前言

当你在买房子的时候会考虑什么?房子的面积?地理位置?产权年限?是否有地下室?多少楼层?是否学区房?交通是否便利?周围设施是否完整?等等。。。没错,当你想要的要求越来越高时,房子的价格也会越来越高,那么如何根据不同的要求来预测房价呢?这就是该篇博文要讲的内容。感谢kaggle,可以让我们获得那么多的数据来建立模型~~~
好了,其实要求很简单啦,就是根据房子不同的特征(包括面积、位置、产权等)来预测房价!
训练数据
kaggle中的数据大多数是以.csv文件保存,这篇博文所涉及的数据亦是如此,下面来看下训练数据吧:
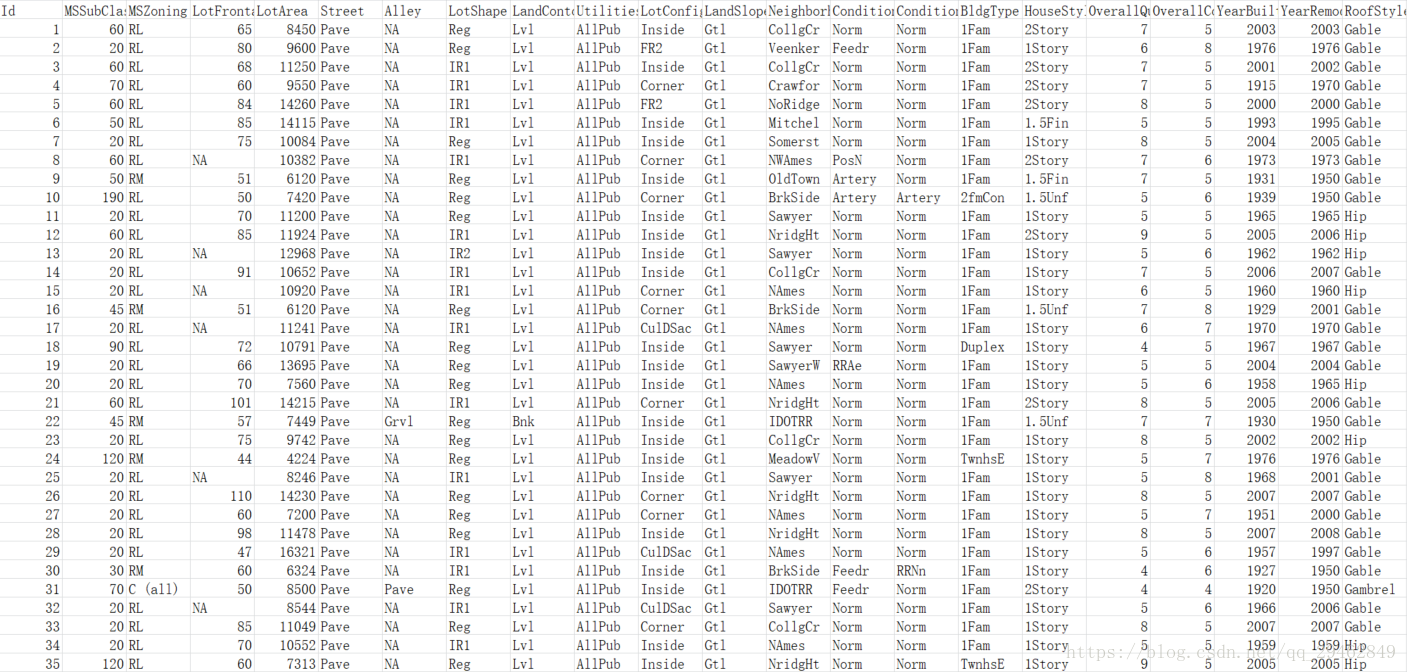
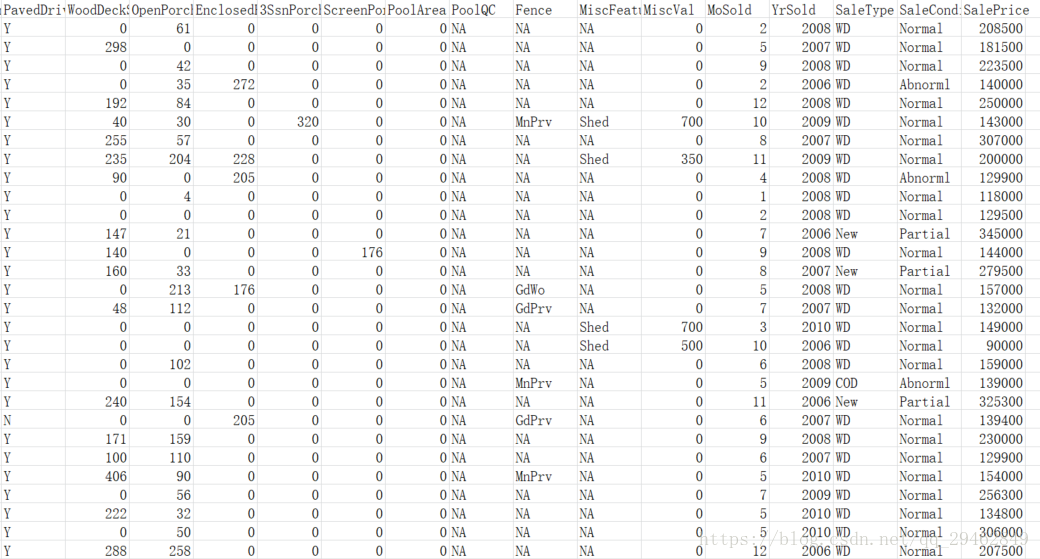
希望你没有密集恐惧症,一条数据包括房子的各个特征值以及销售价格~这里每条数据一共79个特征,1460个训练数据,1459个测试数据,分别保存在train.csv和test.csv文件中。
实现过程
main.py
import numpy as np # linear algebra
import pandas as pd # data processing, CSV file I/O (e.g. pd.read_csv)
import matplotlib.pyplot as plt # Matlab-style plotting
import seaborn as sns
from sklearn.model_selection import train_test_split
from sklearn.ensemble import GradientBoostingRegressor
from sklearn.metrics import mean_absolute_error
from DataClassify import DataClassify
color = sns.color_palette()
sns.set_style('darkgrid')
import warnings
from DataHandle import *
def ignore_warn(*args, **kwargs):
pass
warnings.warn = ignore_warn #ignore annoying warning (from sklearn and seaborn)
from scipy import stats
from scipy.stats import norm, skew #for some statistics
from sklearn.preprocessing import LabelEncoder
train_data_dir='C:/Users/18301/Desktop/data/train.csv'
test_data_dir='C:/Users/18301/Desktop/data/test.csv'
train,test=read_csv_data(train_data_dir,test_data_dir)
train,test,train_ID,test_ID=drop_id(train,test)
#Now drop the 'Id' colum since it's unnecessary for the prediction process.
#check again the data size after dropping the 'Id' variable
print("\nThe train data size after dropping Id feature is : {} ".format(train.shape))
print("The test data size after dropping Id feature is : {} ".format(test.shape))
#把训练数据和测试数据连接到一起,方便后面的统一处理和编码
#这里是记录train和test各有多少数据,既然有连接,到后面处理结束,必然还会有分开,这是用来分开数据的参照
ntrain,ntest,y_train,all_data=get_train_data(train,test)
#[2919,79]
#在这里输出缺失值最严重的前20个特征
all_data_na = (all_data.isnull().sum() / len(all_data)) * 100
all_data_na = all_data_na.drop(all_data_na[all_data_na == 0].index).sort_values(ascending=False)[:30]
missing_data = pd.DataFrame({
'Missing Ratio' :all_data_na