文章目录
一、前言
我之前狭隘地以为只有点云深度学习才能处理分割问题,偶然发现传统方法的分割结果一样可以令我眼前一亮。这三篇论文原文是:VCCS原文 LCCP原文 CPC原文
其中VCCS是分割前的准备工作,LCCP和CPC是分割的方法,二者论文中的效果图如下,有点出乎我的意料。。。。
传统方法我认为最好的点在于通用性很强,并且可解释,应用起来比较友好。这三篇论文出自同一实验室,并且算法实现均已发布到PCL中,所以我准备学习这三篇论文的方法,并整理成笔记与大家交流。
二、VCCS论文阅读
2.1 摘要部分
在2D图像分割任务中,通常会将相似的像素先聚类成超像素(superpixel),通过这步预处理,可以有效降低后续分割的计算开销。2D超像素在3D中对应超体素(supervoxel),传统方法通过比较颜色和深度的差异来完成超体素的生成,但容易出现跨越物体边界的情况,本文解决这个问题的思路是利用三维几何信息。在3D空间中操作与在投影空间中相比有一明显的优势,投影空间只能处理2.5D的点云(使用RGB-D相机进行一次拍摄),而在3D空间中可以处理3D的情况(使用多个标定好的相机进行拍摄)。实验结果证明了提出方法在抑制跨越边界以及处理速度上的优越表现。
2.2 方法
后文中 V r ( i ) = F 1.. n V_r(i)=F_{1..n} Vr(i)=F1..n,代表分辨率为 r r r的体素云中索引为 i i i的体素,体素用 F F F来描述, F F F是一个 n n n维特征向量,包含了体素的颜色、位置、法线等信息。
2.2.1 Adjacency Graph
构建邻接图是本文提出方法中的关键,对于体素化的三维空间,有三种邻接的定义方式,6-,18-和26邻接,可以用魔方来辅助理解,6邻接包含六个面的中心块,18邻接再包含12个棱块,26邻接则是再包含8个角块。遍历每个体素,使用
3
R
v
o
x
e
l
\sqrt 3R_{voxel}
3Rvoxel的搜索半径即可完成邻接图的构建
最后一句话写的怪怪的,最后重新修改一下,这个邻接图到底是啥
2.2.2 Spatial Seeding
种子点会被用于初始化超体素,首先以
R
s
e
e
d
R_{seed}
Rseed的分辨率对空间再做一次栅格化,
R
s
e
e
d
R_{seed}
Rseed需要大于
R
v
o
x
e
l
R_{voxel}
Rvoxel,这很容易理解,因为超体素中需要包含多个体素。然后找到每个大体素(
R
s
e
e
d
R_{seed}
Rseed)中距离中心最近的小体素(
R
v
o
x
e
l
R_{voxel}
Rvoxel)作为初始种子体素。未被占据的大体素将不被考虑。
R
s
e
e
d
R_{seed}
Rseed的大小会直接影响到最后超体素的大小
接着处理噪声,目的是去除因空间中离散的噪声体素而生成的种子点,方法如下:
对于被查询的种子体素,以
R
s
e
a
r
c
h
R_{search}
Rsearch半径搜索其周围的体素个数
N
N
N,
R
s
e
a
r
c
h
R_{search}
Rsearch小于
R
s
e
e
d
R_{seed}
Rseed但大于
R
v
o
x
e
l
R_{voxel}
Rvoxel,在代码中使用的是
0.5
R
s
e
e
d
0.5R_{seed}
0.5Rseed。如果
N
N
N小于某个阈值,则认为是噪声。阈值的确定,结合示意图,我猜测是统计过查询体素的平面(图中的绿色平面)会包含多少在
R
s
e
a
r
c
h
R_{search}
Rsearch范围内的体素,以此作为阈值。代码实现使用了一个经验值:
N
=
0.05
×
R
s
e
a
r
c
h
2
∗
π
R
v
o
x
e
l
2
N=0.05\times \frac{R_{search}^2*\pi}{R_{voxel}^2}
N=0.05×Rvoxel2Rsearch2∗π
滤除噪声后,计算搜索范围内体素的梯度,将梯度最小的体素确定为最终的种子体素。梯度的计算公式如下:
G
(
i
)
=
∑
k
∈
V
adj
∥
V
(
i
)
−
V
(
k
)
∥
C
I
E
L
a
b
N
a
d
j
,
G(i) = \sum_{k \in V_{\text{adj}}} \frac{\| V(i) - V(k) \|_{CIELab}}{N_{adj}},
G(i)=k∈Vadj∑Nadj∥V(i)−V(k)∥CIELab,
CIELab是一种色彩空间,与我们熟悉的RGB、HSV等均用来描述颜色,
- L* 表示亮度,从0(黑色)到100(白色)。
- a* 表示从绿色到红色的颜色对立。
- b* 表示从蓝色到黄色的颜色对立。
他的优势在于可以量化颜色的差异,回想RGB,RGB空间上的距离其实并不能让我们直观的感受出颜色的差异,而在CIELab空间中,数值相差的多少与我们眼睛直观感受到颜色的差异是成正比的。这个地方其实考虑的不是很周到,因为并不是所有点云数据都有对应的颜色信息。
2.2.3 Features and Distance Measure
VCCS的超体素是用一个39维度的向量描述的
F
=
[
x
,
y
,
z
,
L
,
a
,
b
,
F
P
F
H
1..33
]
F=[x, y, z, L, a, b, FPFH_{1..33}]
F=[x,y,z,L,a,b,FPFH1..33]
F
P
F
H
FPFH
FPFH是点云的一种特征描述子,具有位姿不变性,用来描述点云的几何特性,计算复杂度是
O
(
n
⋅
k
)
O(n\cdot k)
O(n⋅k),
n
n
n是点云个数,
k
k
k是计算特征子时所考虑的邻域点个数。
为了计算
F
F
F之间的距离
D
D
D,需要先对各部分做归一化,这很容易理解,因为不同物理量的单位不同,不能使用相同的权重。公式中的
m
m
m是一个常数,没啥用。。。。论文中并没有明确指出测试使用的权重,实际需要根据自己对不同特征的侧重来选择
D
=
λ
D
c
2
m
2
+
μ
D
s
2
3
R
s
e
e
d
+
