import torch.onnx
from CMUNet import CMUNet_new
#Function to Convert to ONNX
import torch import torch.nn as nn import torchvision as tv
def Convert_ONNX(model,save_model_path):
# set the model to inference mode
model.eval()
# Let's create a dummy input tensor
input_shape = (1, 400, 400) # 输入数据,改成自己的输入shape
dummy_input = torch.randn(1,*input_shape)
# Export the model
torch.onnx.export(model, # model being run
dummy_input, # model input (or a tuple for multiple inputs)
save_model_path+".onnx", # where to save the model
)
print('Model has been converted to ONNX')
class DummyModule(nn.Module):
def __init__(self):
super(DummyModule, self).__init__()
def forward(self, x):
return x
def fuse(conv, bn):
w = conv.weight
mean = bn.running_mean
var_sqrt = torch.sqrt(bn.running_var + bn.eps)
gamma = bn.weight
beta = bn.bias
if conv.bias is not None:
b = conv.bias
else:
b = mean.new_zeros(mean.shape)
w = w * (gamma / var_sqrt).reshape([conv.out_channels, 1, 1, 1])
b = (b - mean) / var_sqrt * gamma + beta
fused_conv = nn.Conv2d(conv.in_channels,
conv.out_channels,
conv.kernel_size,
conv.stride,
conv.padding,
bias=True)
fused_conv.weight = nn.Parameter(w)
fused_conv.bias = nn.Parameter(b)
return fused_conv
def fuse_conv_and_bn(conv, bn):
# init
fused_conv = torch.nn.Conv2d(
conv.in_channels,
conv.out_channels,
kernel_size=conv.kernel_size,
stride=conv.stride,
padding=conv.padding,
bias=True
)
# # prepare filters
w_conv = conv.weight.clone().view(conv.out_channels, -1)
w_bn = torch.diag(bn.weight.div(torch.sqrt(bn.eps + bn.running_var)))
fused_conv.weight = nn.Parameter(torch.mm(w_bn, w_conv).view(fused_conv.weight.size()))
# # prepare spatial bias
if conv.bias is not None:
b_conv = conv.bias
else:
b_conv = torch.zeros(conv.weight.size(0))
b_bn = bn.bias - bn.weight.mul(bn.running_mean).div(torch.sqrt(bn.running_var + bn.eps))
fused_conv.bias = nn.Parameter(torch.matmul(w_bn, b_conv) + b_bn)
return fused_conv
def fuse_module(m):
children = list(m.named_children())
# print("***********")
# print(children)
# print("***********")
c = None
cn = None
for name, child in children:
if isinstance(child, nn.BatchNorm2d):
# bc = fuse(c, child)
bc = fuse_conv_and_bn(c, child)
m._modules[cn] = bc
m._modules[name] = DummyModule()
# print("==> name: ", name)
c = None
elif isinstance(child, nn.Conv2d):
c = child
cn = name
else:
fuse_module(child)
def test_net(m):
fuse_module(m)
Convert_ONNX(m,"our_model")
if __name__ == "__main__":
net= tv.models.resnet18(True)
net.eval()
# print("Layer level test: ")
# test_layer()
print("============================")
print("Module level test: ")
test_net(net)
Pytorch合并Conv和BN并转onnx
最新推荐文章于 2023-10-10 18:10:58 发布
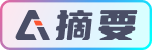