YOLOv8目标检测创新改进与实战案例专栏
专栏目录: YOLOv8有效改进系列及项目实战目录 包含卷积,主干 注意力,检测头等创新机制 以及 各种目标检测分割项目实战案例
专栏链接: YOLOv8基础解析+创新改进+实战案例
介绍
摘要
卷积神经网络(ConvNets)通常在固定的资源预算下开发,如果有更多资源可用,则会进行扩展以提高准确性。在本文中,我们系统地研究了模型扩展,并发现仔细平衡网络的深度、宽度和分辨率可以带来更好的性能。基于这一观察,我们提出了一种新的扩展方法,使用一个简单但非常有效的复合系数均匀扩展深度、宽度和分辨率的所有维度。我们展示了这种方法在扩展MobileNets和ResNet时的有效性。
为了进一步提高,我们使用神经架构搜索设计了一个新的基准网络,并将其扩展,获得了一系列称为EfficientNets的模型,这些模型比以前的ConvNets在准确性和效率方面都有了很大的提升。特别是,我们的EfficientNet-B7在ImageNet上实现了最先进的84.3%的top-1准确率,同时在推理时比现有的最佳ConvNet小8.4倍,快6.1倍。我们的EfficientNets在迁移学习任务中也表现良好,在CIFAR-100(91.7%)、Flowers(98.8%)和其他3个迁移学习数据集上实现了最先进的准确率,参数量减少了一个数量级。源码可在:https://github.com/tensorflow/tpu/tree/master/models/official/efficientnet 找到。
文章链接
论文地址:论文地址
代码地址:代码地址
基本原理
卷积神经网络(ConvNets)通常是在固定资源预算下开发的,当有更多资源可用时,可以通过增加网络深度(depth)、网络宽度(width)和输入图像分辨率(resolution)来提高精度。然而,手动调整这些参数组合非常困难,尤其在计算资源有限的情况下,不同参数的组合空间太大,难以穷举。
创新方法
本文提出了一种新的模型缩放方法,通过使用一个简单而高效的复合系数,从深度(depth)、宽度(width)和分辨率(resolution)三个维度放大网络。相比传统方法,该方法不会随意缩放网络的维度,结合神经结构搜索技术,可以获得最优的一组参数(复合系数)。
复合模型扩张方法
- 问题定义:卷积网络N可以分为多个阶段,每个阶段由多个相同结构的卷积层组成。
- 优化目标:在资源有限的情况下,最大化精度(Accuracy)。更大的网络具有更大的宽度、深度或分辨率,可以获得更高精度,但单一维度的扩展效果有限。
- 模型扩张的局限性:只对单一维度进行扩张的精度增益迅速饱和,说明需要平衡各个维度的扩张。
复合扩张方法的求解
- 求解参数:通过固定φ=1,通过网格搜索(grid search)得到最优的α、β、γ,得到基本模型EfficientNet-B0。
- 扩展模型:固定α、β、γ的值,通过调整φ的大小,获得EfficientNet-B1到B7。φ的大小决定了资源消耗的大小。
核心代码
# Copyright 2019 The TensorFlow Authors. All Rights Reserved.
#
# Licensed under the Apache License, Version 2.0 (the "License");
# you may not use this file except in compliance with the License.
# You may obtain a copy of the License at
#
# http://www.apache.org/licenses/LICENSE-2.0
#
# Unless required by applicable law or agreed to in writing, software
# distributed under the License is distributed on an "AS IS" BASIS,
# WITHOUT WARRANTIES OR CONDITIONS OF ANY KIND, either express or implied.
# See the License for the specific language governing permissions and
# limitations under the License.
# ==============================================================================
"""Contains definitions EfficientNet."""
from absl import logging
import tensorflow.compat.v1 as tf
import sys
sys.path.append('tpu/models/official/efficientnet')
from modeling.architecture import efficientnet_constants
from modeling.architecture import nn_blocks
from modeling.architecture import nn_ops
from official.efficientnet import efficientnet_builder
class Efficientnet(object):
"""Class to build EfficientNet family models."""
def __init__(self,
model_name):
"""EfficientNet initialization function.
Args:
model_name: string, the EfficientNet model name, e.g., `efficient-b0`.
"""
self._model_name = model_name
def __call__(self, inputs, is_training=False):
"""Returns features at various levels for EfficientNet model.
Args:
inputs: a `Tesnor` with shape [batch_size, height, width, 3] representing
a batch of images.
is_training: `bool` if True, the model is in training mode.
Returns:
a `dict` containing `int` keys for continuous feature levels [2, 3, 4, 5].
The values are corresponding feature hierarchy in EfficientNet with shape
[batch_size, height_l, width_l, num_filters].