ϵ
D
H
i
K
2
D = \sqrt{\frac{\lambda D_c^2}{m^2} + \frac{\mu D_s^2}{3R_{seed}} + \epsilon D_{HiK}^2}
D=m2λDc2+3RseedμDs2+ϵDHiK2
2.2.4 Flow Constrained Clustering
本文的聚类方法是k-means的一种变体,会考虑到连通性和流动性。
2.2.4.1 k-means算法
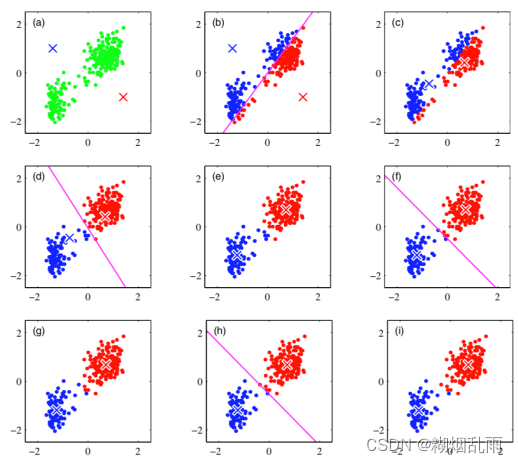
2.2.4.2 本文的改进
从最靠近聚类中心的体素开始,向外流动到相邻的体素,计算与聚类中心的距离(用上一节的距离计算公式),如果距离是该体素见过的最小值,就会设置其标签,并使用邻接图将其邻居均添加到这个标签的搜索队列中。然后处理下一个超体素,这样所有超体素都能同时的向外进行。“We proceed iteratively outwards until we have reached the edge of the search volume for each supervoxel (or have no more neighbors to check).” 结束条件没看懂。。。。
一旦所有超体素邻接图搜索结束,通过取每个组成部分的均值来更新每个超体素聚类的中心,不断迭代,直到聚类中心稳定下来。作者提到,VCCS使用五次迭代就基本稳定下来了。
这种改进的优势在于,超体素的标签不能跨越在3D空间中不接触的物体边界,因为我们只考虑相邻的体素
2.3 PCL代码
测试代码来自pcl的应用示例
#include <pcl/console/parse.h>
#include <pcl/point_cloud.h>
#include <pcl/point_types.h>
#include <pcl/io/pcd_io.h>
#include <pcl/visualization/pcl_visualizer.h>
#include <pcl/segmentation/supervoxel_clustering.h>
//VTK include needed for drawing graph lines
#include <vtkPolyLine.h>
// Types
typedef pcl::PointXYZRGBA PointT;
typedef pcl::PointCloud<PointT> PointCloudT;
typedef pcl::PointNormal PointNT;
typedef pcl::PointCloud<PointNT> PointNCloudT;
typedef pcl::PointXYZL PointLT;
typedef pcl::PointCloud<PointLT> PointLCloudT;
void addSupervoxelConnectionsToViewer (PointT &supervoxel_center,
PointCloudT &adjacent_supervoxel_centers,
std::string supervoxel_name,
pcl::visualization::PCLVisualizer::Ptr & viewer);
int
main (int argc, char ** argv)
{
if (argc < 2)
{
pcl::console::print_error ("Syntax is: %s <pcd-file> \n "
"--NT Dsables the single cloud transform \n"
"-v <voxel resolution>\n-s <seed resolution>\n"
"-c <color weight> \n-z <spatial weight> \n"
"-n <normal_weight>\n", argv[0]);
return (1);
}
PointCloudT::Ptr cloud (new PointCloudT);
pcl::console::print_highlight ("Loading point cloud...\n");
if (pcl::io::loadPCDFile<PointT> (argv[1], *cloud))
{
pcl::console::print_error ("Error loading cloud file!\n");
return (1);
}
bool disable_transform = pcl::console::find_switch (argc, argv, "--NT");
float voxel_resolution = 0.008f;
bool voxel_res_specified = pcl::console::find_switch (argc, argv, "-v");
if (voxel_res_specified)
pcl::console::parse (argc, argv, "-v", voxel_resolution);
float seed_resolution = 0.1f;
bool seed_res_specified = pcl::console::find_switch (argc, argv, "-s");
if (seed_res_specified)
pcl::console::parse (argc, argv, "-s", seed_resolution);
float color_importance = 0.2f;
if (pcl::console::find_switch (argc, argv, "-c"))
pcl::console::parse (argc, argv, "-c", color_importance);
float spatial_importance = 0.4f;
if (pcl::console::find_switch (argc, argv, "-z"))
