1写在前面
临床工作越来越忙了,更新的频率也开始降低了,希望各位小伙伴可以理解一下。😭
最近看到塞尔达-王国之泪
发售了,真的是想买一个,但想想根本没有时间去玩,买回来只能吃灰。🥲
想问问小伙伴们有什么想看的教程吗,可以给我留言,如果合适的话可以做上几期。🧐
OK,今天的教程是超大数据集的网络构建与模块识别,当然如果你的电脑或者服务器够好的话,可以跳过这期。🥳
2用到的包
rm(list = ls())
library(tidyverse)
library(WGCNA)
3示例数据
我们还是和之前一样,把之前清洗好的输入数据拿出来吧。😗
load("./Consensus-dataInput.RData")
4提取数据集个数
首先和之前一样提取一下我们的数据集个数,后面会用到。🤓
nSets <- checkSets(multiExpr)$nSets
5挑选软阈值并可视化
5.1 创建power
先创建一下power
向量吧。🐵
powers <- c(seq(4,10,by=1), seq(12,20, by=2))
5.2 计算power
和之前一样,我们为每个数据集计算一下power
,挑选软阈值
。🥰
这里的机制是通过选择合适软阈值β
来提高共表达相似度,进一步计算邻接度,趋近一个无尺度的拓扑结构。🤓
powerTables = vector(mode = "list", length = nSets)
for (set in 1:nSets){
powerTables[[set]] = list(data = pickSoftThreshold(multiExpr[[set]]$data, powerVector=powers,
