肺腺癌基因19突变 预后
凯拉斯vs法泰 (Keras vs fastai)
Machine learning (ML) has the potential for numerous applications in the health care field. One promising application is in the area of anatomic pathology. ML allows representative images to be used to train a computer to recognize patterns from labeled photographs. Based on a set of images selected to represent a specific tissue or disease process, the computer can be trained to evaluate and recognize new and unique images from patients and render a diagnosis.
机器学习(ML)在医疗保健领域具有众多应用潜力。 一种有希望的应用是解剖病理学领域。 ML允许使用代表性图像训练计算机来识别带有标签的照片中的图案。 基于选择的代表特定组织或疾病过程的一组图像,可以训练计算机以评估和识别来自患者的新的独特图像并进行诊断。
Lung and colon adenocarcinoma are some of the most common cancers affecting numerous patients throughout the world. They frequently spread to other sites of the body. It is not uncommon that the pathologist is faced with the question if biopsy showing adenocarcinoma originated from lung or colon primary site. Pathologists are often forced to use special stains to help them make this determination. In this post, I used two different machine learning libraries (fastai and Keras) to solve the origin of metastatic adenocarcinoma.
肺癌和结肠腺癌是影响世界各地众多患者的最常见癌症。 它们经常扩散到身体的其他部位。 病理学家面临的问题是活检显示腺癌是否起源于肺部或结肠原发部位并不少见。 病理学家常常被迫使用特殊的污渍来帮助他们做出这一决定。 在本文中,我使用了两个不同的机器学习库(fastai和Keras)来解决转移性腺癌的起源。
For this project, I used an image dataset containing 5000 color images of lung adenocarcinoma and 5000 color images of colon adenocarcinoma from the LC25000 dataset, which is freely available for ML researchers. I created a data folder with two subfolders for each class, lung adenocarcinoma class, and colon adenocarcinoma class.
对于这个项目,我使用了一个图像数据集,其中包含来自LC25000数据集的5000例肺腺癌彩色图像和5000例结肠腺癌彩色图像,这对于ML研究人员是免费的。 我为每个类别(肺腺癌类别和结肠腺癌类别)创建了一个包含两个子文件夹的数据文件夹。
My goal was not only to solve the metastatic adenocarcinoma classification problem but also to compare two deep learning libraries : Keras and fastai.
我的目标是不仅要解决转移性腺癌分类问题也比较两个深度学习库: Keras和fastai 。
Both fastai and Keras are high-level APIs build on the top of PyTorch and TensorFlow, respectively. Keras was fully integrated into version 2 of TensorFlow.
fastai和Keras都是分别在PyTorch和TensorFlow之上构建的高级API。 Keras已完全集成到TensorFlow的版本2中。
Following seven coding steps were used to solve the problem of metastatic adenocarcinoma classification:
以下七个编码步骤用于解决转移性腺癌分类问题:
- Importing relevant libraries 导入相关库
- Specifying the path to the data directory 指定数据目录的路径
- Preparing batches of tensor image data for ML model 为ML模型准备一批张量图像数据
- Visualizing samples of training images (optional) 可视化训练图像样本(可选)
- Creating ML model 创建ML模型
- Fitting ML model 拟合ML模型
- Visualizing results with confusion matrix 使用混淆矩阵可视化结果
The Jupyter Notebooks for this post are available on my GitHub website.
这篇文章的Jupyter Notebooks可在我的GitHub网站上找到。
Let us start with the fastai. The first step is to import relevant libraries.
让我们从fastai开始。 第一步是导入相关库。

Next, we specify the path to the directory with our images.
接下来,我们使用图像指定目录的路径。

We create ImageDataBuch object with following parameters: the path to the data directory, validation split (in our case we pick 20% of images for validation and 80% for training), augmentation (False in our case since the LC25000 images are already augmented), image size (299x299 pixels is the native size for ResNet50) and batch size (I found 48 to be a right batch size for my 11 GB 2080Ti video card running fastai ResNet50 code on my Linux machine).
我们使用以下参数创建ImageDataBuch对象:数据目录的路径,验证拆分(在我们的示例中,我们选择20%的图像进行验证,在80%的图像中进行训练),扩充(在我们的示例中为False,因为LC25000图像已经被扩充) ,图片大小(ResNet50的原始大小为299x299像素)和批处理大小(我发现我的Linux计算机上运行fastai ResNet50代码的11 GB 2080Ti视频卡的正确批处理大小为48)。

We visualize some of the images from the training batch with single line of code.
我们用单行代码可视化训练批次中的某些图像。

Here we create our ML model with the following parameters: data object, the model for transfer learning (ResNet50 in this case), and metrics (I pick the accuracy).
在这里,我们使用以下参数创建ML模型:数据对象,用于转移学习的模型(在这种情况下为ResNet50)和指标(我选择准确性)。

Fastai automatically freezes the ResNet50 classifier layer and replaces it with a fastai code. Below is the summary of the fastai classifier. The whole model has approximately 25 million trainable parameters.
Fastai自动冻结ResNet50分类器层,并将其替换为fastai代码。 以下是fastai分类器的摘要。 整个模型具有约2500万个可训练参数。

