1写在前面
当完成了对scRNAseq
数据的Normalization
和混杂因素
去除后,我们就可以开始正式分析了。😘
本期我们介绍一下常用的聚类
方法(clustering
),主要是无监督聚类,包括:👇
-
hierarchical clustering
; -
k-means clustering
; -
graph-based clustering
。
1.1 hierarchical clustering
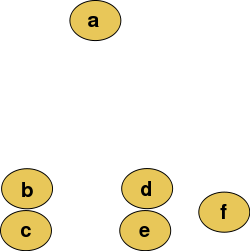
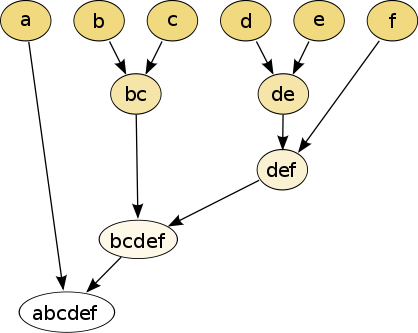
1.2 k-means clustering
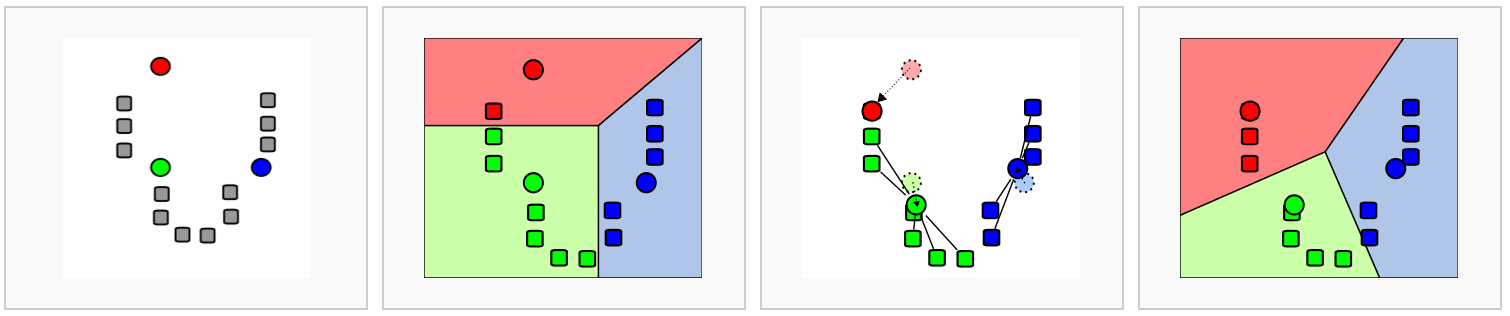
