作者 | 陈冠英 编辑 | 极市平台
原文链接:https://zhuanlan.zhihu.com/p/700895749
点击下方卡片,关注“自动驾驶之心”公众号
戳我-> 领取自动驾驶近15个方向学习路线
本文只做学术分享,如有侵权,联系删文
导读
3D生成领域从2023年末到2024年的一些新进展的概述。
去年我整理了一篇关于“Diffusion Model for 2D/3D Generation 相关论文分类”的知乎文章(https://zhuanlan.zhihu.com/p/617510702),简单列出了一些基于扩散模型实现2D/3D生成的论文。
一年过去了,3D生成领域得到了快速的发展。这篇文章列举了从2023年末到今年的一些工作。由于篇幅有限和时间跨度较长,有很多重要的文献没有列出,敬请理解,并欢迎在评论区指出。
回顾2023 上半年的一些工作
在2023年初,随着DreamFusion工作的爆火,基于扩散模型的3D生成方法主要通过利用2D扩散模型升维实现。由于需要对每个物体进行单独优化,这些方法通常需要较长的计算时间。以下是2023年上半年中几个重要的3D生成工作(因篇幅所限,还有很多重要的工作未列出):
DreamFusion (ICLR23) / Score Jacobian Chaining (CVPR23):提出利用2D扩散模型优化3D模型的方法
Magic3D (CVPR23): 利用两阶段coarse-to-fine的策略,大幅提升了生成的质量
Zero123 (ICCV23):展示了微调扩散模型可以实现新视角生成,并且可以保持较强的泛化性。
Fantasia3D (ICCV 23): 提出解耦几何与材质的生成方法,展示了很强的几何细节
ProlificDreamer (NeurIPS23): 提出VSD和一些技巧将3D生成的图像渲染视觉效果提升到一个新的高度
MVDream (ICLR24): 微调stable diffusion实现多视角图像生成的扩散模型,多视角图像生成可以更好的保持三维一致性,与减少多脸的问题
2024年的一些观察
下面是对3D生成领域近半年发展趋势的一些简单观察:
高效三维表征的使用:2023下半年,3D高斯表示的出现在很大程度上替代了NeRF。近期的工作大多与3D高斯表示有关。
更关注生成效率:相比于2D Diffusion升维方法需要动辄几个小时的优化时间,现在的3D生成方法可以在几秒内完成,极大提升了生成效率。
结合视频生成模型:轨迹连续的三维物体视图可以看作一段视频,结合视频生成模型(如Stable Video Diffusion, SVD)来辅助三维物体的生成是一个自然的思路。另外,视频生成模型在4D物体生成领域也起到了至关重要的作用。
利用Transformer的前向计算实现生成:一些工作开始使用Transformer直接学习图像到三维表示的映射(例如大重建模型LRM),从而无需较长时间的优化。