Next, we fit and train the model. After only five epochs our model achieved 100% training accuracy
接下来,我们拟合并训练模型。 仅经过5个时期,我们的模型即可达到100%的训练精度

With only two lines of code, we visualize the confusion matrix of the validation dataset.
仅用两行代码,我们就能看到验证数据集的混淆矩阵。

Next, let’s move to Keras code. We will use TensorFlow 2 as a backend. As you can see already, to achieve the same results as with fastai, we need many more lines of code. First we import relevant libraries.
接下来,让我们转到Keras代码。 我们将使用TensorFlow 2作为后端。 正如您已经看到的,要获得与fastai相同的结果,我们需要更多的代码行。 首先,我们导入相关的库。

We specify the path to our data directory.
我们指定数据目录的路径。

We create ImageDataGenerator object with two parameters: validation split (20% images for validation and 80% for training) and normalization of images. We create two data objects: one for training and one for validation. We specify image size, batch size (had to pick the batch size of 32, my video card could not handle 48 with tf.keras version of the classifier). Since we don’t want to shuffle our validation set, we set shuffle to False.
我们使用两个参数创建ImageDataGenerator对象:验证分割(验证时使用20%的图像,训练时使用80%的图像)和图像的标准化。 我们创建两个数据对象:一个用于训练,一个用于验证。 我们指定图像大小,批处理大小(必须选择32的批处理大小,我的视频卡使用tf.keras版本的分类器无法处理48)。 由于我们不想改组验证集,因此将shuffle设置为False。

We visualize some of the images from the training batch with the following lines of code.
我们使用以下代码行可视化训练批次中的某些图像。

We create our ML model. We freeze the classifier layer of the ResNet50 model and create our classifier (I tried to replicate the fastai classifier as much as I could; however, Keras does not have AdaptiveAvgPool2d layer, so I used global_max_pooling2d instead).
我们创建我们的ML模型。 我们冻结ResNet50模型的分类器层并创建我们的分类器(我尝试了尽可能多的复制fastai分类器;但是,Keras没有AdaptiveAvgPool2d层,所以我改用global_max_pooling2d)。

The whole model has approximately 25 million trainable parameters, just like fastai model.
就像fastai模型一样,整个模型具有大约2500万个可训练参数。
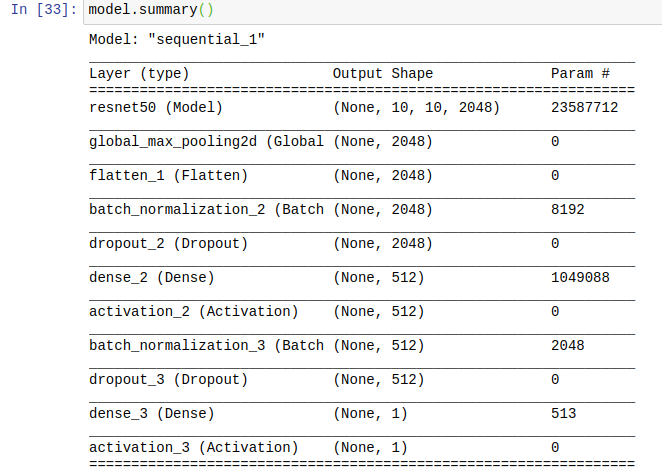
We compile, fit, and train the ML model. After five epochs, our Keras model achieved 98% training accuracy.
我们编译,拟合和训练ML模型。 经过五个纪元,我们的Keras模型达到了98%的训练准确性。

With few lines of code, we visualize the confusion matrix of the validation dataset.
用几行代码,我们就能看到验证数据集的混淆矩阵。

Conclusion
结论
After only five epochs of training, both models achieved high training accuracy. The fastai required fewer lines of code to accomplish the same goals. Although both deep learning libraries are great for new practitioners of machine learning craft, the fastai library is more straightforward and user friendly. The fastai library has a great community of users, and you can find just about any answer on users’ forums. Ultimately, it is a personal preference as to which deep learning library to use.
仅经过五个时期的训练,两个模型均达到了很高的训练精度。 Fastai需要更少的代码行来实现相同的目标。 尽管这两个深度学习库都非常适合机器学习新手,但fastai库更简单易用。 fastai库拥有大量的用户社区,您可以在用户论坛上找到几乎所有答案。 最终,对于使用哪个深度学习库,这是个人喜好。
Note: All fastai code is written in version 1 of the fastai library. Version 2 of the fastai library is officially being released today (8/24/2020).
注意:所有fastai代码均使用fastai库的版本1编写。 fastai库的第2版今天(2020年8月24日)正式发布。
Best wishes to everyone in these difficult times. Stay healthy and safe!Andrew @tampapath
在这些困难时期向所有人致以最良好的祝愿。 保持健康安全!Andrew @tampapath
肺腺癌基因19突变 预后