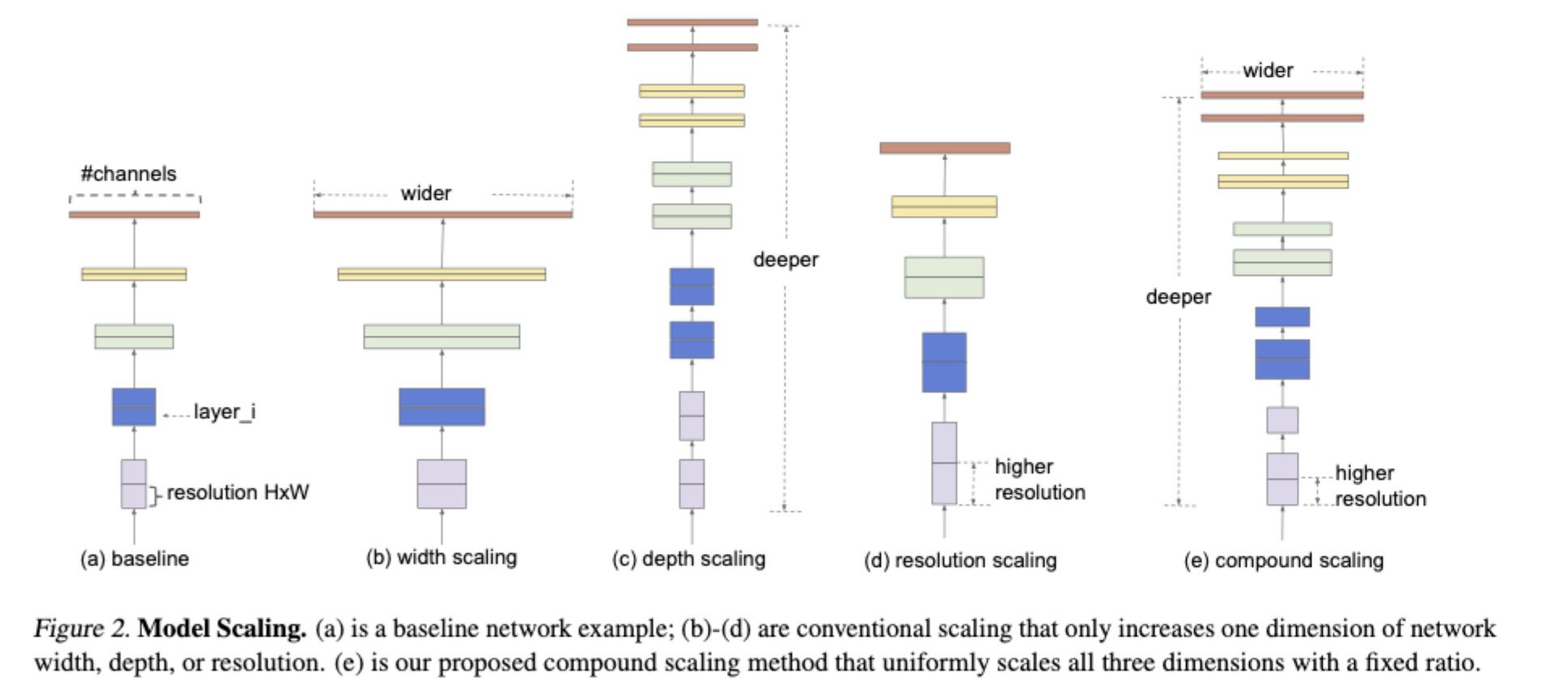
作者的给的思路是
问题定义
- 定义了三个超参数,d,w,r
- d控制L,即深度
- w控制输出channel数量(kernel的数量)
- r控制H x W维度上的放缩
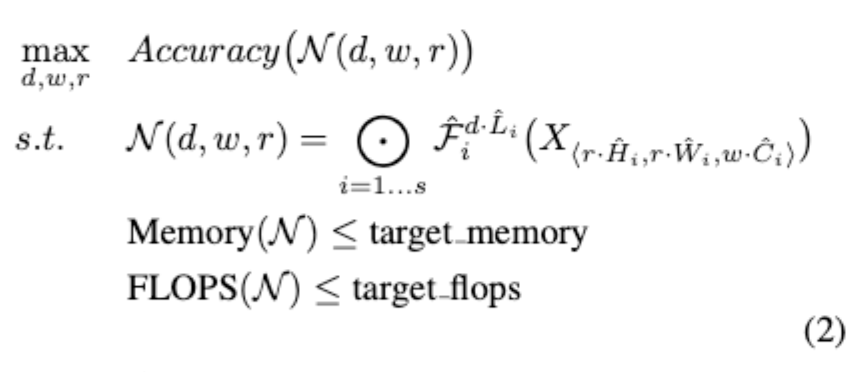
满足如下要求
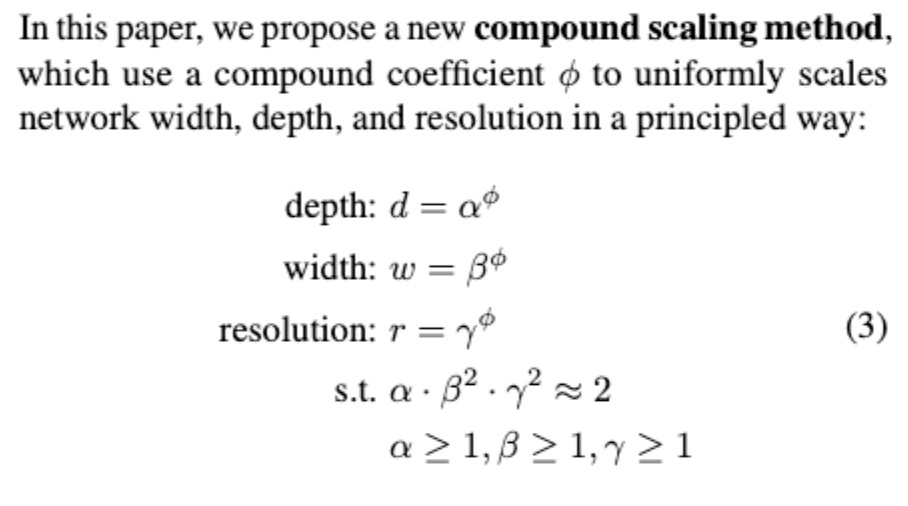
- 需要满足的要求是:
α
∗
β
2
∗
γ
2
≈
2
\alpha * \beta^2*\gamma^2 \approx 2
α∗β2∗γ2≈2,然后通过改变
ϕ
\phi
ϕ实现统一放缩
- 至于这里为什么是这样,我稍微着了一下没找到说明,可能是作者试出来比较好的结果吧(不对此话负责)
代码实现
import math
import copy
from functools import partial
from collections import OrderedDict
from re import L
from typing import Optional, Callable
import torch
from torch._C import device
from torch.functional import norm
import torch.nn as nn
from torch import Tensor
from torch import Tensor
from torch.nn import functional as F
from torch.nn.modules import activation, padding
def _make_divisible(ch, divisor=8, min_ch=None):
"""
This function is taken from the original tf repo.
It ensures that all layers have a channel number that is divisible by 8
It can be seen here:
https://github.com/tensorflow/models/blob/master/research/slim/nets/mobilenet/mobilenet.py
"""
if min_ch is None:
min_ch = divisor
new_ch = max(min_ch, int(ch + divisor / 2) // divisor * divisor)
if new_ch < 0.9 * ch:
new_ch += divisor
return new_ch
def drop_path(x, drop_prob: float = 0., training: bool = False):
"""
Drop paths (Stochastic Depth) per sample (when applied in main path of residual blocks).
"Deep Networks with Stochastic Depth", https://arxiv.org/pdf/1603.09382.pdf
This function is taken from the rwightman.
It can be seen here:
https://github.com/rwightman/pytorch-image-models/blob/master/timm/models/layers/drop.py#L140
"""
if drop_prob == 0. or not training:
return x
keep_prob = 1 - drop_prob
shape = (x.shape[0], ) + (1, ) * (
x.ndim - 1)
random_tensor = keep_prob + torch.rand(
shape, dtype=x.dtype, device=x.device)
output = x.div(keep_prob) * random_tensor
return output
class DropPath(nn.Module):
"""
Drop paths (Stochastic Depth) per sample (when applied in main path of residual blocks).
