论文名称:《A Baseline for 3D Multi-Object Tracking》(AB3DT)
论文地址:https://arxiv.org/pdf/1907.03961.pdf
Github项目地址:https://github.com/xinshuoweng/AB3DMOT
这是一篇CMU还在提交过程中的论文,代码已经开源。在我看来这就是一个3D版本的SORT算法,都用最简原则,组合了一些常规方法,构造了一个简单、精确、实时的3D MOT系统。它最强悍之处就是精度高且速度非常快!只需要CPU,不需要训练直接使用!(除了detector需要单独的预训练)。流程图总结一下就是这么简单,使用的是现成的3D detector,不过将卡尔曼滤波的状态空间拓展到3维算是一个创新。
摘要:
- real-time:近期3D MOT方向的论文都专注于通过更复杂的系统设计来达到更高精度,却不怎么重视计算负担和速度。但在实际应用中,速度是非常重要的衡量指标。这篇论文提出的3D MOT模型速度一骑绝尘,达到 215.7FPS,比SOTA的2D MOT模型要快65倍。
- SOTA:不仅速度快,它的模型性能也很好。在KITTI数据集中,它在3D评价指标(本论文提出的)上达到SOTA;将3D结果投影到2D后,在KITTI标准2D评价指标上位列第二。
- 将卡尔曼滤波的状态空间从2D拓展到3D。
- 3D MOT评价指标优化:将官方KITTI 2D MOT的评价指标拓展到3D,并提出了两个新的准则AMOTA/AMOTP。KITTI-3DMOT evaluation tool 已经开源。
文章目录
1 Introduction
先来感受一下这个模型的威力:
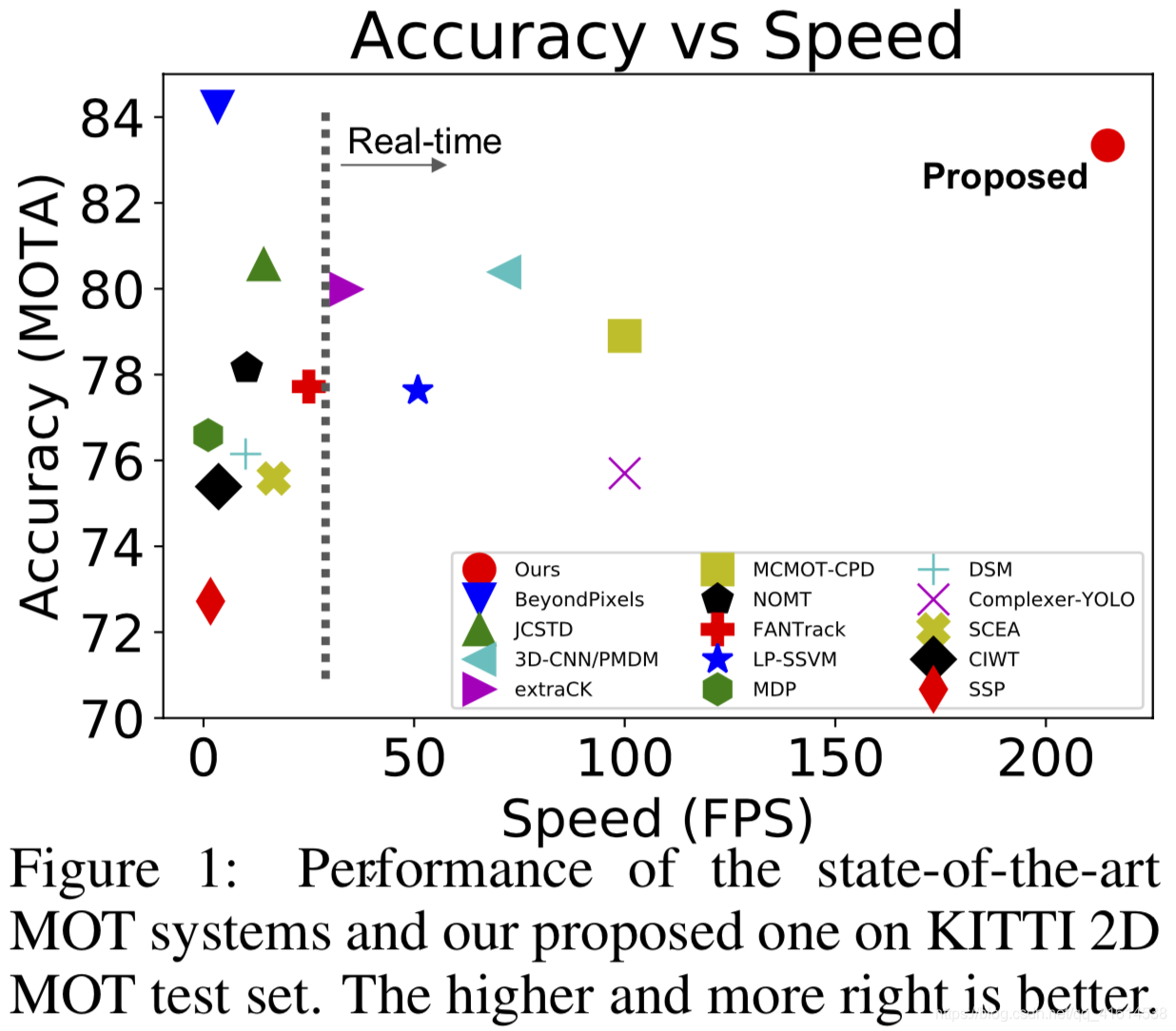
没错,右上角的红点就是AB3DMOT,本文提出的模型。保持高精度的前提下,速度远超其它算法。其它一些特点和贡献在上面摘要中都有提及,不再赘述。
2 Related Works
2D MOT
类型 | 匹配问题建模 | 算法 |
---|---|---|
batch | 网络流图 | 最小费用流 |
online | 二部图 | 匈牙利算法 / 深度匹配神经网络 |
本模型属于online模型,为了简单高速,采用原始匈牙利算法,并且只使用最简单的运动估计模型(匀速),不使用外观模型。
3D MOT
大部分3D MOT系统和2D MOT系统的模型框架是一致的,只不过是检测框从2D变成了3D。这使得我们可以利用3D空间中的信息来对运动模型、外观模型进行建模,不会受透视变形的影响。简单起见,我们的模型只使用了原始卡尔曼滤波,但将状态空间拓展到三维,包括3D速度、3D大小、3D位置以及目标的朝向角度。
3D Object Detection
和2D MOT一样,检测质量可以很大程度上影响整个追踪的质量。本论文中,我们用两个不同的检测器,分别进行实验
。结果可见后面的对照实验。
之前有不少论文都关注于基于雷达点云进行3D bbox的检测:
PointRCNN: 3D Object Proposal Generation and Detection from Point Cloud. CVPR, 2019.