verbose = 2)[[2]])
collectGarbage()
}
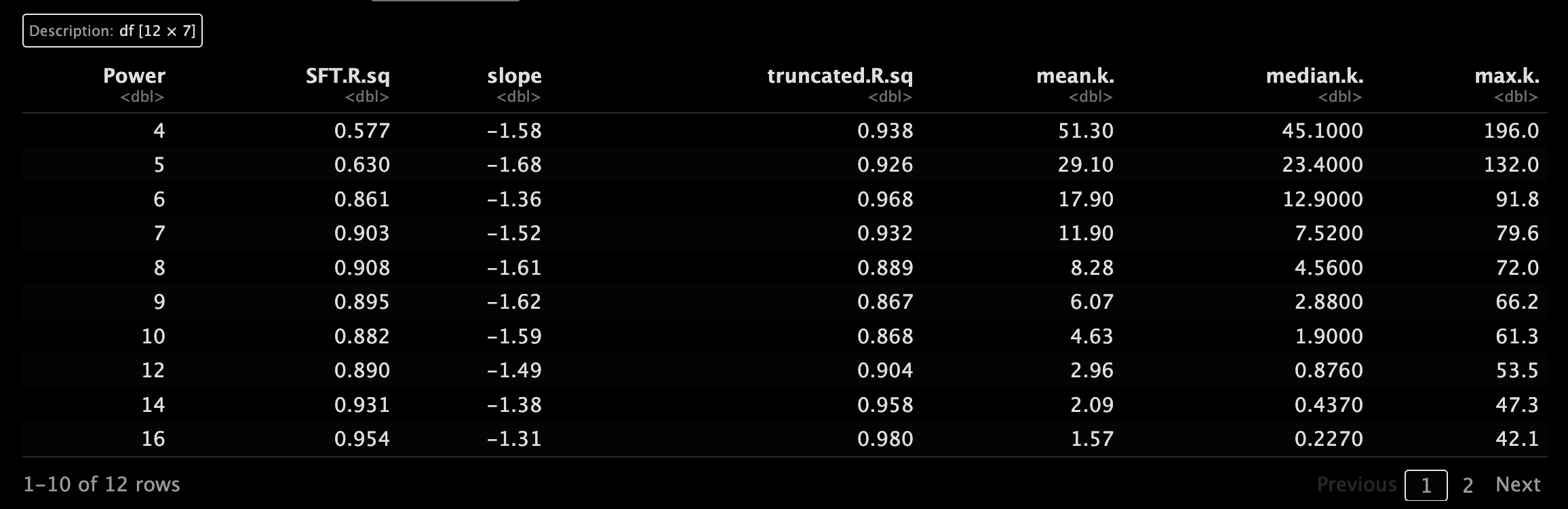
5.3 可视化相关参数设置
设置一下一会可视化出图的参数,提高颜值,嘿嘿!~😘
colors = c("black", "red")
plotCols = c(2,5,6,7)
colNames = c("Scale Free Topology Model Fit", "Mean connectivity", "Median connectivity",
"Max connectivity")
ylim = matrix(NA, nrow = 2, ncol = 4);
for (set in 1:nSets)
{
for (col in 1:length(plotCols))
{
ylim[1, col] = min(ylim[1, col], powerTables[[set]]$data[, plotCols[col]], na.rm = TRUE);
ylim[2, col] = max(ylim[2, col], powerTables[[set]]$data[, plotCols[col]], na.rm = TRUE);
}
}
5.4 可视化一下吧
来搞一下可视化看看吧,颜值还是高的诶。😂
sizeGrWindow(8, 6)
par(mfcol = c(2,2));
par(mar = c(4.2, 4.2 , 2.2, 0.5))