1.3 graph-based clustering
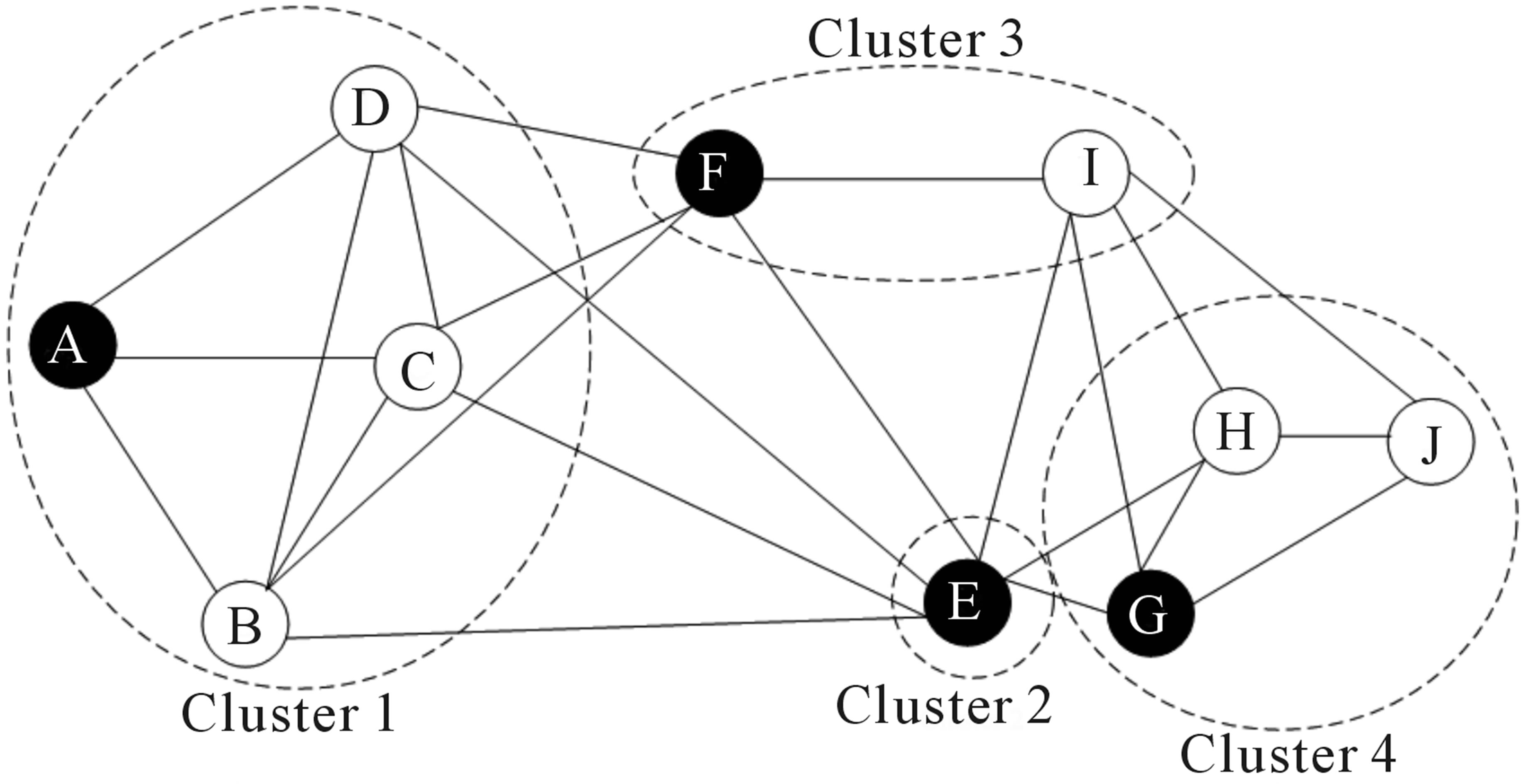
2聚类工具
2.1 SINCERA
-
基于 hierarchical clustering
; -
在聚类前需要进行 z-scores
转换。
2.2 SC3
-
基于 PCA
降维; -
k-means
; -
共识聚类( consensus clustering
)。🌟
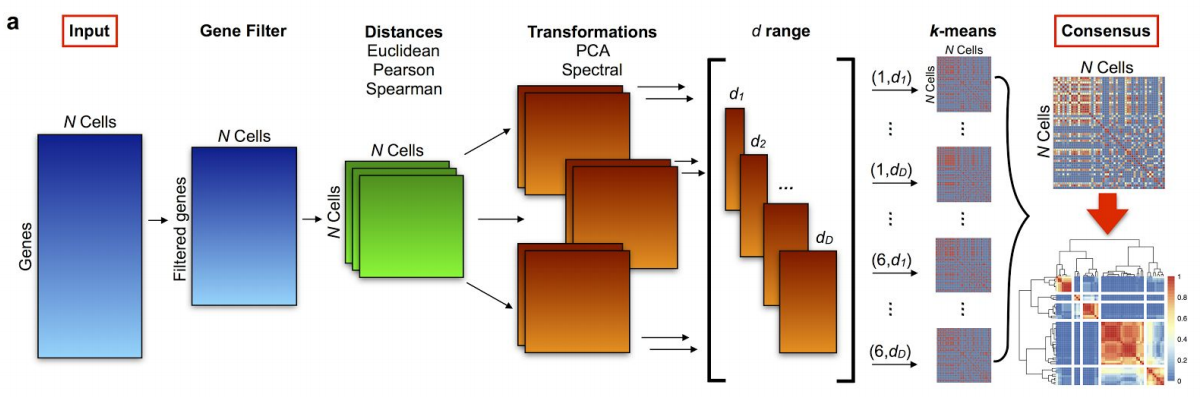
2.3 tSNE + k-means
-
tSNE maps
; -
k-means
。
2.4 Seurat clustering
Seurat clustering
