目录(方便查找自己所需)
目录
1、YOLOv9介绍
YOLOv9 是一种用于实时目标检测的深度学习模型,是由 Joseph Redmon 和 Alexey Bochkovskiy 等人共同开发的。它是 YOLO 系列中的最新版本(现在yolov10已出),采用了一种基于骨干网络的轻量化设计,能够在保持检测准确性的同时提高检测速度,适合应用于实时场景。
2、yolov9模型图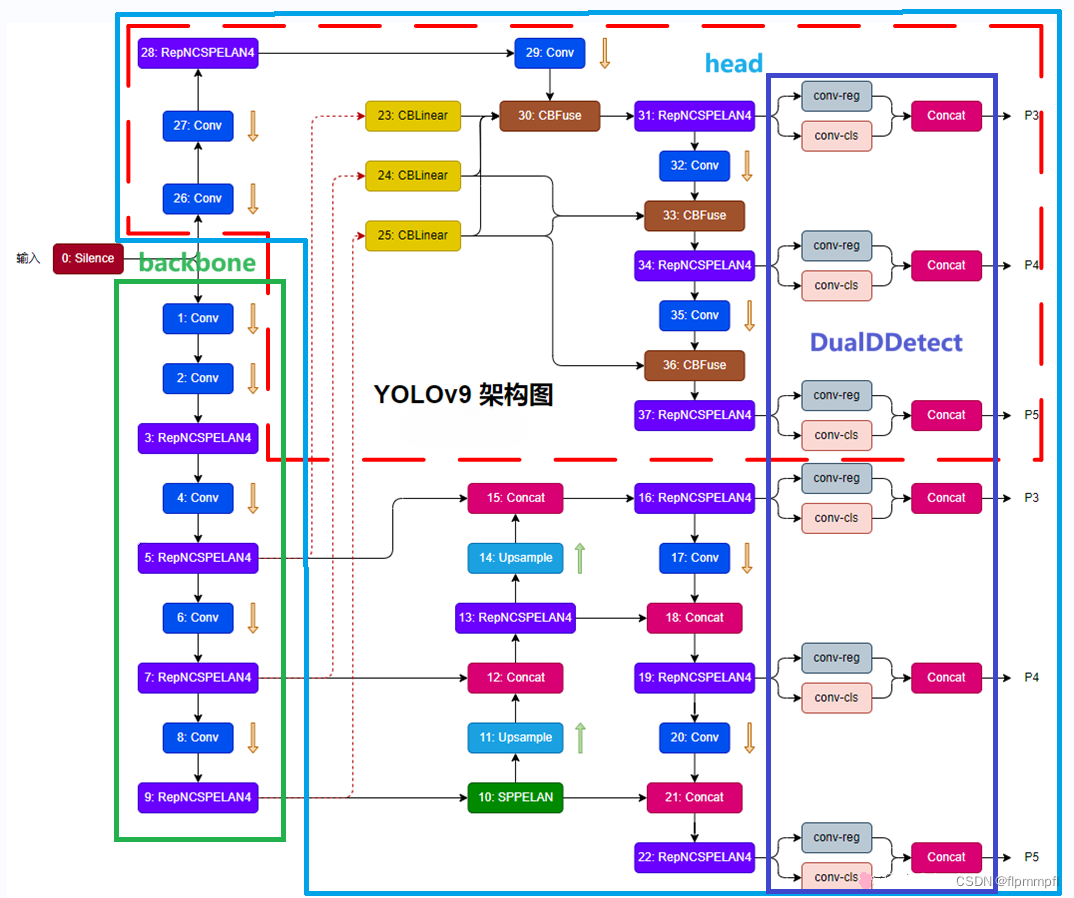
3、前提所需
anaconda3、pycharm下载完成(最好不要最新版本,也不要太旧,本人使用2021.3)
4、yolov9模型、权重下载
1.模型下载:github模型代码下载
2.模型权重下载(非必须):yolov9模型下载相同位置,往下翻
位置通常放models文件下
5、环境配置
5.1.下载yolov9所需虚拟环境
打开anaconda prompt
cd进入你下载的yolov9文件夹中
创建yolov9环境:输入 conda create -n yolov9(代表环境名称)python=3.8(使用Python的版本),按y+回车
conda create -n yolov9 python=3.8
进入你刚安装的yolov9环境:conda activate yolov9(你的环境名称)
conda activate yolov9
进入后()会变成你环境名称 (判断是否安装成功)
5.2.下载yolov9依赖库
加清华端会下载更快,建议加清华端口
//不加清华源
pip install -r requirements.txt
//加清华源
pip install -r requirements.txt -i https://pypi.tuna.tsinghua.edu.cn/simple
只要中间不报错即为下载成功
5.3.下载CUDA
cuda是连接GPU和模型训练的桥梁,pytorch是进入桥梁那段上坡的路(CPU训练慢,GPU快还好)
(最好选择在虚拟环境安装,以下介绍为虚拟机安装过程)
查看cuda最高支持版本,win+R,输入cmd,打开命令窗口,输入nvidia-smi.exe,红框位置为最大可支持安装版本
CUDA一般安装较低版本比较稳定,不会发疯
推荐安装CUDA11.0,进入环境,找到模型文件夹,ctrl+c,v(代码直接复制粘贴即可)
# 安装CUDA # 指定版本
conda install cudatoolkit=11.0
这里是指定搜索包的路径,速度会更快
# 安装CUDA
conda install cudatoolkit=11.0 -c https://mirrors.tuna.tsinghua.edu.cn/anaconda/pkgs/free/linux-64/
个别需要高版本的CUDA,这里也补充上(如:yolov10)
# 安装CUDA # 指定版本
conda install cudatoolkit=11.7
5.4.下载pytorch
cuda和cuDNN是连接GPU和模型训练的桥梁,pytorch是进入桥梁那段上坡的路(pytorch库必须和cuda版本匹配)(其实这个在之前下载yolov9虚拟环境已经下载过了,但是为了确保你的pytorch版本和CUDA版本一致,所以这里在从下一遍以确保环境没有问题)
无脑复制(CUDA 11.0)Y+回车
conda install pytorch==1.7.1 torchvision==0.8.2 torchaudio==0.7.2 cudatoolkit=11.0 -c pytorch
无脑复制(CUDA 11.7)Y+回车
conda install pytorch==1.13.1 torchvision==0.14.1 torchaudio==0.13.1 pytorch-cuda=11.7 -c pytorch -c nvidia
或者去pytorch官网查找
从下载的yolov9文件中去查看所需要的最低版本号
找到requirements.txt,可以看到是最低需要1.7.0版本
然后找到最低的pytorch版本号和对应的cuda版本,复制下面的代码
5.5.检测是否安装正确
(环境已经配好添加好了,6搞完)
建立cuda.py
import torch
print('CUDA版本:',torch.version.cuda)
print('Pytorch版本:',torch.__version__)
print('显卡是否可用:','可用' if(torch.cuda.is_available()) else '不可用')
print('显卡数量:',torch.cuda.device_count())
print('当前显卡型号:',torch.cuda.get_device_name())
print('当前显卡的CUDA算力:',torch.cuda.get_device_capability())
print('当前显卡的总显存:',torch.cuda.get_device_properties(0).total_memory/1024/1024/1024,'GB')
print('是否支持TensorCore:','支持' if (torch.cuda.get_device_properties(0).major >= 7) else '不支持')
print('当前显卡的显存使用率:',torch.cuda.memory_allocated(0)/torch.cuda.get_device_properties(0).total_memory*100,'%')
6、pycharm添加解释器
点击文件、设置
找到python解释器,添加新的解释器
找到conda环境、使用现有环境,找到你前面配置的yolov9(环境名称)