考虑材质的建模:三维物体不仅包含几何属性,还包含材质属性。最近有越来越多的工作关注PBR材质的生成,而不仅仅是生成表面颜色(耦合了光照与材质)。
考虑更大规模的场景生成:最早大家关注的是物体级别的生成,现在有很多工作在研究室内场景、室外场景乃至城市级别场景的生成。
组合式生成:不仅是简单地用一个全局的三维表示表达整个空间,而是每个小物体或者部件有独立的三维表示,从而可以更好地实现编辑和重组。
4D动态场景生成:从3D生成迁移到4D生成是一个很自然的方向,且有更大的发挥空间。3D生成领域目前已经竞争激烈,工作同质化问题日益严重(该文章没有列举4D生成相关论文,后续会再抽空梳理)。
更多资料
下面我列一下近期的综述(简单检索到,可能会漏掉)、Github awesome系列、常看的自媒体。可以了解最新的技术进展。
Survey
Advances in 3D Generation: A Survey: https://arxiv.org/abs/2401.17807
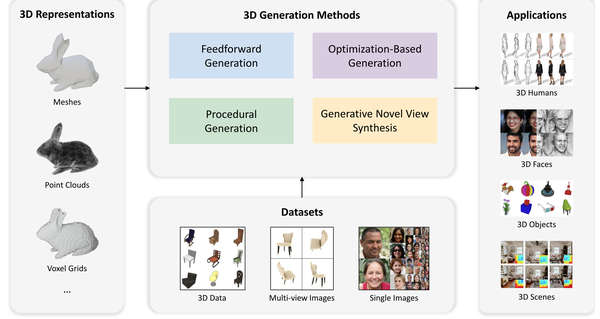
A Comprehensive Survey on 3D Content Generation: https://arxiv.org/abs/2402.01166
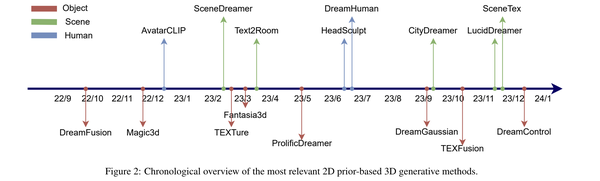
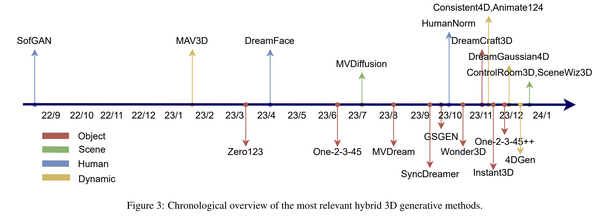
State of the Art on Diffusion Models for Visual Computing
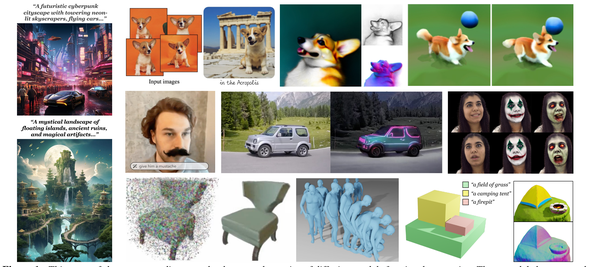
Recent Advances in 3D Gaussian Splatting: https://arxiv.org/abs/2403.11134
A Survey on 3D Gaussian Splatting: https://arxiv.org/abs/2401.03890
Awesome系列
Awesome-AIGC-3D: https://github.com/hitcslj/Awesome-AIGC-3D
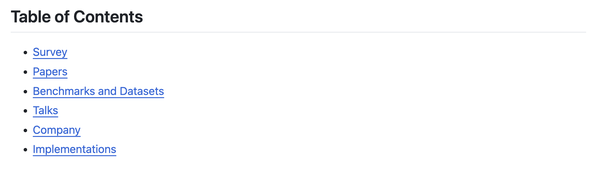
Awesome-CVPR2024-AIGC: https://github.com/Kobaayyy/Awesome-CVPR2024-AIGC
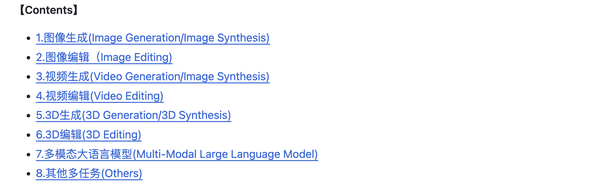
awesome-4d-generation: https://github.com/cwchenwang/awesome-4d-generation
自媒体
NeRF 3DGS日报:https://www.zhihu.com/people/yang-ji-heng-42 (每日更新最新的arxiv的论文)