主要是基于community
的识别进行聚类,这里我们不做具体介绍了,后面会做Seurat
包的详细教程。🤩
2.5 Comparing clustering
当我们需要比较两个聚类结果的时候,我们可以使用adjusted Rand index
,区间在0~1
,大家可以简单理解为,1
表示聚类相同,0
表示偶然相似
,即adjusted Rand index
越大,聚类结果越相似。🤗
3用到的包
rm(list = ls())
library(pcaMethods)
library(SC3)
library(scater)
library(SingleCellExperiment)
library(pheatmap)
library(mclust)
library(ggsci)
4示例数据
这里我为大家准备了一个小鼠的胚胎scRNAseq
数据,文件格式为.rds
。
dat <- readRDS("./deng-reads.rds")
dat
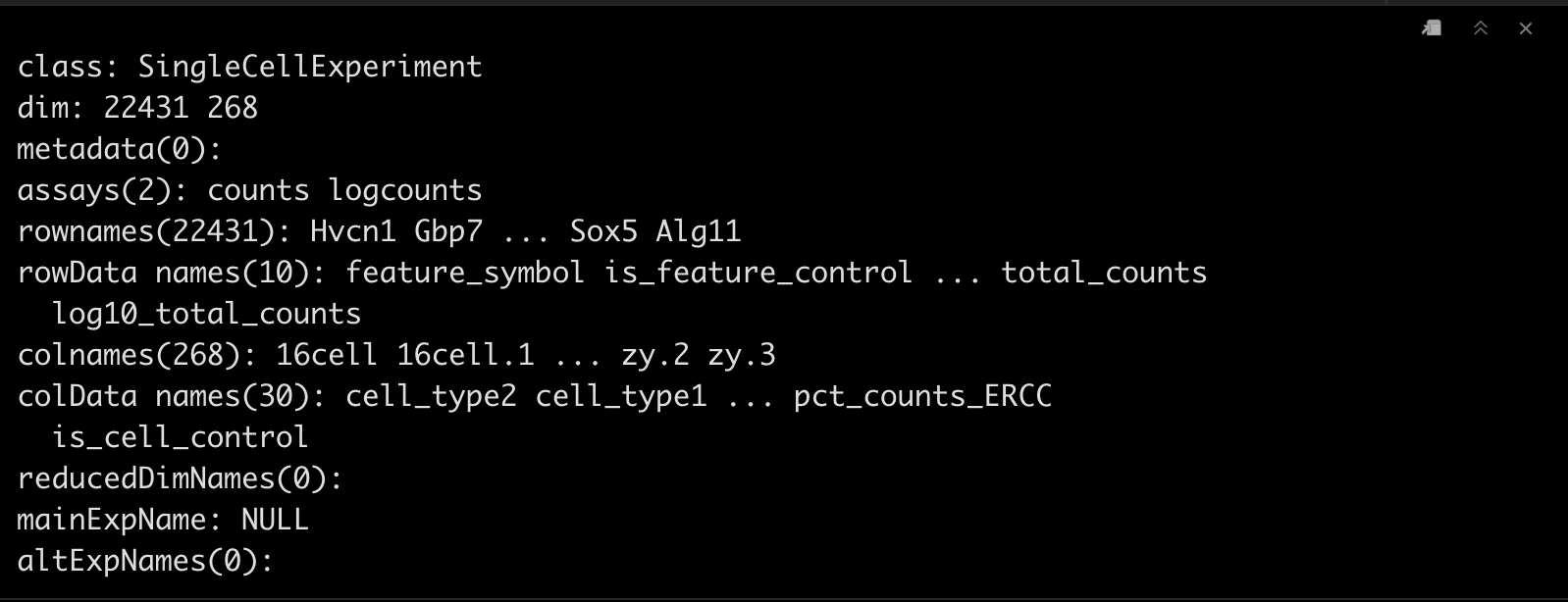
5数据初探
我们先看下细胞类型。
table(colData(dat)$cell_type2)

PCA 🥳 这里看到有的细胞类型是分的很开的,有明显的区分。
dat <- runPCA(dat)
plotPCA(dat, colour_by = "cell_type2")+
scale_fill_npg()+
scale_color_npg()
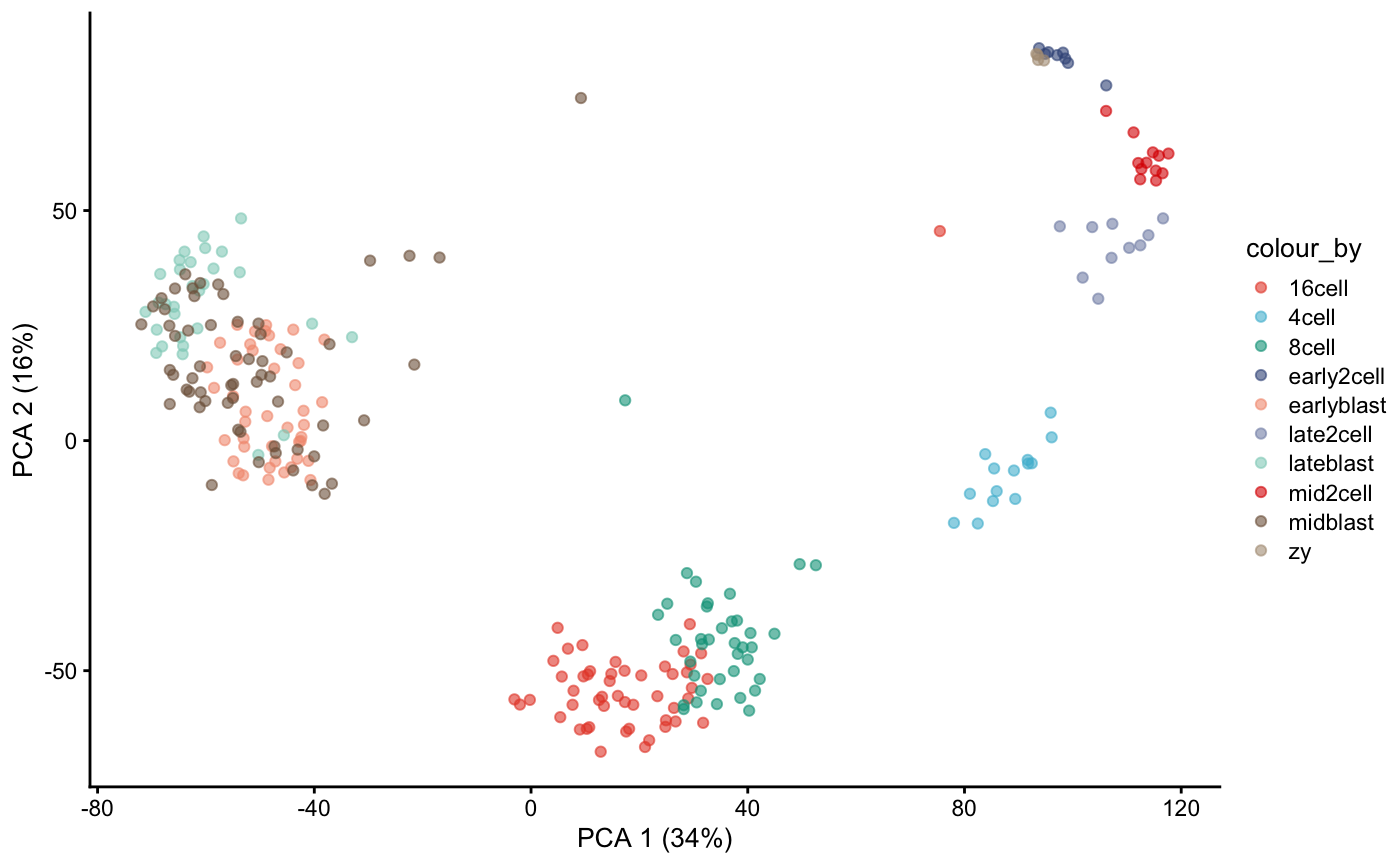
6SC3
这里我们只介绍一下SC3