"""
_, endpoints = efficientnet_builder.build_model(
inputs,
self._model_name,
training=is_training,
override_params=None)
u2 = endpoints['reduction_2']
u3 = endpoints['reduction_3']
u4 = endpoints['reduction_4']
u5 = endpoints['reduction_5']
return {2: u2, 3: u3, 4: u4, 5: u5}
class BlockSpec(object):
"""A container class that specifies the block configuration for EfficientNet."""
def __init__(self, num_repeats, block_fn, expand_ratio, kernel_size, se_ratio,
output_filters, act_fn):
self.num_repeats = num_repeats
self.block_fn = block_fn
self.expand_ratio = expand_ratio
self.kernel_size = kernel_size
self.se_ratio = se_ratio
self.output_filters = output_filters
self.act_fn = act_fn
def build_block_specs(block_specs=None):
"""Builds the list of BlockSpec objects for EfficientNet."""
if not block_specs:
block_specs = efficientnet_constants.EFFICIENTNET_X_B0_BLOCK_SPECS
if len(block_specs) != efficientnet_constants.EFFICIENTNET_NUM_BLOCKS:
raise ValueError(
'The block_specs of EfficientNet must be a length {} list.'.format(
efficientnet_constants.EFFICIENTNET_NUM_BLOCKS))
logging.info('Building EfficientNet block specs: %s', block_specs)
return [BlockSpec(*b) for b in block_specs]
class EfficientNetX(object):
"""Class to build EfficientNet and X family models."""
def __init__(self,
block_specs=build_block_specs(),
batch_norm_activation=nn_ops.BatchNormActivation(),
data_format='channels_last'):
"""EfficientNet initialization function.
Args:
block_specs: a list of BlockSpec objects that specifies the EfficientNet
network. By default, the previously discovered EfficientNet-A1 is used.
batch_norm_activation: an operation that includes a batch normalization
layer followed by an optional activation layer.
data_format: An optional string from: "channels_last", "channels_first".
Defaults to "channels_last".
"""
self._block_specs = block_specs
self._batch_norm_activation = batch_norm_activation
self._data_format = data_format
def __call__(self, images, is_training=False):
"""Generate a multiscale feature pyramid.
Args:
images: The input image tensor.
is_training: `bool` if True, the model is in training mode.
Returns:
a `dict` containing `int` keys for continuous feature levels
[min_level, min_level + 1, ..., max_level]. The values are corresponding
features with shape [batch_size, height_l, width_l,
endpoints_num_filters].
"""
x = images
with tf.variable_scope('efficientnet'):
x = nn_ops.conv2d_fixed_padding(
inputs=x,
filters=32,
kernel_size=3,
strides=2,
data_format=self._data_format)
x = tf.identity(x, 'initial_conv')
x = self._batch_norm_activation(x, is_training=is_training)
endpoints = []
for i, block_spec in enumerate(self._block_specs):
bn_act = nn_ops.BatchNormActivation(activation=block_spec.act_fn)
with tf.variable_scope('block_{}'.format(i)):
for j in range(block_spec.num_repeats):
strides = (1 if j > 0 else
efficientnet_constants.EFFICIENTNET_STRIDES[i])
if block_spec.block_fn == 'conv':
x = nn_ops.conv2d_fixed_padding(
inputs=x,
filters=block_spec.output_filters,
kernel_size=block_spec.kernel_size,
strides=strides,
data_format=self._data_format)
x = bn_act(x, is_training=is_training)
elif block_spec.block_fn == 'mbconv':
x_shape = x.get_shape().as_list()
in_filters = (
x_shape[1]
if self._data_format == 'channel_first' else x_shape[-1])
x = nn_blocks.mbconv_block(
inputs=x,
in_filters=in_filters,
out_filters=block_spec.output_filters,
expand_ratio=block_spec.expand_ratio,
strides=strides,
kernel_size=block_spec.kernel_size,
se_ratio=block_spec.se_ratio,
batch_norm_activation=bn_act,
data_format=self._data_format,
is_training=is_training)
elif block_spec.block_fn == 'fused_mbconv':
x_shape = x.get_shape().as_list()
in_filters = (
x_shape[1]
if self._data_format == 'channel_first' else x_shape[-1])
x = nn_blocks.fused_mbconv_block(
inputs=x,
in_filters=in_filters,
out_filters=block_spec.output_filters,
expand_ratio=block_spec.expand_ratio,
strides=strides,
kernel_size=block_spec.kernel_size,
se_ratio=block_spec.se_ratio,
batch_norm_activation=bn_act,
data_format=self._data_format,
is_training=is_training)
else:
raise ValueError('Un-supported block_fn `{}`!'.format(
block_spec.block_fn))
x = tf.identity(x, 'endpoints')
endpoints.append(x)
return {2: endpoints[1], 3: endpoints[2], 4: endpoints[4], 5: endpoints[6]}
下载YoloV8代码
直接下载
Git Clone
git clone https://github.com/ultralytics/ultralytics
安装环境
进入代码根目录并安装依赖。
pip install -r requirements.txt -i https://pypi.tuna.tsinghua.edu.cn/simple
在最新版本中,官方已经废弃了requirements.txt
文件,转而将所有必要的代码和依赖整合进了ultralytics
包中。因此,用户只需安装这个单一的ultralytics
库,就能获得所需的全部功能和环境依赖。
pip install ultralytics
