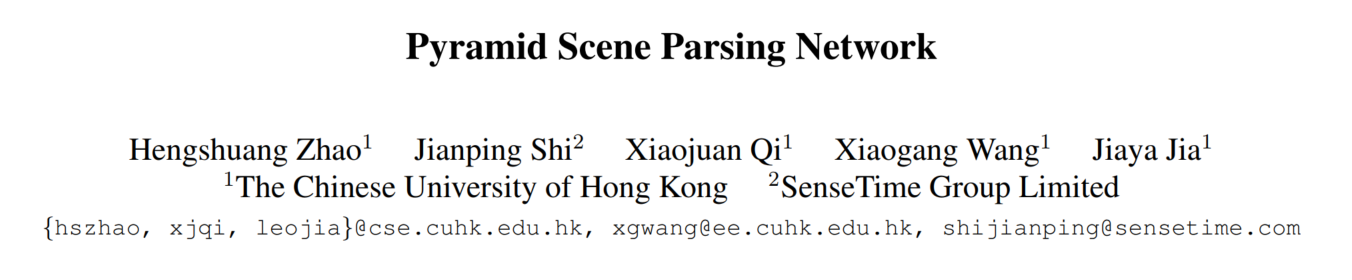
一、主要思想
提出了pyramid pooling module (PPM) 模块,聚合不同区域的上下文信息,从而提高获取全局信息的能力。
现有的深度网络方法中,某一个操作的感受野直接决定了这个操作可以获得多少上下文信息,所以提升感受野可以为网络引入更多的上下文信息。
二、方法
Step1:使用global averag pooling得到不同尺度的特征,PPM模块融合了4个不同尺度的特征:
- 红色是最粗糙尺度,使用一个global average pooling 实现
- 其他的都是将特征图切分为不同数量的块,在每个块内使用global average pooling (文中四个尺度分别是 1x1, 2x2, 3x3, 6x6)
Step2:global average pooling 之后,每层都接一个1x1的卷积来降低通道维度。
Step3:上采样到和原图相同的尺寸,然后和进入PPM头之前的feature map 进行concat 来预测结果。
import torch
import torch.nn as nn
from mmcv.cnn import ConvModule
from mmseg.ops import resize
from ..builder import HEADS
from .decode_head import BaseDecodeHead
from .Attention_layer import HardClassAttention as HCA
class PPM(nn.ModuleList):
"""Pooling Pyramid Module used in PSPNet.
Args:
pool_scales (tuple[int]): Pooling scales used in Pooling Pyramid
Module.
in_channels (int): Input channels.
channels (int): Channels after modules, before conv_seg.
conv_cfg (dict|None): Config of conv layers.
norm_cfg (dict|None): Config of norm layers.
act_cfg (dict): Config of activation layers.
align_corners (bool): align_corners argument of F.interpolate.
"""
def __init__(self, pool_scales, in_channels, channels, conv_cfg, norm_cfg,
act_cfg, align_corners):
super(PPM, self).__init__()
self.pool_scales = pool_scales
self.align_corners = align_corners
self.in_channels = in_channels
self.channels = channels
self.conv_cfg = conv_cfg
self.norm_cfg = norm_cfg
self.act_cfg = act_cfg
for pool_scale in pool_scales:
self.append(
nn.Sequential(
nn.AdaptiveAvgPool2d(pool_scale),
ConvModule(
self.in_channels,
self.channels,
1,
conv_cfg=self.conv_cfg,
norm_cfg=self.norm_cfg,
act_cfg=self.act_cfg)))
def forward(self, x):
"""Forward function."""
ppm_outs = []
for ppm in self:
ppm_out = ppm(x)
upsampled_ppm_out = resize(
ppm_out,
size=x.size()[2:],
mode='bilinear',
align_corners=self.align_corners)
ppm_outs.append(upsampled_ppm_out)
return ppm_outs
@HEADS.register_module()
class PSPHead(BaseDecodeHead):
"""Pyramid Scene Parsing Network.
This head is the implementation of
`PSPNet <https://arxiv.org/abs/1612.01105>`_.
Args:
pool_scales (tuple[int]): Pooling scales used in Pooling Pyramid
Module. Default: (1, 2, 3, 6).
"""
def __init__(self, pool_scales=(1, 2, 3, 6), **kwargs):
super(PSPHead, self).__init__(**kwargs)
assert isinstance(pool_scales, (list, tuple))
self.pool_scales = pool_scales
self.psp_modules = PPM(
self.pool_scales,
self.in_channels,
self.channels,
conv_cfg=self.conv_cfg,
norm_cfg=self.norm_cfg,
act_cfg=self.act_cfg,
align_corners=self.align_corners)
self.bottleneck = ConvModule(
self.in_channels + len(pool_scales) * self.channels,
self.channels,
3,
padding=1,
conv_cfg=self.conv_cfg,
norm_cfg=self.norm_cfg,
act_cfg=self.act_cfg)
def forward(self, inputs):
"""Forward function."""
# inputs [4, 512, 64, 128]
x = self._transform_inputs(inputs) #[4, 2048, 64, 128]
psp_outs = [x] # list, len=1, psp_outs[0].shape = [4, 2048, 64, 128]
# self.psp_models(x), list, len=4
psp_outs.extend(self.psp_modules(x)) # len(psp_outs) = 5, psp_out[1-4].shape = [4, 512, 64, 128]
psp_outs = torch.cat(psp_outs, dim=1) # [4, 4096, 64, 128]
output = self.bottleneck(psp_outs) # [4, 512, 64, 128]
output = self.cls_seg(output) # [4, 19, 64, 128]
# import pdb; pdb.set_trace()
return output