的方法进行聚类,其他方法耗时过长。
SC3
的输入数据直接是SingleCellExperiment
,非常方便。😁
6.1 sc3_estimate_k
dat <- sc3_estimate_k(dat)
6.2 查看聚类结果
我们看一下sc3
提供的聚类方法,帮我们聚成了几类~
metadata(dat)$sc3$k_estimation

Note! 这里只帮我们聚了6
类,但我们实际上不只6
类啊。🫠
但是, 如果我们不考虑early
, mid
, late
这种时间点的话,正好的6
类。Nice !~🥳
6.3 可视化一下
plotPCA(dat, colour_by = "cell_type1")+
scale_fill_npg()+
scale_color_npg()
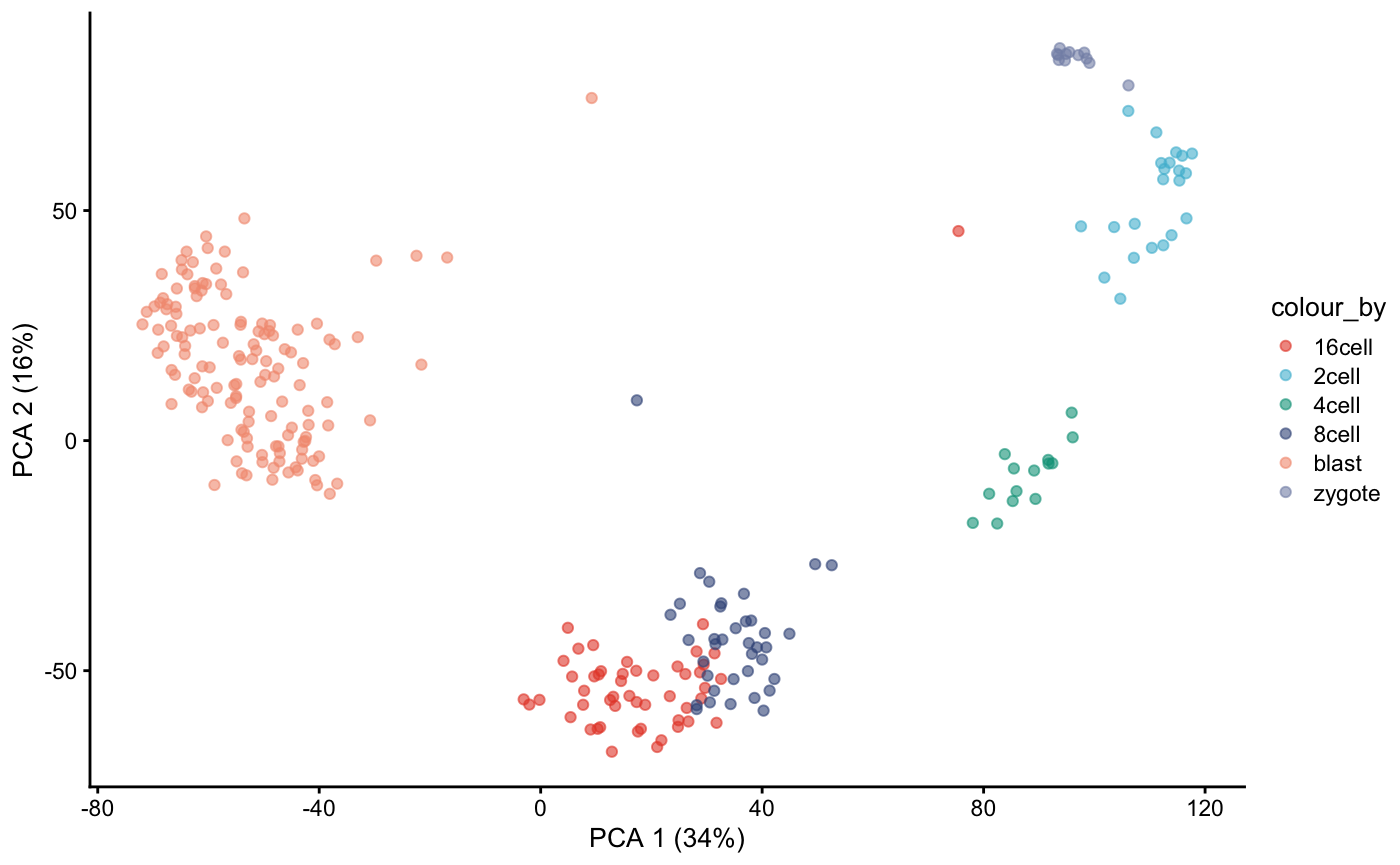
6.4 生物学分组聚类
这里我们将生物学分组纳入考虑中,进行聚类。
dat <- sc3(dat,
ks = 10, # a range/single of the number of clusters k used for clustering
biology = TRUE,
n_cores = 4 # 默认是1
)
6.5 通过Shiny结果可视化
SC
包提供了一种交互的方式进行结果展示,就和网页工具一样简单。
sc3_interactive(dat)
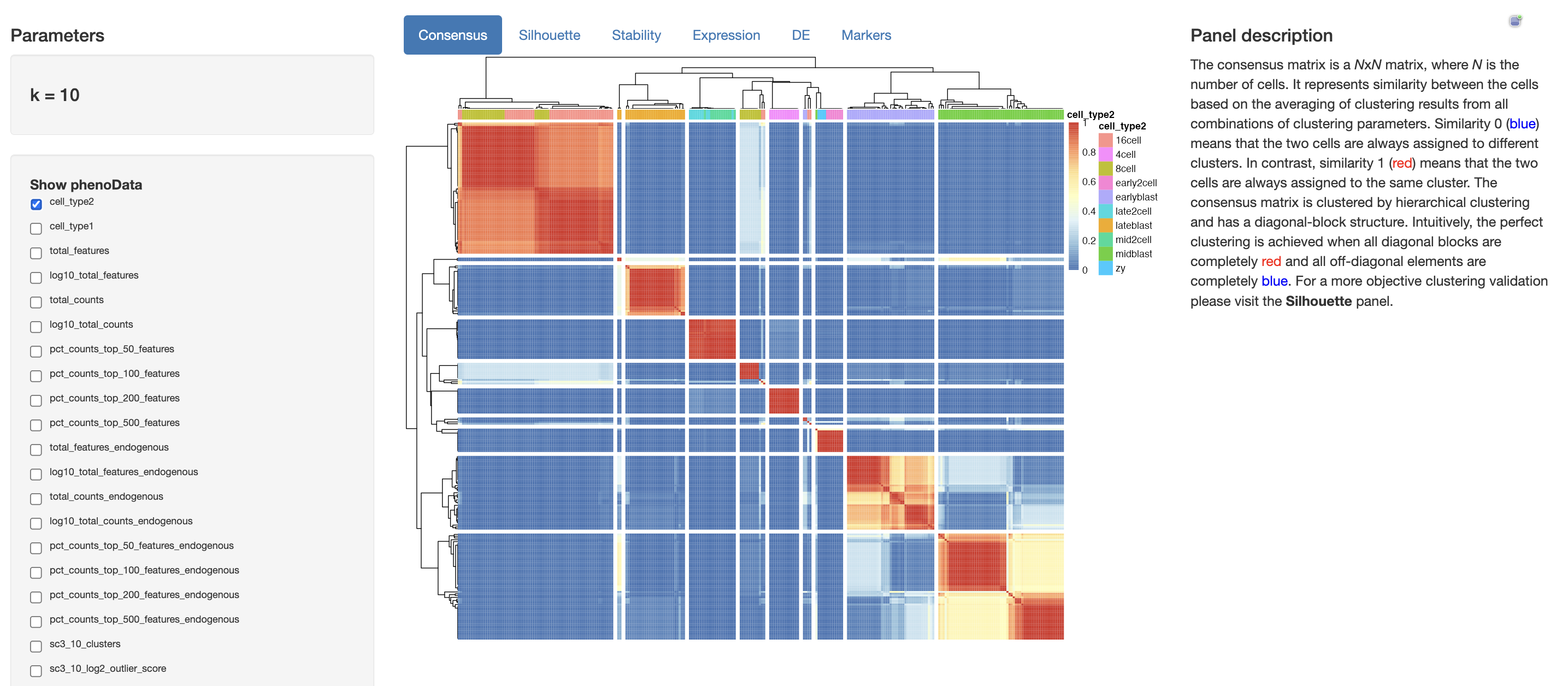
🤨 这里我们只讲一下如何使用代码实现结果可视化。