引入代码
在根目录下的ultralytics/nn/
目录,新建一个 backbone
目录,然后新建一个以 EfficientNet
为文件名的py文件, 把代码拷贝进去。
import re
import math
import collections
from functools import partial
import torch
from torch import nn
from torch.nn import functional as F
from torch.utils import model_zoo
GlobalParams = collections.namedtuple('GlobalParams', [
'width_coefficient', 'depth_coefficient', 'image_size', 'dropout_rate',
'num_classes', 'batch_norm_momentum', 'batch_norm_epsilon',
'drop_connect_rate', 'depth_divisor', 'min_depth', 'include_top'])
BlockArgs = collections.namedtuple('BlockArgs', [
'num_repeat', 'kernel_size', 'stride', 'expand_ratio',
'input_filters', 'output_filters', 'se_ratio', 'id_skip'])
GlobalParams.__new__.__defaults__ = (None,) * len(GlobalParams._fields)
BlockArgs.__new__.__defaults__ = (None,) * len(BlockArgs._fields)
if hasattr(nn, 'SiLU'):
Swish = nn.SiLU
else:
class Swish(nn.Module):
def forward(self, x):
return x * torch.sigmoid(x)
class SwishImplementation(torch.autograd.Function):
@staticmethod
def forward(ctx, i):
result = i * torch.sigmoid(i)
ctx.save_for_backward(i)
return result
@staticmethod
def backward(ctx, grad_output):
i = ctx.saved_tensors[0]
sigmoid_i = torch.sigmoid(i)
return grad_output * (sigmoid_i * (1 + i * (1 - sigmoid_i)))
class MemoryEfficientSwish(nn.Module):
def forward(self, x):
return SwishImplementation.apply(x)
def round_filters(filters, global_params):
multiplier = global_params.width_coefficient
if not multiplier:
return filters
divisor = global_params.depth_divisor
min_depth = global_params.min_depth
filters *= multiplier
min_depth = min_depth or divisor
new_filters = max(min_depth, int(filters + divisor / 2) // divisor * divisor)
if new_filters < 0.9 * filters:
new_filters += divisor
return int(new_filters)
def round_repeats(repeats, global_params):
multiplier = global_params.depth_coefficient
if not multiplier:
return repeats
return int(math.ceil(multiplier * repeats))
def drop_connect(inputs, p, training):
assert 0 <= p <= 1, 'p must be in range of [0,1]'
if not training:
return inputs
batch_size = inputs.shape[0]
keep_prob = 1 - p
random_tensor = keep_prob
random_tensor += torch.rand([batch_size, 1, 1, 1], dtype=inputs.dtype, device=inputs.device)
binary_tensor = torch.floor(random_tensor)
output = inputs / keep_prob * binary_tensor
return output
def get_width_and_height_from_size(x):
if isinstance(x, int):
return x, x
if isinstance(x, list) or isinstance(x, tuple):
return x
else:
raise TypeError()
def calculate_output_image_size(input_image_size, stride):
if input_image_size is None:
return None
image_height, image_width = get_width_and_height_from_size(input_image_size)
stride = stride if isinstance(stride, int) else stride[0]
image_height = int(math.ceil(image_height / stride))
image_width = int(math.ceil(image_width / stride))
return [image_height, image_width]
def get_same_padding_conv2d(image_size=None):
if image_size is None:
return Conv2dDynamicSamePadding
else:
return partial(Conv2dStaticSamePadding, image_size=image_size)
class Conv2dDynamicSamePadding(nn.Conv2d):
def __init__(self, in_channels, out_channels, kernel_size, stride=1, dilation=1, groups=1, bias=True):
super().__init__(in_channels, out_channels, kernel_size, stride, 0, dilation, groups, bias)
self.stride = self.stride if len(self.stride) == 2 else [self.stride[0]] * 2
def forward(self, x):
ih, iw = x.size()[-2:]
kh, kw = self.weight.size()[-2:]
sh, sw = self.stride
oh, ow = math.ceil(ih / sh), math.ceil(iw / sw)
pad_h = max((oh - 1) * self.stride[0] + (kh - 1) * self.dilation[0] + 1 - ih, 0)
pad_w = max((ow - 1) * self.stride[1] + (kw - 1) * self.dilation[1] + 1 - iw, 0)
if pad_h > 0 or pad_w > 0:
x = F.pad(x, [pad_w // 2, pad_w - pad_w // 2, pad_h // 2, pad_h - pad_h // 2])
return F.conv2d(x, self.weight, self.bias, self.stride, self.padding, self.dilation, self.groups)
class Conv2dStaticSamePadding(nn.Conv2d):
def __init__(self, in_channels, out_channels, kernel_size, stride=1, image_size=None, **kwargs):
super().__init__(in_channels, out_channels, kernel_size, stride, **kwargs)
self.stride = self.stride if len(self.stride) == 2 else [self.stride[0]] * 2
assert image_size is not None
ih, iw = (image_size, image_size) if isinstance(image_size, int) else image_size
kh, kw = self.weight.size()[-2:]
sh, sw = self.stride
oh, ow = math.ceil(ih / sh), math.ceil(iw / sw)
pad_h = max((oh - 1) * self.stride[0] + (kh - 1) * self.dilation[0] + 1 - ih, 0)
pad_w = max((ow - 1) * self.stride[1] + (kw - 1) * self.dilation[1] + 1 - iw, 0)
if pad_h > 0 or pad_w > 0:
self.static_padding = nn.ZeroPad2d((pad_w // 2, pad_w - pad_w // 2, pad_h // 2, pad_h - pad_h // 2))