还有老版的pycharm,使用应该找Anaconda3\envs\yolov9(环境名称)\python.exe
点击应用
7、数据集制作
最终结构图
在yolo9-main文件夹中创建datasets(数据文件夹),fish(你要训练的数据),Annotations(json文件),images(原图),lables(txt文件)ImageSets(数据集分组),效果如下:
XML转txt
(xml和json选自己所需)
在datasets(数据文件夹)创建xmltotxt.py用于将xml转化为txt文件,复制粘贴即可,需修改转化的类别名称,源文件地址和转化后文件地址(最好使用绝对路径)
import xml.etree.ElementTree as ET
import pickle
import os
from os import listdir, getcwd
from os.path import join
def convert(size, box):
# size=(width, height) b=(xmin, xmax, ymin, ymax)
# x_center = (xmax+xmin)/2 y_center = (ymax+ymin)/2
# x = x_center / width y = y_center / height
# w = (xmax-xmin) / width h = (ymax-ymin) / height
x_center = (box[0] + box[1]) / 2.0
y_center = (box[2] + box[3]) / 2.0
x = x_center / size[0]
y = y_center / size[1]
w = (box[1] - box[0]) / size[0]
h = (box[3] - box[2]) / size[1]
# print(x, y, w, h)
return (x, y, w, h)
def convert_annotation(xml_files_path, save_txt_files_path, classes):
xml_files = os.listdir(xml_files_path)
# print(xml_files)
for xml_name in xml_files:
# print(xml_name)
xml_file = os.path.join(xml_files_path, xml_name)
out_txt_path = os.path.join(save_txt_files_path, xml_name.split('.')[0] + '.txt')
out_txt_f = open(out_txt_path, 'w')
tree = ET.parse(xml_file)
root = tree.getroot()
size = root.find('size')
w = int(size.find('width').text)
h = int(size.find('height').text)
for obj in root.iter('object'):
difficult = obj.find('difficult').text
cls = obj.find('name').text
if cls not in classes or int(difficult) == 1:
continue
cls_id = classes.index(cls)
xmlbox = obj.find('bndbox')
b = (float(xmlbox.find('xmin').text), float(xmlbox.find('xmax').text), float(xmlbox.find('ymin').text),
float(xmlbox.find('ymax').text))
# b=(xmin, xmax, ymin, ymax)
# print(w, h, b)
bb = convert((w, h), b)
out_txt_f.write(str(cls_id) + " " + " ".join([str(a) for a in bb]) + '\n')
if __name__ == "__main__":
# 把forklift_pallet的voc的xml标签文件转化为yolo的txt标签文件
# 1、需要转化的类别
classes = ['fish']
# 2、voc格式的xml标签文件路径
xml_files1 = r'D:\TestMain\yolov9-main\datasets\fish\Annotations'
# 3、转化为yolo格式的txt标签文件存储路径
save_txt_files1 = r'D:\TestMain\yolov9-main\datasets\fish\lables'
convert_annotation(xml_files1, save_txt_files1, classes)
json转txt
(xml和json选自己所需)
在datasets(数据文件夹)创建jsontotxt.py用于将json转化为txt文件,复制粘贴即可,需修改转化的类别名称,源文件地址和转化后文件地址(最好使用绝对路径)、
import json
import os
name2id = {'blood': 0} # 标签名称
def convert(img_size, box):
dw = 1. / (img_size[0])
dh = 1. / (img_size[1])
x = (box[0] + box[2]) / 2.0 - 1
y = (box[1] + box[3]) / 2.0 - 1
w = box[2] - box[0]