6.6 Consensus Matrix
sc3_plot_consensus(
dat,
k = 10,
show_pdata = c(
"cell_type2")
)
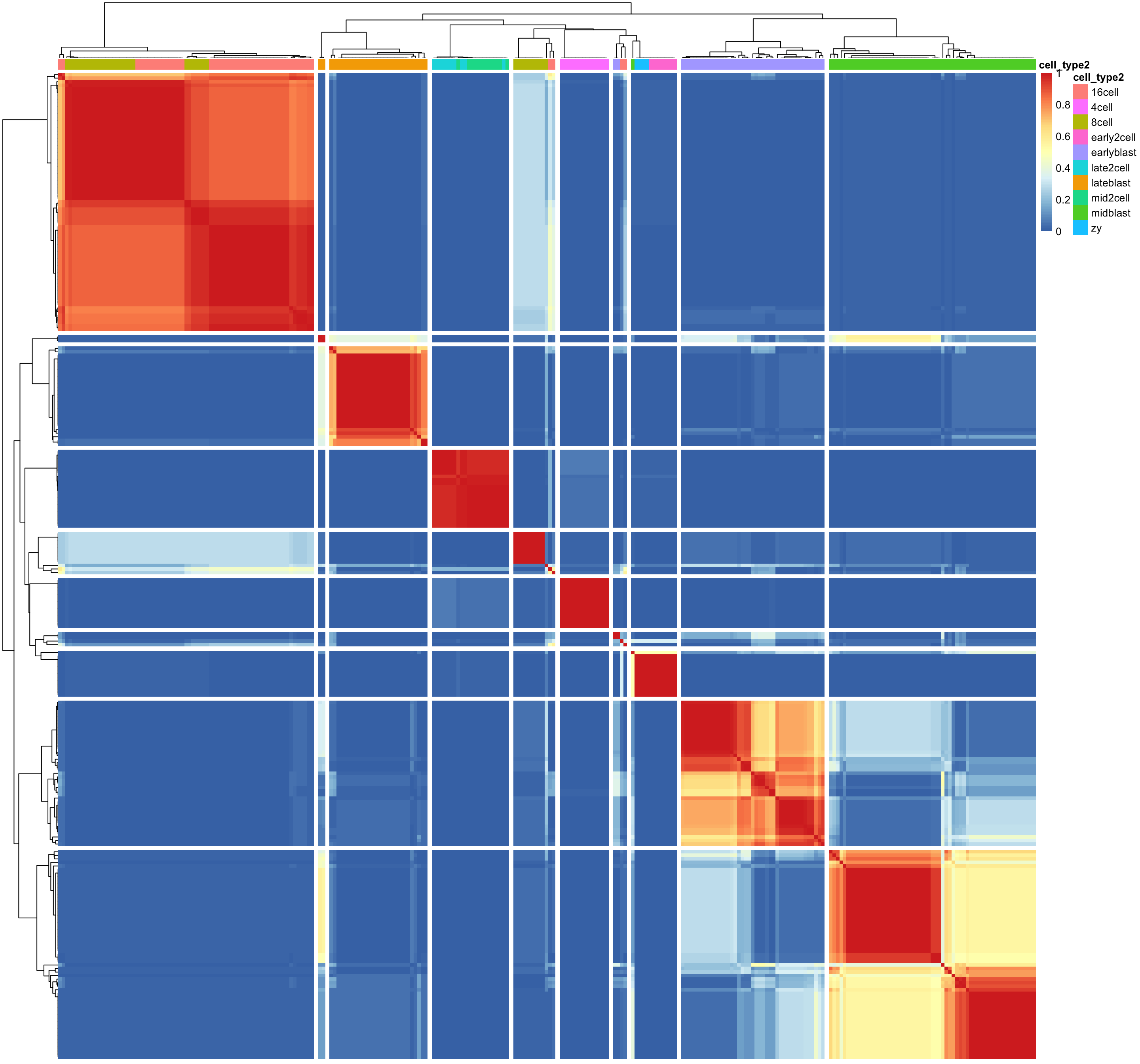
6.7 Silhouette Plot
sc3_plot_silhouette(dat, k = 10)
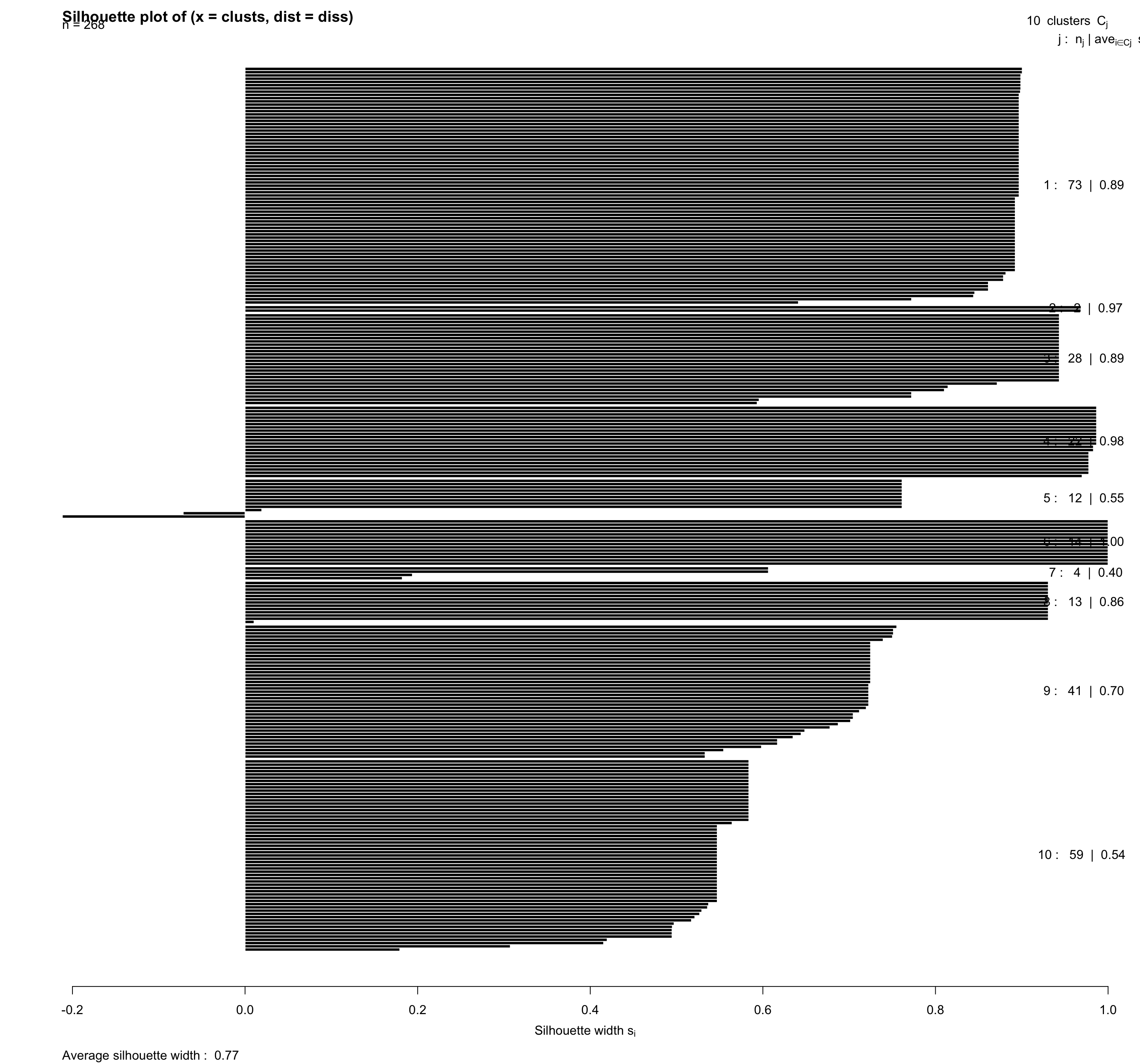
6.8 Expression Matrix
sc3_plot_expression(
dat, k = 10,
show_pdata = c(
"cell_type2")
)
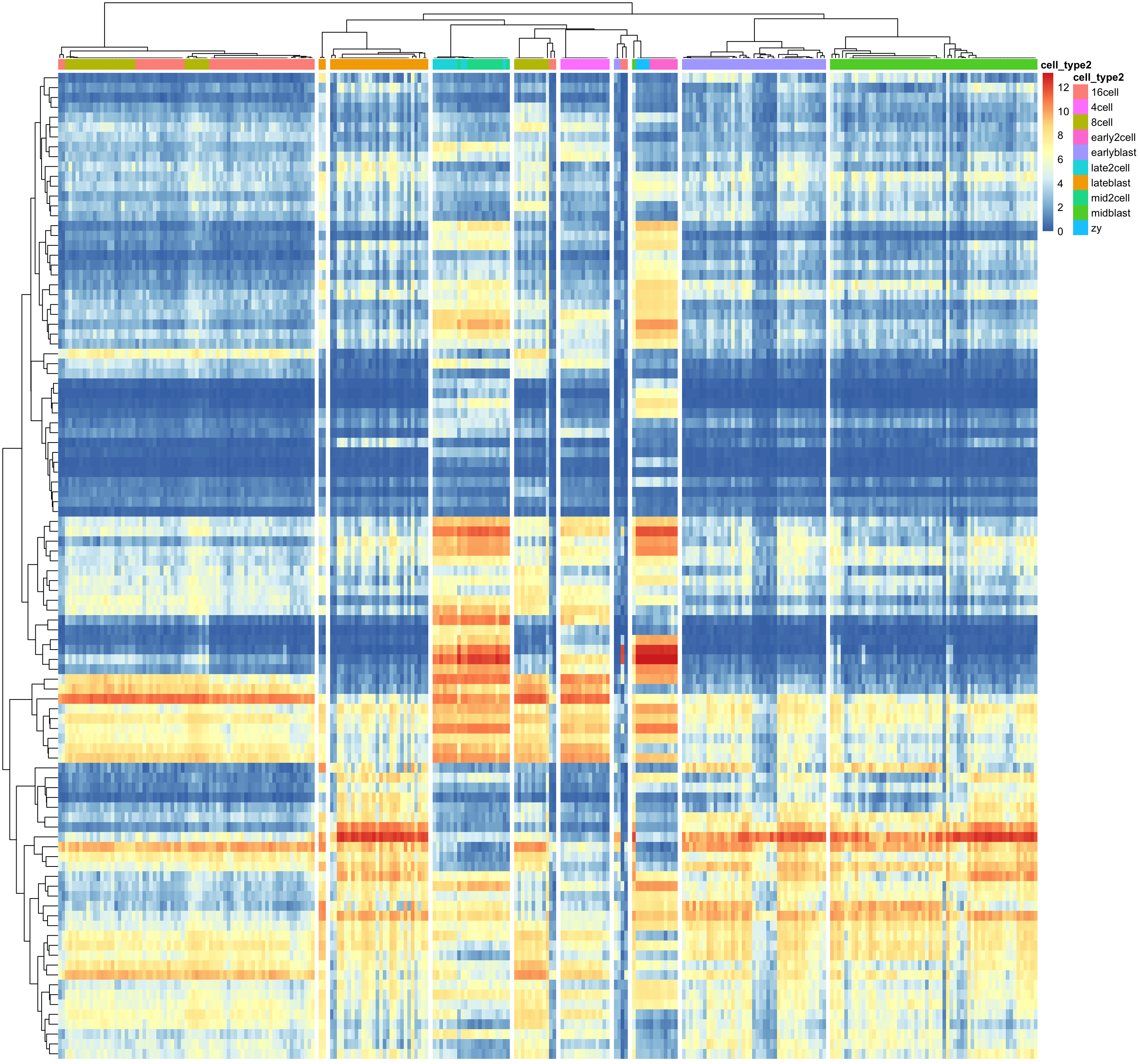