公众号AIGC Research:https://mp.weixin.qq.com/s/v92K8vnpdSaBU6jzViJ38g
AI产品汇: https://mp.weixin.qq.com/s/zM7RPWyIggWg92X0P_veDQ
3D 扩散模型
Shap-E: Generating Conditional 3D Implicit Functions, arXIV
Point-E的改进版,利用扩散模型生成SDF MLP的权重。
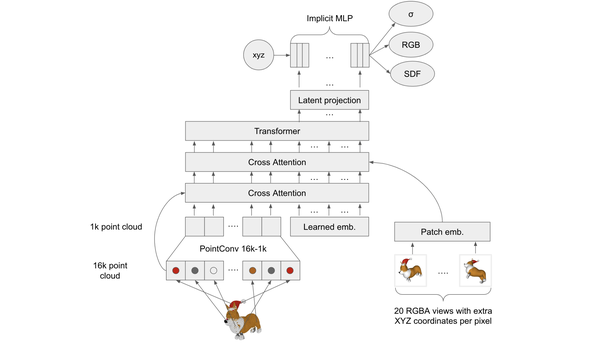
One-2-3-45++: Fast Single Image to 3D Objects with Consistent Multi-View Generation and 3D Diffusion, CVPR24
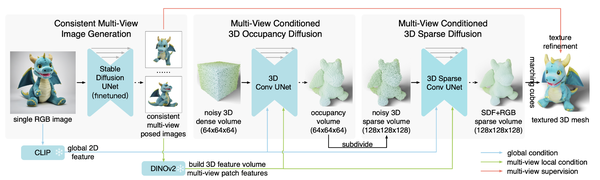
Text-to-3D Generation with Bidirectional Diffusion using both 2D and 3D priors, CVPR24
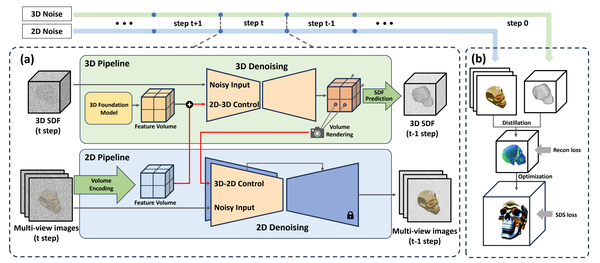
3DTopia: Large Text-to-3D Generation Model with Hybrid Diffusion Priors, arXiv24
沿着3DGen (3DGen: Triplane Latent Diffusion for Textured Mesh Generation)的思路,利用扩散模型生成基于Triplane的三维表示
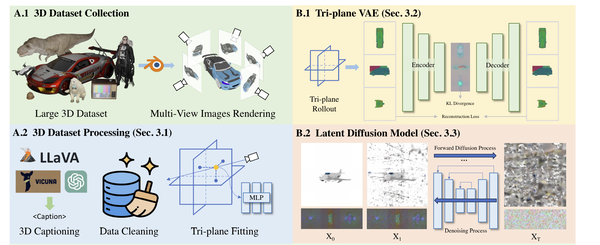
Direct3D: Scalable Image-to-3D Generation via 3D Latent Diffusion Transformer, arXiv24
也是沿着3DGen的思路,采用了Diffusion Transformer (DiT)的结构。该工作专注图像到几何的生成,
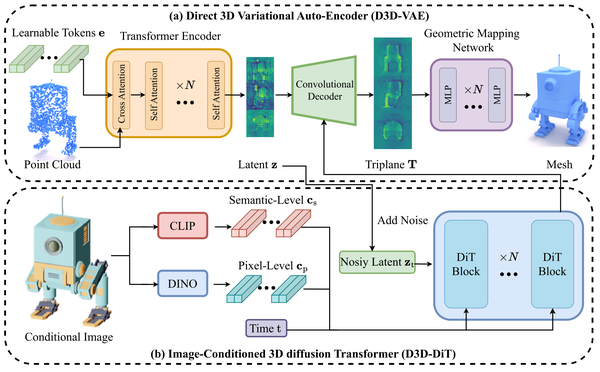
引入几何先验
引入法线、点云等几何先验帮助3D生成
Wonder3D: Single Image to 3D using Cross-Domain Diffusion
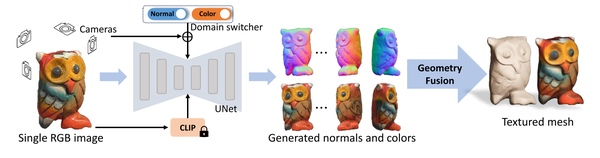
GaussianDreamer: Fast Generation from Text to 3D Gaussians by Bridging 2D and 3D Diffusion Models, CVPR24
利用Shape-E作为几何先验
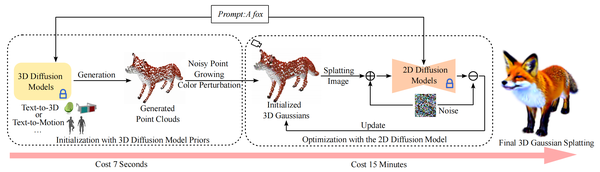