pcl::console::parse (argc, argv, "-z", spatial_importance);
float normal_importance = 1.0f;
if (pcl::console::find_switch (argc, argv, "-n"))
pcl::console::parse (argc, argv, "-n", normal_importance);
// //
// This is how to use supervoxels
// //
pcl::SupervoxelClustering<PointT> super (voxel_resolution, seed_resolution);
if (disable_transform)
super.setUseSingleCameraTransform (false);
super.setInputCloud (cloud);
super.setColorImportance (color_importance);
super.setSpatialImportance (spatial_importance);
super.setNormalImportance (normal_importance);
std::map <std::uint32_t, pcl::Supervoxel<PointT>::Ptr > supervoxel_clusters;
pcl::console::print_highlight ("Extracting supervoxels!\n");
super.extract (supervoxel_clusters);
pcl::console::print_info ("Found %d supervoxels\n", supervoxel_clusters.size ());
pcl::visualization::PCLVisualizer::Ptr viewer (new pcl::visualization::PCLVisualizer ("3D Viewer"));
viewer->setBackgroundColor (0, 0, 0);
PointCloudT::Ptr voxel_centroid_cloud = super.getVoxelCentroidCloud ();
viewer->addPointCloud (voxel_centroid_cloud, "voxel centroids");
viewer->setPointCloudRenderingProperties (pcl::visualization::PCL_VISUALIZER_POINT_SIZE,2.0, "voxel centroids");
viewer->setPointCloudRenderingProperties (pcl::visualization::PCL_VISUALIZER_OPACITY,0.95, "voxel centroids");
PointLCloudT::Ptr labeled_voxel_cloud = super.getLabeledVoxelCloud ();
viewer->addPointCloud (labeled_voxel_cloud, "labeled voxels");
viewer->setPointCloudRenderingProperties (pcl::visualization::PCL_VISUALIZER_OPACITY,0.8, "labeled voxels");
PointNCloudT::Ptr sv_normal_cloud = super.makeSupervoxelNormalCloud (supervoxel_clusters);
//We have this disabled so graph is easy to see, uncomment to see supervoxel normals
//viewer->addPointCloudNormals<PointNormal> (sv_normal_cloud,1,0.05f, "supervoxel_normals");
pcl::console::print_highlight ("Getting supervoxel adjacency\n");
std::multimap<std::uint32_t, std::uint32_t> supervoxel_adjacency;
super.getSupervoxelAdjacency (supervoxel_adjacency);
//To make a graph of the supervoxel adjacency, we need to iterate through the supervoxel adjacency multimap
for (auto label_itr = supervoxel_adjacency.cbegin (); label_itr != supervoxel_adjacency.cend (); )
{
//First get the label
std::uint32_t supervoxel_label = label_itr->first;
//Now get the supervoxel corresponding to the label
pcl::Supervoxel<PointT>::Ptr supervoxel = supervoxel_clusters.at (supervoxel_label);
//Now we need to iterate through the adjacent supervoxels and make a point cloud of them
PointCloudT adjacent_supervoxel_centers;