else:
self.static_padding = nn.Identity()
def forward(self, x):
x = self.static_padding(x)
x = F.conv2d(x, self.weight, self.bias, self.stride, self.padding, self.dilation, self.groups)
return x
def get_same_padding_maxPool2d(image_size=None):
if image_size is None:
return MaxPool2dDynamicSamePadding
else:
return partial(MaxPool2dStaticSamePadding, image_size=image_size)
class MaxPool2dDynamicSamePadding(nn.MaxPool2d):
def __init__(self, kernel_size, stride, padding=0, dilation=1, return_indices=False, ceil_mode=False):
super().__init__(kernel_size, stride, padding, dilation, return_indices, ceil_mode)
self.stride = [self.stride] * 2 if isinstance(self.stride, int) else self.stride
self.kernel_size = [self.kernel_size] * 2 if isinstance(self.kernel_size, int) else self.kernel_size
self.dilation = [self.dilation] * 2 if isinstance(self.dilation, int) else self.dilation
def forward(self, x):
ih, iw = x.size()[-2:]
kh, kw = self.kernel_size
sh, sw = self.stride
oh, ow = math.ceil(ih / sh), math.ceil(iw / sw)
pad_h = max((oh - 1) * self.stride[0] + (kh - 1) * self.dilation[0] + 1 - ih, 0)
pad_w = max((ow - 1) * self.stride[1] + (kw - 1) * self.dilation[1] + 1 - iw, 0)
if pad_h > 0 or pad_w > 0:
x = F.pad(x, [pad_w // 2, pad_w - pad_w // 2, pad_h // 2, pad_h - pad_h // 2])
return F.max_pool2d(x, self.kernel_size, self.stride, self.padding,
self.dilation, self.ceil_mode, self.return_indices)
class MaxPool2dStaticSamePadding(nn.MaxPool2d):
def __init__(self, kernel_size, stride, image_size=None, **kwargs):
super().__init__(kernel_size, stride, **kwargs)
self.stride = [self.stride] * 2 if isinstance(self.stride, int) else self.stride
self.kernel_size = [self.kernel_size] * 2 if isinstance(self.kernel_size, int) else self.kernel_size
self.dilation = [self.dilation] * 2 if isinstance(self.dilation, int) else self.dilation
assert image_size is not None
ih, iw = (image_size, image_size) if isinstance(image_size, int) else image_size
kh, kw = self.kernel_size
sh, sw = self.stride
oh, ow = math.ceil(ih / sh), math.ceil(iw / sw)
pad_h = max((oh - 1) * self.stride[0] + (kh - 1) * self.dilation[0] + 1 - ih, 0)
pad_w = max((ow - 1) * self.stride[1] + (kw - 1) * self.dilation[1] + 1 - iw, 0)
if pad_h > 0 or pad_w > 0:
self.static_padding = nn.ZeroPad2d((pad_w // 2, pad_w - pad_w // 2, pad_h // 2, pad_h - pad_h // 2))
else:
self.static_padding = nn.Identity()
def forward(self, x):
x = self.static_padding(x)
x = F.max_pool2d(x, self.kernel_size, self.stride, self.padding,
self.dilation, self.ceil_mode, self.return_indices)
return x
class BlockDecoder(object):
@staticmethod
def _decode_block_string(block_string):
assert isinstance(block_string, str)
ops = block_string.split('_')
options = {}
for op in ops:
splits = re.split(r'(\d.*)', op)
if len(splits) >= 2:
key, value = splits[:2]
options[key] = value
assert (('s' in options and len(options['s']) == 1) or
(len(options['s']) == 2 and options['s'][0] == options['s'][1]))
return BlockArgs(
num_repeat=int(options['r']),
kernel_size=int(options['k']),
stride=[int(options['s'][0])],
expand_ratio=int(options['e']),
input_filters=int(options['i']),
output_filters=int(options['o']),
se_ratio=float(options['se']) if 'se' in options else None,
id_skip=('noskip' not in block_string))
@staticmethod
def _encode_block_string(block):
args = [
'r%d' % block.num_repeat,
'k%d' % block.kernel_size,
's%d%d' % (block.strides[0], block.strides[1]),
'e%s' % block.expand_ratio,
'i%d' % block.input_filters,
'o%d' % block.output_filters
]
if 0 < block.se_ratio <= 1:
args.append('se%s' % block.se_ratio)
if block.id_skip is False:
args.append('noskip')
return '_'.join(args)
@staticmethod
def decode(string_list):
assert isinstance(string_list, list)
blocks_args = []
for block_string in string_list:
blocks_args.append(BlockDecoder._decode_block_string(block_string))
return blocks_args
@staticmethod
def encode(blocks_args):
block_strings = []
for block in blocks_args:
block_strings.append(BlockDecoder._encode_block_string(block))
return block_strings
def efficientnet_params(model_name):
params_dict = {
'efficientnet-b0': (1.0, 1.0, 224, 0.2),
'efficientnet-b1': (1.0, 1.1, 240, 0.2),
'efficientnet-b2': (1.1, 1.2, 260, 0.3),
'efficientnet-b3': (1.2, 1.4, 300, 0.3),
'efficientnet-b4': (1.4, 1.8, 380, 0.4),
'efficientnet-b5': (1.6, 2.2, 456, 0.4),
'efficientnet-b6': (1.8, 2.6, 528, 0.5),
'efficientnet-b7': (2.0, 3.1, 600, 0.5),
'efficientnet-b8': (2.2, 3.6, 672, 0.5),
'efficientnet-l2': (4.3, 5.3, 800, 0.5),
}
return params_dict[model_name]
def efficientnet(width_coefficient=None, depth_coefficient=None, image_size=None,