cex1 = 0.7;
for (col in 1:length(plotCols)) for (set in 1:nSets)
{
if (set==1)
{
plot(powerTables[[set]]$data[,1], -sign(powerTables[[set]]$data[,3])*powerTables[[set]]$data[,2],
xlab="Soft Threshold (power)",ylab=colNames[col],type="n", ylim = ylim[, col],
main = colNames[col]);
addGrid();
}
if (col==1)
{
text(powerTables[[set]]$data[,1], -sign(powerTables[[set]]$data[,3])*powerTables[[set]]$data[,2],
labels=powers,cex=cex1,col=colors[set]);
} else
text(powerTables[[set]]$data[,1], powerTables[[set]]$data[,plotCols[col]],
labels=powers,cex=cex1,col=colors[set]);
if (col==1)
{
legend("bottomright", legend = setLabels, col = colors, pch = 20) ;
} else
legend("topright", legend = setLabels, col = colors, pch = 20) ;
}
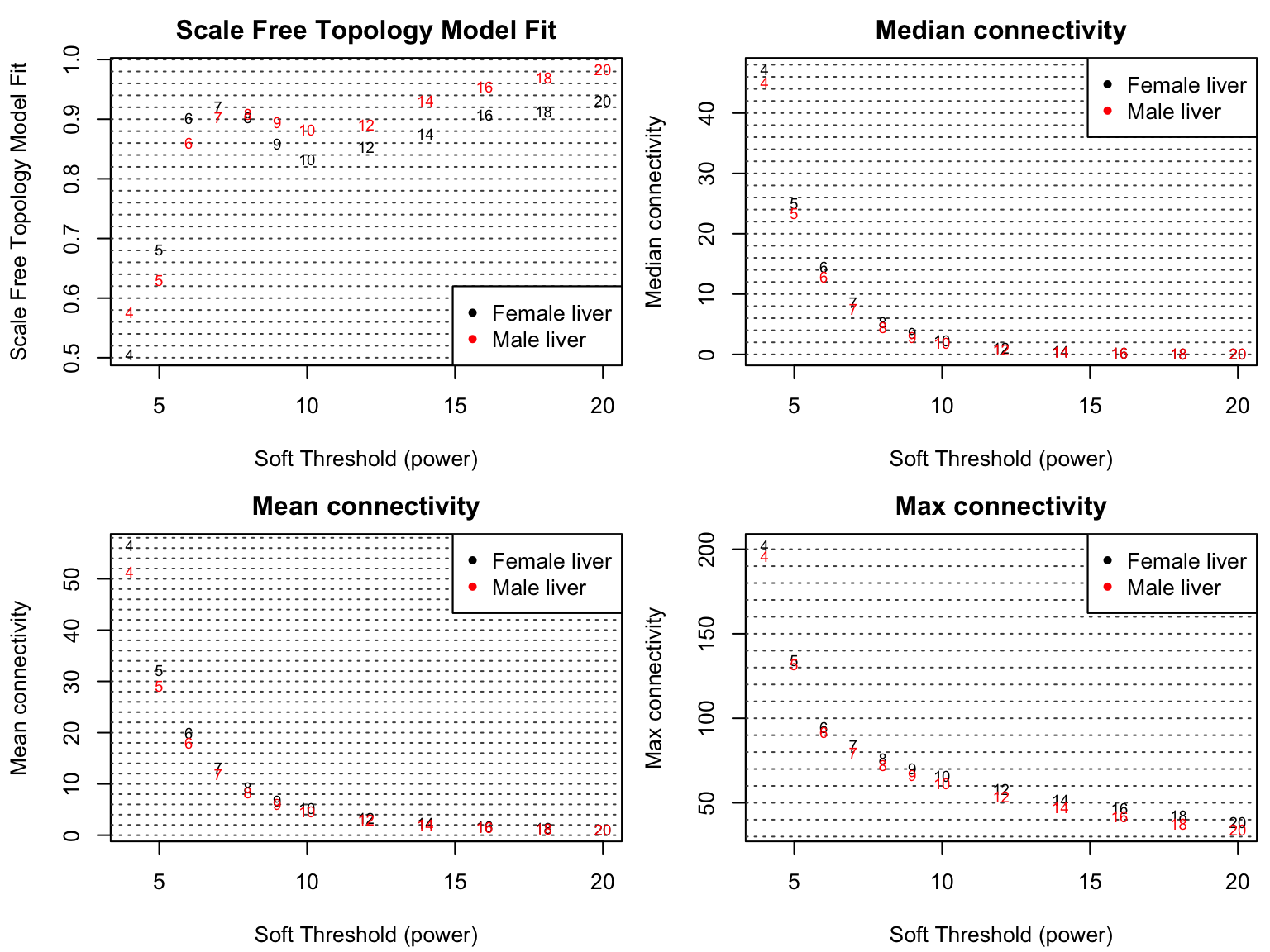
6Block-wise的方式构建网络
为简单起见,假设硬件极限是可以同时分析的基因数量为2000
。😅
基本思想是使用two-level
聚类。🤒
首先,我们使用快速、计算成本低且相对粗略的聚类方法,将基因预聚类到大小接近但不超过2000
个基因block
大小。😘
然后我们在每个block
中执行完整的共识网络分析和模块
识别。🤓
最后,合并特征基因在所有数据集中高度相关的模块
。🥳
bnet <- blockwiseConsensusModules(
multiExpr, maxBlockSize = 2000, power = 6, minModuleSize = 30,
deepSplit = 2,
pamRespectsDendro = F,
mergeCutHeight = 0.25, numericLabels = T,
minKMEtoStay = 0,
saveTOMs = T, verbose = 5)
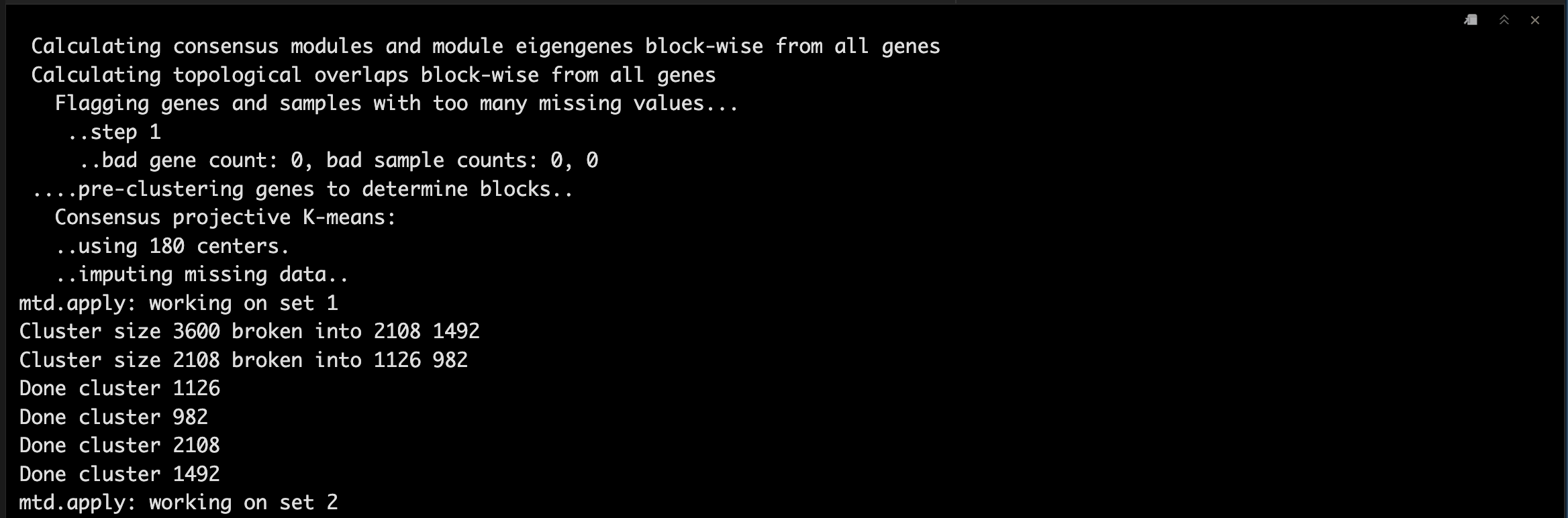
7对比一下
为了对比一下Block-wise
是否可以达到一步法
的效果,我们把一步法
计算的结果加载进来,对比一下。😗
7.1 一步法结果
加载一步法
结果进来吧,把label
和color
匹配一下哦。🤨
load(file = "./Consensus-NetworkConstruction-auto.RData")