SweetDreamer: Aligning Geometric Priors in 2D Diffusion for Consistent Text-to-3D, ICLR24
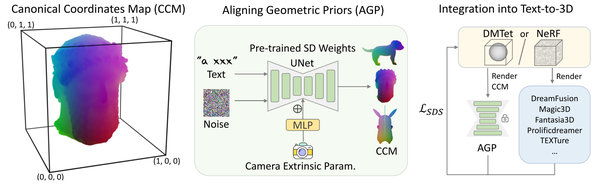
RichDreamer: A Generalizable Normal-Depth Diffusion Model for Detail Richness in Text-to-3D, CVPR24
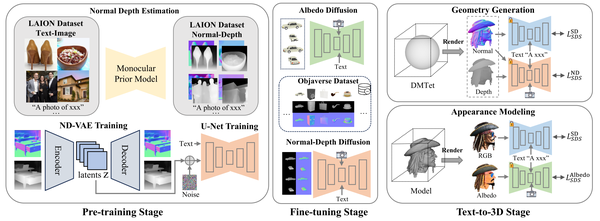
EpiDiff: Enhancing Multi-View Synthesis via Localized Epipolar-Constrained Diffusion, CVPR24
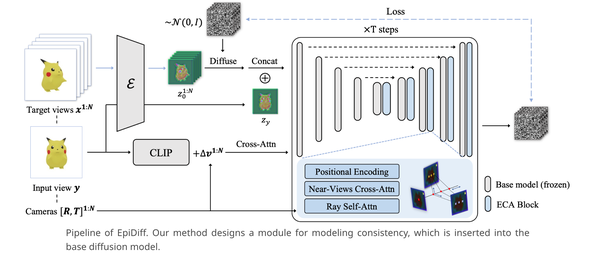
大重建模型(LRM)
Adobe这一系列工作均发表(LRM, PF-LRM, DMV3D, Instant3D)在ICLR 2024,主要思路是利用Transformer直接学习图像到三维表达(Triplane)的映射。
One-2-3-45: Any Single Image to 3D Mesh in 45 Seconds without Per-Shape Optimization
该工作不叫大重建模型,但结合多视角重建实现单图的三维生成,所以也列在此。给定一张图像,该工作首先利用zero123生成多视角图像,然后构建cost volume并用一个3D卷积网络估计SDF场的特征。
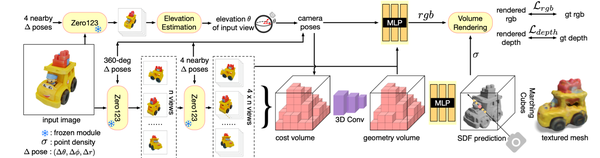
LRM: Large Reconstruction Model for Single Image to 3D, ICLR24
2D图像特征和3D表示使用cross-attention进行关联
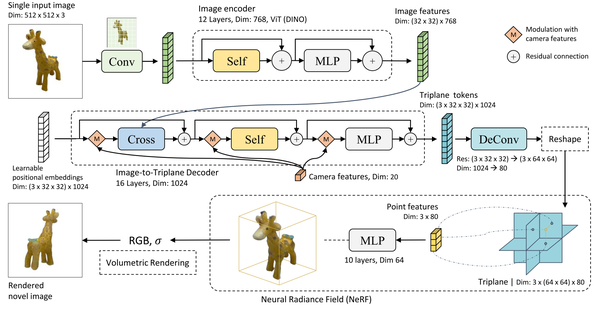
PF-LRM: Pose-Free Large Reconstruction Model for Joint Pose and Shape Prediction, ICLR24
2D图像特征和3D表示使用self-attention进行关联
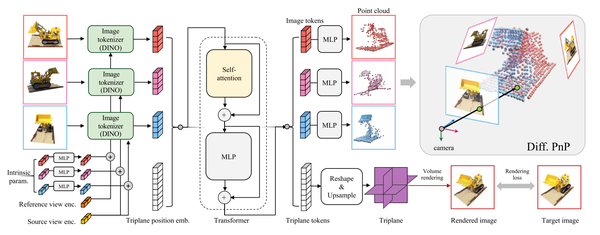
DMV3D:Denoising Multi-View Diffusionusing 3D Large Reconstruction Model, ICLR24
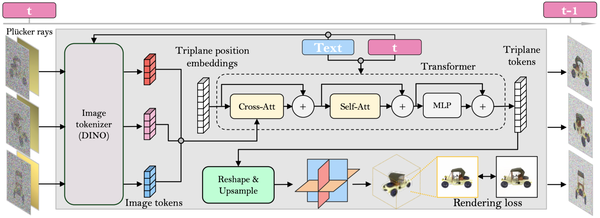
Instant3D: Fast Text-to-3D with Sparse-View Generation and Large Reconstruction Model, ICLR24