实验
Real Time End-to-End 3D Detection, Tracking and Motion Forecasting with a Single Convolutional Net. CVPR, 2018.
End-to-End Learning for Point Cloud Based 3D Object Detection. CVPR, 2018.
HDNET: Exploiting HD Maps for 3D Object Detection. CoRL, 2018.
当然也有直接基于单张图片进行3D检测的论文:
Monocular 3D Object Detection with Pseudo-LiDAR Point Cloud. 2019
实验
Monocular 3D Object Detection Leveraging Accurate Proposals and Shape Reconstruction. CVPR, 2019.
3 Approach
系统整体架构如上图所示,主要分以下几步:
- 检测当前帧的3D bbox
- 3D卡尔曼滤波器 通过前一帧的目标状态 预测 当前帧的目标状态
- 用匈牙利算法对检测结果和目标预测状态进行匹配
- 用匹配上的检测结果去更新目标状态
- 没有被匹配上的检测结果将用于生成新目标,没有匹配上的目标预测状态将被删除
3.1 3D Object Detection
我们直接使用现成的3D detector,它们的原理请自行学习相应论文。
对于每一帧 t t t,3D detector的输出应该是一系列检测结果 D t = { D t 1 , D t 2 , ⋯ , D t n t } D_{t}=\left\{D_{t}^{1}, D_{t}^{2}, \cdots, D_{t}^{n_{t}}\right\} Dt={
Dt1,Dt2,⋯,Dtnt},其中 n t n_t nt是检测到的物体的数量,在不同帧很可能是不同的值。每一个检测结果 D t i D_t^i Dti是一个八元组信息 ( x , y , z , l , w , h , θ , s ) (x, y, z, l, w, h, \theta, s) (x,y,z,l,w,h,θ,s)。其中 ( x , y , z ) (x,y,z) (x,y,z)是物体中心的三维坐标, ( l , w , h ) (l,w,h) (l,w,h)是物体的长宽高尺寸, θ \theta θ是朝向角, s s s是置信度。
3.2 3D Kalman Filter —– State Prediction
采用匀速模型,独立于相机的自身运动。
我们将目标轨迹状态建模为10维的向量 T = ( x , y , z , θ , l , w , h , v x , v y , v z ) T=\left(x, y, z, \theta, l, w, h, v_{x}, v_{y}, v_{z}\right) T=(x,y,z,θ,l,w,h,vx,vy,vz),其中 v x , v y , v z v_{x}, v_{y}, v_{z} vx