h = box[3] - box[1]
x = x * dw
w = w * dw
y = y * dh
h = h * dh
return (x, y, w, h)
def decode_json(json_floder_path, json_name):
txt_name = r'D:\TestMain\yolov10\datasets\bfish\lables/' + json_name[0:-5] + '.txt'
# 存放txt的绝对路径
txt_file = open(txt_name, 'w')
json_path = os.path.join(json_floder_path, json_name)
data = json.load(open(json_path, 'r', encoding='gb2312', errors='ignore'))
img_w = data['imageWidth']
img_h = data['imageHeight']
for i in data['shapes']:
label_name = i['label']
if (i['shape_type'] == 'rectangle'):
x1 = int(i['points'][0][0])
y1 = int(i['points'][0][1])
x2 = int(i['points'][1][0])
y2 = int(i['points'][1][1])
bb = (x1, y1, x2, y2)
bbox = convert((img_w, img_h), bb)
txt_file.write(str(name2id[label_name]) + " " + " ".join([str(a) for a in bbox]) + '\n')
if __name__ == "__main__":
json_floder_path = r'D:\TestMain\yolov10\datasets\bfish\json'
# 存放json的文件夹的绝对路径
json_names = os.listdir(json_floder_path)
for json_name in json_names:
decode_json(json_floder_path, json_name)
分组
在datasets(数据文件夹)建立splitDataset.py,用于数据集分组[训练集:验证集:测试集(7:2:1)](可修改),需修改分组数据位置(即ImageSets位置),图片存放位置,txt文件存放位置(最好使用绝对路径)
import os, shutil, random
from tqdm import tqdm
"""
标注文件是yolo格式(txt文件)
训练集:验证集:测试集 (7:2:1)
"""
def split_img(img_path, label_path, split_list):
try:
Data = 'D:/TestMain/yolov9-main/datasets/fish/ImageSets'
# Data是你要将要创建的文件夹路径(路径一定是相对于你当前的这个脚本而言的)
# os.mkdir(Data)
train_img_dir = Data + '/images/train'
val_img_dir = Data + '/images/val'
test_img_dir = Data + '/images/test'
train_label_dir = Data + '/labels/train'
val_label_dir = Data + '/labels/val'
test_label_dir = Data + '/labels/test'
# 创建文件夹
os.makedirs(train_img_dir)
os.makedirs(train_label_dir)
os.makedirs(val_img_dir)
os.makedirs(val_label_dir)
os.makedirs(test_img_dir)
os.makedirs(test_label_dir)
except:
print('文件目录已存在')
train, val, test = split_list
all_img = os.listdir(img_path)
all_img_path = [os.path.join(img_path, img) for img in all_img]
# all_label = os.listdir(label_path)
# all_label_path = [os.path.join(label_path, label) for label in all_label]
train_img = random.sample(all_img_path, int(train * len(all_img_path)))
train_img_copy = [os.path.join(train_img_dir, img.split('\\')[-1]) for img in train_img]
train_label = [toLabelPath(img, label_path) for img in train_img]
train_label_copy = [os.path.join(train_label_dir, label.split('\\')[-1]) for label in train_label]
for i in tqdm(range(len(train_img)), desc='train ', ncols=80, unit='img'):
_copy(train_img[i], train_img_dir)