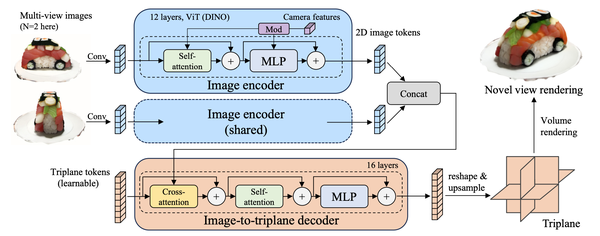
Triplane Meets Gaussian Splatting: Fast and Generalizable Single-View 3D Reconstruction with Transformers, arXiv24
提出结合3D高斯和Triplane的混合表征,加速LRM。高斯点的位置使用3D点云表达。
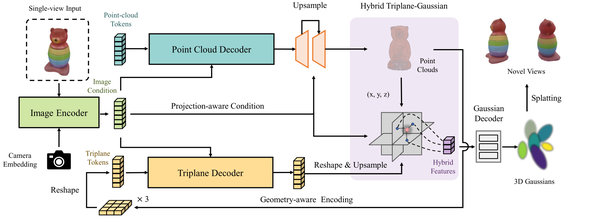
Splatter Image: Ultra-Fast Single-View 3D Reconstruction, CVPR24
提出利用图像存储3D高斯的参数,每一个像素存储一个3D高斯。这样就可以利用一个网络直接回归图像到3D高斯的参数。同时期的LGM和GRM采用了类似的思路("Pixel-aligned Gaussian")。
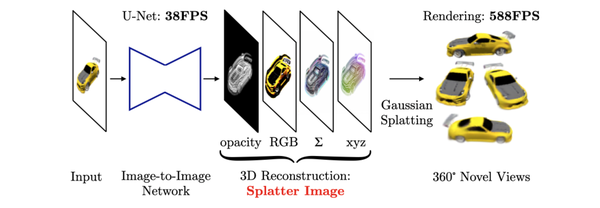
LGM: Large Multi-View Gaussian Model for High-Resolution 3D Content Creation, arXiv24
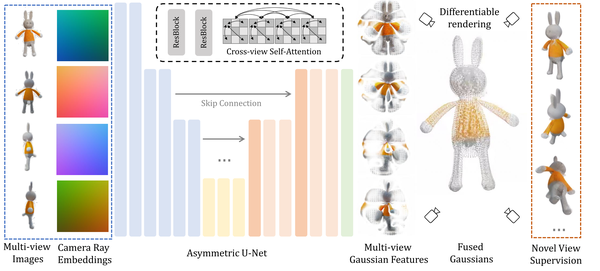
GRM: Large gaussian reconstruction model for efficient 3d reconstruction and generation, arXiv24
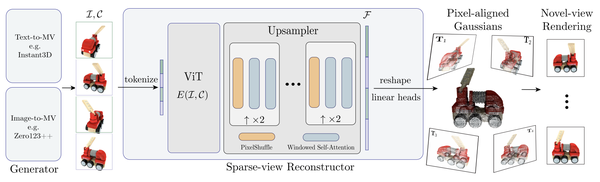
结合视频生成模型(多视角图像生成)
Stable Video Diffusion: Scaling Latent Video Diffusion Models to Large Datasets, arXiv23
这篇论展示了SVD-MV,利用Stable Video Diffusion生成多视角图像实现3D生成
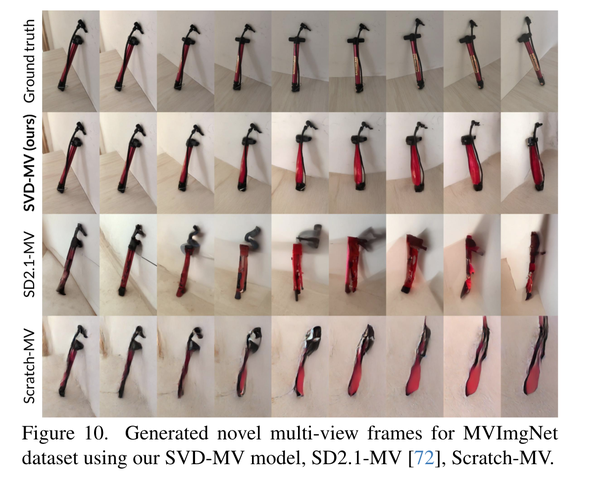
ViVid-1-to-3: Novel View Synthesis with Video Diffusion Models, CVPR24
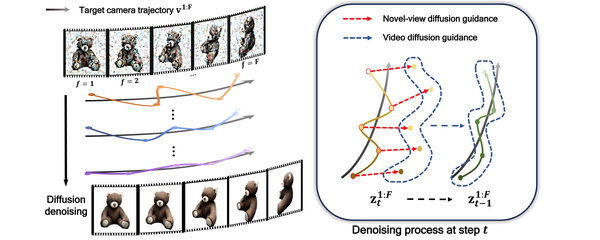
V3D: Video Diffusion Models are Effective 3D Generators, arXiv24
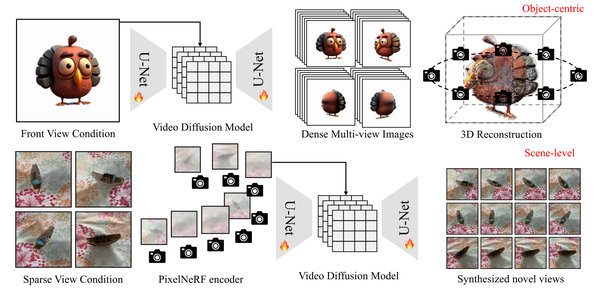
SV3D: Novel Multi-view Synthesis and 3D Generation from a Single Image using Latent Video Diffusion, arXiv24