bwLabels <- matchLabels(bnet$colors, moduleLabels, pThreshold = 1e-7)
bwColors <- labels2colors(bwLabels)
table(bwLabels)

7.2 block分别可视化
我们对每个block
都进行一下可视化。😘
sizeGrWindow(12,6)
layout(matrix(c(1:4), 2, 2), heights = c(0.8, 0.2), widths = c(1,1))
nBlocks = length(bnet$dendrograms)
for (block in 1:nBlocks){
plotDendroAndColors(bnet$dendrograms[[block]],
moduleColors[bnet$blockGenes[[block]]],
"Module colors",
main = paste("Gene dendrogram and module colors in block", block),
dendroLabels = F, hang = 0.03,
addGuide = T, guideHang = 0.05,
setLayout = F)
}
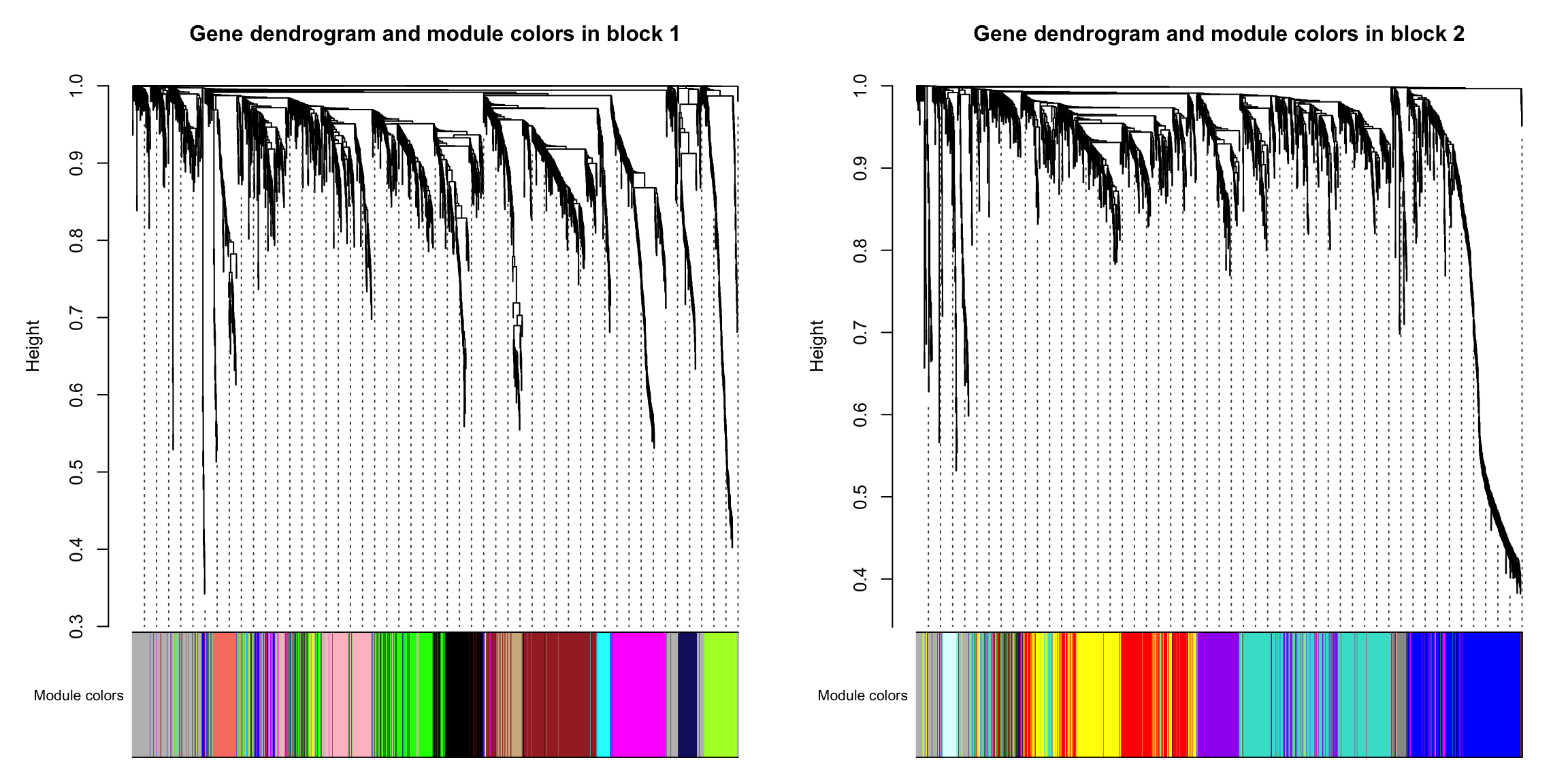
7.3 对比一下结果
我们把一步法和Block-wise
得到的结果画在一张图上,对比一下吧。🤓
差的不多,还是可以接受的。🐵
sizeGrWindow(12,9)
plotDendroAndColors(consTree,
