项目演示
GraspNet+YOLO
目录
3 下载GraspNet-baseline的权重(训练好的)
GraspNet计算抓取姿态
GraspNet能够基于点云数据生成抓取点及其对应的夹抓姿态,并输出置信度最高的结果,确保抓取的可靠性。
YOLO-World目标检测
YOLO-World是一种高效的目标检测模型,能够通过用户提供的文本提示识别任意目标类别。与传统的目标检测模型依赖于预定义的类别不同,YOLO-World 可以通过简单的文本提示,如 “检测飞机” 或 “查找街道上的汽车”,来实现对新物体的检测。
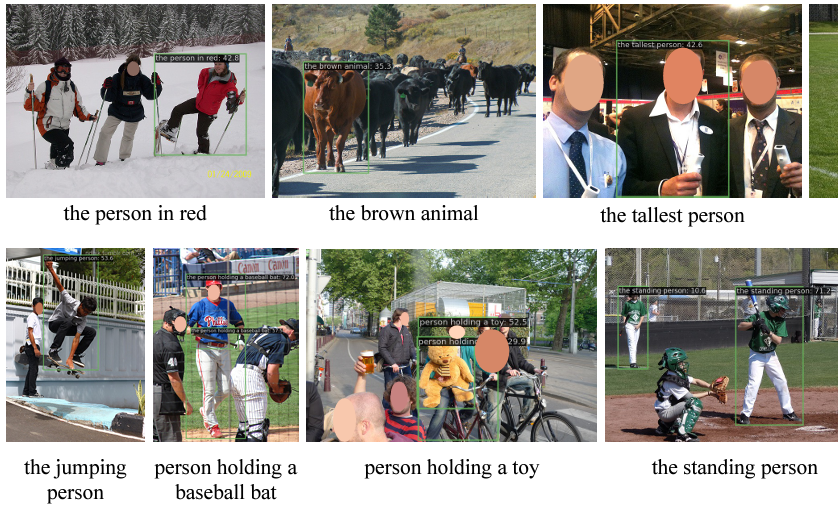
YOLO-World目标检测
SAM图像分割
SAM(Segment Anything Model)能够生成高质量的掩码图。是由MetaAI最新发布的一个图像分割领域的预训练模型。SAM的提出突破了分割界限,极大地促进了计算机视觉基础模型的发展。
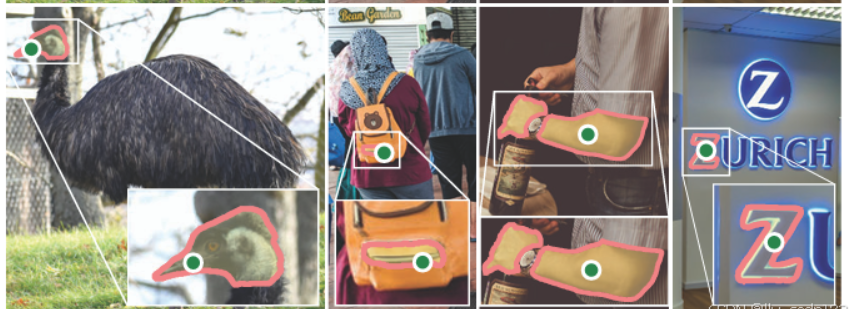
SAM图像分割
抓取仿真流程
UR5机械臂通过深度相机获取场景的RGB图像和深度图。使用YOLO-World模型对图像中的待抓取物体进行目标检测,定位物体的边界框。然后利用SAM对检测到的物体进行图像分割,生成高精度的掩码图。深度相机提取物体的三维点云数据,基于点云数据,通过GraspNet模型计算抓取点及其对应的夹抓姿态,并选择置信度最高的结果。最后UR5机械臂根据计算出的夹抓姿态执行抓取动作,完成整个抓取任务。
项目实现(以下步骤均在虚拟环境下执行)
1 环境配置
创建虚拟环境
conda env remove -n mujoco_graspnet
conda create -n mujoco_graspnet python=3.9
conda activate mujoco_graspnet
进入虚拟环境后下载开源manipulator_grasp项目
git clone https://gitee.com/chaomingsanhua/manipulator_grasp.git
cd manipulator_grasp
下载graspnet-baseline项目
GraspNet-Baseline 是一个开源的抓取算法基准模型,它是 GraspNet-1Billion 大规模抓取数据集的基线模型。
git clone https://github.com/graspnet/graspnet-baseline.git
cd graspnet-baseline
修改requirements.txt
sudo gedit requirements.txt
这里要指定numpy的安装版本为1.23.4。删除torch,我们后面自行安装
保存后执行安装指令
pip install -r requirements.txt
安装torch等所需的环境包
pip install torch==1.12.1+cu113 torchvision==0.13.1+cu113 torchaudio==0.12.1 --extra-index-url https://download.pytorch.org/whl/cu113
pip install spatialmath-python==1.1.14
pip install roboticstoolbox-python==1.1.1
pip install modern-robotics==1.1.1
pip install mujoco==3.3.1
2 编译并安装
2.1 编译并安装PointNet++自定义算子
- PointNet++是处理3D点云的经典神经网络,需要自定义CUDA算子实现高效采样和特征聚合
- VoteNet是基于PointNet++的3D目标检测框架
cd pointnet2
python setup.py install
cd ../
安装时发现报错:The detected CUDA version mismatches the version that was used to compile PyTorch.具体解决参考这篇文章:
报错解决:The detected CUDA version mismatches the version that was used to compile PyTorch._the detected cuda version (10.1) mismatches the ve-CSDN博客文章浏览阅读239次,点赞3次,收藏2次。Anaconda虚拟环境中,PyTorch版本与系统CUDA版本不匹配时,推荐在Conda虚拟环境中安装CUDA。确认问题已解决,这种方法避免了因Ubuntu版本限制而无法安装低版本CUDA的问题。_the detected cuda version (10.1) mismatches the version that was used to comhttps://blog.csdn.net/agentssl/article/details/147960670?spm=1001.2014.3001.5502若出现报错“cuda_runtime_api.h: No such file or directory.”参考这篇文章:报错解决:cuda_runtime_api.h: No such file or directory-CSDN博客文章浏览阅读242次,点赞9次,收藏5次。在conda虚拟环境中使用gcc编译依赖CUDA时报错fatal error: cuda_runtime_api.h: No such file or directory:使用conda安装的cuda是的,而精简版的cuda没有办法编译,会缺少头文件。缺少的头文件指的就是这部分提到的。而NVIDIA原版的CUDA Toolkit是完整版的CUDA,可以进行编译,且不缺少头文件。:将系统CUDA库的路径加入系统环境变量,使用系统CUDA的cuda_runtime_api.h(此解决方法同样适合。
https://blog.csdn.net/agentssl/article/details/147989459?spm=1001.2014.3001.5502
编译并安装基于PyTorch CUDA实现的k-最近邻(k-NN)算子:
- k-NN算子:用于快速计算数据点之间最近邻关系的算法模块
- CUDA加速:利用NVIDIA显卡进行并行计算加速
- PyTorch扩展:为深度学习框架添加自定义C++/CUDA操作
cd knn
python setup.py install
cd ../
安装graspnetAPI工具包
git clone https://github.com/graspnet/graspnetAPI.git
cd graspnetAPI
修改setup.py,将sklearn替换为scikit-learn
sudo gedit setup.py
修改后开始安装
pip install .
cd ../
3 下载GraspNet-baseline的权重(训练好的)
在manipulator_grasp文件夹新建logs/log_rs文件夹,将下载好的权重checkpoint-rs.tar放进log_rs,如图
4 运行manipulator_grasp项目
进入manipulator_grasp文件夹,运行主函数
python main.py
出现报错
Traceback (most recent call last): File "/home/song/hjx/manipulator_grasp/main.py", line 19, in <module> from graspnet_dataset import GraspNetDataset File "/home/song/hjx/manipulator_grasp/graspnet-baseline/dataset/graspnet_dataset.py", line 12,https://download.pytorch.org/whl/torch_stable.html ModuleNotFoundError: No module named 'torch._six'
修改graspnet-baseline/dataset/graspnet_dataset.py 即可。
# 原代码(旧版PyTorch)
from torch._six import container_abcs
# 修改为(适配PyTorch 2.x)
import collections.abc as container_abcs
修改后再次运行
关闭Open3D窗口,机械臂开始执行抓取,如下图所示
5 运行YOLO_World-SAM-GraspNet项目
安装额外的环境包
pip install ultralytics==8.3.98
pip install git+https://github.com/openai/CLIP.git
下载开源代码
这里感谢大佬分享https://pan.baidu.com/s/1NNeS5C_9irYVFHOGCWR0ZQ 提取码: rri4
运动代码过程中发现苹果抓取效果不是特别好,初步认为是因为位置摆放太远
修改苹果位置:
通过VScode打开项目,修改红框位置即可
进入YOLO_World-SAM-GraspNet文件夹运行:
python main_yoloWorld_sam.py
在命令终端输入待抓取物体,第一次运行命令会下载模型,如图所示
随后会生成目标检测结果、SAM掩码图以及预测抓取姿态
项目成功运行!
制作不易,对您有帮助的话就点赞收藏支持下,感谢感谢~
参考文章