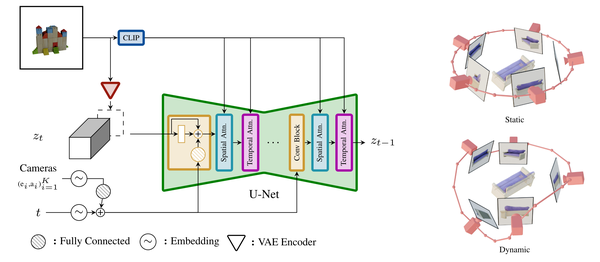
CAT3D: Create Anything in 3D with Multi-View Diffusion Models
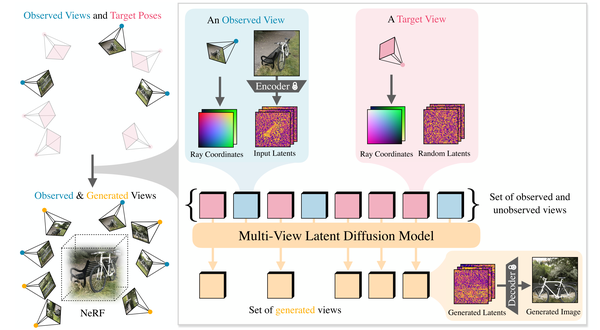
VideoMV: Consistent Multi-View Generation Based on Large Video Generative Model, arXiv24
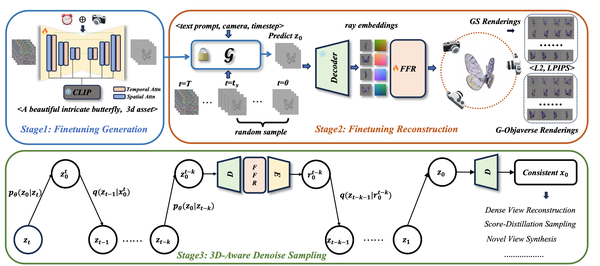
PBR材质的生成
DreamMat: High-quality PBR Material Generation with Geometry- and Light-aware Diffusion Modelst, SIGGRAPH24
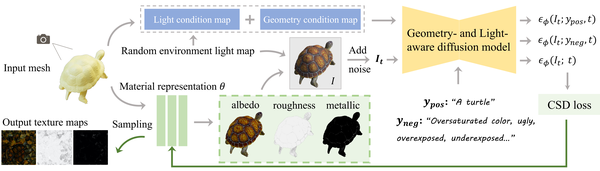
Collaborative Control for Geometry-Conditioned PBR Image Generation, arXiv24
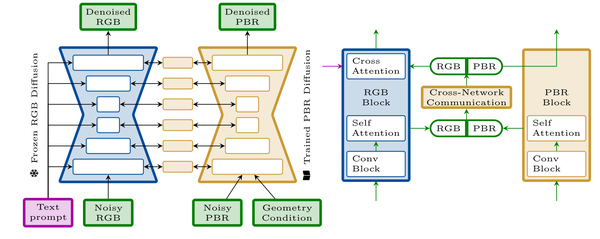
MaPa: Text-driven Photorealistic Material Painting for 3D Shapes, SIGGRAPH 2024
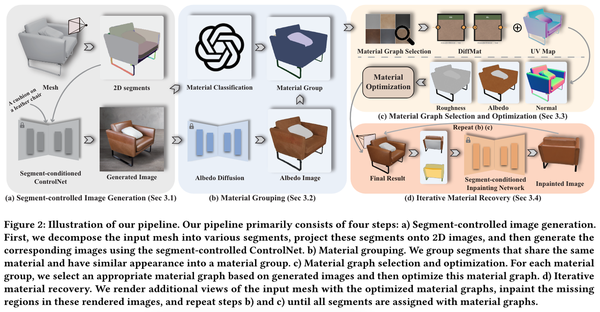
Make-it-Real: Unleashing Large Multimodal Model's Ability for Painting 3D Objects with Realistic Materials, arXiv24
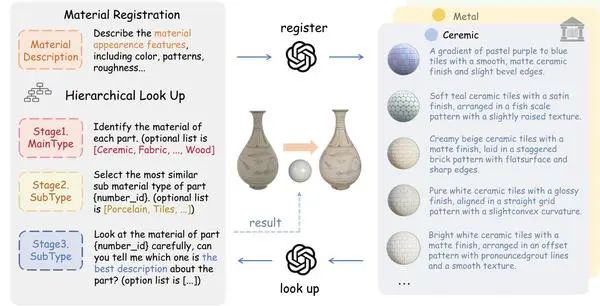
SceneTex: High-Quality Texture Synthesis for Indoor Scenes via Diffusion Priors, CVPR24]
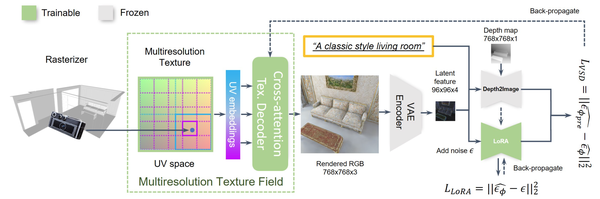
Paint-it: Text-to-Texture Synthesis via Deep Convolutional Texture Map Optimization and Physically-Based Rendering, CVPR24
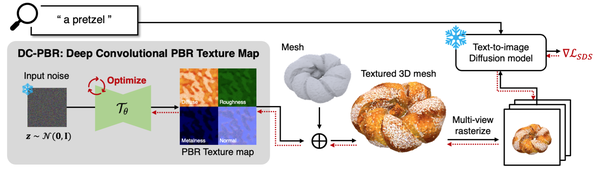
数字人/衣服生成
数字人生成的有很多很好的工作,这里仅简单列几个工作。
HumanNorm: Learning Normal Diffusion Model for High-quality and Realistic 3D Human Generation, CVPR24