_copy(train_label[i], train_label_dir)
all_img_path.remove(train_img[i])
val_img = random.sample(all_img_path, int(val / (val + test) * len(all_img_path)))
val_label = [toLabelPath(img, label_path) for img in val_img]
for i in tqdm(range(len(val_img)), desc='val ', ncols=80, unit='img'):
_copy(val_img[i], val_img_dir)
_copy(val_label[i], val_label_dir)
all_img_path.remove(val_img[i])
test_img = all_img_path
test_label = [toLabelPath(img, label_path) for img in test_img]
for i in tqdm(range(len(test_img)), desc='test ', ncols=80, unit='img'):
_copy(test_img[i], test_img_dir)
_copy(test_label[i], test_label_dir)
def _copy(from_path, to_path):
shutil.copy(from_path, to_path)
def toLabelPath(img_path, label_path):
img = img_path.split('\\')[-1]
label = img.split('.jpg')[0] + '.txt'
return os.path.join(label_path, label)
if __name__ == '__main__':
img_path = 'D:\TestMain\yolov9-main\datasets\\fish\images' # 你的图片存放的路径(路径一定是相对于你当前的这个脚本文件而言的)
label_path = 'D:\TestMain\yolov9-main\datasets\\fish\lables' # 你的txt文件存放的路径(路径一定是相对于你当前的这个脚本文件而言的)
split_list = [0.7, 0.2, 0.1] # 数据集划分比例[train:val:test]
split_img(img_path, label_path, split_list)
分组完成
在data文件夹中创建data.yaml文件,需要改train,val,test文件对应的路径(最好使用绝对路径)
#path: D:/TestMain/yolov9-main/datasets/fish # dataset root dir
train: D:\TestMain\yolov9-main\datasets\fish1\ImageSets\images\train # train images (relative to 'path') 118287 images
val: D:\TestMain\yolov9-main\datasets\fish1\ImageSets\images\val # val images (relative to 'path') 5000 images
test: D:\TestMain\yolov9-main\datasets\fish1\ImageSets\images\test # 20288 of 40670 images, submit to https://competitions.codalab.org/competitions/20794
# Classes
names:
0: fish
#放你数据集类型对应的类别,多个就0,1,2,3往后排
8、项目结构(选看,可跳过)
总体项目一览
yolo.py
yolo.py单独运行,给出训练模型的网络
.yaml文件
[-1,1,conv,[64,6,2,2]]
第一个参数为来源层数
第二个参数为卷积次数
第三个参数为卷积名称
第四个参数为卷积[输出通道层数,卷积大小(为ture/false,是否有残差卷积),步长,padding(如为空则和原图一样大)]
输入通道数对应一个卷积有几个通道,有多少卷积对应输出有多少通道
[[-1,4],1,concat,[1]]
第一个参数为来源层数
第二个参数为卷积次数
第三个参数为卷积名称
第四个参数为维度
[-1, 1, nn.Upsample, [None, 2, 'nearest']]
第一个参数为来源层数
第二个参数为卷积次数
第三个参数为卷积名称
第四个参数为[输出图像尺寸,缩放比例,插值方式](其中一个二是必须有一个为none,因为都是对输出图片的大小进行确定,否则起冲突,报错)
[[31, 34, 37, 16, 19, 22], 1, DualDDetect, [nc]]
第一个参数为来源层数
第二个参数为卷积次数
第三个参数为卷积名称
第四个参数为类别,图片标记类别fish标记
9、训练
挑选合适的训练文件和训练模型,本人选择是train_dual.py和yolov9-c.yaml
需修改(最好为绝对路径,多仔细看看)
预训练权重(yolov9-c-converted.pt(权重文件)位置,无可以不填)
模型文件(yolov9-c.yaml(模型文件)位置)
训练图片(data.yaml文件所在位置)
训练轮次(基本为300)
训练所放图片个数(8/16,看自己电脑量力而行)
训练存储地址和名称(可不改)
点击运行,万事大吉
觉得好的就点点赞,点点收藏,有什么意见或者建议都可以提(字码错了也可以说,毕竟经常这样),谢谢