"Deep Networks with Stochastic Depth", https://arxiv.org/pdf/1603.09382.pdf
"""
def __init__(self, drop_prob=None):
super(DropPath,self).__init__()
self.drop_prob = drop_prob
def forward(self, x):
return drop_path(x, self.drop_prob, self.training)
class ConvBNActivation(nn.Sequential):
def __init__(self,
in_planes: int,
out_planes: int,
kernel_size: int = 3,
stride: int = 1,
groups: int = 1,
norm_layer: Optional[Callable[..., nn.Module]] = None,
activation_layer: Optional[Callable[..., nn.Module]] = None):
padding = (kernel_size - 1) // 2
if norm_layer is None:
nrom_layer = nn.BatchNorm2d
if activation_layer is None:
activation_layer = nn.SiLU
super(ConvBNActivation, self).__init__(
nn.Conv2d(in_channels=in_planes,
out_channels=out_planes,
kernel_size=kernel_size,
stride=stride,
padding=padding,
groups=groups,
bias=False), norm_layer(out_planes), activation_layer())
class SqueezeExcitation(nn.Module):
def __init__(self, input_c: int, expand_c: int, squeeze_factor: int = 4):
super(SqueezeExcitation, self).__init__()
squeeze_c = input_c // squeeze_factor
self.fc1 = nn.Conv2d(expand_c, squeeze_c, 1)
self.ac1 = nn.SiLU()
self.fc2 = nn.Conv2d(squeeze_c, expand_c, 1)
self.ac2 = nn.Sigmoid()
def forward(self, x: Tensor) -> Tensor:
scale = F.adaptive_avg_pool2d(x, output_size=(1, 1))
scale = self.fc1(scale)
scale = self.ac1(scale)
scale = self.fc2(scale)
scale = self.ac2(scale)
return x * scale
class InvertedResidualConfig:
def __init__(self, kernel: int, input_c: int, out_c: int,
expanded_ratio: int, stride: int, use_se: bool,
drop_rate: float, index: str,
width_coefficient: float) -> None:
self.input_c = self.adjust_channels(input_c, width_coefficient)
self.kernel = kernel
self.expanded_c = self.input_c * expanded_ratio
self.out_c = self.adjust_channels(out_c,
width_coefficient=width_coefficient)
self.use_se = use_se
self.stride = stride
self.drop_rate = drop_rate
self.index = index
@staticmethod
def adjust_channels(channels: int, width_coefficient: float):
return _make_divisible(channels * width_coefficient, 8)
class InvertedResidual(nn.Module):
def __init__(self, cnf: InvertedResidualConfig,
norm_layer: Callable[..., nn.Module]):
super(InvertedResidual, self).__init__()
if cnf.stride not in [1, 2]:
raise ValueError("illegal stride value")
self.use_res_connect = (cnf.stride == 1 and cnf.input_c == cnf.out_c)
layers = OrderedDict()
activation_layer = nn.SiLU
if cnf.expanded_c != cnf.input_c:
layers.update({
"expand_conv":
ConvBNActivation(cnf.input_c,
cnf.expanded_c,
kernel_size=1,
norm_layer=norm_layer,
activation_layer=activation_layer)
})
layers.update({
"dwconv":
ConvBNActivation(cnf.expanded_c,
cnf.expanded_c,
norm_layer=norm_layer,
activation_layer=activation_layer)
})
if cnf.use_se:
layers.update(
{"se": SqueezeExcitation(cnf.input_c, cnf.expanded_c)})
layers.update({
"project_conv":
ConvBNActivation(cnf.expanded_c,
cnf.out_c,
kernel_size=1,
norm_layer=norm_layer,
activation_layer=nn.Identity)
})
self.block = nn.Sequential(layers)
self.out_channels = cnf.out_c
self.is_strided = cnf.stride > 1
if self.use_res_connect and cnf.drop_rate > 0:
self.dropout = DropPath(cnf.drop_rate)
else:
self.dropout = nn.Identity()
def forward(self, x: Tensor) -> Tensor:
result = self.block(x)
result = self.dropout(result)
if self.use_res_connect:
result += x
return result
class EfficientNet(nn.Module):
def __init__(self,
width_coefficient: float,
depth_coefficient: float,
num_classes: int = 1000,
dropout_rate: float = 0.2,
drop_connect_rate: float = 0.2,
block: Optional[Callable[..., nn.Module]] = None,
norm_layer: Optional[Callable[..., nn.Module]] = None):