cbind(moduleColors, bwColors),
c("Single block", "Blockwise"),
dendroLabels = F, hang = 0.03,
addGuide = T, guideHang = 0.05,
main = "Single block consensus gene dendrogram and module colors")
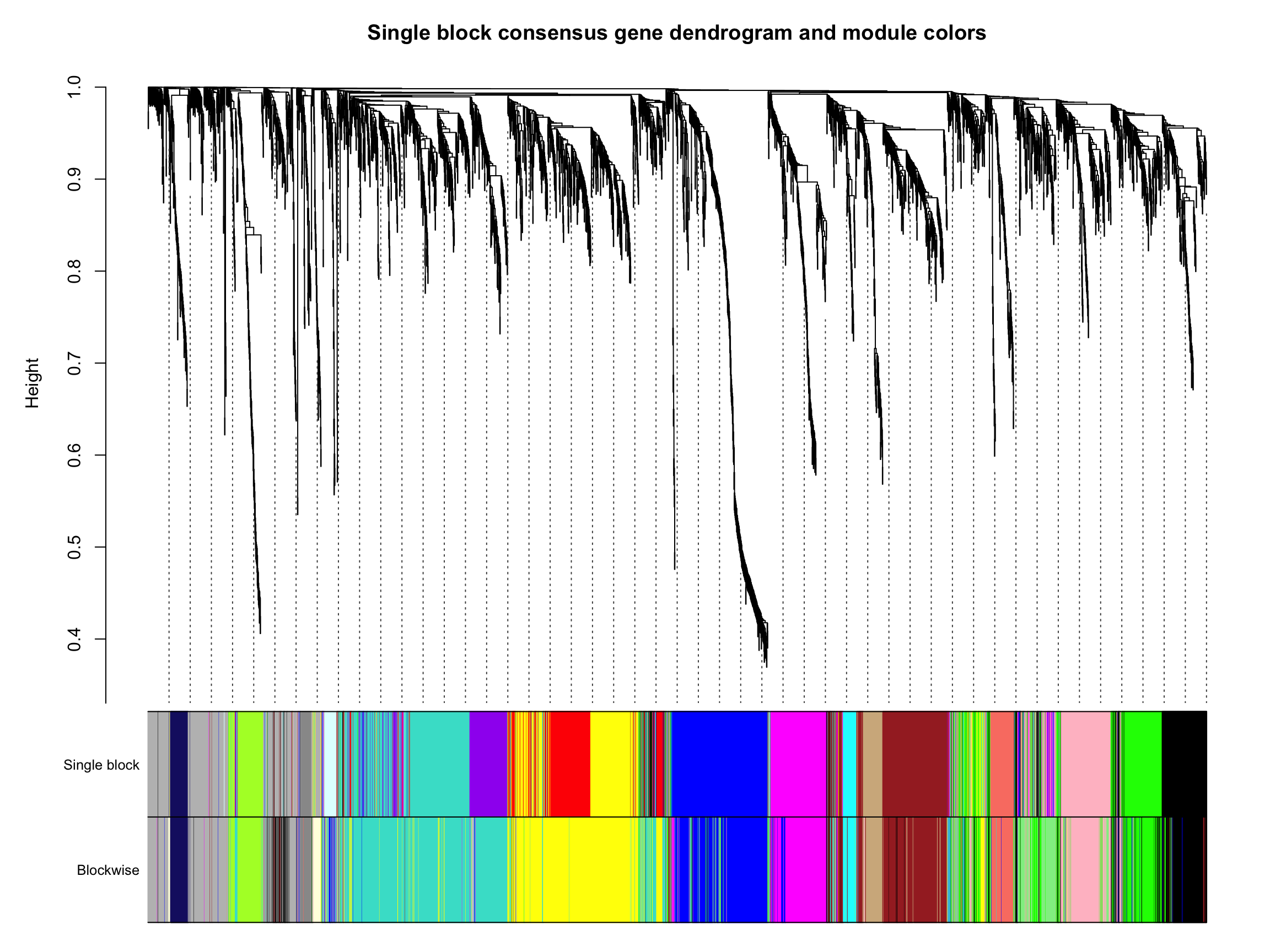

点个在看吧各位~ ✐.ɴɪᴄᴇ ᴅᴀʏ 〰
📍 🤣 chatPDF | 别再自己读文献了!让chatGPT来帮你读吧!~
📍 🤩 WGCNA | 值得你深入学习的生信分析方法!~
📍 🤩 ComplexHeatmap | 颜狗写的高颜值热图代码!
📍 🤥 ComplexHeatmap | 你的热图注释还挤在一起看不清吗!?
📍 🤨 Google | 谷歌翻译崩了我们怎么办!?(附完美解决方案)
📍 🤩 scRNA-seq | 吐血整理的单细胞入门教程
📍 🤣 NetworkD3 | 让我们一起画个动态的桑基图吧~
📍 🤩 RColorBrewer | 再多的配色也能轻松搞定!~
📍 🧐 rms | 批量完成你的线性回归
📍 🤩 CMplot | 完美复刻Nature上的曼哈顿图
📍 🤠 Network | 高颜值动态网络可视化工具
📍 🤗 boxjitter | 完美复刻Nature上的高颜值统计图
📍 🤫 linkET | 完美解决ggcor安装失败方案(附教程)
📍 ......
本文由 mdnice 多平台发布