6.9 DE genes
sc3_plot_de_genes(
dat, k = 10,
show_pdata = c(
"cell_type2")
)
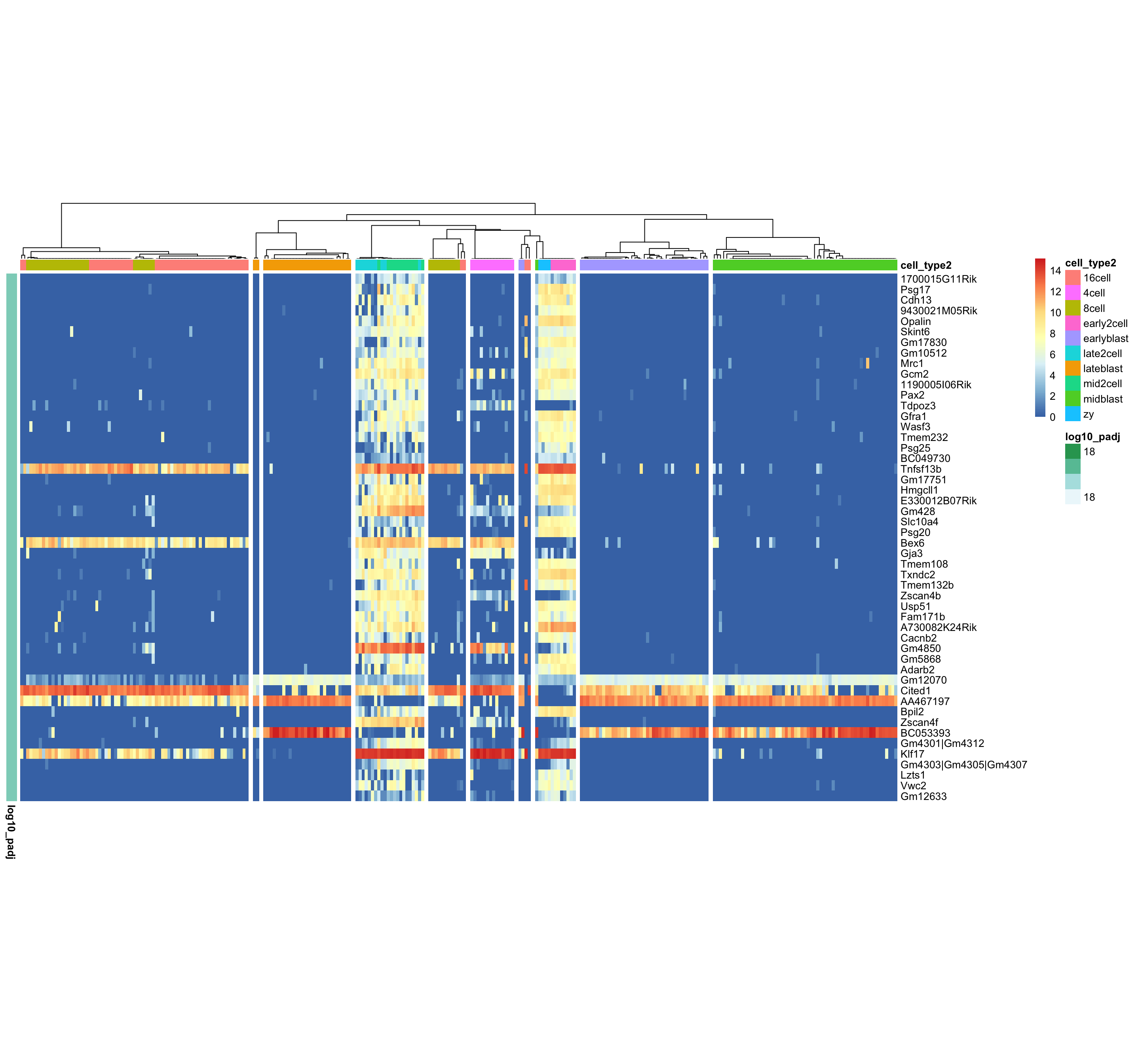
6.10 Marker Genes
sc3_plot_markers(
dat, k = 10,
show_pdata = c(
"cell_type2")
)
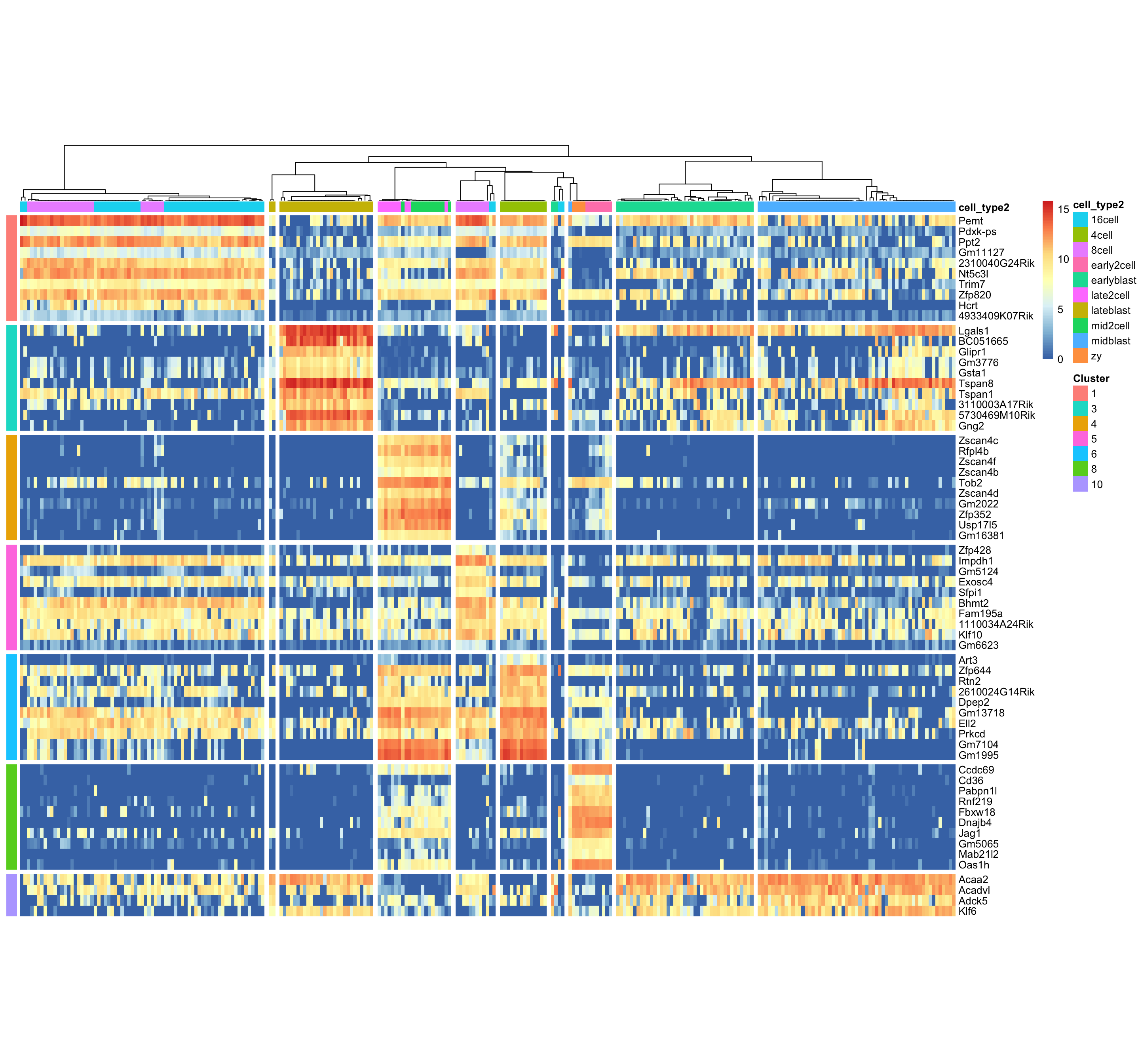
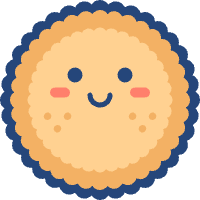
需要示例数据的小伙伴,在公众号回复
scRNAseq2
获取吧!点个在看吧各位~ ✐.ɴɪᴄᴇ ᴅᴀʏ 〰
本文由 mdnice 多平台发布