super(EfficientNet, self).__init__()
default_cnf = [[3, 32, 16, 1, 1, True, drop_connect_rate, 1],
[3, 16, 24, 6, 2, True, drop_connect_rate, 2],
[5, 24, 40, 6, 2, True, drop_connect_rate, 2],
[3, 40, 80, 6, 2, True, drop_connect_rate, 3],
[5, 80, 112, 6, 1, True, drop_connect_rate, 3],
[5, 112, 192, 6, 2, True, drop_connect_rate, 4],
[3, 192, 320, 6, 1, True, drop_connect_rate, 1]]
def round_repeats(repeats):
return int(math.ceil(depth_coefficient * repeats))
if block is None:
block = InvertedResidual
if norm_layer is None:
norm_layer = partial(nn.BatchNorm2d, eps=1e-3, momentum=0.1)
adjust_channels = partial(InvertedResidualConfig.adjust_channels,
width_coefficient=width_coefficient)
bneck_conf = partial(InvertedResidualConfig,
width_coefficient=width_coefficient)
b = 0
num_blocks = float(sum(round_repeats(i[-1]) for i in default_cnf))
inverted_residual_setting = []
for stage, args in enumerate(default_cnf):
cnf = copy.copy(args)
for i in range(round_repeats(cnf.pop(-1))):
if i > 0:
cnf[-3] = 1
cnf[1] = cnf[2]
cnf[-1] = args[-2] * b / num_blocks
index = str(stage + 1) + chr(i + 97)
inverted_residual_setting.append(bneck_conf(*cnf, index))
b += 1
layers = OrderedDict()
layers.update({
"stem_conv":
ConvBNActivation(in_planes=3,
out_planes=adjust_channels(channels=32),
kernel_size=3,
stride=2,
norm_layer=norm_layer)
})
for cnf in inverted_residual_setting:
layers.update({cnf.index: block(cnf, norm_layer=norm_layer)})
last_conv_input_c = inverted_residual_setting[-1].out_c
last_conv_output_c = adjust_channels(1280)
layers.update({
"top":
ConvBNActivation(in_planes=last_conv_input_c,
out_planes=last_conv_output_c,
kernel_size=1,
norm_layer=norm_layer)
})
self.features = nn.Sequential(layers)
self.avgpool = nn.AdaptiveAvgPool2d(1)
classifier = []
if dropout_rate > 0:
classifier.append(nn.Dropout(p=dropout_rate, inplace=True))
classifier.append(nn.Linear(last_conv_output_c, num_classes))
self.classifier = nn.Sequential(*classifier)
for m in self.modules():
if isinstance(m, nn.Conv2d):
nn.init.kaiming_normal_(m.weight, mode="fan_out")
if m.bias is not None:
nn.init.zeros_(m.bias)
elif isinstance(m, nn.BatchNorm2d):
nn.init.ones_(m.weight)
nn.init.zeros_(m.bias)
elif isinstance(m, nn.Linear):
nn.init.normal_(m.weight, 0, 0.01)
nn.init.zeros_(m.bias)
def _forward_impl(self, x: Tensor) -> Tensor:
x = self.features(x)
x = self.avgpool(x)
x = torch.flatten(x, 1)
x = self.classifier(x)
return x
def forward(self, x: Tensor) -> Tensor:
return self._forward_impl(x)
EfficientNetConfig = {
"efficientnet_b0": {
"width_coefficient": 1.0,
"depth_coefficient": 1.0,
"dropout_rate": 0.2
},
"efficientnet_b1": {
"width_coefficient": 1.0,
"depth_coefficient": 1.1,
"dropout_rate": 0.2
},
"efficientnet_b2": {
"width_coefficient": 1.1,
"depth_coefficient": 1.2,
"dropout_rate": 0.3
},
"efficientnet_b3": {
"width_coefficient": 1.2,
"depth_coefficient": 1.4,
"dropout_rate": 0.3
},
"efficientnet_b4": {
"width_coefficient": 1.4,
"depth_coefficient": 1.8,
"dropout_rate": 0.3
},
"efficientnet_b5": {
"width_coefficient": 1.6,
"depth_coefficient": 2.2,
"dropout_rate": 0.4
},
"efficientnet_b6": {
"width_coefficient": 1.8,
"depth_coefficient": 2.6,
"dropout_rate": 0.5
},
"efficientnet_b7": {
"width_coefficient": 2.0,
"depth_coefficient": 3.1,
"dropout_rate": 0.5
}
}
def efficientnet(model_name="efficientnet_b0", num_classes=1000):
cfg = EfficientNetConfig[model_name]
return EfficientNet(width_coefficient=cfg["width_coefficient"],
depth_coefficient=cfg["depth_coefficient"],
dropout_rate=cfg["dropout_rate"],
num_classes=num_classes)