dropout_rate=0.2, drop_connect_rate=0.2, num_classes=1000, include_top=True):
blocks_args = [
'r1_k3_s11_e1_i32_o16_se0.25',
'r2_k3_s22_e6_i16_o24_se0.25',
'r2_k5_s22_e6_i24_o40_se0.25',
'r3_k3_s22_e6_i40_o80_se0.25',
'r3_k5_s11_e6_i80_o112_se0.25',
'r4_k5_s22_e6_i112_o192_se0.25',
'r1_k3_s11_e6_i192_o320_se0.25',
]
blocks_args = BlockDecoder.decode(blocks_args)
global_params = GlobalParams(
width_coefficient=width_coefficient,
depth_coefficient=depth_coefficient,
image_size=image_size,
dropout_rate=dropout_rate,
num_classes=num_classes,
batch_norm_momentum=0.99,
batch_norm_epsilon=1e-3,
drop_connect_rate=drop_connect_rate,
depth_divisor=8,
min_depth=None,
include_top=include_top,
)
return blocks_args, global_params
def get_model_params(model_name, override_params):
if model_name.startswith('efficientnet'):
w, d, s, p = efficientnet_params(model_name)
blocks_args, global_params = efficientnet(
width_coefficient=w, depth_coefficient=d, dropout_rate=p, image_size=s)
else:
raise NotImplementedError('model name is not pre-defined: {}'.format(model_name))
if override_params:
global_params = global_params._replace(**override_params)
return blocks_args, global_params
url_map = {
'efficientnet-b0': 'https://github.com/lukemelas/EfficientNet-PyTorch/releases/download/1.0/efficientnet-b0-355c32eb.pth',
'efficientnet-b1': 'https://github.com/lukemelas/EfficientNet-PyTorch/releases/download/1.0/efficientnet-b1-f1951068.pth',
'efficientnet-b2': 'https://github.com/lukemelas/EfficientNet-PyTorch/releases/download/1.0/efficientnet-b2-8bb594d6.pth',
'efficientnet-b3': 'https://github.com/lukemelas/EfficientNet-PyTorch/releases/download/1.0/efficientnet-b3-5fb5a3c3.pth',
'efficientnet-b4': 'https://github.com/lukemelas/EfficientNet-PyTorch/releases/download/1.0/efficientnet-b4-6ed6700e.pth',
'efficientnet-b5': 'https://github.com/lukemelas/EfficientNet-PyTorch/releases/download/1.0/efficientnet-b5-b6417697.pth',
'efficientnet-b6': 'https://github.com/lukemelas/EfficientNet-PyTorch/releases/download/1.0/efficientnet-b6-c76e70fd.pth',
'efficientnet-b7': 'https://github.com/lukemelas/EfficientNet-PyTorch/releases/download/1.0/efficientnet-b7-dcc49843.pth',
}
url_map_advprop = {
'efficientnet-b0': 'https://github.com/lukemelas/EfficientNet-PyTorch/releases/download/1.0/adv-efficientnet-b0-b64d5a18.pth',
'efficientnet-b1': 'https://github.com/lukemelas/EfficientNet-PyTorch/releases/download/1.0/adv-efficientnet-b1-0f3ce85a.pth',
'efficientnet-b2': 'https://github.com/lukemelas/EfficientNet-PyTorch/releases/download/1.0/adv-efficientnet-b2-6e9d97e5.pth',
'efficientnet-b3': 'https://github.com/lukemelas/EfficientNet-PyTorch/releases/download/1.0/adv-efficientnet-b3-cdd7c0f4.pth',
'efficientnet-b4': 'https://github.com/lukemelas/EfficientNet-PyTorch/releases/download/1.0/adv-efficientnet-b4-44fb3a87.pth',
'efficientnet-b5': 'https://github.com/lukemelas/EfficientNet-PyTorch/releases/download/1.0/adv-efficientnet-b5-86493f6b.pth',
'efficientnet-b6': 'https://github.com/lukemelas/EfficientNet-PyTorch/releases/download/1.0/adv-efficientnet-b6-ac80338e.pth',
'efficientnet-b7': 'https://github.com/lukemelas/EfficientNet-PyTorch/releases/download/1.0/adv-efficientnet-b7-4652b6dd.pth',
'efficientnet-b8': 'https://github.com/lukemelas/EfficientNet-PyTorch/releases/download/1.0/adv-efficientnet-b8-22a8fe65.pth',
}
def load_pretrained_weights(model, model_name, weights_path=None, load_fc=True, advprop=False, verbose=True):
if isinstance(weights_path, str):
state_dict = torch.load(weights_path)
else:
url_map_ = url_map_advprop if advprop else url_map
state_dict = model_zoo.load_url(url_map_[model_name])
if load_fc:
ret = model.load_state_dict(state_dict, strict=False)
assert not ret.missing_keys, 'Missing keys when loading pretrained weights: {}'.format(ret.missing_keys)
else:
state_dict.pop('_fc.weight')
state_dict.pop('_fc.bias')
ret = model.load_state_dict(state_dict, strict=False)
assert set(ret.missing_keys) == set(
['_fc.weight', '_fc.bias']), 'Missing keys when loading pretrained weights: {}'.format(ret.missing_keys)
assert not ret.unexpected_keys, 'Missing keys when loading pretrained weights: {}'.format(ret.unexpected_keys)
if verbose:
print('Loaded pretrained weights for {}'.format(model_name))
VALID_MODELS = (
'efficientnet-b0', 'efficientnet-b1', 'efficientnet-b2', 'efficientnet-b3',
'efficientnet-b4', 'efficientnet-b5', 'efficientnet-b6', 'efficientnet-b7',
'efficientnet-b8',
'efficientnet-l2'
)
class MBConvBlock(nn.Module):
def __init__(self, block_args, global_params, image_size=None):
super().__init__()