for (auto adjacent_itr = supervoxel_adjacency.equal_range (supervoxel_label).first; adjacent_itr!=supervoxel_adjacency.equal_range (supervoxel_label).second; ++adjacent_itr)
{
pcl::Supervoxel<PointT>::Ptr neighbor_supervoxel = supervoxel_clusters.at (adjacent_itr->second);
adjacent_supervoxel_centers.push_back (neighbor_supervoxel->centroid_);
}
//Now we make a name for this polygon
std::stringstream ss;
ss << "supervoxel_" << supervoxel_label;
//This function is shown below, but is beyond the scope of this tutorial - basically it just generates a "star" polygon mesh from the points given
addSupervoxelConnectionsToViewer (supervoxel->centroid_, adjacent_supervoxel_centers, ss.str (), viewer);
//Move iterator forward to next label
label_itr = supervoxel_adjacency.upper_bound (supervoxel_label);
}
while (!viewer->wasStopped ())
{
viewer->spinOnce (100);
}
return (0);
}
void
addSupervoxelConnectionsToViewer (PointT &supervoxel_center,
PointCloudT &adjacent_supervoxel_centers,
std::string supervoxel_name,
pcl::visualization::PCLVisualizer::Ptr & viewer)
{
vtkSmartPointer<vtkPoints> points = vtkSmartPointer<vtkPoints>::New ();
vtkSmartPointer<vtkCellArray> cells = vtkSmartPointer<vtkCellArray>::New ();
vtkSmartPointer<vtkPolyLine> polyLine = vtkSmartPointer<vtkPolyLine>::New ();
//Iterate through all adjacent points, and add a center point to adjacent point pair
for (auto adjacent_itr = adjacent_supervoxel_centers.begin (); adjacent_itr != adjacent_supervoxel_centers.end (); ++adjacent_itr)
{
points->InsertNextPoint (supervoxel_center.data);
points->InsertNextPoint (adjacent_itr->data);
}
// Create a polydata to store everything in
vtkSmartPointer<vtkPolyData> polyData = vtkSmartPointer<vtkPolyData>::New ();
// Add the points to the dataset
polyData->SetPoints (points);
polyLine->GetPointIds ()->SetNumberOfIds(points->GetNumberOfPoints ());
for(unsigned int i = 0; i < points->GetNumberOfPoints (); i++)
polyLine->GetPointIds ()->SetId (i,i);
cells->InsertNextCell (polyLine);
// Add the lines to the dataset
polyData->SetLines (cells);
viewer->addModelFromPolyData (polyData,supervoxel_name);
}
效果以及调参建议
- 需要调整的参数有五个 R s e e d R_{seed} Rseed, R v o x e l R_{voxel} Rvoxel, λ \lambda λ, μ \mu μ, ϵ \epsilon ϵ,对于没有RGB信息的点云,颜色权重可以设为0
- 比较好的效果应该如下图所示(注释掉了
addSupervoxelConnectionsToViewer
)
不同颜色代表不同的supervoxel,放大点云观察物体交界的地方,可以看到VCCS确实具有抑制supervoxel越过物体边界的效果。降低normal_importance之后,效果减弱,supervoxel会跨越边界。 - 如果发现有空洞的情况出现,下图进行了一种比较极端的展示
这种情况是因为 R v o x e l R_{voxel} Rvoxel设置的太小,已经接近了实际点云的点间距,尤其是对于RGB-D数据,距离越远点云越稀疏,所以出现了很多没被栅格化的区域;而同时 R s e e d R_{seed} Rseed设置的太大,导致很多点被当作离群点去除了。