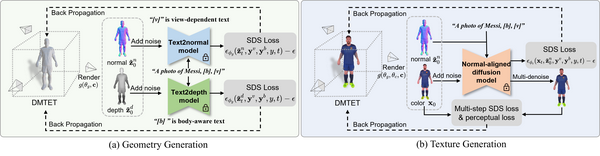
Make-A-Character: High Quality Text-to-3D Character Generation within Minutes, arXiv23
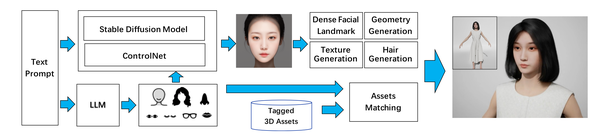
HumanGaussian: Text-Driven 3D Human Generation with Gaussian Splatting, CVPR24
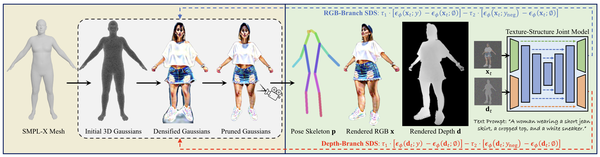
GaussianAvatars: Photorealistic Head Avatars with Rigged 3D Gaussians, CVPR24
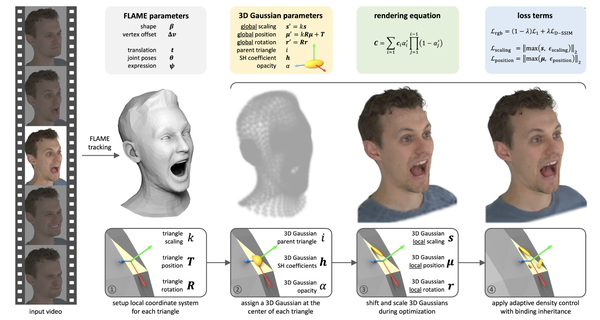
DressCode: Autoregressively Sewing and Generating Garments from Text Guidance, arXiv24
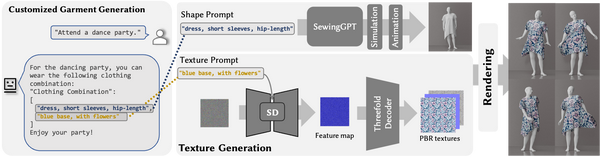
TELA: Text to Layer-wise 3D Clothed Human Generation, arXiv24
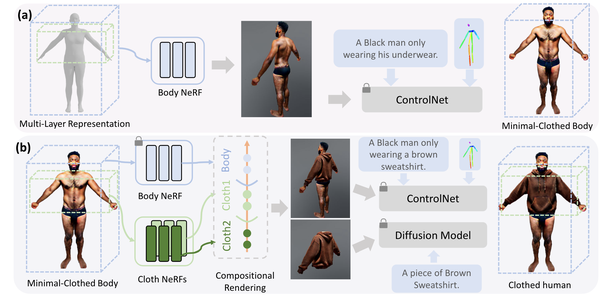
室内场景生成
Text2Room: Extracting Textured 3D Meshes from 2D Text-to-Image Models, ICCV23
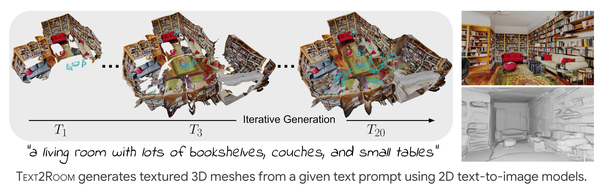
MVDiffusion: Enabling Holistic Multi-view Image Generation with Correspondence-Aware Diffusion, NeurIPS 23
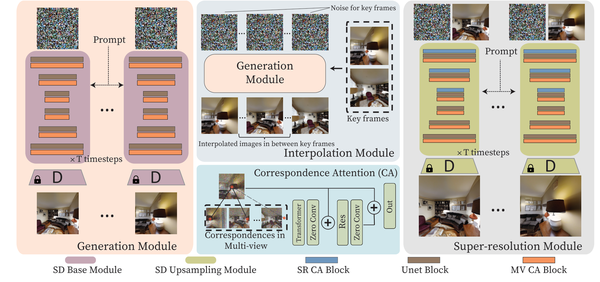
Ctrl-Room: Controllable Text-to-3D Room Meshes Generation with Layout Constraints, arXiv23
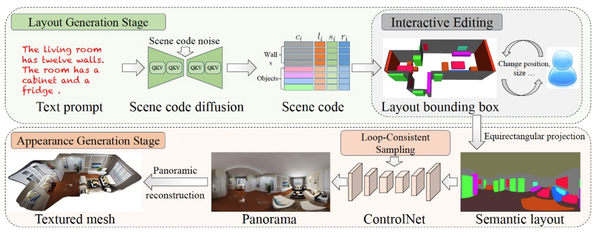
ControlRoom3D: Room Generation using Semantic Controls, CVPR24
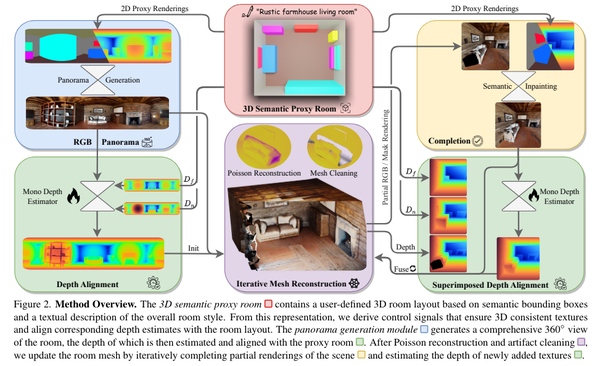
室外大场景生成
CityDreamer: Compositional Generative Model of Unbounded 3D Cities, CVPR24