self._block_args = block_args
self._bn_mom = 1 - global_params.batch_norm_momentum
self._bn_eps = global_params.batch_norm_epsilon
self.has_se = (self._block_args.se_ratio is not None) and (0 < self._block_args.se_ratio <= 1)
self.id_skip = block_args.id_skip
inp = self._block_args.input_filters
oup = self._block_args.input_filters * self._block_args.expand_ratio
if self._block_args.expand_ratio != 1:
Conv2d = get_same_padding_conv2d(image_size=image_size)
self._expand_conv = Conv2d(in_channels=inp, out_channels=oup, kernel_size=1, bias=False)
self._bn0 = nn.BatchNorm2d(num_features=oup, momentum=self._bn_mom, eps=self._bn_eps)
k = self._block_args.kernel_size
s = self._block_args.stride
Conv2d = get_same_padding_conv2d(image_size=image_size)
self._depthwise_conv = Conv2d(
in_channels=oup, out_channels=oup, groups=oup,
kernel_size=k, stride=s, bias=False)
self._bn1 = nn.BatchNorm2d(num_features=oup, momentum=self._bn_mom, eps=self._bn_eps)
image_size = calculate_output_image_size(image_size, s)
if self.has_se:
Conv2d = get_same_padding_conv2d(image_size=(1, 1))
num_squeezed_channels = max(1, int(self._block_args.input_filters * self._block_args.se_ratio))
self._se_reduce = Conv2d(in_channels=oup, out_channels=num_squeezed_channels, kernel_size=1)
self._se_expand = Conv2d(in_channels=num_squeezed_channels, out_channels=oup, kernel_size=1)
final_oup = self._block_args.output_filters
Conv2d = get_same_padding_conv2d(image_size=image_size)
self._project_conv = Conv2d(in_channels=oup, out_channels=final_oup, kernel_size=1, bias=False)
self._bn2 = nn.BatchNorm2d(num_features=final_oup, momentum=self._bn_mom, eps=self._bn_eps)
self._swish = MemoryEfficientSwish()
def forward(self, inputs, drop_connect_rate=None):
x = inputs
if self._block_args.expand_ratio != 1:
x = self._expand_conv(inputs)
x = self._bn0(x)
x = self._swish(x)
x = self._depthwise_conv(x)
x = self._bn1(x)
x = self._swish(x)
if self.has_se:
x_squeezed = F.adaptive_avg_pool2d(x, 1)
x_squeezed = self._se_reduce(x_squeezed)
x_squeezed = self._swish(x_squeezed)
x_squeezed = self._se_expand(x_squeezed)
x = torch.sigmoid(x_squeezed) * x
x = self._project_conv(x)
x = self._bn2(x)
input_filters, output_filters = self._block_args.input_filters, self._block_args.output_filters
if self.id_skip and self._block_args.stride == 1 and input_filters == output_filters:
if drop_connect_rate:
x = drop_connect(x, p=drop_connect_rate, training=self.training)
x = x + inputs
return x
def set_swish(self, memory_efficient=True):
self._swish = MemoryEfficientSwish() if memory_efficient else Swish()
class EfficientNet(nn.Module):
def __init__(self, blocks_args=None, global_params=None):
super().__init__()
assert isinstance(blocks_args, list), 'blocks_args should be a list'
assert len(blocks_args) > 0, 'block args must be greater than 0'
self._global_params = global_params
self._blocks_args = blocks_args
bn_mom = 1 - self._global_params.batch_norm_momentum
bn_eps = self._global_params.batch_norm_epsilon
image_size = global_params.image_size
Conv2d = get_same_padding_conv2d(image_size=image_size)
in_channels = 3
out_channels = round_filters(32, self._global_params)
self._conv_stem = Conv2d(in_channels, out_channels, kernel_size=3, stride=2, bias=False)
self._bn0 = nn.BatchNorm2d(num_features=out_channels, momentum=bn_mom, eps=bn_eps)
image_size = calculate_output_image_size(image_size, 2)
self._blocks = nn.ModuleList([])
for block_args in self._blocks_args:
block_args = block_args._replace(
input_filters=round_filters(block_args.input_filters, self._global_params),
output_filters=round_filters(block_args.output_filters, self._global_params),
num_repeat=round_repeats(block_args.num_repeat, self._global_params)
)
self._blocks.append(MBConvBlock(block_args, self._global_params, image_size=image_size))
image_size = calculate_output_image_size(image_size, block_args.stride)
if block_args.num_repeat > 1:
block_args = block_args._replace(input_filters=block_args.output_filters, stride=1)
for _ in range(block_args.num_repeat - 1):
self._blocks.append(MBConvBlock(block_args, self._global_params, image_size=image_size))
in_channels = block_args.output_filters
out_channels = round_filters(1280, self._global_params)
Conv2d = get_same_padding_conv2d(image_size=image_size)
self._conv_head = Conv2d(in_channels, out_channels, kernel_size=1, bias=False)
self._bn1 = nn.BatchNorm2d(num_features=out_channels, momentum=bn_mom, eps=bn_eps)
self._avg_pooling = nn.AdaptiveAvgPool2d(1)
if self._global_params.include_top:
self._dropout = nn.Dropout(self._global_params.dropout_rate)