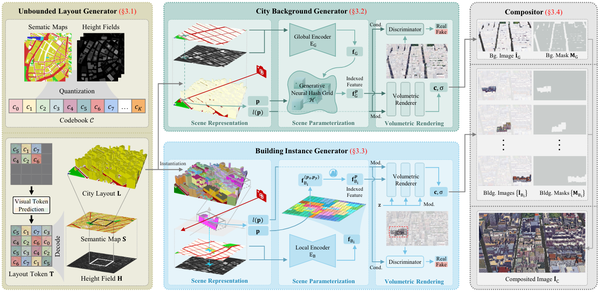
CityGen: Infinite and Controllable 3D City Layout Generation, arXiv24
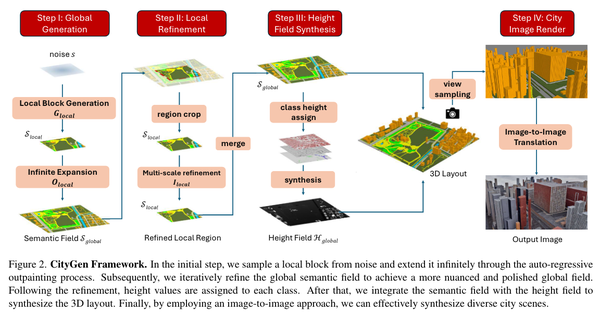
Sat2Scene: 3D Urban Scene Generation from Satellite Images with Diffusion, CVPR24
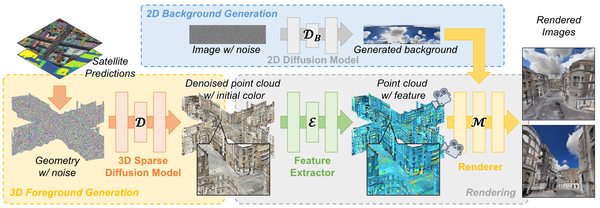
DreamScene360: Unconstrained Text-to-3D Scene Generation with Panoramic Gaussian Splatting, arXiv24
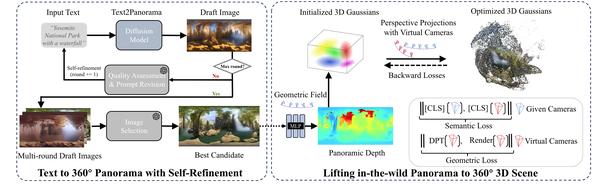
SceneX : Procedural Controllable Large-scale Scene Generation via Large-language Models, arXiv24
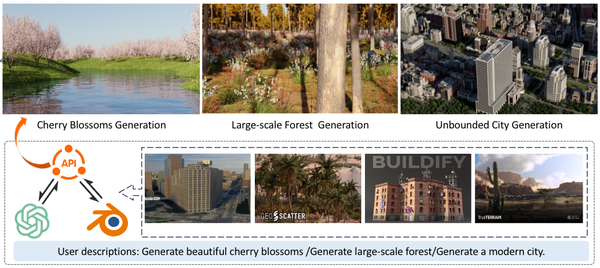
投稿作者为『自动驾驶之心知识星球』特邀嘉宾,欢迎加入交流!
① 全网独家视频课程
BEV感知、BEV模型部署、BEV目标跟踪、毫米波雷达视觉融合、多传感器标定、多传感器融合、多模态3D目标检测、车道线检测、轨迹预测、在线高精地图、世界模型、点云3D目标检测、目标跟踪、Occupancy、cuda与TensorRT模型部署、大模型与自动驾驶、Nerf、语义分割、自动驾驶仿真、传感器部署、决策规划、轨迹预测等多个方向学习视频(扫码即可学习)
② 国内首个自动驾驶学习社区
国内最大最专业,近3000人的交流社区,已得到大多数自动驾驶公司的认可!涉及30+自动驾驶技术栈学习路线,从0到一带你入门自动驾驶感知(2D/3D检测、语义分割、车道线、BEV感知、Occupancy、多传感器融合、多传感器标定、目标跟踪)、自动驾驶定位建图(SLAM、高精地图、局部在线地图)、自动驾驶规划控制/轨迹预测等领域技术方案、大模型、端到端等,更有行业动态和岗位发布!欢迎扫描下方二维码,加入自动驾驶之心知识星球,这是一个真正有干货的地方,与领域大佬交流入门、学习、工作、跳槽上的各类难题,日常分享论文+代码+视频
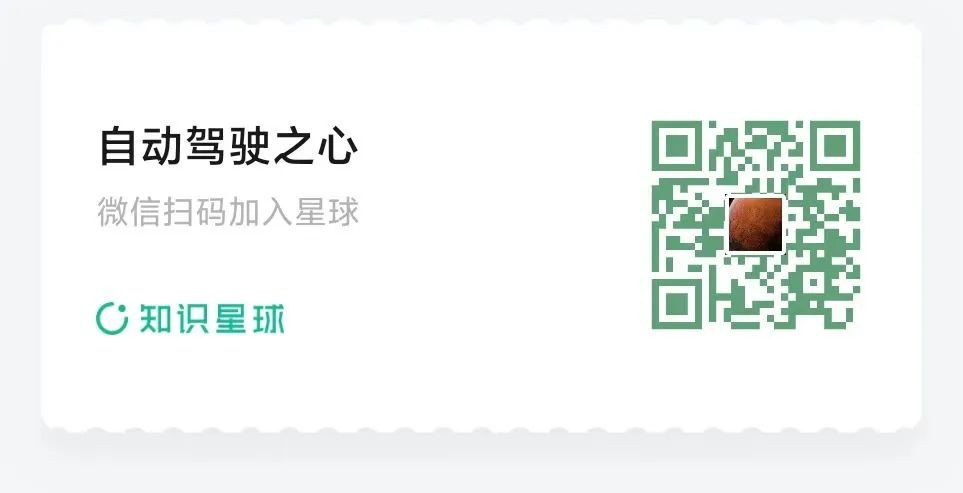
③【自动驾驶之心】技术交流群
自动驾驶之心是首个自动驾驶开发者社区,聚焦感知、定位、融合、规控、标定、端到端、仿真、产品经理、自动驾驶开发、自动标注与数据闭环多个方向,目前近60+技术交流群,欢迎加入!扫码添加汽车人助理微信邀请入群,备注:学校/公司+方向+昵称(快速入群方式)
④【自动驾驶之心】全平台矩阵