self._fc = nn.Linear(out_channels, self._global_params.num_classes)
self._swish = MemoryEfficientSwish()
self.channels = [i.size(1) for i in self.forward(torch.randn(1, 3, 640, 640))]
def set_swish(self, memory_efficient=True):
self._swish = MemoryEfficientSwish() if memory_efficient else Swish()
for block in self._blocks:
block.set_swish(memory_efficient)
def extract_endpoints(self, inputs):
endpoints = dict()
x = self._swish(self._bn0(self._conv_stem(inputs)))
prev_x = x
for idx, block in enumerate(self._blocks):
drop_connect_rate = self._global_params.drop_connect_rate
if drop_connect_rate:
drop_connect_rate *= float(idx) / len(self._blocks)
x = block(x, drop_connect_rate=drop_connect_rate)
if prev_x.size(2) > x.size(2):
endpoints['reduction_{}'.format(len(endpoints) + 1)] = prev_x
elif idx == len(self._blocks) - 1:
endpoints['reduction_{}'.format(len(endpoints) + 1)] = x
prev_x = x
x = self._swish(self._bn1(self._conv_head(x)))
endpoints['reduction_{}'.format(len(endpoints) + 1)] = x
return endpoints
def forward(self, inputs):
x = self._swish(self._bn0(self._conv_stem(inputs)))
unique_tensors = {}
for idx, block in enumerate(self._blocks):
drop_connect_rate = self._global_params.drop_connect_rate
if drop_connect_rate:
drop_connect_rate *= float(idx) / len(self._blocks)
x = block(x, drop_connect_rate=drop_connect_rate)
width, height = x.shape[2], x.shape[3]
unique_tensors[(width, height)] = x
result_list = list(unique_tensors.values())[-4:]
return result_list
@classmethod
def from_name(cls, model_name, in_channels=3, **override_params):
cls._check_model_name_is_valid(model_name)
blocks_args, global_params = get_model_params(model_name, override_params)
model = cls(blocks_args, global_params)
model._change_in_channels(in_channels)
return model
@classmethod
def from_pretrained(cls, model_name, weights_path=None, advprop=False,
in_channels=3, num_classes=1000, **override_params):
model = cls.from_name(model_name, num_classes=num_classes, **override_params)
load_pretrained_weights(model, model_name, weights_path=weights_path,
load_fc=(num_classes == 1000), advprop=advprop)
model._change_in_channels(in_channels)
return model
@classmethod
def get_image_size(cls, model_name):
cls._check_model_name_is_valid(model_name)
_, _, res, _ = efficientnet_params(model_name)
return res
@classmethod
def _check_model_name_is_valid(cls, model_name):
if model_name not in VALID_MODELS:
raise ValueError('model_name should be one of: ' + ', '.join(VALID_MODELS))
def _change_in_channels(self, in_channels):
if in_channels != 3:
Conv2d = get_same_padding_conv2d(image_size=self._global_params.image_size)
out_channels = round_filters(32, self._global_params)
self._conv_stem = Conv2d(in_channels, out_channels, kernel_size=3, stride=2, bias=False)
def efficient(model_name='efficientnet-b0', pretrained=False):
if pretrained:
model = EfficientNet.from_pretrained('{}'.format(model_name))
else:
model = EfficientNet.from_name('{}'.format(model_name))
return model
tasks注册
在ultralytics/nn/tasks.py
中进行如下操作:
步骤1:
from ultralytics.nn.backbone.EfficientNet import efficient
步骤2
修改def parse_model(d, ch, verbose=True)
:
elif m in {efficient}:
m = m(*args)
c2 = m.channels
增加代码1
在def parse_model(d, ch, verbose=True)
:增加t=m
for i, (f, n, m, args) in enumerate(d["backbone"] + d["head"]): # from, number, module, args
t = m
修改代码
修改def parse_model(d, ch, verbose=True)
中的:
# m_ = nn.Sequential(*(m(*args) for _ in range(n))) if n > 1 else m(*args) # module
# t = str(m)[8:-2].replace("__main__.", "") # module type
# m.np = sum(x.numel() for x in m_.parameters()) # number params
# m_.i, m_.f, m_.type = i, f, t # attach index, 'from' index, type
# if verbose:
# LOGGER.info(f"{i:>3}{str(f):>20}{n_:>3}{m.np:10.0f} {t:<45}{str(args):<30}") # print
# save.extend(x % i for x in ([f] if isinstance(f, int) else f) if x != -1) # append to savelist
# layers.append(m_)
# if i == 0:
# ch = []
# ch.append(c2)
修改为
if isinstance(c2, list):
m_ = m
m_.backbone = True
else:
m_ = nn.Sequential(*(m(*args) for _ in range(n))) if n > 1 else m(*args)
t = str(m)[8:-2].replace('__main__.', '')
m.np = sum(x.numel() for x in m_.parameters())
m_.i, m_.f, m_.type = i + 4 ,f,t
if verbose:
LOGGER.info(f'{i:>3}{str(f):>20}{n_:>3}{m.np:10.0f} {t:<45}{str(args):<30}')
save.extend(x % (i + 4 ) for x in ([f] if isinstance(f, int) else f) if x != -1)
layers.append(m_)
if i == 0:
ch = []
if isinstance(c2, list):
ch.extend(c2)
if len(c2) != 5:
ch.insert(0, 0)
else:
ch.append(c2)
替换_predict_once方法
把_predict_once
替换为下面的代码:
def _predict_once(self, x, profile=False, visualize=False, embed=None):
"""
Perform a forward pass through the network.
Args:
x (torch.Tensor): The input tensor to the model.
profile (bool): Print the computation time of each layer if True, defaults to False.
visualize (bool): Save the feature maps of the model if True, defaults to False.
embed (list, optional): A list of feature vectors/embeddings to return.
Returns:
(torch.Tensor): The last output of the model.
"""
y, dt, embeddings = [], [], []
for m in self.model:
if m.f != -1:
x = y[m.f] if isinstance(m.f, int) else [x if j == -1 else y[j] for j in m.f] # from earlier layers
if profile:
self._profile_one_layer(m, x, dt)
if hasattr(m, 'backbone'):
x = m(x)
if len(x) != 5: # 0 - 5
x.insert(0, None)
for index, i in enumerate(x):
if index in self.save:
y.append(i)
else:
y.append(None)
x = x[-1]
else:
x = m(x)
y.append(x if m.i in self.save else None)
if visualize:
feature_visualization(x, m.type, m.i, save_dir=visualize)
if embed and m.i in embed:
embeddings.append(nn.functional.adaptive_avg_pool2d(x, (1, 1)).squeeze(-1).squeeze(-1)) # flatten
if m.i == max(embed):
return torch.unbind(torch.cat(embeddings, 1), dim=0)
return x
配置yolov8_EfficientNet.yaml
ultralytics/cfg/models/v8/yolov8_EfficientNet.yaml
# Ultralytics YOLO 🚀, AGPL-3.0 license
# YOLOv8 object detection model with P3-P5 outputs. For Usage examples see https://docs.ultralytics.com/tasks/detect
# Parameters
nc: 80 # number of classes
scales: # model compound scaling constants, i.e. 'model=yolov8n.yaml' will call yolov8.yaml with scale 'n'
# [depth, width, max_channels]
n: [0.33, 0.25, 1024] # YOLOv8n summary: 225 layers, 3157200 parameters, 3157184 gradients, 8.9 GFLOPs
s: [0.33, 0.50, 1024] # YOLOv8s summary: 225 layers, 11166560 parameters, 11166544 gradients, 28.8 GFLOPs
m: [0.67, 0.75, 768] # YOLOv8m summary: 295 layers, 25902640 parameters, 25902624 gradients, 79.3 GFLOPs
l: [1.00, 1.00, 512] # YOLOv8l summary: 365 layers, 43691520 parameters, 43691504 gradients, 165.7 GFLOPs
x: [1.00, 1.25, 512] # YOLOv8x summary: 365 layers, 68229648 parameters, 68229632 gradients, 258.5 GFLOP
# YOLOv8.0n backbone
backbone:
# [from, repeats, module, args]
- [-1, 1, efficient, []] # 4
- [-1, 1, SPPF, [1024, 5]] # 5
# YOLOv8.0n head
head:
- [-1, 1, nn.Upsample, [None, 2, 'nearest']] # 6
- [[-1, 3], 1, Concat, [1]] # 7 cat backbone P4
- [-1, 3, C2f, [512]] # 8
- [-1, 1, nn.Upsample, [None, 2, 'nearest']] # 9
- [[-1, 2], 1, Concat, [1]] # 10 cat backbone P3
- [-1, 3, C2f, [256]] # 11 (P3/8-small)
- [-1, 1, Conv, [256, 3, 2]] # 12
- [[-1, 8], 1, Concat, [1]] # 13 cat head P4
- [-1, 3, C2f, [512]] # 14 (P4/16-medium)
- [-1, 1, Conv, [512, 3, 2]] # 15
- [[-1, 5], 1, Concat, [1]] # 16 cat head P5
- [-1, 3, C2f, [1024]] # 17 (P5/32-large)
- [[11, 14, 17], 1, Detect, [nc]] # Detect(P3, P4, P5)
实验
脚本
import os
from ultralytics import YOLO
yaml = 'ultralytics/cfg/models/v8/yolov8_EfficientNet.yaml'
model = YOLO(yaml)
model.info()
if __name__ == "__main__":
results = model.train(data='ultralytics/datasets/original-license-plates.yaml',
name='yolov8_EfficientNet',
epochs=10,
workers=8,
batch=1)
结果
文章目录
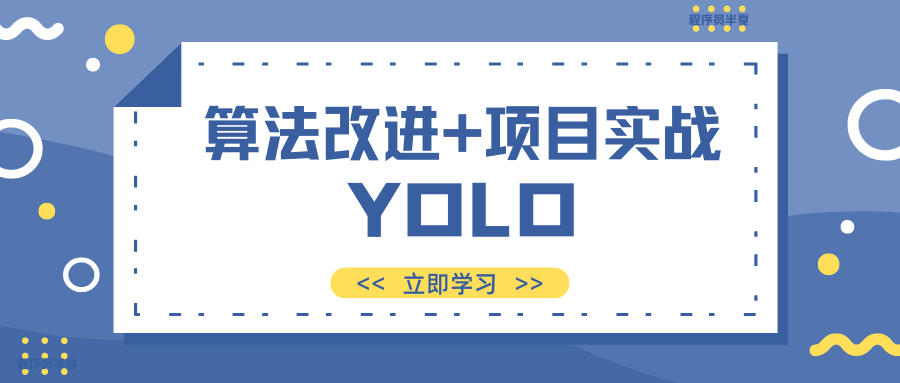